What is Data Completeness? Examples, Differences & Steps
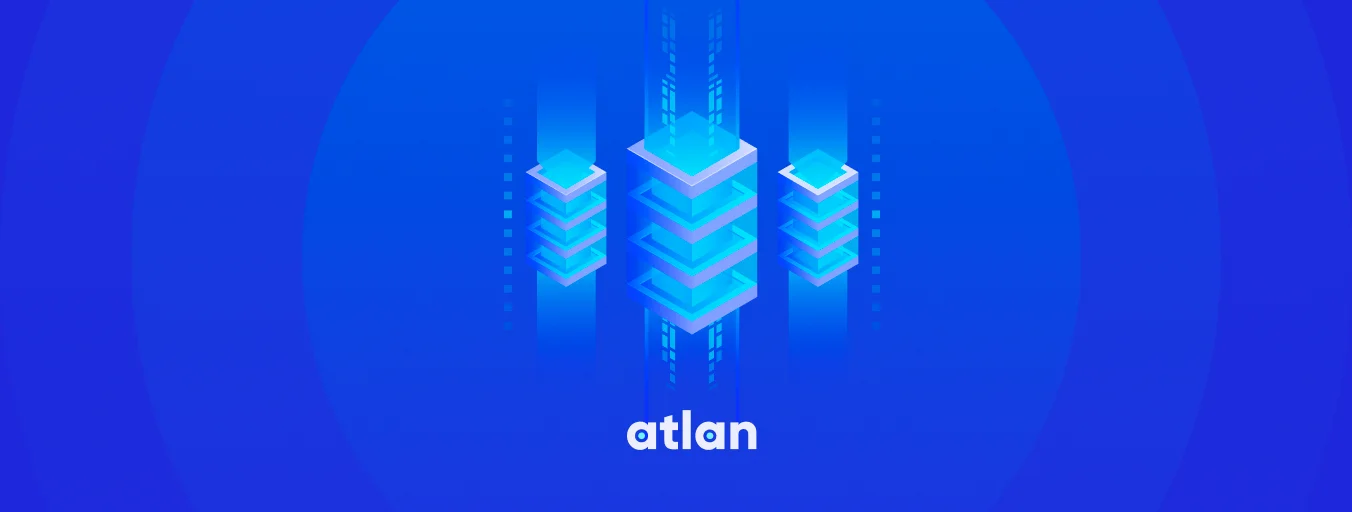
Share this article
Data completeness is the extent to which all required and expected data elements are present within a dataset, ensuring that no essential information is missing.
It is crucial for accurate insights, as missing information can lead to incomplete analyses and flawed decision-making. Neglecting data completeness risks distorted conclusions and undermines the reliability of business strategies or research outcomes.
Just imagine setting out on a journey with only half a map or baking a cake with only some of the ingredients. Similarly, when data isn’t complete, our analyses, strategies, and subsequent decisions can go away. That’s where data completeness comes in!
Modern data problems require modern solutions - Try Atlan, the data catalog of choice for forward-looking data teams! 👉 Book your demo today
In this article, we will explore:
- What is data completeness?
- Importance of data completeness
- Differences among data completeness, accuracy, and consistency
- How incomplete data affects success of your business?
- Advanced techniques you can follow to ensure data completeness
Ready? Let’s dive in!
Table of contents
- What is data completeness?
- 12 Reasons why data completeness is important
- 5 Fundamental examples of data completeness
- Data completeness vs data accuracy vs data consistency: How are they different?
- How incomplete data undermines business success: The silent saboteur!
- How to ensure data completeness? 8 Advanced techniques
- 8 Challenges in ensuring data completeness
- Conclusion
- What is data completeness: Related reads
What is data completeness?
Data completeness is the extent to which a dataset contains all the necessary elements and observations for a given purpose or analysis. It enhances the integrity and reliability of analyses, preventing gaps in understanding and supporting more robust decision-making processes.
In a complete dataset, all variables that are relevant to the research questions or operational requirements should be present and fully populated with valid data values.
