Machine Learning Data Catalog: Evolution, Benefits, Business Impacts and Use Cases in 2024
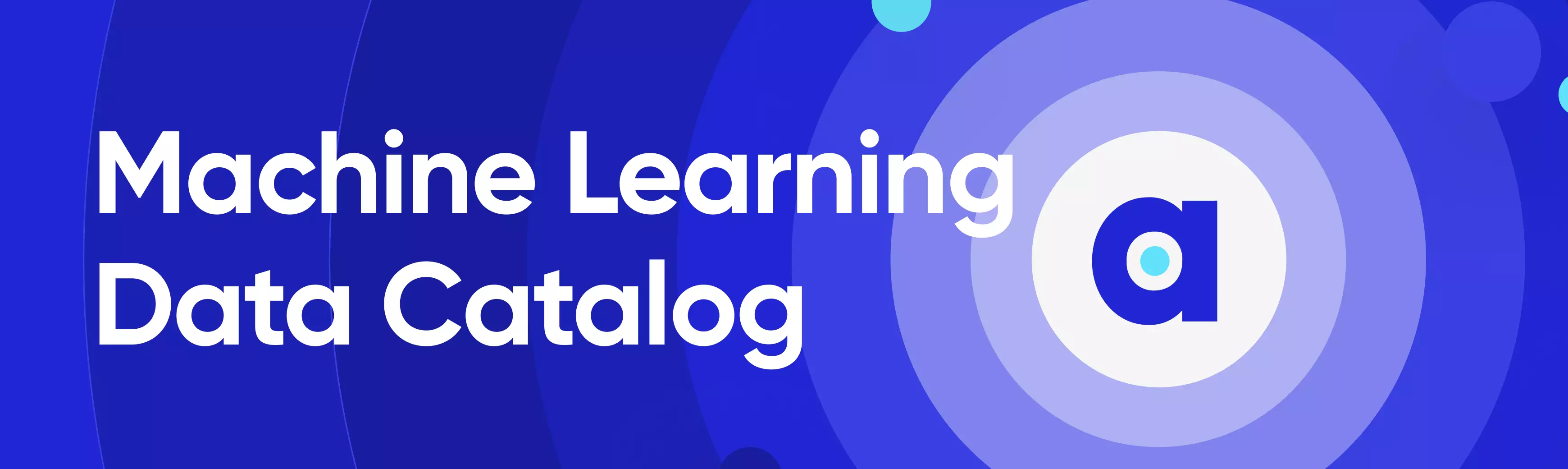
Share this article
A machine learning data catalog scours through your metadata to automate various aspects of data discovery, classification, profiling, and lineage. It saves time on tedious tasks and ensures consistent, accurate, updated, and easy-to-access data.
Recently, advancements in generative AI have disrupted content creation, healthcare, art, and music. It’s akin to the moment when the popularity of the internet changed our lives.
This has renewed interest in machine learning data catalogs and their potential to drive the most value out of your data estate.
Table of Contents #
- What is a machine learning data catalog?
- The advent of machine learning data catalogs
- AI data catalogs vs Automated data catalogs vs Machine learning data catalogs
- Business impact of machine learning data catalogs
- Key capabilities of machine learning data catalogs
- Machine learning data catalogs: Case studies
- Machine learning data catalog: Related reads
So, let’s understand the evolution of machine learning data catalogs, and then explore the business impact and use of machine learning data catalogs.
What is a machine learning data catalog? #
A machine learning data catalog is a next-generation data catalog that enables real-time data discovery and automates cataloging, crawling of metadata, and classification of PII data.
They uses metadata to automate recurring tasks in data discovery and cataloging. These tasks can include metadata discovery, data classification and tagging, automated quality audits, data profiling, and more.
According to Dave Wells, the Practice Director, Data Management at Eckerson Group, machine learning is essential to automate data discovery:
“Use of machine learning for metadata collection, semantic inference, and automated tagging is important to get maximum value from automation and to minimize the manual effort of data cataloging.”
G2 Grid® Report for Machine Learning Data Catalog Source: G2.
The advent of machine learning data catalogs #
Traditionally, data cataloging was all about setting up an inventory of your data. As the volume, velocity, and veracity of data grew, this wasn’t enough.
In 2017, 39% of the organizations surveyed by Forrester Analytics mentioned that sourcing, gathering, managing, and governing the data as it grows was a challenge.
Machine learning data catalogs were supposed to help organizations get value from their data quickly.
