11 Essential Benefits of Data Profiling You Can't Overlook in 2024!
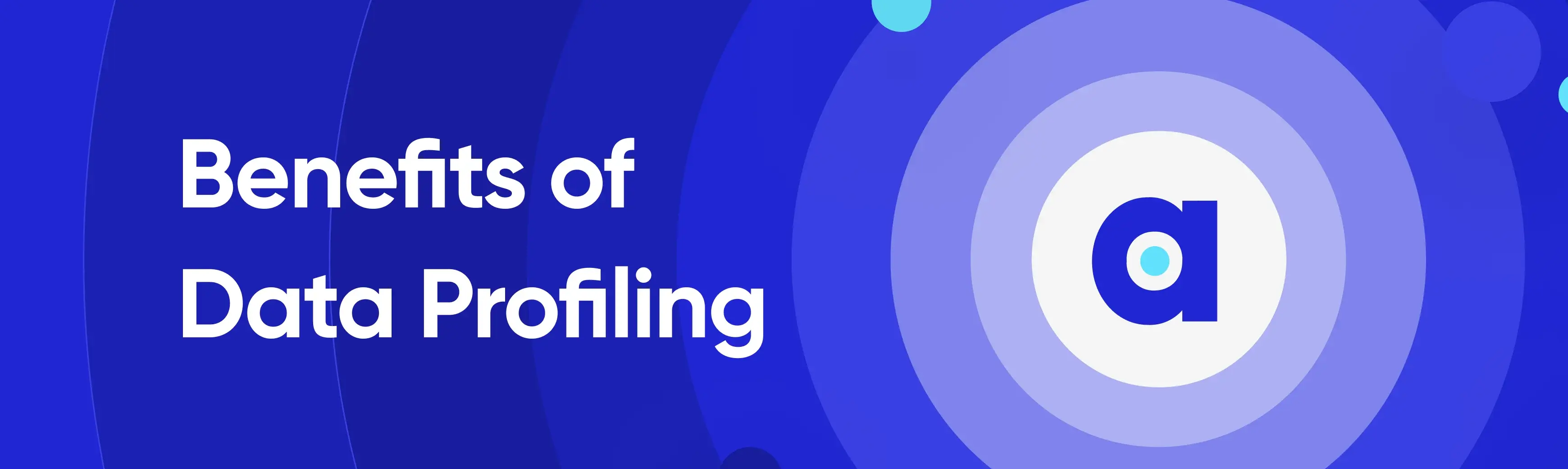
Share this article
Data profiling offers a range of critical benefits, such as improving data quality, resolving data issues, ensuring compliance, and mitigating risk. But there’s much more to uncover.
Modern data problems require modern solutions - Try Atlan, the data catalog of choice for forward-looking data teams! 👉 Book your demo today
In this article, we will understand the different benefits of data profiling, and how to evaluate data catalog and governance solutions with data profiling capabilities.
Let’s dive in!
Table of contents #
- Benefits of data profiling: 11 Essential benefits you can’t ignore!
- Understanding benefits of data profiling with examples
- Data profiling vs data quality: What’s the difference?
- Key considerations for assessing data profiling benefits
- Key considerations for evaluating data catalog and governance solutions with robust data profiling capabilities
- Bringing it all together
- Benefits of Data Profiling: Related reads
Benefits of data profiling: 11 Essential benefits you can’t ignore! #
Let’s explore the multifaceted benefits of data profiling in detail. Data profiling not only mitigates risks and ensures compliance but also empowers businesses to gain insights into customer behavior, achieve cost savings, and make informed decisions while maintaining a robust data governance strategy.
Here’s how data profiling empower you with its following eleven essential benefits:
- Understand data quality
- Identify data issues
- Understand data structure and relationships
- Mitigate risk
- Support data governance
- Facilitate data democratization
- Support data integration
- Enhance efficiency of data-driven processes
- Understand customer behavior
- Cost savings
- Ensure compliance
Let us look at the above benefits of data profiling briefly:
1. Understand data quality #
Profiling your data gives you a sense of its quality - the accuracy, consistency, completeness, and reliability of the data. Knowing this is critical in a financial context where data quality can impact business decisions, compliance, and customer service.
2. Identify data issues #
Data profiling helps you identify issues like missing or inconsistent data, duplicate entries, invalid data, or data that does not conform to your business rules or standards. It can uncover potential errors or anomalies that could impact your analytics, operations, or reporting.
3. Understand data structure and relationships #
Data profiling can provide insights into how data is structured and how different data elements relate to each other. This knowledge can help in designing data models, ETL processes, and integrations.
4. Mitigate risk #
Many industries are subject to regulatory requirements related to data handling. Data profiling can help businesses comply with these regulations by providing visibility into data content and structure. It can also help detect and prevent potential data breaches by identifying sensitive data.
