9 Notable Data Collection Techniques for Data Teams in 2024!
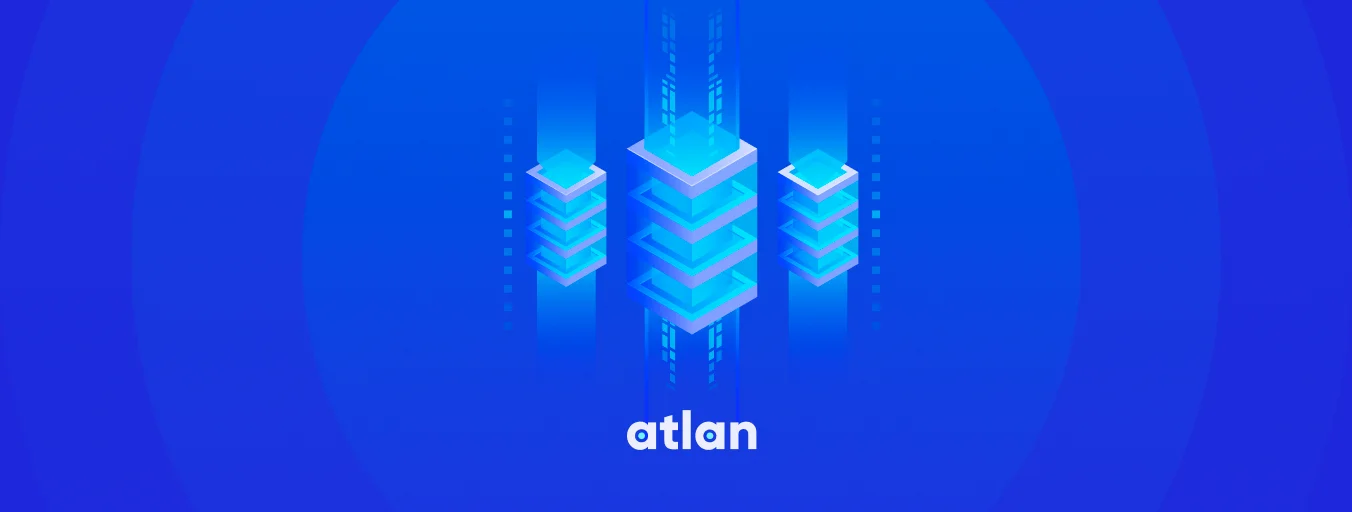
Share this article
Statista reported that the world’s data volume is predicted to reach 181 zettabytes by 2025, the sheer magnitude of this data can be daunting without a clear checkpoint. Data collection techniques are fundamental in assessing, quantifying, and managing these vast surges of data.
Modern data problems require modern solutions - Try Atlan, the data catalog of choice for forward-looking data teams! 👉 Book your demo today
Data collection is crucial in managing vast data surges because it provides a structured approach to gathering relevant information, ensuring that the immense volume of data is not just vast but valuable, organized, and actionable.
Without systematic data collection, massive data inflows can become chaotic and unmanageable, leading to lost insights and missed opportunities.
These notable data collection techniques for data teams would guide you towards methodologies that promise precision, efficiency, and innovation. But why should you care about these techniques? Let’s delve a bit deeper.
Table of contents #
- What is data collection?
- 5 Reasons why data collection is important
- What are the types of data collection?
- 9 Notable data collection techniques for data teams
- 9 Key challenges in data collection techniques
- Summary
- Related reads
What is data collection? #
Data collection is the foundation of research, analysis, and decision-making across various disciplines. By sourcing information from various channels, data collection ensures accuracy and informed interpretation.
Whether qualitative or quantitative, this information provides valuable insights. Ultimately, it transforms raw data into meaningful knowledge, driving innovation and understanding in our data-driven world.
5 Reasons why data collection is important #
In the age of information, data serves as a powerful tool that can guide decisions, shape policies, and transform industries. But before any of these changes can occur, the raw data must be meticulously collected.
Understanding the value and importance of data collection can illuminate its central role in modern society. Below, we delve into the key reasons why data collection is so crucial:
- Informed decision-making
- Precision and accuracy
- Monitoring and evaluation
- Enhancing transparency and credibility
- Academic and scientific research
Now let’s explore the importance of data collection in detail.
1. Informed decision-making #
Informed decision-making plays a pivotal role in ensuring strategic precision and minimizing uncertainties. By harnessing the power of data, decisions transform from mere hunches to well-reasoned actions.
As businesses and cities alike turn to data, the value of an informed approach shines through, fostering clearer strategies and diminishing the realm of guesswork. Through data collection, the pathway to informed decisions becomes clearer, safer, and more strategic.
