Data Catalog vs. Data Lineage: Differences, Use Cases, and Evolution of Available Solutions
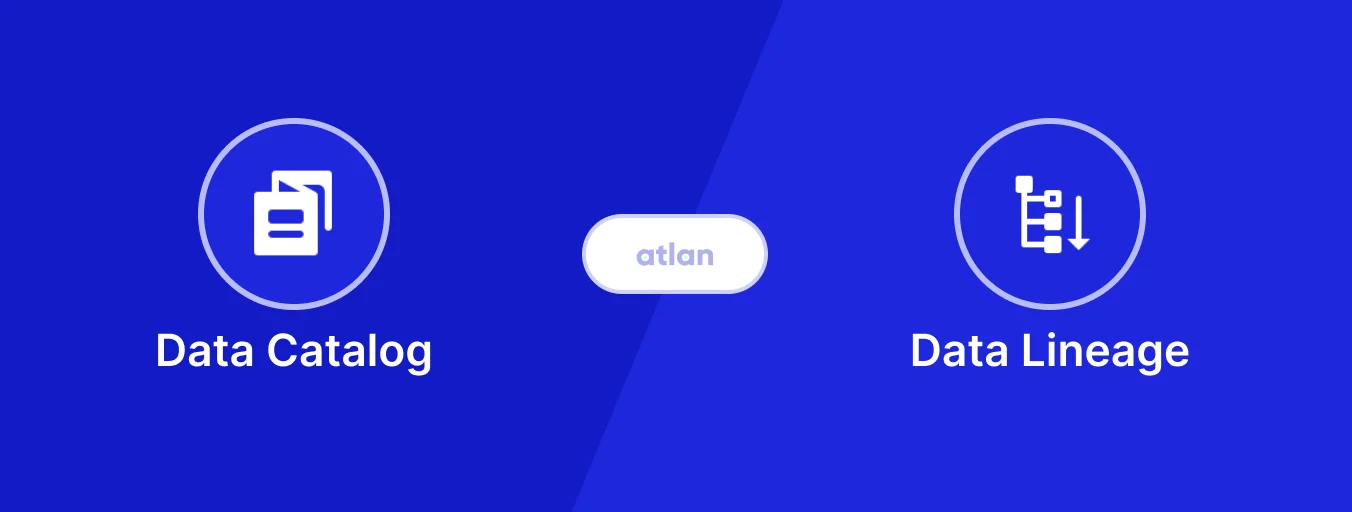
Share this article
According to IDG & Matillion, most businesses handle data from between 400 and 1,000 separate sources. Both data catalog and data lineage technology are indispensable for managing this complexity. Here’s how the two work synergistically together to empower employees to discover, access, and utilize the right data.
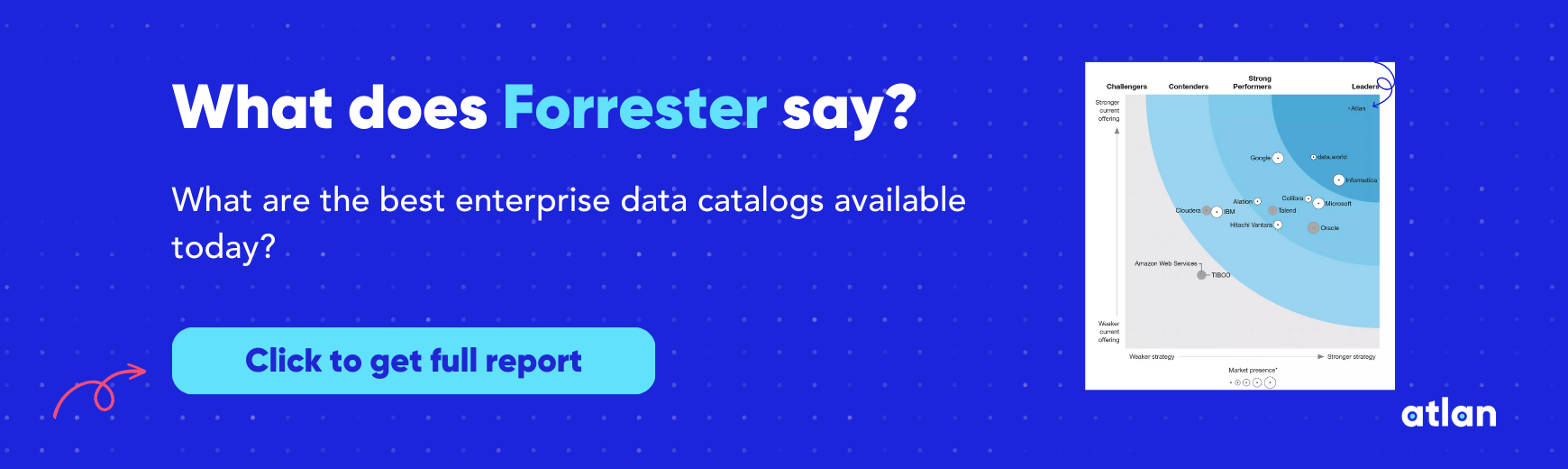
Table of Contents #
- What is a data catalog?
