Is Your Data AI-Ready? Here’s What You Need to Know
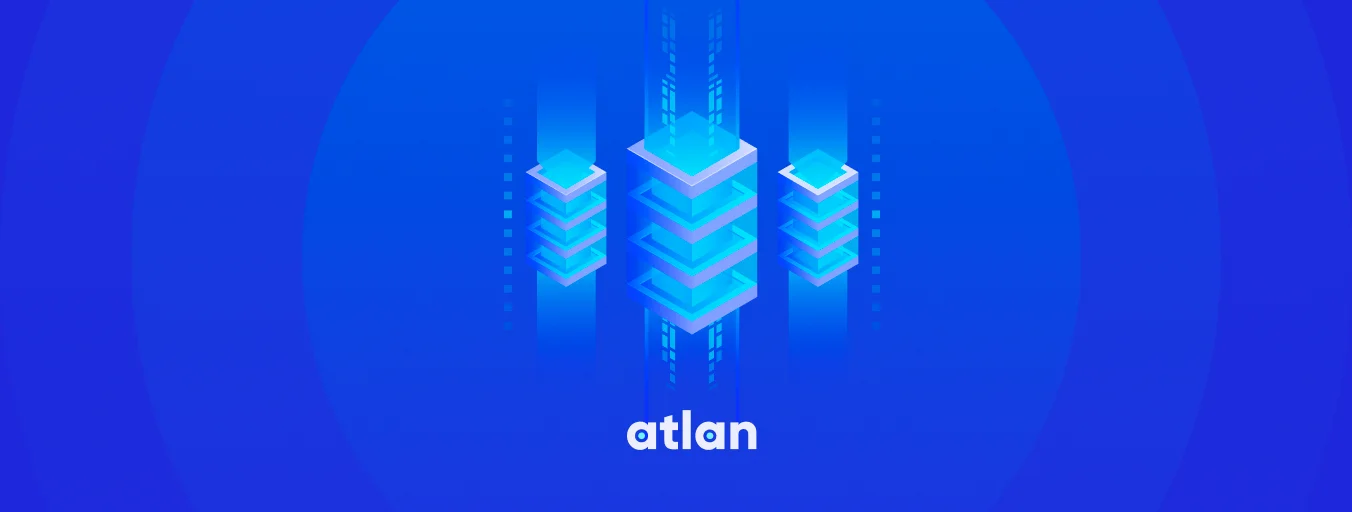
Share this article
In recent years, AI adoption has more than doubled across industries, according to a McKinsey survey on the state of AI. IBM’s 2023 CEO study highlights how 75% of the CEOs surveyed believe competitive advantage will depend on who has the most advanced generative AI.
However, there’s no success with AI without AI-ready data. Gable’s CEO Chad Sanderson uses a healthy diet as an analogy for data — if garbage goes in, then garbage comes out.
AI-ready data is well-governed, secure, free of bias, enriched, accurate, and of high quality.
In this article, we’ll look at the concept of data readiness for AI and explore critical factors that make your data ready for generative AI systems.
Table of contents #
- What is data readiness for AI?
- What does AI-ready data look like?
- Is your data AI-ready?
- How to ensure data readiness for AI?
- Wrapping up
- Related Reads
What is data readiness for AI? #
Data readiness for AI is the process of preparing your data for generative AI. For generative AI to be effective, your data must be:
- Understandable with the right context
- Of high quality — accurate, complete, consistent, timely, unique
- Well-governed to support ethical and compliant use of data
- Available, discoverable, and accessible
What does AI-ready data look like? 4 factors to consider #
The standards defining AI readiness are still evolving, with each expert and analyst coming up with their own set of standards.
For instance, McKinsey highlights that AI-ready data is data that’s:
- Known
- Understood
- Available
- Fit for purpose
- Secure
Meanwhile, Gartner states that AI-ready data is data that’s:
- Ethically governed
- Secure
- Free of bias
- Enriched
- Accurate
So, here’s how we interpret AI-readiness — AI-ready data doesn’t live in siloes. It’s readily available and accessible (to the right folks) in a unified data platform. This platform helps you visualize lineage, i.e., how data flows through your systems, where it originates, how it gets transformed, etc.