- Clarity in strategy: Decisions anchored in data are often more accurate and effective. Whether it’s a business choosing its next market or a city planning its infrastructure, reliable data offers a clearer picture and aids in mapping out strategic steps.
- Reducing guesswork: Instead of relying on intuition or assumptions, stakeholders can make choices rooted in empirical evidence, minimizing the risks associated with guesswork.
2. Precision and accuracy #
In the vast realm of data and analytics, precision and accuracy are more than mere buzzwords. They stand as pillars that uphold the integrity and relevance of collected information.
Without precision and accuracy, data can be misleading, leading to erroneous conclusions and misguided strategies. Here’s why these elements are pivotal in the data collection process:
- Reliable outcomes: Systematic data collection ensures the information obtained is both precise and dependable. This boosts the confidence of analysts, researchers, and decision-makers in the results derived from such data.
- Standardized information: Uniformity in data collection means that different stakeholders or researchers can achieve consistent findings under similar circumstances.
3. Monitoring and evaluation #
Monitoring and evaluation serve as two fundamental processes that, when effectively implemented, can guide an organization towards its desired goals. Here’s an in-depth look into these processes:
- Track progress: Organizations and institutions can monitor their progress towards set goals by consistently collecting and analyzing data. For instance, a business might track its monthly sales to gauge growth.
- Evaluate impact: Data provides a baseline and subsequent measurements to assess the effectiveness of interventions, policies, or strategies. This helps in understanding what’s working and what needs to be revised.
4. Enhancing transparency and credibility #
In an era where information is abundant yet misinformation is rampant, the credibility of claims is under constant scrutiny. Herein lies the invaluable role of data. When assertions are underpinned by clear, verifiable data, it elevates the transparency and credibility of those claims.
Two primary aspects emphasize the significance of data in bolstering transparency and credibility:
- Building trust: When claims or decisions are backed by data, they gain more credibility. This builds trust among stakeholders, clients, or the general public.
- Accountability: Data serves as evidence. Institutions can use it to showcase their achievements or to hold themselves accountable for their actions.
5. Academic and scientific research #
The pursuit of knowledge and understanding is a fundamental aspect of human curiosity. In the academic and scientific spheres, this quest takes a structured and rigorous form, where data collection becomes paramount.
Two pivotal components underscore the significance of data collection in these fields: the expansion of knowledge and the principle of replicability.
- Expand knowledge: In academia and scientific fields, data collection is essential for experiments, case studies, and observational research. It helps in validating hypotheses and contributes to the body of knowledge in various disciplines.
- Replicability: Proper data collection allows other researchers to replicate studies, a cornerstone of scientific integrity.
Data collection is the foundation upon which our modern, information-driven world stands. Its importance spans sectors, industries, and disciplines. By prioritizing meticulous data collection, we pave the way for informed decisions, accurate insights, and a better understanding of the complex world around us.
What are the types of data collection? #
Data collection plays a fundamental role in research, business, and decision-making processes. Understanding the various types of data collection is crucial for choosing the method that best suits the specific needs of a project or study.
Here, we delineate the prominent types of data collection:
- Quantitative data collection
- Qualitative data collection
- Primary data collection
- Secondary data collection
- Cross-sectional data collection
- Longitudinal data collection
- Observational data collection
- Experimental data collection
- Big data collection
Let’s delve deep into the types of data collection.
1. Quantitative data collection #
Quantitative data collection pertains to the systematic gathering of numerical data. This type of data, distinct for its ability to be measured and counted, provides a foundation upon which statistical analysis can be performed.
The objective nature of quantitative data makes it an ideal choice for drawing generalized conclusions and identifying patterns and relationships among variables.
Methods
- Surveys with close-ended questions
- Observations of quantifiable behaviors
- Experiments that produce numerical results
2. Qualitative data collection #
Qualitative data collection centers on capturing non-numerical data. This approach prioritizes depth over breadth, providing insights into the nuances of subjects’ experiences, feelings, beliefs, and motivations.
By exploring complex phenomena in their natural settings, it paints a comprehensive picture of underlying contexts, intentions, and interpretations.
Methods
- In-depth interviews
- Focus groups
- Observational research
- Open-ended surveys
- Content analysis
3. Primary data collection #
Primary data collection refers to the gathering of fresh, firsthand data that hasn’t been previously collected or processed. It is data derived directly from its source, tailor-made to address a particular research question or objective.
Since primary data is curated for a specific purpose, it holds a higher degree of relevancy and accuracy for the research at hand, making it invaluable for in-depth analyses.
Methods
- Surveys and questionnaires
- Interviews
- Observations
- Experiments
4. Secondary data collection #
Secondary data collection refers to the practice of sourcing and using data that was previously collected for a different research objective or purpose. This contrasts with primary data collection, where data is gathered firsthand for specific research or analysis.
