Rigorous Data Management: How to Go About It in 2024?
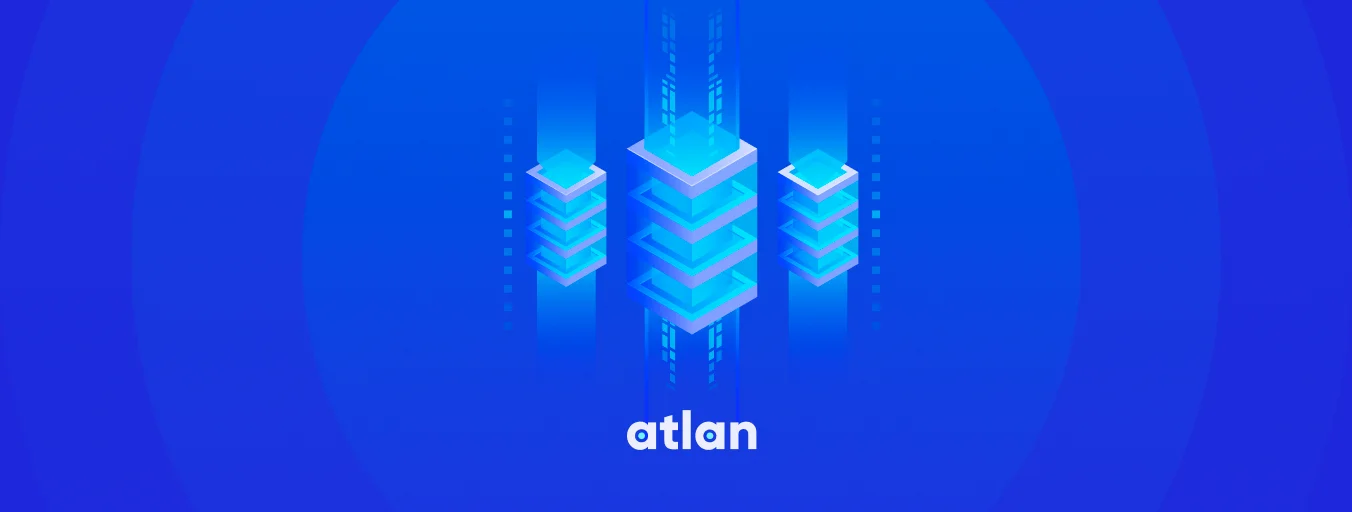
Share this article
Having a sea of data isn’t valuable unless it’s managed effectively. Poor data management not only costs time and resources, but it can also result in inaccurate analyses, compliance risks, and missed opportunities. Rigorous data management addresses these pain points by ensuring data quality, accessibility, and security.
It streamlines decision-making, enhances compliance with regulations like GDPR, and unlocks the full potential of data-driven insights.
Modern data problems require modern solutions - Try Atlan, the data catalog of choice for forward-looking data teams! 👉 Book your demo today
But what makes it “rigorous”?
In this article, we will understand the term rigorous, and also learn how this rigorous data management ensures that the data you manage is complete, accurate, and readily available for use. So, let’s dive in!
Table of contents #
- What is rigorous data management?
- Why is rigorous data management key to reducing claims fraud?
- 8 Types of rigorous data management systems
- What does a rigorous data management team do?
- The 10 pillars of rigorous data management systems
- 5 Proven rigorous data management tools to elevate your data game
- 9 Steps to overcome rigorous data management challenges
- Conclusion
- Related reads
What is rigorous data management? #
Rigorous data management refers to the meticulous process of handling, organizing, and maintaining data. This rigorous approach ensures data completeness, meaning every data piece is present, accurate, and usable.
When we talk about data management, it isn’t merely about storing data. It’s about maintaining its quality, security, and accessibility.
Simply put, effective data management turns raw data into a strategic asset, empowering organizations to make informed decisions, innovate, and maintain a competitive edge.
Why is rigorous data management key to reducing claims fraud? #
Rigorous data management is pivotal in reducing claims fraud because it ensures the integrity, accuracy, and timeliness of data, which are crucial for effective fraud detection.
By ensuring that the data is of high quality, organized, and easily accessible, rigorous data management acts as the backbone of any robust anti-fraud system.
