Data Lineage & Data Observability: Why Are They Important?
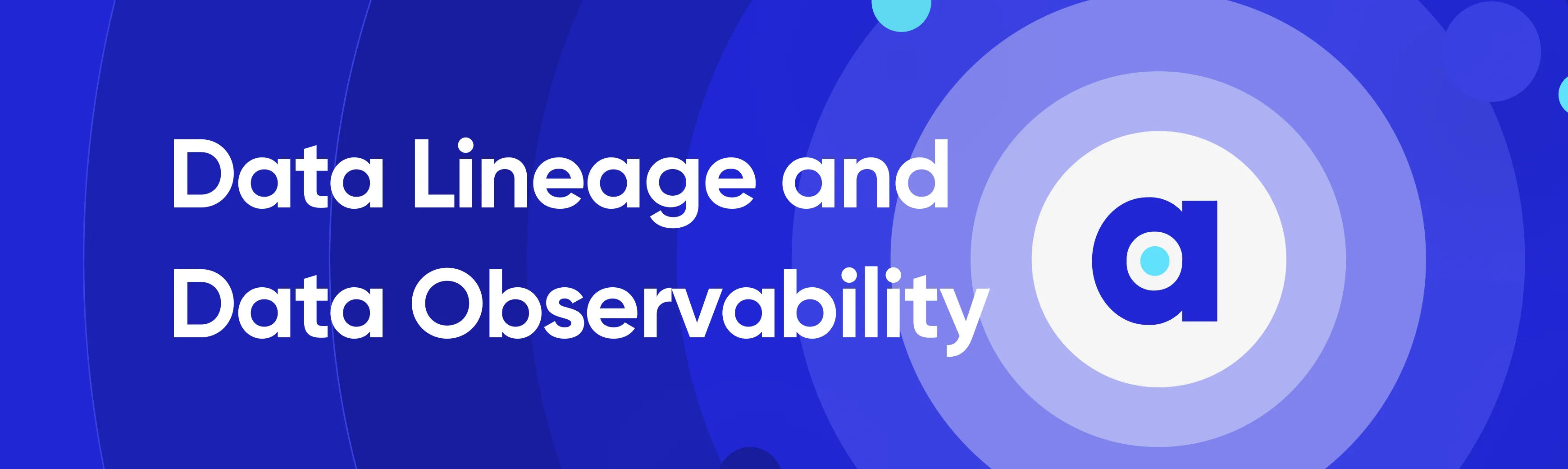
Share this article
Data lineage in data observability refers to the ability to trace the origin, movement, and transformation of data throughout its lifecycle within a data system. It provides a detailed record of the data’s journey from its source to its destination, highlighting every step and transformation it undergoes along the way.
This article delves into the ten critical reasons why data lineage is an indispensable component of data observability and how it fosters a deeper understanding of data flow, quality, and overall data health within modern enterprises.
Let’s dive in!
Table of contents #
- What is data lineage in data observability?
- Difference between data lineage and data observability
- Benefits
- Why is data lineage important for data observability?
- How is data lineage used in data observability?
- Summary
- Related reads
What is data lineage in data observability? #
Data lineage is a critical aspect of data observability as it offers insights into how data is processed, integrated, and consumed within a data infrastructure. It helps data engineers, data scientists, and other stakeholders understand and validate the data’s reliability, quality, and compliance with business requirements.
Key components of data lineage in data observability include:
- Data source
- Data transformation
- Data storage
- Data consumers
- Data processing steps
- Data relationships
- Metadata and annotations
Let us understand each of them in detail:
1. Data source #
The starting point of data lineage is the data source, where data originates. This could be a database, application, sensor, or any other data-producing system.
2. Data transformation #
As data moves through the data infrastructure, it often undergoes various transformations, such as cleansing, aggregation, and enrichment. Data lineage tracks each of these transformations, documenting how data changes at each stage.
3. Data storage #
Data lineage shows where and how data is stored at different stages of its journey. It identifies intermediate and final storage locations, including data warehouses, data lakes, or other repositories.
4. Data consumers #
Data lineage tracks which applications, reports, or users consume the data. It helps identify downstream dependencies and users who rely on specific datasets.
5. Data processing steps #
Data lineage provides a step-by-step view of data processing, highlighting the sequence of actions taken on the data. This can include ETL (Extract, Transform, Load) processes, data pipelines, and data workflows.
6. Data relationships #
Data lineage reveals relationships between different datasets and tables, illustrating how they are connected and interrelated.