Secondary data is often historical, providing a backdrop against which new research can be contextualized.
Methods
- Literature reviews
- Archival research
- Database exploration
5. Cross-sectional data collection #
Cross-sectional data collection is akin to taking a photographic snapshot. Instead of capturing changes over time, it focuses on understanding the situation or phenomenon at one specific instance.
This approach is especially useful for comparisons, allowing researchers to discern patterns, distributions, and relationships within the captured data.
Methods
- One-time surveys
- Snapshots of specific indicators
6. Longitudinal data collection #
Longitudinal data collection is characterized by its emphasis on temporal continuity. It entails the repeated observations or measurements of the same set of individuals or sample groups over extended periods.
By focusing on the same subjects, researchers can obtain clearer insights into the evolution of specific behaviors, conditions, or variables, effectively capturing the ebbs and flows of real-life phenomena.
Methods
- Panel studies
- Cohort studies
- Time-series studies
7. Observational data collection #
Observational data collection revolves around the principle of watching, noting, and analyzing behaviors and events without direct intervention or alteration of the environment. It’s a method deeply rooted in objectivity, as researchers strive to be passive onlookers rather than active participants.
This ensures that the data collected represents the subjects’ authentic behaviors, untainted by external influences or biases.
Methods
- Direct or participant observation
- Non-participant observation
8. Experimental data collection #
Experimental data collection involves the gathering of data in a controlled setting where specific variables can be manipulated to observe their effects on other variables.
This technique is vital for establishing cause-and-effect relationships, and it provides a clearer understanding of the mechanisms at play in particular situations.
Methods
- Laboratory experiments
- Field experiments
9. Big data collection #
Big Data collection pertains to the accumulation of vast amounts of data from a multitude of sources. The three defining characteristics of big data are often referred to as the three V’s.
- Volume: The enormous quantity of data generated
- Variety: The different types of data, be it structured (like databases) or unstructured (like social media posts)
- Velocity: The rapid pace at which new data is generated and collected
Methods
- Web scraping
- Social media mining
- Transactional data tracking
- IoT (Internet of Things) sensors
The method chosen for data collection largely depends on the objectives of the study, the nature of the data required, and the resources available. Understanding the advantages and limitations of each type helps researchers and professionals ensure the effectiveness of their data collection process.
9 Notable data collection techniques for data teams #
Data teams, central to the data-driven culture of organizations, are responsible for the efficient gathering, processing, and analysis of data. Their role is pivotal in ensuring the data is accurate, relevant, and actionable.
The techniques they employ for data collection determine the quality and utility of the insights derived. Here, we’ll explore some crucial data collection techniques that data teams commonly use:
- Web scraping
- API integration
- Database mining
- Direct instrumentation
- Surveys and feedback forms
- A/B testing
- Log file analysis
- Mobile data collection
- Data warehousing
Let us understand the data collection techniques for data teams in detail.
1. Web scraping #
Web scraping, at its core, involves the automated process of navigating the web and extracting specific data from websites. This is achieved using specialized software or scripts tailored to retrieve information from the webpage’s underlying code.
Unlike manual data collection, web scraping can swiftly capture data across numerous web pages, making it a preferred method for large-scale data extraction tasks.
- Market research, competitive analysis, sentiment analysis.
- Benefits: Access to large volumes of real-time data, capability to extract data from dynamic web pages.
2. API integration #
APIs function as a set of rules and tools that allow applications to communicate with each other. When we talk about API integration, we refer to the process of connecting different software systems using these APIs.
This technique allows for the retrieval of data from various applications, platforms, or services in a unified manner. Instead of isolated silos of information, API integration provides a consolidated and streamlined data view.
- Integrating third-party services, collecting data from social media platforms, accessing cloud-based data storage.
- Benefits: Direct and structured data access, scalability, real-time data retrieval.
3. Database mining #
Database mining, sometimes referred to as ‘data mining,’ is the process of discovering patterns, correlations, and insights within large sets of data stored in databases. By utilizing algorithms and statistical methods, this technique transforms raw data into meaningful information.
The objective is not just to access data but to unveil hidden patterns, relationships, and anomalies that might not be evident at a surface glance.
- Customer relationship management, trend analysis, fraud detection.
- Benefits: Working with structured datasets, in-depth analyses, uncovering patterns in large volumes of data.
4. Direct instrumentation #
Direct instrumentation involves the use of specialized sensors or instruments to automatically gather data without manual intervention. These instruments, tuned to specific tasks, provide a conduit to translate physical occurrences or changes into quantifiable data points.
The transition from an analog event, like a change in temperature, to a digital record, such as a data log entry, epitomizes this technique.