Approaches to using rigorous data management for reducing claims fraud are:
- Data cleansing
- Data integration
- Automated flags
- Machine learning algorithms
- User access management
- Regular audits
- Continuous monitoring and updating
Let us understand each of them in detail:
1. Data cleansing #
The first step in any data management process geared towards fraud detection is ensuring that the data is clean and formatted correctly. This makes subsequent analysis more reliable.
By standardizing and cleaning your data, you establish a reliable foundation upon which fraud detection algorithms can operate.
2. Data integration #
Combining data from various sources (e.g., claims data, customer interaction data, third-party databases) can provide a more comprehensive view of each claim, making it easier to spot inconsistencies or anomalies.
A comprehensive dataset allows for deeper analysis and more robust detection algorithms, helping to identify inconsistencies or anomalies that may indicate fraud.
3. Automated flags #
Setting up automated flags for certain types of behaviors or claims characteristics (e.g., multiple claims in a short period, unusually large claims, etc.) can help in identifying potentially fraudulent activity for further investigation.
These flags serve as an early warning system for potentially fraudulent activities, facilitating timely investigation.
4. Machine learning algorithms #
With a well-managed and clean dataset, machine learning models can be more effectively trained to recognize patterns indicative of fraud.
These algorithms can be finely tuned to reduce false positives while increasing detection rates, automating the initial stage of fraud identification before human investigators take over.
5. User access management #
Limiting who can access what data can reduce the risk of internal fraud.
This can prevent internal fraud and also reduce the likelihood of accidental data corruption, which could impair your fraud detection capabilities.
6. Regular audits #
Periodic checks and audits of the data and the fraud detection mechanisms can ensure that they remain effective over time.
Audits also serve to verify that the data being used remains of high quality over time.
7. Continuous monitoring and updating #
Fraudsters continuously evolve their tactics, and the data management strategy should evolve accordingly. This includes regular updates to algorithms, data sources, and flagging criteria.
This keeps your fraud detection measures agile and responsive to emerging threats.
By employing these approaches under the umbrella of rigorous data management, organizations can significantly reduce the risks and costs associated with claims fraud.
8 Types of rigorous data management systems #
In today’s digital-driven landscape, understanding the intricacies of data management becomes imperative. Rigorous data management isn’t just a singular process—it’s a multifaceted discipline, each with its nuances.
As we venture into these types, we’ll uncover their distinct roles and the collective synergy they offer to harness data’s full potential.
The types of rigorous data management include:
- Database management
- Data warehousing
- Data security management
- Data quality management
- Master data management (MDM)
- Data governance
- Data analytics and business intelligence (BI)
- Data integration
Let’s look into each type of rigorous data management in brief:
1. Database management #
Database management isn’t just about storing data; it’s an intricate art of organizing and structuring it. This system ensures that data is stored efficiently, can be retrieved quickly when needed, is kept secure from threats, and remains consistent and accurate over time.
With optimal database management, businesses can maintain a smooth flow of information.
2. Data warehousing #
Beyond mere storage, data warehousing is about centralizing data from diverse sources. This structure aids in consistent data processing, making it easier for analysts to derive insights.
By having a unified repository, businesses can ensure data consistency and simplify their analytical processes, making decision-making swifter and more informed.
3. Data security management #
In our interconnected world, data’s sanctity is always at risk. Data security management is a vigilant process, guarding against unauthorized accesses, potential breaches, and unexpected data losses.
It’s a continuous endeavor, adapting to new threats and ensuring data remains in trusted hands, maintaining its integrity and confidentiality.
4. Data quality management #
Raw data often comes with inconsistencies, inaccuracies, and redundancies. Data quality management ensures data is not just available but is reliable and accurate.
By constantly refining, cleaning, and validating data, businesses ensure that their decisions are based on trustworthy information, minimizing errors and improving outcomes.
5. Master data management (MDM) #
At the heart of every organization is its master data—core data that remains consistent across different departments. MDM ensures this data is uniform, accurate, and consistently available, reducing discrepancies and confusion.
It acts as the bedrock, providing a single version of truth for critical organizational data.
6. Data governance #
Ensuring data’s quality isn’t a one-time task; it requires a robust framework. Data governance lays down the guidelines, protocols, and policies for data management across an organization.
By outlining clear responsibilities, procedures, and standards, it ensures that data is used and managed effectively and ethically.