5. Support data governance #
As part of your data governance program, data profiling supports:
- Data lineage (tracing data from its source to its destination)
- Data discovery (understanding what data you have), and
- Data literacy (helping people understand what the data means)
6. Facilitate data democratization #
By understanding and cataloging your data, you make it easier for people across the organization to find, understand, and use the data they need. This supports data democratization and empowers business users, analysts, and data scientists to work more effectively.
7. Support data integration #
When merging data from different sources, understanding the structure, content, and relationships of the data is essential. Data profiling can provide this understanding, making data integration projects more efficient and successful.
8. Enhance efficiency of data-driven processes #
By improving the quality of data and understanding it better, businesses can make their data-driven processes, like data analysis or machine learning, more efficient. It saves considerable time that could be spent handling data errors or dealing with inaccurate results due to poor data quality.
9. Understand customer behavior #
Data profiling can help businesses understand their customer data better, which in turn can lead to insights about customer behavior. These insights can support more effective marketing and customer service.
10. Cost savings #
By identifying and correcting data issues early, data profiling can help prevent the costly consequences of poor data quality, like faulty business decisions or non-compliance with regulations.
11. Ensure compliance #
In the financial sector, maintaining compliance with regulations is crucial. Data profiling can help identify sensitive data, monitor its use, and ensure appropriate security and privacy measures are in place.
While selecting a data governance/catalog tool, a feature that allows data profiling would be essential. It will help to ensure that your data is reliable and accessible, contributing to a strong data governance strategy.
Understanding benefits of data profiling with examples #
Data profiling offers several benefits that can lead to significant business outcomes. Here are some of the key benefits explained through examples:
- Improves decision making
- Supports compliance efforts
- Reduces operational costs
- Enhances customer service
- Facilitates mergers and acquisitions
- Improves data security
- Accelerates onboarding and training
Let us now look into each of these benefits in brief:
1. Improves decision making #
High-quality data enables more accurate and reliable analytics, which drives better decision-making.
For instance, an e-commerce business can profile its customer data to gain a clearer understanding of purchasing behaviors, which can inform marketing strategies and drive increased sales.
2. Supports compliance efforts #
Data profiling can help businesses identify sensitive or regulated data, which is crucial for maintaining compliance with data protection regulations like GDPR or CCPA.
For example, a healthcare provider profiling its patient data might discover unsecured personally identifiable information (PII) that needs to be better protected to comply with HIPAA.
3. Reduces operational costs #
Data profiling can identify inconsistencies, errors, and redundancies that could lead to operational inefficiencies.
For example, a manufacturing firm may discover through data profiling that its inventory data is inaccurate, leading to overproduction or stockouts. By rectifying these data issues, the company can better align production with demand and reduce costs.
4. Enhances customer service #
By profiling customer data, businesses can gain a better understanding of their customer’s needs and preferences, allowing them to provide more personalized service.
A telecommunication company, for instance, might profile its data to discover which customers are likely to churn based on their usage patterns. They can then take proactive measures to improve their experience and retain them.
5. Facilitates mergers and acquisitions #
During a merger or acquisition, data profiling can help identify compatibility issues between the merging companies’ data systems and structures. This can prevent potential conflicts or problems in data integration after the merger, ensuring a smoother transition.
6. Improves data security #
Data profiling can help organizations identify data vulnerabilities and implement necessary security measures.
For example, a financial institution might use data profiling to find patterns indicating fraudulent transactions, thus improving its fraud detection capabilities.
7. Accelerates onboarding and training #
With well-profiled and cataloged data, new employees or stakeholders can be quickly familiarized with the available data assets, reducing the learning curve and enabling them to contribute faster.
For example, a new data analyst in a financial institution would be able to quickly understand the data landscape, accelerating their onboarding process.
By employing data profiling, businesses can leverage the data profiling benefits and turn their data into a strategic asset that supports better decision-making, compliance, efficiency, customer service, and security.
Data profiling vs data quality: What’s the difference? #
Data quality refers to the overall condition of a data set and involves aspects like accuracy, completeness, reliability, relevance, and timeliness. On the other hand, data profiling is a process or technique used to assess and understand the quality of data. It’s a way of “getting to know” your data by examining its characteristics and quirks.
Data quality #
- High-quality data correctly represents the real-world construct that it is intended to model. It’s consistent, unambiguous, and free from corruption.
- The concept of “data quality” goes beyond just the data itself to include factors like how easily data can be processed and analyzed, how well it supports its intended uses, and how trustworthy it is perceived to be.
Data profiling #
- The goal is to discover anomalies, inconsistencies, and patterns, understand the structure, relationships, and values within the data, and assess whether the data meets the requirements for its intended use.
- Data profiling can reveal where data quality problems exist and help guide efforts to clean and improve the data.
In essence, data quality is a property or attribute of the data, while data profiling is a method or tool used to investigate and enhance that attribute. You can think of data profiling as the “diagnostic test” and data quality as the “health condition” that the test is trying to assess and improve.
Improving data quality often involves an iterative process of data profiling to identify problems, data cleaning to fix them, and then more data profiling to confirm the fixes and uncover more issues. This process continues until the data reaches an acceptable level of quality for its intended purpose.