Any missing, incorrect, or incomplete entries in the dataset can compromise the quality of analyses, interpretations, and decisions based on that data.
And there are several reasons why data completeness can’t be ignored. Let’s dive into them.
12 Reasons why data completeness is important
Why does data completeness matter? Data completeness is a fundamental aspect of data quality and plays a crucial role in various aspects of data management and decision-making.
Here are several reasons highlighting the importance of data completeness:
- Accurate analysis
- Effective decision-making
- Operational efficiency
- Customer insight
- Regulatory compliance
- Data integration
- Forecasting and planning
- Quality assurance
- Data mining and machine learning
- Strategic insight
- Customer trust
- Data governance
Let’s look at each of them in detail:
1. Accurate analysis
Incomplete data can lead to inaccurate and misleading analysis. When critical data points are missing, it can skew results, making it challenging to draw valid conclusions or identify trends.
2. Effective decision-making
Complete data is essential for informed decision-making. Whether it’s in business, healthcare, research, or any other field, having access to all relevant data ensures that decisions are based on a comprehensive understanding of the situation.
3. Operational efficiency
Incomplete data can hinder operational efficiency. For example, in supply chain management, missing inventory or shipping data can lead to disruptions and delays, impacting business operations.
4. Customer insights
Incomplete customer data can result in an incomplete view of customer behavior and preferences. This can limit a company’s ability to provide personalized services, targeted marketing, and exceptional customer experiences.
5. Regulatory compliance
Many industries are subject to regulations that require complete and accurate data reporting. Failure to maintain data completeness can lead to legal issues, fines, and reputational damage.
6. Data integration
Data completeness is crucial when integrating data from various sources to ensure that all relevant information is available for analysis. Incomplete data can hinder integration efforts and result in data silos.
7. Forecasting and planning
Incomplete historical data can affect forecasting and planning efforts. For instance, in financial forecasting, missing financial data points can lead to inaccurate predictions and budgeting.
8. Quality assurance
Data completeness is a key component of data quality assurance. It helps identify and rectify issues related to missing or incomplete data, ensuring data accuracy and reliability.
9. Data mining and machine learning
Complete datasets are essential for training machine learning models and conducting data mining. Missing data can lead to model bias and reduced predictive accuracy.
10. Strategic insights
Organizations rely on data completeness to gain strategic insights. Whether it’s identifying market opportunities, optimizing operations, or assessing risks, complete data is vital for strategic planning.
11. Customer trust
Incomplete or inaccurate data can erode customer trust. When customers notice errors in their data or experiences that suggest incomplete information, it can lead to dissatisfaction and lost trust.
12. Data governance
Data completeness is one of the pillars of effective data governance. Organizations need to establish policies and processes to ensure data is complete, accurate, and consistent to maintain data integrity.
In conclusion, data completeness is not just a data quality issue; it’s a critical factor in data-driven decision-making, operational efficiency, compliance, and customer satisfaction. Organizations must prioritize data completeness as part of their broader data management strategies to unlock the full potential of their data assets and gain a competitive edge in today’s data-driven landscape.
5 Fundamental examples of data completeness
Data completeness is crucial for ensuring the reliability and validity of data-driven insights and decisions. It plays a pivotal role in a wide array of domains, from healthcare and finance to technology and social research, by guaranteeing that analyses are based on a full and accurate representation of the intended dataset.
Here are five examples of data completeness, followed by a more detailed explanation of each:
- Complete demographic information
- Historical data coverage
- Geographical data representation
- Sensor data in IoT
- Medical records completeness
Let’s understand them one by one.
1. Complete demographic information
In surveys or research studies, having complete demographic information (age, gender, income level, etc.) for each respondent ensures that analysis can accurately reflect the diverse characteristics of the population.
For example: In a health survey, if certain demographic groups are underrepresented (e.g., specific age groups or ethnicities), the conclusions drawn might not be applicable to these groups. Complete demographic data ensures that the findings are relevant and applicable to all sections of the population.
2. Historical data coverage
For financial or economic analyses, having complete historical data allows for more accurate trend analysis and forecasting. Missing historical data can lead to incorrect assumptions about market behaviors or economic conditions.