The data platform also offers adequate context with active metadata — ensuring your data ecosystem is always on, intelligent, and action-oriented. With this metadata, AI systems can deliver business value.
Lastly, there’s a solid AI data governance program in place to ensure the ethical, secure, and responsible use of AI-ready data.
That’s why the critical factors for AI-ready data are:
- Metadata management
- Data and metadata quality management
- Data lineage
- Data governance
Let’s explore each factor further.
1. Metadata management #
Metadata management is at the heart of ensuring data readiness for AI. Metadata offers context on data, helping you understand what it means and how to use it.
It supports everything from data discovery and context to quality and lineage.
Organizing the right metadata using a set of pre-defined metadata standards in a single location is essential to streamline your metadata management efforts.
Other essential elements include:
- 360° view of each data asset to get all the context in one location
- End-to-end active, actionable data lineage to understand how data flows through your systems
- A connected, semantic layer that helps create and explore relationships between definitions, metrics, and assets
- Access controls that are personalized — defined according to roles, business domains, or project context
These elements will help generative AI models effectively understand data assets and provide useful recommendations.
“If, as predicted, LLMs will soon be functioning as ‘agents’ for human users, delivering answers and results in response to natural-language queries and instructions, the LLMs not only need access to all relevant data, but to information about the data that gives it context and meaning. Without excellent metadata management, it will be difficult or impossible for LLM agents to be effective.” Jonathan Sims, Consultant at Cognizant
2. Data and metadata quality management #
As mentioned earlier, AI-assisted systems need high-quality data to be useful. So, your data assets must be continuously evaluated according to the most important data quality measures, such as relevance, reliability, accuracy, etc.
Read more → Data quality measures 101
However, an oft-sidelined aspect here is metadata quality.
“In this coming era of AI and LLMs, metadata quality will be as important as data quality. LLM applications need rich, high quality metadata in order to use data.” David Jayatillake, Co-Founder & CEO @ Delphi
So, your data management practices should include data and metadata quality measures. It’s also crucial to prioritize measures to help avoid biases, as that will undermine the results you get from AI.
“The more accurate and trustworthy the data, the more reliable the A.I.-generated answers.” Steve Lohr, The New York Times reporter on technology and its impact on the economy
3. Data lineage management #
An HBR article refers to an ‘ontology’—a way of mapping data assets and their relationships—being essential to developing AI systems.
“An ontology is a consistent representation of data and data relationships within your business. [Without that], AI systems can only develop in a piecemeal, fragmented way — they will lack the underpinning that would allow them to be smart enough to make an impact.” Harvard Business Review
In other words, an essential part of ensuring data readiness for AI would be data lineage — a visual representation of how data flows through your data estate.
Read more → Column-level lineage for better data quality and visibility
4. Data governance management #
Steve Lohr from The New York Times calls data a bottleneck for big businesses in their race to build AI programs. Data without standards, context, or ownership is a major hurdle to generating value from AI systems.
“Without a system of data ownership and change management, your models will be constantly hallucinating, regularly breaking, and consistently failing to deliver the business value companies expect.” Chad Sanderson, CEO of Gable.ai
That’s where data governance can help. A framework that establishes AI standards and policies ensures ethical and compliant AI practices and supports responsible data stewardship is vital.
Such information will help in setting up an environment where there’s complete transparency and trust in data.
Read more → Ensuring efficient data governance: Everything you need to know
Various experts interpret this need differently.
For instance, Steve Lohr from The New York Times calls it a data-labeling system. He reports on data provenance standards being developed to describe the origin, generation method, history, lineage mapping, and legal rights to data.
“The data-labeling system (i.e., data provenance standards) will be similar to the fundamental standards for food safety that require basic information like where food came from, who produced and grew it and who handled the food on its way to a grocery shelf.” Steve Lohr in an article for The New York Times
While data provenance usually involves lineage mapping, establishing provenance standards should be a part of your data governance program.
Ultimately, data governance that ensures the security of your data, the safety of user interfaces, and testing standards to maintain trust is vital before implementing AI use cases.
