Why is Metadata Important for Effective Data Management?
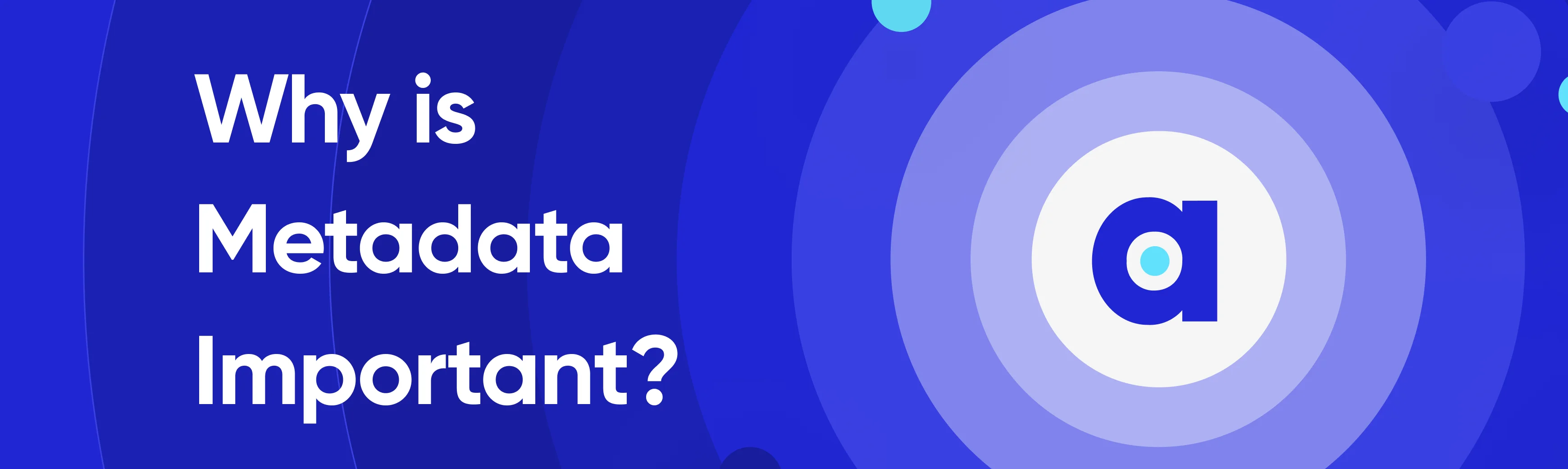
Share this article
Metadata is important because it provides context with details such as the source, type, owner, and relationships to other data sets, thus helping you understand the relevance of a particular data set and guiding you on how to use it.
Modern metadata management is at a crucial point of evolution, necessitating a shift in approach to keep up with the rest of the data infrastructure.
This new approach acknowledges that today’s diverse data teams, made up of engineers, analysts, data scientists, and more, require a more comprehensive, flexible, and collaborative system for metadata.
In this article, we will understand in greater depth why metadata is important for modern data teams with the help of examples.
Let’s dive in!
Table of contents #
- Why is metadata important for a dataset? Unlocking the power of data insights
- Why is metadata important in data management for organizations: Explained with examples
- Understanding different types of metadata and how they are used
- Bringing it all together
- Why is metadata important: Related reads
Why is metadata important for a dataset? Unlocking the power of data insights #
Metadata is essentially “data about data”. It provides information about a certain item’s content. For instance, a music file’s metadata could contain the song’s name, the artist, the album it’s from, and the year it was released.
Similarly, in the context of a dataset, metadata can include information about what’s in the dataset, how it was collected, who collected it, when it was collected, and so forth.
Metadata is vital for various reasons. It enhances the understanding, use, and management of data. Without metadata, it would be nearly impossible to effectively use or manage large amounts of data, as we wouldn’t know the source, quality, applicability, or any other relevant details about the data we’re working with.
In this section of the article, we will delve into the benefits of metadata that make it crucial for your business. Let’s dive into the primary benefits one by one:
- Data context understanding
- Data quality
- Data integration
- Data governance
- Data search and discovery
- Data lineage
- Data security
- Improved decision-making
- Support for AI and machine learning
- Operational efficiency
Let us look at each of the above points in brief:
1. Data context understanding #
Metadata provides context for data. It provides clarity on what data represents, its type, origin, and structure. Without metadata, data can often be challenging to interpret, especially when the datasets are complex or extensive.
It helps us understand where the data came from, how it was gathered, what it represents, and how it relates to other data. This context is vital when it comes to accurately interpreting and analyzing data.