7. Metadata and annotations #
Data lineage is often enriched with metadata and annotations, providing additional context and insights into the data’s characteristics and usage.
What is the difference between data lineage and data observability? #
Data lineage and data observability are related concepts, but they serve different purposes in the context of data management and analytics. Here’re the key differences between them:
1. Focus #
Data lineage is focused on visualizing and tracking the data flow, from its origin to its destination, providing a historical view of data movement. Data observability, on the other hand, focuses on real-time monitoring and understanding of data system behavior and performance.
2. Granularity #
Data lineage provides a detailed, step-by-step view of data flow and transformations. Data observability looks at aggregated metrics and insights about data systems and processes to identify patterns and trends.
3. Application #
Data lineage is particularly useful for understanding data provenance, data quality assurance, and impact analysis. Data observability is essential for detecting issues, optimizing data workflows, and ensuring data system reliability in real time.
4. Representation #
Data lineage is often represented visually as a data flow diagram, while data observability is represented through dashboards, alerts, and monitoring tools showcasing metrics and logs.
In summary, data lineage focuses on understanding the historical flow of data, whereas data observability is about real-time monitoring and insights into data system behavior. Both concepts play crucial roles in data management, ensuring data accuracy, reliability, and efficiency in different ways.
What are the benefits of data lineage in data observability? #
Now, let us look at the key benefits of data lineage in data observability, which include:
- Data quality assurance
- Compliance and governance
- Troubleshooting and root cause analysis
- Impact analysis
- Auditing and lineage validation
Let us understand each of them in detail:
1. Data quality assurance #
Data lineage helps identify potential data quality issues by tracing data transformations and highlighting any discrepancies or errors introduced during processing.
2. Compliance and governance #
Data lineage aids in compliance efforts by providing a transparent view of data movement, ensuring data privacy and regulatory requirements are met.
3. Troubleshooting and root cause analysis #
When issues arise, data lineage facilitates quicker troubleshooting and root cause analysis by pinpointing the source of problems and tracking the data flow.
4. Impact analysis #
Data lineage allows organizations to understand the impact of changes to data sources, transformations, or data models on downstream processes and applications.
5. Auditing and lineage validation #
Data lineage serves as an audit trail, enabling stakeholders to verify the accuracy and integrity of data for regulatory and business purposes.
In summary, data lineage is a critical aspect of data observability, providing valuable insights into data movement, transformations, and relationships within a data system. It plays a significant role in ensuring data quality, compliance, and trustworthiness, enabling organizations to make data-driven decisions with confidence.
Why is data lineage important for data observability? #
Data lineage is of paramount importance for data observability due to several key reasons:
- Data quality assurance
- Root cause analysis and troubleshooting
- Compliance and governance
- Impact analysis
- Auditing and lineage validation
- Monitoring and performance optimization
- Data governance and data stewardship
- Collaboration and data sharing
- Risk mitigation
- Data strategy and decision making
Let us understand each of them in detail:
1. Data quality assurance #
As we mentioned above in the ‘Benefits of data lineage in data observability’ section, data lineage provides a clear and transparent view of how data moves and is transformed throughout the data ecosystem. It helps identify potential data quality issues, ensuring that data remains accurate, consistent, and reliable at every stage of its journey.
2. Root cause analysis and troubleshooting #
In case of data-related issues or errors, data lineage enables quick and efficient root cause analysis. By tracing the data’s path, data engineers and analysts can identify where and how problems occurred, facilitating faster troubleshooting and resolution.
3. Compliance and governance #
Data lineage plays a vital role in ensuring compliance with data privacy regulations and other legal requirements. It helps organizations track data lineage to prove that data is handled appropriately, maintaining data security and compliance.
4. Impact analysis #
Data lineage allows organizations to understand the potential impact of changes to data sources, transformations, or data models on downstream processes and applications. This helps in predicting the consequences of data changes and making informed decisions.
5. Auditing and lineage validation #
Data lineage serves as an audit trail that allows stakeholders to verify the accuracy and integrity of data. It aids in conducting data lineage validation, which is essential for regulatory and business auditing purposes.
6. Monitoring and performance optimization #
Data lineage provides insights into the data flow and data processing steps. This information is valuable for monitoring data pipelines and data workflows, optimizing their performance, and identifying areas for improvement.
7. Data governance and data stewardship #
Data lineage helps establish effective data governance practices by tracking data ownership, usage, and access. It aids in defining data stewardship roles and responsibilities for data products and domains.
8. Collaboration and data sharing #
Data lineage fosters collaboration between different teams and departments. It allows domain experts to understand the origin and transformations of shared data, promoting effective data sharing and collaboration.