Example:
In stock market analysis, missing data from certain periods can skew the understanding of market trends and volatility. Complete historical data allows analysts to recognize patterns and make more informed predictions about future market movements.
3. Geographical data representation
In geographical information systems (GIS) or location-based studies, complete geographical data is essential for accurate mapping and analysis. Incomplete geographical data can lead to misrepresentation of areas, affecting planning and decision-making.
Example:
For urban planning, incomplete geographical data might overlook certain areas, leading to biased resource allocation or emergency response planning. Complete data ensures that all regions, including remote or less-populated areas, are considered in planning processes.
4. Sensor data in IoT
In the Internet of Things (IoT), complete sensor data from all devices ensures accurate monitoring and control. Incomplete data due to missing or non-reporting sensors can lead to incorrect conclusions about the monitored environment or system.
Example:
In a smart home system, if some sensors (like those for smoke or carbon monoxide detection) fail to transmit data, it could lead to serious safety oversights. Complete sensor data ensures that all aspects of the environment are monitored, enhancing safety and efficiency.
5. Medical records completeness
In healthcare, complete medical records are vital for accurate diagnosis and treatment planning. Incomplete records can result in misdiagnosis or ineffective treatment plans.
Example:
In patient care, missing information about past treatments or allergies can have dire consequences. Complete medical records ensure that healthcare providers have a full understanding of a patient’s medical history, leading to better-informed and safer treatment decisions.
By prioritizing data completeness, organizations and researchers can make well-informed decisions, derive accurate insights, and implement effective solutions that cater to the needs and dynamics of their respective domains.
Data completeness vs data accuracy vs data consistency: How are they different?
In a nutshell, data completeness focuses on having all necessary information, data accuracy ensures closeness to true values, and data consistency emphasizes uniformity and coherence across datasets. Together, they address different aspects of data quality, covering comprehensiveness, correctness, and coherence in information.
Let’s delve deeper and provide a comprehensive table differentiating between data completeness, data accuracy, and data consistency.
Criteria | Data completeness | Data accuracy | Data consistency |
---|---|---|---|
Definition | Ensures that every necessary piece of information is present in the dataset. | Ensures that the information in the dataset is correct and represents real-world entities accurately. | Ensures that the data remains uniform, synchronized, and doesn't show discrepancies across various platforms. |
Analogy | Like having all pieces of a jigsaw puzzle. | Like making sure every puzzle piece belongs to the intended puzzle. | Like ensuring all versions of the puzzle represent the exact same image. |
Primary focus | Availability of data entries. | Correctness of data entries. | Uniformity of data across platforms and databases. |
Importance | Without completeness, the dataset's utility is compromised as it can lead to incomplete analysis. | Inaccurate data can lead to misguided decisions, impacting operations, strategy, and financial health. | Ensuring a consistent data view irrespective of where the data is accessed, which is crucial for integrated systems. |
Impact on decision making | Incomplete data can lead to faulty assumptions and missed opportunities. | Decisions based on inaccurate data can steer a business in the wrong direction. | Inconsistent data can cause confusion, leading to paralysis in decision-making or conflicting strategies. |
Potential risks | Loss of essential insights, inability to meet regulatory compliance, decreased data usability. | Misinformed strategies, wasted resources, tarnished reputation. | Operational inefficiencies, difficulties in data integration, mistrust in data systems. |
Mitigation methods | Regular data audits, feedback loops for data input, and robust data collection mechanisms. | Data validation checks, regular audits, training, and data cleansing. | Data governance policies, data synchronization tools, and consistent data entry protocols. |
Key metrics | Percentage of missing values, number of null values in mandatory fields. | Error rates, percentage of data entries that fail validation checks. | Number of discrepancies across systems, data synchronization failures. |
Completeness, accuracy, and consistency are the three musketeers of data management. While each has its distinct role, together they form an invincible force that guarantees top-tier data quality.
How incomplete data undermines business success: The silent saboteur!