By giving context, metadata makes it possible to understand the data, promoting efficient data analysis and decision-making.
2.Data quality #
Metadata can provide information about data quality, for instance, when and how the data was collected, and by whom. This is vital for assessing the reliability and relevance of data, as well as for maintaining data standards.
Ensuring high-quality data is critical for business operations, analytics, and decision-making.
3. Data integration #
Metadata aids in data integration by providing essential details like data structure and data origin. It helps to ensure that data from different sources or systems can be accurately mapped and consolidated.
Thereby, it facilitates a unified view of the data for analysis or operational use.
4. Data governance #
Metadata plays a crucial role in data governance by helping to enforce standards and policies for data management. This includes access permissions, data usage policies, and ensuring regulatory compliance.
For example, a metadata attribute might indicate that a certain dataset contains sensitive information, triggering specific data handling requirements.
5. Data search and discovery #
Metadata is key to sorting and organizing data. It allows for efficient data retrieval and use, especially in large databases or data warehouses where volumes of data are stored.
Metadata facilitates data discovery, making it easier to locate and retrieve specific datasets from potentially vast data stores. This is essential for efficiency and productivity, as users can quickly find the data they need without having to sift through irrelevant data.
6. Data lineage #
Metadata is key to understanding data lineage - that is, tracking data from its origin through its lifecycle. This helps users understand where data comes from, how it moves and transforms, and how its quality might have changed over time. This is particularly important for audit trails, debugging data issues, and ensuring data integrity.
7. Data security #
Metadata can also contribute to data security by providing information about who has accessed the data, when, and the changes they have made. This helps in maintaining accountability, detecting suspicious activities, and protecting sensitive information.
8. Improved decision-making #
Metadata supports improved decision-making by offering context that aids in data interpretation. This context provides the foundation for accurate, data-driven decisions, which are increasingly important in the modern business environment.
9. Support for AI and machine learning #
In AI and machine learning, metadata can help with data preparation, feature selection, model interpretation, and tracking model performance over time. This is important for developing and maintaining effective AI models.
10. Operational efficiency #
With metadata, data management tasks can be automated and made more efficient. It helps data professionals navigate data infrastructure, reducing time spent on data-related tasks and increasing productivity.
Metadata provides context, supports data governance, and improves the efficiency and effectiveness of data-related tasks. Thus, metadata is an invaluable resource for any data-driven organization.
Why is metadata important in data management for organizations? Explained with examples #
Now, let’s delve deeper into why metadata is important in data management for organizations (with respect to the points discussed above) and explained with specific examples:
1. Data understanding #
Suppose a database contains a column labeled “A123.” Without metadata, you won’t know what this column represents. But with appropriate metadata, you will understand that “A123” represents ‘Annual Revenue in USD.’
Such clarity is especially important when multiple teams are accessing the same data, ensuring that everyone has a unified understanding.
2. Data quality #
Imagine a dataset containing customer feedback scores. The metadata might reveal that these scores were collected via an online survey distributed to a random sample of customers. Knowing this, you can assess the reliability of this data and you know that the data is quite reliable because it’s based on a random sample.
3. Data integration #
Let’s say a company merges with another, and they both have customer databases that need to be combined. The metadata helps in mapping fields between these databases, ensuring that, for instance, ‘Customer_ID’ in one database corresponds to ‘CustID’ in the other.
4. Data governance #
Metadata can show that certain data is classified as Personal Identifiable Information (PII), requiring it to be handled according to specific policies and regulations, such as GDPR or CCPA. Without metadata, this sensitive information might not be adequately protected.
5. Data search and discovery #
A researcher looking for data on monthly sales of a specific product can use metadata to quickly locate the relevant datasets, filtering by date, product category, etc. Without metadata, they might have to manually search through countless datasets to find the right one.
6. Data lineage #
Suppose a report shows a sudden drop in sales last quarter, but it’s unclear why. By examining the metadata, you can trace the data lineage back to its source and discover that a new sales recording system was implemented midway through the quarter, which may have affected the data.
7. Data security #
Metadata can record that an unauthorized user attempted to access a sensitive dataset at a particular time. This can alert security teams to potential data breaches and help them take appropriate action.
8. Improved decision making #
Consider a business leader deciding whether to launch a new product. Metadata can provide context to the sales data of similar products, like which region the sales data is from, allowing them to make a more informed decision.
9. Support for AI and machine learning #
For instance, in an image recognition AI model, the metadata of an image dataset (like image resolution, creation date, source) can help in understanding the model’s performance and its potential biases.