7. Data analytics and business intelligence (BI) #
Beyond storage and management, data’s real power is harnessed when it’s analyzed. Data analytics and BI convert raw data into meaningful insights, helping businesses anticipate trends, understand consumer behaviors, and make predictive decisions.
With the right tools, raw data transforms into actionable strategies.
8. Data integration #
In our diverse digital ecosystem, data often resides in silos across multiple platforms. Data integration bridges these gaps, allowing different data sources to communicate and provide a holistic view.
This interconnectivity ensures that businesses can draw insights from a comprehensive dataset, rather than fragmented pieces.
Data management’s vastness is its very strength, offering businesses tools and methodologies tailored to distinct needs. As data continues to burgeon, understanding these domains becomes non-negotiable.
The journey through the different facets of data management reminds us that in this age, effective data harnessing is not just about collection, but about quality, integration, analysis, and security.
What does a rigorous data management team do? #
A rigorous data management team focuses on several key activities to ensure the quality, security, and usability of an organization’s data assets.
Below are some of the essential responsibilities and tasks that such a team would typically undertake:
1. Strategy and governance #
- Establish data governance framework: The team sets up a governance framework that outlines data policies, procedures, and responsibilities. This ensures that data is managed in a way that is compliant with internal guidelines and external regulations.
- Define data ownership and stewardship: The team identifies who within the organization is responsible for various types of data and what processes they must follow for its upkeep.
2. Data quality #
- Data cleansing: The team is responsible for identifying and correcting errors, inconsistencies, and inaccuracies in data. This may involve manual reviews as well as automated processes.
- Data validation: The team establishes rules and processes for validating incoming data to ensure that it meets predefined quality criteria.
- Metadata management: Metadata, or “data about data,” is meticulously managed to provide context that makes the data easier to understand, categorize, and use.
3. Data security #
- Access control: The team implements security measures, such as role-based access controls, to ensure that only authorized individuals can view or modify certain data.
- Data encryption and masking: Sensitive data is encrypted and, where necessary, masked to protect it from unauthorized access.
- Security audits: Regular audits are performed to identify potential vulnerabilities and to ensure that existing security measures are effective.
4. Data operations #
- Data extraction, transformation, and loading (ETL): The team manages the ETL process, which involves extracting data from various sources, transforming it into a format suitable for analysis, and then loading it into a data warehouse or database.
- Data integration: Data from disparate sources are integrated into a unified view, making it easier to draw insights from them.
5. Lifecycle management #
- Data archiving and retention: Policies are established for how long different types of data should be stored and when they should be archived or deleted.
- Data backup and recovery: The team is responsible for ensuring that critical data is regularly backed up and can be quickly recovered in the event of a system failure or data loss incident.
By managing these various aspects of data management rigorously, the team helps the organization to harness its data effectively, securely, and responsibly, enabling better decision-making and operational efficiency.
The 10 pillars of rigorous data management systems #
In an age where data is the new currency, understanding the mechanisms behind its management is imperative. But beneath the umbrella of data management lies a sophisticated architecture of systems and structures, diligently working in harmony. Let’s journey through the foundational pillars that not only manage but amplify the potential of our data.
The pillars of rigorous management systems are:
- Centralized vs. decentralized databases
- Evolution of data security protocols
- Importance of quality assurance mechanisms
- The future of scalable storage solutions
- Navigating through backup and recovery systems
- Compliance in a globalised world
- Harnessing advanced analytical engines
- The rise of integration and interoperability modules
- The role of metadata in data management
- Embracing automation in data management
Let’s look into each of the above pillars in brief:
1. Centralized vs. decentralized databases #
Centralized databases act as a hub, consolidating data into a single point, ensuring uniformity, and simplifying data access. Decentralized databases spread data across different locations or networks.
While they promote data democratization and resilience against localized failures, they require specialized syncing techniques and strategies to prevent data fragmentation and inconsistencies.
2. Evolution of data security protocols #
Modern data security protocols aren’t static; they’ve evolved to anticipate emerging threats. With the rise of cyberattacks, these protocols now employ multi-layered encryption, zero-trust frameworks, biometric authentication, and behavioral monitoring.
Each layer is designed to thwart unauthorized data access, ensuring a fortress-like security posture.