Key considerations for assessing data profiling benefits #
When assessing the benefits of data profiling, there are several factors to consider beyond the immediate benefits.
Here is what you need to keep in mind:
- Scope of profiling
- Resources required
- Integration with existing systems
- Data security and privacy
- Ongoing management
- User adoption
- Metrics and measurement
- Data governance and stewardship
Let us take a look at the above factors in brief:
1. Scope of profiling #
Data profiling can range from basic checks (like minimum, maximum, average, and number of null values) to complex analysis (like determining relationships between different data elements or identifying patterns and trends). The level of detail you want from your profiling efforts will influence the type of tool or service you need and the cost and complexity of the task.
2. Resources required #
Comprehensive data profiling can require significant resources, including specialized software and skilled personnel. Consider whether you have the necessary resources or if you need to allocate an additional budget or hire additional staff.
3. Integration with existing systems #
How well will data profiling processes and tools integrate with your existing systems? You need a solution that will work smoothly with your current technology stack and workflows.
4. Data security and privacy #
Data profiling often involves analyzing sensitive or private information. Consider the measures you need to put in place to ensure data privacy and meet any regulatory requirements.
5. Ongoing management #
Data profiling isn’t a one-time task - it should be an ongoing process as your data changes and grows. Think about how you will manage this process over time and keep your data profile up-to-date.
6. User adoption #
Consider how you will ensure the adoption of data profiling insights across the organization. The best insights won’t help if they aren’t used. This may involve training and change management efforts.
7. Metrics and measurement #
Consider how you will measure the benefits and success of your data profiling efforts. This could involve tracking improvements in data quality, reductions in errors, or increased efficiency in decision-making.
8. Data governance and stewardship #
Data profiling should be part of a broader data governance and stewardship strategy. Consider how these activities align and support each other.
By keeping these considerations in mind, you’ll be better positioned to implement and benefit from data profiling in a way that best meets your needs and supports your business objectives.
Key considerations for evaluating data catalog and governance solutions with robust data profiling capabilities #
Data profiling is a crucial part of data governance because:
- It supports the establishment of standards and rules
- enforces of data quality
- Promotes data usage across the organization.
It aids in promoting data consistency, reliability, and transparency, which are the fundamental goals of data governance.
So, when evaluating a data catalog/governance solution, here are the data profiling capabilities that are important to consider:
- Data quality assessment
- Data statistics
- Pattern recognition
- Data relationship discovery
- Data classification
- Anomaly detection
- Metadata capture
- Integration capabilities
- Automated profiling
Let us look into each of the data profiling capabilities in brief:
1. Data quality assessment #
The tool should provide functionality to assess the quality of data. This includes identifying missing, inconsistent, and duplicate data, and measuring conformity to specified formats and business rules.
2. Data statistics #
The tool should provide statistical information about the data like minimum, maximum, average, median, standard deviation, etc. This aids in spotting numerical data and frequency, patterns, lengths, etc., for categorical or text-based data.
3. Pattern recognition #
The tool should be able to identify and highlight patterns within the data. This is particularly important in identifying structured data stored in unstructured fields (like emails, phone numbers, etc.).
4. Data relationship discovery #
The tool should be capable of understanding and displaying the relationships between different data elements. This includes foreign key relationships in databases, correlations between variables, and lineage across different systems.
5. Data classification #
It should be able to categorize data based on data types, patterns, or predefined rules. This is important for managing sensitive data like personally identifiable information (PII).
6. Anomaly detection #
The tool should be able to detect and highlight unusual data points or outliers that may indicate errors or exceptions that need further investigation.
7. Metadata capture #
The tool should capture and present metadata about the data, including where it came from (lineage), who has accessed it, how often it’s updated, and its level of sensitivity.
8. Integration capabilities #
The tool should integrate with a variety of data sources, systems, and platforms, allowing for a comprehensive view of data across the organization.
9. Automated profiling #
The tool should support automated and scheduled data profiling to ensure that data governance is up-to-date and consistent.
By ensuring a data catalog/governance tool has these capabilities, you can create a strong foundation for effective data governance that supports quality, consistency, transparency, and regulatory compliance.
Bringing it all together #
Data profiling is a critically important step in any data management or analytics project. So, it should come at the beginning so that you can provide an accurate project timeline estimate, ensure the availability of high-quality data, and enable data-driven decisions.
By applying data profiling in your data governance strategy, you’re on the right path to creating a robust data-driven culture within your organization. It will help you manage your data assets, meet compliance requirements, enhance operational efficiency, and drive customer satisfaction.
Benefits of data profiling: Related reads #
- Data Profiling: Definition, Techniques, Process, and Examples
- How to automate data profiling
- How to Improve Data Quality in 12 Actionable Steps?
- What is data observability: Definition, importance, framework & benefits
- Data observability vs. data monitoring: How are they different?
- What is data integrity? Definition, importance, and best practices
Share this article