10. Operational efficiency #
A data engineer is asked to update a dataset used in several weekly reports. The metadata shows exactly which reports use this dataset, allowing the engineer to update the data efficiently and notify relevant parties about the change.
In essence, metadata helps bring clarity, efficiency, and precision to data management. It serves as the roadmap that guides users to the right data and helps them understand it thoroughly, making it a critical element in any data-driven operation.
Understanding different types of metadata and how they are used #
There are several types of metadata that exist within data ecosystems, each serving a unique purpose and offering distinct benefits. Here are the primary ones:
- Descriptive metadata
- Structural metadata
- Administrative metadata
- Reference metadata
- Statistical metadata
- Business metadata
- Operational metadata
- Legal metadata
Now, let us look at each of the above metadata types in brief:
1. Descriptive metadata #
This type of metadata provides information about a resource for the purposes of discovery and identification. It can include elements such as title, abstract, author, and keywords.
Example
For example, in a dataset containing customer information, descriptive metadata might include details about when the data was collected, by whom, method of collection, etc. It is crucial for finding specific datasets and understanding their basic nature.
Or in another instance, a data analyst searching for customer feedback data can use descriptive metadata to quickly find the right datasets.
2. Structural metadata #
This refers to how the components of a dataset are organized or how they relate to each other. In a database, structural metadata might include tables, schemas, relationships between tables (like foreign keys), database indexes, etc.
This type of metadata is crucial when integrating data from different sources, performing database operations, or constructing data models.
Example
Structural metadata can help data engineers design and optimize databases. For instance, understanding the relationships between different tables can help optimize query performance.
3. Administrative metadata #
This metadata type provides information to help manage a resource, like when and how it was created, file type, and who can access it. This is crucial for data governance, ensuring proper data usage, and maintaining data security.
Example
For example, administrative metadata helps in knowing who has access to a specific dataset which helps enforce appropriate access controls.
4. Reference metadata #
This metadata provides information about the contents and quality of statistical data. This can include details about the methodologies and instruments used to collect the data, as well as data validation and quality assurance processes.
Example
Let us imagine a customer survey dataset for a moment, In this dataset, reference metadata might include the survey questions, response scales used, and quality control steps taken during data collection. Knowing that survey data has gone through a rigorous quality assurance process can give analysts confidence in the data.
5. Statistical metadata #
Also known as process metadata, it refers to data about the processes that collect, process, or produce statistical data. This can include the details of data processing techniques, ETL processes, data lineage, and other operational aspects. This metadata type is critical for debugging data issues and tracing data back to its source.
Example
For example, if a problem arises with a dataset, statistical metadata can help trace the problem back to a particular step in the data processing pipeline.
6. Business metadata #
Business metadata is important for business users. It includes definitions of data elements, business rules around data, data ownership information, and other documentation useful for business users. It is crucial for understanding the business context of data.
Example
For instance, knowing the business definitions of specific data elements can help business analysts interpret data correctly.
7. Operational metadata #
Operational metadata includes technical details, like the version of a dataset or when the data was last updated. It can be used to track changes in the dataset over time, manage data migrations, or maintain data versions.
8. Legal metadata #
This includes information about the legal status and obligations of data, such as copyright status, data retention requirements, or privacy classification. This metadata is crucial for ensuring compliance with data regulations.
Bringing it all together #
Metadata is often described as “data about data,” and is an essential aspect of business, particularly as organizations become more data-driven. It provides essential information about other data, which makes understanding and working with the data easier.
In conclusion, metadata serves as a guide in the complex world of data. As businesses continue to generate and leverage more data, the importance of understanding and managing this data through metadata will only grow.
Robust metadata management is the key for data-driven teams to discover, understand, trust, and collaborate on data assets across your data universe. If you are looking to implement a metadata management tool for your organization, do take Atlan for a spin.
Why is metadata important: Related reads #
- Types of metadata: How each helps with faster data discovery and better insights
- What is the difference between data catalog and metadata management?
- Metadata management 101: Benefits, tools, and best practices
- 6 metadata management best practices to follow in 2023
- What is metadata: Examples, types, benefits, use cases, and why it’s so important?
- Data vs. Metadata: Understand the differences
- Enterprise metadata management and its importance in the modern data stack
- Mastering Business Metadata: How to Achieve Data Excellence and Streamlined Collaboration
- The Power of Metadata: A Guide to Effortless Data Discovery and Analysis
- Metadata Governance: The Missing Piece of the Data Governance Puzzle for Achieving Your Business Goals
Share this article