9. Risk mitigation #
By providing visibility into data movement and transformations, data lineage helps mitigate the risks associated with data errors, data inconsistencies, and unauthorized data access.
10. Data strategy and decision making #
Understanding data lineage enables data-driven decision-making. It allows organizations to identify data bottlenecks, prioritize data improvements, and align data initiatives with business goals.
In summary, data lineage is vital for data observability as it offers transparency and visibility into the entire lifecycle of data. It empowers organizations to maintain data quality, ensure compliance, facilitate troubleshooting, and make informed data-related decisions. By leveraging data lineage, organizations can build a robust data observability framework, enhancing the reliability, efficiency, and value of their data assets.
How is data lineage used in data observability? #
In data observability, data lineage is utilized to provide a clear and comprehensive understanding of how data moves and transforms within a data system.
Correct usage of data lineage in data observability involves the following key practices:
1. Monitoring data flow #
Data lineage is used to monitor the flow of data from its source to its destination.
This involves tracking data as it moves through data pipelines, ETL (Extract, Transform, Load) processes, and data workflows.
Data engineers and analysts can use data lineage to ensure that data is flowing smoothly and accurately between different components of the data ecosystem.
2. Data quality assurance #
Data lineage is an essential tool for data quality assurance. By tracing the data’s journey, data observability teams can identify any data quality issues or transformations that might introduce errors.
Data lineage helps ensure that data remains consistent, accurate, and trustworthy throughout its lifecycle.
3. Troubleshooting and root cause analysis #
In the event of data-related issues, data lineage serves as a valuable resource for troubleshooting and root cause analysis.
When discrepancies or errors occur, data engineers can follow the data lineage to pinpoint where the problem originated and understand the series of steps that led to the issue.
4. Impact analysis #
Data lineage is used to conduct impact analysis, allowing organizations to assess the potential consequences of changes to data sources, data models, or data transformations.
This helps in making informed decisions about data changes and updates.
5. Compliance and governance #
Data lineage is crucial for ensuring compliance with data privacy regulations and other legal requirements.
It provides a transparent record of data movement and processing, aiding in data governance practices and compliance audits.
6. Data documentation and metadata #
Data lineage is often enriched with metadata and annotations, providing additional context and insights into the data’s characteristics and usage. This data documentation facilitates better understanding and management of data assets.
7. Collaboration and communication #
Data lineage promotes collaboration between different teams and stakeholders. It helps data engineers, data scientists, and domain experts understand the flow of data, fostering better communication and collaboration around data-related tasks.
8. Monitoring data pipelines and workflows #
Data lineage is used to monitor the performance of data pipelines and workflows. By observing the data flow and processing steps, organizations can identify potential bottlenecks and areas for optimization.
9. Data security and auditing #
Data lineage aids in data security efforts by tracking data access and usage. It supports auditing and compliance requirements by providing an audit trail of data movement and transformations.
10. Data strategy and decision making #
Data lineage insights support data-driven decision-making. Understanding data lineage allows organizations to prioritize data improvements, align data initiatives with business goals, and optimize data processes.
By effectively using data lineage in data observability, organizations can enhance the reliability, efficiency, and governance of their data systems. It empowers data teams to gain better insights into data behavior, optimize data processes, and maintain high data quality standards.
Summarizing it all together #
Data lineage and data observability are two essential aspects of data management that complement each other to ensure the reliability, integrity, and efficiency of data systems.
The relationship between data lineage and data observability is symbiotic. Data lineage provides the historical context and understanding of how data has been processed and transformed over time. This knowledge is invaluable for data observability practices as it helps in setting up appropriate monitoring and alerts to track data system behavior effectively.
In conclusion, data lineage and data observability are essential components of a robust data management strategy. While data lineage provides the historical context and static view of data flow, data observability focuses on real-time monitoring and insights into data system behavior. Together, they offer a complete picture of the data ecosystem, ensuring data reliability, efficiency, and integrity for data-driven organizations.
Data lineage and data observability: Related reads #
- Automated Data Lineage: Making Lineage Work For Everyone
- Open Source Data Lineage Tools: 5 Popular to Consider in 2023
- Amundsen Data Lineage Setup with dbt
- Data lineage for Snowflake, Redshift, and BigQuery
- Data Catalog vs. Data Lineage: Differences, Use Cases, and Evolution of Available Solutions
- Data Lineage: An In-Depth Guide to Understanding the Importance of Tracking Your Data’s Journey
Share this article