3. Importance of quality assurance mechanisms #
Data quality is the backbone of sound decision-making. Rigorous QA mechanisms like validation checks, routine data audits, and anomaly detection are indispensable.
These mechanisms root out inaccuracies, standardize data entries, and ensure data remains pristine, thereby fortifying its reliability across all applications.
4. The future of scalable storage solutions #
Modern businesses can’t be shackled by storage limitations. As we produce more data than ever, scalable storage solutions, encompassing cloud, hybrid, and edge architectures, are rising.
They offer on-demand scalability, ensuring businesses don’t overpay for storage and yet can scale up seamlessly during data surges.
5. Navigating through backup and recovery systems #
Modern backup systems are more than just data replicas. They encapsulate incremental backups, point-in-time recovery, and geo-redundant storage.
Coupled with advanced recovery mechanisms, they ensure businesses can bounce back from both minor data mishaps and catastrophic failures, safeguarding business continuity.
6. Compliance in a globalized world #
As data becomes global, so do compliance challenges. It’s a maze of laws, standards, and norms. Ensuring adherence to varied data protection regulations means implementing robust data classification, regionalized storage, and regular compliance audits.
It’s not just about legal protection but also about maintaining customer trust across geographies.
7. Harnessing advanced analytical engines #
Traditional analytics scratched the surface; today’s engines dive deep. With the infusion of AI and ML, these engines can predict trends, uncover hidden patterns, and even suggest actionable insights.
They transform raw data into strategic gold, driving innovation and competitive advantage.
8. The rise of integration and interoperability modules #
In an interconnected tech world, silos are detrimental. Data management systems now come equipped with APIs, connectors, and integration modules.
These ensure seamless data flow across disparate platforms, from CRMs to ERPs, creating a unified data ecosystem that promotes holistic insights.
9. The role of metadata in data management #
Metadata is the essential “data about data” that offers context to stored information. It streamlines data discovery, ensuring users pinpoint relevant datasets efficiently.
Metadata’s traceability feature lets users understand data’s origin and transformations, reinforcing data integrity and trustworthiness. Effective metadata management involves careful curation, structured storage, and user-friendly search tools.
10. Embracing automation in data management #
The constant influx of data today necessitates automation for efficient data management. Automation ensures speed and accuracy in routine tasks like ingestion and transformation.
By automating repetitive tasks, such as tagging and backup, potential manual errors decrease. This shift not only enhances efficiency but redirects human resources towards strategic, value-driven activities.
Data, in its vastness, offers infinite possibilities. Yet, harnessing its potential requires more than just tools—it requires systems and strategies crafted with precision.
5 Proven rigorous data management tools to elevate your data game #
In the dynamic realm of data management, having the right tools and techniques at your disposal can be a game-changer. As data volumes surge and complexities rise, traditional methods alone don’t suffice. Instead, businesses and data professionals require a modern arsenal, tailored for today’s challenges.
Let us look at the proven tools and techniques in a tabular format:
Sl no. | Tools | Description |
---|---|---|
1. | Data integration tools | Solutions like ETL (Extract, Transform, Load) tools streamline the merging of data from various sources, ensuring seamless data flow and comprehensive analytics. |
2. | Data visualization software tools | Tools like Tableau or PowerBI that transform complex datasets into visual stories, enabling better understanding and decision-making. |
3. | Cloud storage solutions | Platforms like AWS S3 or Google cloud storage facilitate scalable, flexible, and cost-effective data storage, retrieval, and management. |
4. | Automated data quality tools | Software that autonomously cleanses, validates, and maintains data quality, ensuring business decisions are based on accurate, timely, and consistent data. |
5. | AI & ML-driven data tools | Advanced tools leveraging artificial intelligence and machine learning to provide predictive analytics, anomaly detection, and automated insights. |
An adept data manager understands the value of their tools and techniques, much like a master craftsman. With the ever-evolving landscape of data management, staying updated and equipped with the latest tools is not just beneficial—it’s paramount.
As we’ve seen, these tools don’t merely manage data; they transform it, revealing insights, ensuring accuracy, and facilitating sound decision-making. Whether you’re a seasoned expert or a budding data enthusiast, arming yourself with these resources ensures you’re primed for success.
9 Steps to overcome rigorous data management challenges #
Every endeavor has its challenges, and data management is no exception. But with rigorous processes, we can convert these challenges into stepping stones.