Read more → Data governance for AI
Is your data AI-ready? #
“While AI dominates the headlines, there is consensus on the following: data maturity necessarily comes before AI maturity.” Harvard Business Review
Before you consider data readiness for AI, you should assess your data ecosystem. If your current data estate is in chaos and there’s a lack of trust in using it for decisions, then your organization is not ready for AI.
Let’s look at some common challenges that act as barriers to adopting AI.
The 2023 CEO survey by The IBM Institute for Business Value, in cooperation with Oxford Economics, lists concerns around data lineage, security, and compliance as prominent issues faced.
Barriers to AI adoption, according to IBM’s 2023 CEO survey - Source: IBM.
Meanwhile, an article in Harvard Business Review lists siloed data as the biggest hurdle to AI-readiness:
“Every one of our competitors and most of the organizations of our size in other industries have spent at least a few million dollars on failed AI initiatives. Why? … because promises of AI vendors don’t pay off unless a company’s data systems are properly prepared for AI.”
Data quality is also a key challenge — incomplete or missing data, inaccuracies and inconsistencies, etc. affect the outcomes of AI-assisted systems. If the training data is of poor quality, so will be the outcomes.
A Gartner press release on AI ambition and readiness echoes similar sentiments — your data is AI-ready only when it’s fair, accurate, and governed by the lighthouse principles.
Here’s how Mary Mesaglio, Distinguished VP Analyst at Gartner, defines these lighthouse principles:
”To navigate decisions about AI in their organization, CIOs and IT leaders need lighthouse principles — a vision for AI that lights the way and says what kind of human-machine relationships they will and will not accept.”
To conclude, if your data is siloed, not transparent, not properly governed and documented, it isn’t ready for AI.
How to ensure data readiness for AI #
On surveying CIOs and data leaders in 2023, Gartner found that only 9% have an AI vision statement in place, and more than one-third had no plans to draft an AI vision statement.
The first step to ensuring data readiness for AI is having an AI vision statement and the lighthouse principles in place. These principles will dictate how AI-powered systems engage with and use your data.
The next step is to create an environment promoting secure, compliant, and unbiased use of your data for AI-assisted decision-making. Here’s how Gartner emphasizes security, especially for data practitioners using public AI solutions:
“For every positive use of AI, someone is putting that same technology to negative use. This is the dark side of AI. CIOs should prepare for new attack vectors and work with the executive team to create an acceptable use policy for public generative AI solutions.”
Ensuring data readiness for AI: 7 guidelines to follow #
As mentioned earlier, your data must already be generating business value for it to be considered AI-ready. Here are a few guidelines to ensure data readiness for AI:
- Identify AI-specific use cases
- Set up a single source of truth supporting automation and generative AI
- Make sure that you have a solid data governance framework in place
- Ensure the security, integrity, and privacy of your data estate to avoid data leaks, breaches, and non-compliance
- Enrich your data assets with proper context — active metadata management, business glossary, classification and tagging, etc.
- Set up and track data quality metrics continuously to ensure that your data is valuable, accurate, and trustworthy
- Make your data estate observable so that you have mechanisms in place to fix data issues before they affect your pipelines — data observability and cross-system, column-level lineage can help you with data quality and governance
These guidelines are all interconnected as data that is well-documented and observable is already of better quality and value. These, in turn, support your data governance efforts. Together, they ensure data readiness for AI.
Wrapping up #
To sum things up, achieving data readiness for AI means ensuring your data is secure, accurate, free of bias, enriched, available, accessible, and of high quality. Critical factors include metadata management, quality management, data lineage, and governance.
By ensuring these factors and following the above guidelines, you can help generative AI models understand data assets and provide useful recommendations.
Data Readiness for AI: Related Reads #
- Data Governance for AI
- AI Data Catalog: Its Everything You Hoped For & More
- 8 AI-Powered Data Catalog Workflows For Power Users
- AI Data Governance: Why Is It A Compelling Possibility?
- Atlan AI for data exploration
- Atlan AI for lineage analysis
- Atlan AI for documentation
- What is Active Metadata? — Definition, Characteristics, Example & Use Cases
Share this article