In today’s digital epoch, data is the lifeblood of business decision-making. It fuels innovations, strategies, and day-to-day operations. But imagine steering your ship with a map that has missing territories; navigating without the full picture is perilous.
Similarly, incomplete data is like a narrative with gaping plot holes - you’re never quite sure where it might lead.
The dangers lurking in the shadows of incomplete data are:
- Skewed interpretations
- Economic pitfalls
- Diluted brand integrity
- The blind spot effect
- Stunted innovation
- Operational hiccups
- Misallocated resources
- Regulatory and compliance risks
Let us look into each of the above dangers in detail:
1. Skewed interpretations
When businesses operate on fragmented datasets, they are essentially working with an incomplete story. These missing fragments can lead to misconceptions about market trends, customer preferences, and more.
The result? Businesses may take actions based on these misconceptions, which can have significant repercussions. It’s analogous to making decisions based on a half-told story, leading to strategies that might be misaligned with actual market needs and customer desires.
2. Economic pitfalls
Financial planning is an intricate art that requires comprehensive data to craft a strategy that will ensure success. Without complete information, financial projections can go awry, leading to budget overflows, underfunded projects, or failed business ventures.
Imagine charting a course on a map with parts missing. You might end up in unforeseen obstacles, culminating in wasted time, effort, and money.
3. Diluted brand integrity
Brands are built on trust, and each decision a company makes affects that trust. When actions based on incomplete data become visible to the public and they backfire, it can significantly damage a brand’s reputation.
This isn’t merely about immediate financial losses; it’s about the intangible yet invaluable asset of customer trust. Once diminished, regaining this trust is an uphill battle, making future endeavors even more challenging.
4. The blind spot effect
The corporate landscape is dynamic, with new opportunities and threats emerging continually. Incomplete data acts as a blindfold, rendering companies unaware of these changes.
Like a driver unaware of a vehicle in their blind spot, businesses can crash into unforeseen challenges or miss golden opportunities right next to them. Remaining oblivious in a world that’s always evolving is a sure-shot strategy to obsolescence.
5. Stunted innovation
Innovation is the engine of business growth. To innovate effectively, companies need a holistic view of market demands, customer preferences, and technological advancements. With incomplete data, the innovation engine sputters.
Imagine trying to create a new product with only half the necessary ingredients or attempting to meet a need you’re only partially aware of. The outcome is often products or services that miss the mark, failing to resonate with target audiences.
6. Operational hiccups
Every cog in the business machine, from procurement to customer service, relies on data to function smoothly. If any segment gets fed incomplete data, the machine grinds, creating bottlenecks, inefficiencies, and subpar output.
It’s like running an assembly line where one station doesn’t get all the parts it needs, causing hiccups that affect the entire production process.
7. Misallocated resources
Resources, be it time, manpower, or capital, are finite. Their correct allocation can be the difference between success and failure. When businesses operate on incomplete data, they risk investing heavily in areas that don’t yield significant returns while neglecting high-potential opportunities.
It’s comparable to watering the weeds while ignoring the plants. Not only is there a loss of potential growth, but there’s also a waste of valuable resources.
8. Regulatory and compliance risks
Beyond the realm of strategy and operations lies the legal domain. Many industries are governed by stringent regulations that mandate complete and accurate reporting. Using incomplete data not only risks non-compliance but can also lead to hefty penalties and legal complications.
This isn’t just a matter of financial repercussions; a legal misstep can scar a company’s reputation, making future business engagements more challenging.
In the expansive landscape of business, data serves as both the compass and the map. Gaps in this map don’t just represent minor oversights; they signify potential pitfalls. Assembling a complete data picture isn’t about hoarding every bit of information—it’s about ensuring you have the right pieces to see the bigger, clearer picture.
How to ensure data completeness? 8 Advanced techniques
In the vast ocean of modern business, data stands as the navigational star guiding enterprises to success. Complete and accurate data, therefore, isn’t merely a resource—it’s a beacon, ensuring decisions aren’t based on mirages but on tangible truths.