Here’s how you can overcome data challenges with rigorous data management:
- Volume and velocity of data
- Data accuracy and consistency
- Evolving regulatory landscape
- Data security and breaches
- Integration of disparate data sources
- Skill and expertise gap
- Cost and resource management
- Data ownership and governance
- Keeping pace with technological advancements
Let’s look into each of the above aspects in brief:
1. Volume and velocity of data #
Today, organizations grapple with not just the staggering amount of data but also its rapid generation rate. Whether it’s from transactional systems, sensors, or user interactions, this deluge of information is relentless.
Rigorous data management necessitates proactive measures, like adaptive storage solutions and real-time processing capabilities, to harness this vast data ocean without getting overwhelmed.
2. Data accuracy and consistency: #
As data sources proliferate, maintaining a uniform view becomes arduous. Discrepancies or duplications can cripple analytics and decision-making. Data accuracy plays a major role here.
Through rigorous management, automated cleaning processes, regular audits, and uniform entry standards are implemented, ensuring that data across systems remains harmonized and error-free.
3. Evolving regulatory landscape #
The regulatory world of data is a labyrinth of intricate laws that vary across regions. From GDPR in Europe to CCPA in California, keeping pace with compliance is a task.
A rigorous approach involves continuous monitoring, timely updates, and adherence checks to ensure that every data operation aligns with prevailing regulations.
4. Data security and breaches #
The repercussions of data breaches are dire, affecting brand reputation, customer trust, and incurring hefty penalties.
Rigorous data management prioritizes robust encryption techniques, multi-factor authentication, and continuous threat monitoring. This ensures that data sanctity remains uncompromised amidst an array of cyber threats.
5. Integration of disparate data sources #
Data silos can severely impede a holistic view of operations. Integrating diverse datasets, whether from third-party collaborations or internal platforms, is challenging.
With rigorous management, custom integration pipelines, middleware solutions, and standardized data models are employed, ensuring seamless data flow and amalgamation.
6. Skill and expertise gap #
The intricate realm of data demands specialized skill sets. From data engineers to analysts, the expertise bar is continuously rising.
Rigorous management necessitates cultivating a culture of continuous learning, sponsoring certifications, and fostering a collaborative environment to bridge this expertise gap.
7. Cost and resource management #
The infrastructure and human capital required for comprehensive data management can strain resources. Balancing quality with cost is a tightrope walk.
Rigorous practices emphasize resource optimization, capitalizing on scalable cloud architectures, and harnessing automation to deliver quality without exorbitant expenses.
8. Data ownership and governance #
In multifaceted organizations, data ownership can blur, leading to bottlenecks or mismanagement. Clearly defined roles, meticulous access controls, and transparent data lineage are the pillars of rigorous data management.
These ensure that data processes are accountable and traceable.
9. Keeping pace with technological advancements #
The tech landscape is dynamic, with newer tools and solutions constantly entering the fray. Staying current is not just about competitive advantage but also about efficiency and innovation.
Rigorous data management means regular tech stack evaluations, piloting emerging tools, and fostering adaptability to ride the wave of technological progress.
As we move further into the information age, businesses that can robustly manage their data will stand tall, making informed decisions and paving their path to success.
Conclusion #
In the age of information, the way we handle and interpret data defines our competitive edge. Rigorous data management stands as a beacon of excellence in this scenario, transforming raw data into invaluable insights and ensuring its utmost accuracy, reliability, and security.
This elevated standard not only optimizes operations and strategies for businesses but also fosters trust, particularly when data breaches and misinformation are on the rise.
Embracing rigorous data management is, therefore, more than just a technical necessity—it’s a strategic imperative.
By prioritizing meticulous data handling and processing, organizations not only safeguard their assets but also unlock the untapped potential within, paving the way for innovation, growth, and long-term success in a data-driven world.
Related reads #
- Data Management 101: Four Things Every Human of Data Should Know
- Modern Data Management: 8 Things You Can Gain From It
- Metadata Management: Benefits, Automation, Use cases, and Framework
- Top 6 Metadata Management Best Practices — Guide for 2024
- What is DataOps & How Does it Help Data Management?
- Data Governance and Metadata Management: Understanding Their Synergy for Data-Driven Success
- Why is Metadata Important for Effective Data Management?
Share this article