- What is data lineage?
- Data catalog vs. data lineage: enabling efficient use of data
- Data catalog use cases
- Data lineage use cases
- How data catalogs and data lineage work together
- Future of data catalogs and data lineage
- Conclusion: data catalog vs. data lineage
- Related reads
What is a data catalog? #
A data catalog is a workspace where users can discover and annotate data. Data catalogs contain rich metadata about an asset’s origins, purpose, and business context. They also provide fine-grained permission controls and a collaborative environment that supports editing across the company.
Data catalog integrating with diverse data sources and data tools. Image by Atlan.
The modern “third-generation” data catalog enables everyone from product managers to data scientists to track and collaboratively maintain an organization’s diverse data assets at scale. Data lineage tools then use techniques such as SQL parsing, API crawling, and custom API ingestion to track and visualize the history of these assets as they flow through the company.
Companies can select from a wide variety of open-source and commercial data catalog solutions to implement a solution that meets their needs.
What is data lineage? #
Data lineage tracks the lifecycle of your data. A good data lineage implementation tells you the origin of your data and how it’s changed over time. It tracks table-level lineage and column-level lineage, showing how your organization’s data structures change over time.
Automated data lineage: Understand how data flows from the source to the dashboards. Image by Atlan.
Data catalogs and data lineage together solve the problem of metadata management. A data catalog centralizes critical business information in a single source of truth. Lineage provides confidence that data is current and enables tracing the impact of any changes across the company.
Data catalog vs. data lineage: enabling efficient use of data #
Every company produces tons of data. And every company needs a way to account for it. The difference between a data catalog vs. data lineage lies in how both contribute to solving this problem at scale.
The data catalog provides the foundation for describing and securing access to data. Enterprise data catalogs are used by a diverse range of business users - data scientists, engineers, product managers, and executives - to discover data and generate insights essential to running the business.
Modern data problems require modern solutions - Try Atlan, the data catalog of choice for forward-looking data teams! 👉 Book your demo today
Once that’s in place, a company can track where that data came from and - just as importantly - who’s using it, both upstream and downstream. A good data lineage tool will record your data’s journey across the company over time. It enables users to identify the root causes of problems, assess the impact of changes, and implement end-to-end data governance.
Both of these pieces - data catalog and data lineage - are essential for meeting many business compliance requirements. Take the European Union’s General Data Protection Regulation (GDPR). Under GDPR, you need to know what data is Personally Identifiable Information (PII) that belongs to a customer. You also need to know all the locations where data lives - databases, support tickets, report caches, cold storage, etc. - so you can purge it upon request.
A data catalog answers the first question. Data lineage answers the second.
Data catalog use cases #
To clarify this, let’s look at the different ways you would use both a data catalog and data lineage.
The common uses for a data catalog include:
- Context plane for data
- Data discovery
- Metadata management
- Data governance
Context plane for data #
Without a data catalog, it can be hard - if not impossible - to know what data you have. This complicates every data-related task in your company from delivering data-driven apps to ensuring regulatory compliance.
Data that sits dormant or undiscovered is called “dark data.” Veritas estimates that over half of a company’s data is dark. It sits unused or underutilized, its value untapped.
A data catalog eliminates the dark data problem by accounting for all data sources in a central repository. This basic capability enables all other data catalog use cases, such as discovery and metadata management.
Data discovery #
Data discovery becomes much easier once all data sources are unified in a data catalog. Users can create natural language queries using a data catalog’s search interface to find the data they need within minutes instead of days.
Metadata management #
Data catalogs don’t just track data, but also metadata - i.e., data about data. Metadata includes everything from ownership and description information to data classifications used in regulatory compliance. Rich metadata increases data’s value and quality by certifying its timeliness, accuracy, and business purpose.
Data catalogs enable crowdsourcing metadata management across the company. With all data tracked in one single source of truth, more employees can not only discover data but also help enrich it.