As we delve into the nuances of data completeness and the prowess of tools like Atlan, we’ll discover the significant role they play in sculpting a data-driven future.
The advanced techniques that ensure data completeness are:
- The imperative of complete data
- Attribute-level approach with Atlan’s analytics
- Record-level scrutiny
- Quick insight via data sampling
- Deep dive with data profiling
- Data visualization for completeness
- Automated alerts and monitoring
- Building a culture of data integrity
Let’s look into each of the above techniques in brief:
1. The imperative of complete data
The 21st-century business landscape is intricately woven with digital threads. In this mesh, data stands paramount. As decisions pivot on the fulcrum of data, even slight inaccuracies can lead to monumental missteps.
Platforms like Atlan fortify this foundation, ensuring the data used is unblemished and comprehensive, shielding organizations from potential pitfalls caused by incomplete datasets.
2. Attribute-level approach with Atlan’s analytics
Delving into datasets can sometimes be like traversing a labyrinth. Atlan’s attribute-level analytics illuminate this maze, focusing on individual data fields.
By highlighting how consistently these fields are populated, businesses can pinpoint and reinforce weak spots in data collection, ensuring no attribute is left in obscurity.
3. Record-level scrutiny
Data integrity isn’t just about isolated fields—it’s about the holistic story each record tells. Atlan transcends surface-level analysis, diving deep into records to reveal any voids in the narrative.
This ensures that every record doesn’t merely exist, but adds significant value, weaving a richer tapestry of insights.
4. Quick insight via data sampling
In the overwhelming torrents of data, it’s easy to feel lost. Data sampling in Atlan serves as a lighthouse, offering a truncated yet revealing view of data’s overall health.
By examining these subsets, businesses get an indicative pulse of their data’s wellness, paving the way for comprehensive audits.
5. Deep dive with data profiling
True data mastery demands more than surface skims—it requires profound dives. Atlan’s data profiling is this deep dive, meticulously examining data’s character and quality.
Beyond identifying voids, it uncovers redundancies and anomalies, ensuring data is a pristine reflection of reality.
6. Data visualization for completeness
Numbers and records, while informative, might lack intuitive clarity. Atlan’s visualization tools transpose data into vivid graphics, making patterns of completeness (or lack thereof) instantly recognizable.
By translating data into visual narratives, businesses can intuitively grasp and address the intricacies of data gaps.
7. Automated alerts and monitoring
Vigilance is the sentinel of data integrity. Atlan’s automated monitoring tools stand as this ever-watchful guardian, sending out alerts when data health wavers.
This proactive approach ensures that any drift from data completeness is swiftly recognized and rectified.
8. Building a culture of data integrity
Technological prowess, while pivotal, needs to be complemented by an organizational ethos. Embracing platforms like Atlan catalyzes a cultural shift, where data integrity becomes a shared vision.
Coupled with ongoing education and training, businesses can nurture a workforce that’s not just data-literate, but data-passionate.
Data completeness is more than a metric—it’s the lifeblood of informed, strategic decision-making. In equipping themselves with platforms like Atlan, businesses aren’t just adopting a tool; they’re embracing a vision—a vision where every data point matters, every insight is rooted in reality, and every decision is founded on unwavering confidence in the data’s integrity.
8 Challenges in ensuring data completeness
The vast digital landscape of the 21st century has redefined the role of data, propelling it to the forefront of organizational decision-making. However, harnessing its full potential demands more than just accumulation; it requires pristine data completeness.
Much like a highway journey, this path has its unique set of challenges, often unforeseen and potentially disruptive.
The major challenges of data completeness are:
- Inadequate data collection processes
- Data entry issues
- Integration hurdles
- Validation and verification dilemmas
- Legacy system limitations
- Data decay
- Lack of data ownership
- Insufficient feedback loops
Let’s look into each of the above challenges in brief:
1. Inadequate data collection processes
An efficient data journey begins at the collection stage. When systems are not streamlined or are outdated, they can produce inconsistent or even fragmentary datasets.
A key solution lies in consistently reviewing and refining collection methods, aligning them with the latest technological tools, and customizing them based on specific organizational needs.