To understand the rise, fall, and resurgence of machine learning data catalogs, we’ll look at:
- The challenges of the machine learning data catalogs introduced in 2012
- A need for data cataloging with context and not just automated discovery
- The rise of active metadata management and its role in data cataloging
- The resurgence of machine learning data catalogs because of advancements in AI
1. The problem with machine learning data catalogs introduced in 2012 #
The problems with generating value from data persisted even after several machine learning data catalogs were introduced in 2012.
While machine learning helped get a clearer picture of the data within an organization, it didn’t fully address the challenges around data lineage and governance.
For instance, data catalogs had evolved to support the automatic collection and enrichment of metadata. These were called automated data catalogs. However, this still didn’t help in offering deep transparency into data flow and delivery.
Without knowing how data moves through or gets transformed across your data estate, it’s challenging to understand how it affects your workflows or predict its impact.
2. A need for data cataloging with context, not just automated discovery #
In early 2022, Forrester dropped machine learning in its Now Tech report on Machine Learning Data Catalogs.
The reasoning — provisioning data is more complex under a distributed cloud, edge computing, intelligent applications, automation, and self-service analytics use cases. Data teams need a data catalog that does more than generate a wiki about data and metadata.
G2 also noticed how the interest in machine learning data catalogs dropped in 2021-2022.
“While MLDC has helped companies immensely with data discovery, businesses are now looking for solutions that provide clarity to metadata. To ensure companies make the most of their MLDC offerings and provide useful business insights, we can use the third phase of machine learning data catalogs—active metadata management.”
3. The rise of active metadata management and its significance to data cataloging #
Active metadata facilitates the two-way movement of your metadata using open APIs that connect all the tools in your data stack.
An active metadata management platform enables this bidirectional metadata movement. It extracts metadata from different data sources, analyzes and enriches it, and then sends it back to the tools in your data stack.
Read more → Active metadata: Your 101 guide
In 2021-2022, a new category of data catalogs emerged to meet this need. G2 called them a building block of the DataOps architecture.
Gartner launched a market guide for active metadata management, calling it “an emerging set of capabilities across multiple data management markets.”
4. The resurgence of machine learning data catalogs because of advances in AI #
Recently though, the proliferation of AI has renewed interest in machine learning data catalogs. According to a 2022 McKinsey survey, AI adoption has more than doubled over the past five years.
The rise of generative AI tools, such as ChatGPT and DALL-E2, shows how the right training data can improve machine learning models’ accuracy. McKinsey explains the potential of these tools with the following examples:
- ChatGPT can produce a “solid A-” essay comparing theories of nationalism from Benedict Anderson and Ernest Gellner in ten seconds
- DALL-E can create strange, beautiful images on demand, like a Raphael painting of a Madonna and child eating pizza
While both tools have demonstrated their shortcomings, the possibilities are still enormous and endless. Machine learning data catalogs will also benefit from advancements in AI, allowing them to learn and evolve more efficiently.
All these advances have generated several categories of data catalogs. So, before proceeding further with machine learning data catalogs, let’s quickly look at the differences between these categories.
Are AI data catalogs, automated data catalogs, and machine learning data catalogs the same? #
AI data catalogs, automated data catalogs, and machine learning data catalogs are related but not the same.
AI data catalogs use artificial intelligence to mimic human intelligence. This can include using AI to ask questions, create documentation, generate SQL queries to explore data, and more.
Also read → 8 ways AI-powered data catalogs save time spent on documentation, tagging, querying, and more
Automated data catalogs use algorithms to automate recurring tasks. This can include automatically classifying and organizing data sets, performing quality checks, generating logs, and more.
Once you set up a script, they run continuously to fulfill the conditions of that script. However, unlike AI data catalogs, they won’t analyze your data to offer suggestions or recommendations for improvement.
Machine learning data catalogs are a type of AI data catalog. Google Cloud elaborates on the difference between AI and ML as follows:
“While artificial intelligence encompasses the idea of a machine that can mimic human intelligence, machine learning does not. Machine learning aims to teach a machine how to perform a specific task and provide accurate results by identifying patterns.”
Chatbots are an example of AI, whereas search engines are examples of machine learning in action.
What is the business impact of machine learning data catalogs compared to traditional data catalogs? #
Traditional data catalogs were built for handling structured data. They weren’t equipped to handle unstructured data (text, images, videos). They also didn’t capture all kinds of metadata and still relied on manual tagging and classification.