Data governance #
Regulations such as GDPR require securing customer’s Personally Identifiable Information (PII) and deleting it when you no longer have a business use requiring retention. With a data catalog, you can classify data, measure how much data is appropriately tagged, and issue alerts when you detect potential anomalies (e.g., an untagged field holding what appears to be a credit card number).
Data lineage use cases #
Now let’s look at the most common use cases for data lineage:
- Root cause analysis
- Impact analysis
- Automating data maintenance tasks
Root cause analysis #
What happens if a data transformation process changes the format of a field in a database? Or a data engineer deletes a column that she thought “no one was using”?
In most companies, this results in reports and data pipelines that are downstream consumers of the data source breaking. As a result, multiple people spend hours figuring out what went wrong and deploying fixes.
Data lineage enables tracking such problems down to their root cause. Because you can see how data flows from data sources to data consumers, you can easily pinpoint which process made the change and fix it at its source.
Impact analysis #
Root cause analysis is good. But preventing breaking changes in the first place is even better. With data lineage, data engineers can see exactly which tables and columns are being used by downstream consumers. The data team can then work with these stakeholders closely whenever it needs to deploy a breaking change.
Automating data maintenance tasks #
Companies can leverage data lineage to automate tasks that would otherwise take days, weeks, or months to complete. For example, they can utilize the auditing trails created by data lineage to help certify that they handle data according to regulatory requirements and their own data governance standards.
How data catalogs and data lineage work together #
This case of Postman, makers of the popular API development and testing tool, shows how a data catalog and data lineage evolve together in practice.
The company originally struggled with duplicated metrics and daily Slack user inquiries about data provenance. Data duplication and user confusion sowed distrust in the company’s data.
As Postman’s Prudhvi Vasa puts it, “building trust is hard, but losing it is easy—it just takes one mistake.”
To solve this, it first tried cataloging data in a Confluence document, and then Google Sheets. It quickly outgrew both solutions. They eventually moved to Atlan as their dedicated data cataloging solution.
Once the company had its data in a data catalog, it built its lineage system over it. This involved gathering information on their data’s origins (a process you can perform manually but that’s accomplished much more efficiently via automation.
Postman could finally ask - and answer - questions about their data’s origins and its interconnections. Users could now discover data easily. They could also see how a proposed change to a data asset would affect other assets and users across the company.
Netflix faced and solved a similar set of challenges. In their case, they worked backward from the goal of developing a comprehensive data lineage network. That first entailed creating a flexible data catalog representing the company’s diverse assets. They then developed a separate data lineage model that users can navigate via a graph database.
Future of data catalogs and data lineage #
Creating a data catalog is only the first step. No one will use a data catalog unless it’s usable. And that means moving away from the notion of a data catalog as a standalone entity.
Data catalogs will continue to evolve to bring metadata to users where they work - e.g., in Slack or their favorite BI tool. Data catalogs will become repositories of active metadata that cut across all of a company’s data infrastructure and business productivity tools.
Similarly, data lineage networks are indispensable - but only if users can easily visualize the journey their data takes. A simple flow visualization is a good start. But in the future, we’ll see more data lineage systems take Netflix’s lead, representing lineage flows as searchable, navigable graphs.
Conclusion: data catalog vs. data lineage #
In today’s businesses, data is repeatedly imported, exported, transformed, stored, and displayed in multiple, redundant ways. Without a way to catalog and trace this movement, finding accurate data can become a nightmare - and compliance becomes nearly impossible.
Understanding data catalog vs. data lineage means understanding the two different but essential roles each plays in solving this problem. A data catalog tells you what you have. Data lineage tells you where everything is and how it all relates. Using both solutions, your company can ensure data accuracy, implement data governance, and manage business change.
Want to go deeper? Learn more about the modern data stack and its components - and how data catalog and data lineage fit within the larger picture.
Data catalog vs. Data lineage: Related reads #
- Data Lineage 101: Importance, Use Cases, and Their Role in Governance
- Data Catalog Lineage: Core Components & Business Benefits
- Data Catalog vs. Data Dictionary: Definitions, Differences, Benefits & Why Do You Need Them?
- 5 Main Benefits of Data Catalog & Why do you Need it?
- What Is a Data Catalog? & Do You Need One?
- Best Alation Alternative: 5 Reasons Why Customers Choose Atlan
- Data Inventory vs. Data Catalog: Definitions, Differences, and Examples
- What Is the Difference between Data cataloging and Metadata Management?
Share this article