Regular training sessions can also ensure that everyone involved understands the evolving nuances of data collection.
2. Data entry issues
Despite technological advancements, human involvement in data entry remains significant, bringing along the risk of errors. These errors can manifest as typographical mistakes, data omissions, or even misinterpretations.
Addressing this requires a multifaceted approach: rigorous training programs, the introduction of double-entry systems, and the integration of AI-powered error detection mechanisms to highlight and rectify discrepancies in real-time.
3. Integration hurdles
In the age of diversified digital tools, data often resides in isolated pockets. Merging this fragmented information can become a monumental challenge, leading to data overlap or even data loss.
To counter this, organizations can look towards adopting universally accepted data formats, leveraging middleware solutions for seamless integration, and meticulous data mapping to ensure every data piece finds its rightful place.
4. Validation and verification dilemmas
Once data is collected, its authenticity and accuracy become paramount. Absence of stringent validation checks can allow erroneous data to seep into the system.
To fortify the validation process, organizations can introduce automated validation tools, conduct periodic data audits, and cross-reference data against reliable external sources, ensuring its veracity and relevance.
5. Legacy system limitations
Legacy systems, despite their reliability, often become roadblocks in the contemporary data landscape. Their inability to support modern data formats or advanced features can hinder data completeness.
By regularly updating these systems, transitioning to more modern platforms, or even deploying compatibility solutions, organizations can bridge the old with the new, ensuring no data is left behind.
6. Data decay
Time can erode the relevance of data. As situations evolve, previously crucial data might become obsolete, leading to an incomplete or misleading dataset.
Combatting this requires a proactive stance: scheduling regular data updates, automating data refresh cycles, and actively purging data that no longer aligns with the current context.
7. Lack of data ownership
In the vast data ecosystem, the absence of clear ownership can lead to oversight and neglect. By clearly defining data ownership, delineating specific roles, and instituting a dedicated team for data stewardship, organizations can imbue a sense of responsibility, ensuring that data remains at its most complete and most accurate.
8. Insufficient feedback loops
Continuous improvement in data quality demands feedback. Without a robust mechanism to garner insights about data quality, potential improvement areas might remain shadowed.
Encouraging feedback from all stakeholders, both internal and external, setting up platforms for easy reporting of discrepancies, and most importantly, acting on this feedback, can set the stage for ongoing data quality enhancements.
The challenges on the path to data completeness, though significant, are not insurmountable. With a blend of strategic foresight, technological aid, and a commitment to constant refinement, organizations can navigate this data highway’s twists and turns, ensuring a journey that’s not just smooth, but also insightful and profoundly impactful.
Conclusion
Data completeness is the extent to which a dataset contains all the expected and required information without any omissions. It signifies the inclusiveness of data, ensuring that the dataset encompasses all relevant elements for accurate and reliable analysis or decision-making.
The journey to achieving data completeness is continuous. With evolving technologies and increasing data sources, businesses must remain vigilant and proactive. Embracing advancements like data observability can be the game-changer in this pursuit.
In a world driven by data, completeness isn’t just an aspiration; it’s a necessity. Ensuring every piece of data is present, accurate, and timely is the commitment every business should make for sustainable success.
What is data completeness: Related reads
- Data Accuracy: Examples, Steps to Measure & Maintain (2023)
- Data Consistency 101: Causes, Types and Examples
- What is Data Reliability & How to Go About It in 2023?
- Data Integrity vs Data Quality: Nah, They Aren’t Same!
- Data Quality vs Data Accuracy: 15 Key Differences To Know
- Data Validation vs Data Quality: 12 Key Differences
- Dark Data: Concept, Challenges, and Ways to Manage It
- Stale Data Explained: Your Ultimate Guide in 2023!
- Data Quality Measures: Best Practices to Implement
- Data Quality Metrics: Understand How to Monitor the Health of Your Data Estate
- Data Quality Testing: Key to Ensuring Accurate Insights!
- Crafting a Winning Data Quality Strategy!
Share this article