Machine learning data catalogs can deal with data from various sources and formats. They can capture all kinds of metadata in addition to technical metadata, such as usage or collaboration metadata.
Also read → The six types of metadata and their use cases
Furthermore, machine learning data catalogs can automatically classify, tag, and profile data assets, and help maintain consistency to provide a common understanding of data.
As a result, the business impact of machine learning data catalogs can be significant in terms of:
- Faster insights with automated data discovery and search
- Greater efficiency by identifying potential data pipeline issues beforehand
- Ensure better regulatory compliance with data consistency
Let’s see how.
Faster insights with automated data discovery and search #
As mentioned earlier, machine learning data catalogs can scour through your data estate and help organize it with the right classifications or tags. This can lead to substantial time savings. Let’s look at an example from the pharmaceutical industry to understand this correlation.
The Toronto-based BenchSci wanted to speed up its drug development process.
In a study published by HBR, BenchSci realized that using machine learning to read, classify, and present insights halved the number of experiments typically required to advance a drug to clinical trials. The impact of the time saved also affects costs — HBR states that it adds up to potential savings of over $17 billion annually.
Similarly, machine learning data catalogs can help data teams reduce the time spent looking for and organizing data by automating metadata curation from various sources, data asset classification, and data profiling.
They can also help data practitioners simplify data search and discovery with a keyword-based search engine that lets them look for the data they need. Think of it as what Google has been doing for the internet.
Greater efficiency by identifying potential data pipeline issues beforehand #
The goal of any machine learning system is to learn from data. This can be extremely useful in dealing with crises, as machine learning can help you identify the triggers and act immediately.
BlueDot, a Canadian start-up, used AI and ML to detect disease outbreaks.
According to an article from the World Economic Forum, BlueDot used machine learning algorithms to sift through news reports in 65 languages, along with airline data and animal disease networks, to detect outbreaks and anticipate disease dispersion. Epidemiologists then reviewed those results.
Similarly, machine learning data catalogs can study existing data pipelines to help your engineers identify potential issues whenever they make changes or adjust their workflows. The catalogs can also help your team spot the downstream applications affected and notify the relevant people immediately.
This ensures that your data practitioners are well-equipped to tackle potential issues and be more efficient with their daily workflows.
Ensure better regulatory compliance with data consistency #
Machine learning can help you classify and tag data automatically according to its content and context. This ensures data consistency and develops a shared understanding of all data assets in your organization.
Let’s consider the healthcare industry, for instance. Machine learning models can be trained to recognize confidential or restricted patient data —medical diagnoses or Social Security numbers — and automatically classify it.
Likewise, machine learning data catalogs can analyze existing metadata on sensitive data, and then automatically label similar data assets across multiple sources as Personally Identifiable Information (PII). This ensures that data assets are consistently organized.
Machine learning data catalogs can be configured to identify other potential compliance issues and to help you take proactive steps to mitigate those risks.
This way, you can avoid costly penalties, damage to your brand’s image, and other consequences of non-compliance.
What are the key capabilities of machine learning data catalogs? #
While the essential capabilities of machine learning data catalogs will keep evolving, here are five attributes that you should look for:
- Automated data tagging
- Automated, 360-degree data profiling
- Google-like semantic search for all data assets
- Automated, cross-system data lineage mapping
- Automated quality audits
Automated data tagging #
A machine learning data catalog should automatically crawl metadata for data assets across all sources and label them with relevant tags.
Regardless of where the data comes from (cloud warehouses, data lakes, or RDBMS), the catalog must be able to crawl metadata and, using machine learning algorithms, identify the key attributes of each asset.
Once the attributes have been identified, the catalog should be able to automatically assign relevant tags to each data asset. This makes it easier for users to search for and find the data they need.
Auto-tagging also helps in identifying relationships between different data assets. For instance, if two data assets have the same tag, then they could be related. By identifying these relationships, the catalog can help data practitioners spot patterns and get insights that may not have been obvious before.
Automated, 360-degree data profiling #
After crawling the metadata, a machine learning data catalog should auto-populate complete data asset profiles containing all the information available for each asset. This information could include:
- Number of rows and columns
- Connections
- Asset description
- Certification status (verified, work in progress, or deprecated)
- Asset owner
- Lineage view
- README
- Column preview
By automatically generating complete data asset profiles, the machine learning data catalog provides a centralized location for all data assets, making it easier for data practitioners to find and access data sets with adequate context.
Google-like semantic search for all data assets #
A machine learning data catalog should offer visual and intuitive searches like search engines. The search should let you look up all kinds of data assets, such as columns, databases, SQL queries, BI dashboards, and more.
This ensures that anyone can look up the data they need without running complex SQL queries or relying on data engineers.
It should also offer filters to help you fine-tune the search results and display similar search terms to help you expand your research and discover all relevant data assets.
Automated, cross-system data lineage mapping #
A machine learning data catalog should parse through your query logs across data sources automatically to create a visual map of data flows through your data estate.
The catalog should track every transformation a data set undergoes — including its movement across systems — and represent it graphically to help users map its lineage.
This visual map can help data practitioners better understand the relationships between data sets, leading to better insights and outcomes.
Automated quality audits #
Running scheduled audits can help you spot data regressions, data loss, or distributional shifts over time and can help certify data accuracy.
Automated quality audits use machine learning algorithms to detect anomalies in the data, such as missing values, outliers, and inconsistencies. These algorithms can also identify patterns and trends in the data, making it easier for data practitioners to identify potential issues and take appropriate action right away.
This reduces the risk of errors and inaccuracies and helps you ensure that your data is accurate and up-to-date.
Machine learning data catalogs: Case studies #
Machine learning data catalogs can help data practitioners:
- Find the data they need by scouring through their data estate
- Tag, categorize, and classify data automatically
- Identify and tag sensitive or confidential data and enforce the required data access controls automatically
- Spot and offer suggestions to fix inconsistencies in the data
- Track the lineage of data, from its source to its final use
- Set up a centralized platform for storing, sharing, and collaborating on data
Let’s look at two case studies to understand the unlimited potential of machine learning data catalogs.
How an insurance firm solved its data discovery woes using a machine learning data catalog #
The California-based CSE Insurance was grappling with siloed data assets and inconsistent documentation efforts. The company was an early adopter of the modern data stack and needed a modern data catalog powered by machine learning to solve its data woes.
According to a data analyst at CSE Insurance, looking for the right data took hours:
“When I searched for a column, the same one would appear in multiple tables. I used to go through every column in every table to find the right fit. It would take hours.”
The company decided to set up Atlan — a leader in G2’s Grid® for Machine Learning Data Catalogs. With Atlan’s Google-like search interface, an analyst can find the information they need within minutes, as each asset has a comprehensive, shareable 360° data profile.
They could share these asset profiles with anyone — technical and business users. This helped in quickly understanding data and using it to get meaningful insights.
Read more → How CSE Insurance cultivates a data-driven culture with Atlan
How a non-profit healthcare organization used a machine learning data catalog for data governance and security #
The San Diego-based Scripps Health was struggling with getting enterprise-scale visibility into what they were developing.
They needed a Google or Wikipedia-like search tool to easily see stats on their source systems and metadata. They also required a platform that ensured HIPAA compliance, protecting sensitive healthcare records.
Scripps Health chose Atlan as it integrated natively with Snowflake, so the data teams spent less time investigating — what report used a certain table, when was an asset last updated, or which process loads what data — and more time developing.
Moreover, Atlan let them propagate custom classifications downstream and upstream so that they could secure sensitive data with appropriate masking and hashing policies. For example, Atlan automatically applies the relevant access policies whenever an asset is tagged as PII.
Read more → Why a $3 billion healthcare provider chose Atlan as its modern data catalog
So, what’s next? #
The evolution of machine learning data catalogs has been driven by a need for effective data cataloging with context, not just automated discovery.
With the ability to automate data and metadata discovery, tagging, and profiling, machine learning data catalogs can help you get valuable insights quickly and improve the overall efficiency of your data teams.
As the category continues to evolve, we’re excited to see how machine learning data catalogs will transform our data landscapes and the scope of data management.
Machine learning data catalog: Related reads #
- Data Catalog: The Must-Have Tool for Data Leaders in 2024
- AI Data Catalog: Exploring the Possibilities That Artificial Intelligence Brings to Your Metadata Applications & Data Interactions
- 8 Ways AI-Powered Data Catalogs Save Time Spent on Documentation, Tagging, Querying & More
- 15 Essential Data Catalog Features to Look For in 2024
- Top Data Catalog Use Cases Intrinsic to Data-Led Enterprises
- Business Data Catalog: Users, Differentiating Features, Evolution & More
Share this article