- IoT devices, environmental monitoring, manufacturing processes.
- Benefits: Real-time data capture, high precision, continuous data streams.
5. Surveys and feedback forms #
Surveys and feedback forms are systematic tools designed to gather structured feedback. Typically consisting of a series of questions or prompts, they allow respondents to provide information about their experiences, perceptions, and preferences.
Depending on the need, these can be distributed physically, emailed, or hosted online, enabling easy access and completion.
- Customer satisfaction analysis, product development, employee engagement metrics.
- Benefits: Direct insights from respondents, customizable to specific research questions, scalable to large audiences.
6. A/B testing #
A/B testing, also known as split testing, is a method wherein two versions of a webpage, product, or service are presented to different segments of users simultaneously. The performance of each version is then tracked based on specific metrics, such as conversion rates, click-through rates, or user engagement levels.
The version that achieves better results, as per the predefined objectives, is deemed the more effective one.
- Website optimization, marketing strategies, product feature testing.
- Benefits: Empirical decision-making, direct performance comparison, improved user experiences.
7. Log file analysis #
Log file analysis involves the systematic review of log files. These files, automatically generated by servers, applications, or devices, act as a detailed record of all events, transactions, and interactions that take place within a system.
They’re akin to a black box in an airplane, capturing a timeline of events leading up to any particular moment.
- Website traffic analysis, cybersecurity, system performance optimization.
- Benefits: Detailed user activity trails, diagnostics, security monitoring.
8. Mobile data collection #
Mobile data collection involves harnessing the power and ubiquity of mobile devices to gather information. This technique utilizes specially designed apps or mobile-optimized forms to efficiently collect data on-the-go.
Given the widespread use of smartphones and tablets, this approach ensures that data can be collected in real-time, in varied locations, and directly from users.
- Field research, real-time feedback, location-based studies.
- Benefits: Flexibility in data collection, geolocation capabilities, direct user-device interaction insights.
9. Data warehousing #
Data warehousing refers to the practice of consolidating diverse data from multiple sources into a single, central repository. This repository is specifically designed to support business intelligence activities, facilitating quick query and analysis processes.
Through the warehousing approach, data is not just stored but organized, cleansed, and made readily accessible.
- Business intelligence, historical data analysis, integrative insights.
- Benefits: Centralized data access, improved data quality, comprehensive analyses.
In the age of big data, the techniques employed by data teams are becoming increasingly sophisticated and diversified. By leveraging the right data collection techniques, data teams not only ensure the accuracy and relevancy of the data but also empower organizations to harness insights that drive impactful decisions. Staying updated with these techniques and continuously refining them is essential for data teams to remain effective and valuable in their roles.
9 Key challenges in data collection techniques #
In the data-centric world of today, data teams are at the forefront of harnessing the potential of information to drive insights and strategy. However, while the benefits of data collection techniques are numerous, they do not come without their set of challenges.
Let’s explore the hurdles that data teams often encounter in their data collection journey.
- Data quality and accuracy
- Volume overwhelm
- Integration issues
- Data security concerns
- Real-time collection difficulties
- Ethical dilemmas
- High costs
- Skill and training gaps
- Evolution of data sources
Let us discuss the challenges of data collection techniques in detail.
1. Data quality and accuracy #
- Ensuring consistent data quality can be a formidable task.
- Challenge: Inaccurate or outdated data can lead to misleading insights, rendering the entire analysis counterproductive.
2. Volume overwhelm #
- The sheer volume of data available can sometimes be overwhelming.
- Challenge: Sifting through vast amounts of data to identify what’s relevant can be time-consuming and resource-intensive.
3. Integration issues #
- Merging data from diverse sources often presents compatibility issues.
- Challenge: Integration can be complex, especially when dealing with different data formats, leading to potential data loss or misinterpretation.
4. Data security concerns #
- Collecting and storing data brings about security and privacy concerns.
- Challenge: Ensuring robust protection against breaches, and compliance with data protection regulations, adds layers of complexity to the collection process.
5. Real-time collection difficulties #
- Real-time data collection is sought after for its immediate insights.
- Challenge: Implementing real-time collection mechanisms without causing system lags or inaccuracies can be technically challenging.
6. Ethical dilemmas #
- Collecting data, especially personal data, presents ethical challenges.
- Challenge: Balancing the need for comprehensive data with respect for privacy and informed consent can be a tightrope walk.
7. High costs #
- Some advanced data collection techniques require significant investments.
- Challenge: Procuring, implementing, and maintaining sophisticated data collection tools can strain an organization’s budget.
8. Skill and training gaps #
- Advanced data collection techniques demand specialized knowledge.
- Challenge: The scarcity of skilled professionals and the need for continuous training can pose staffing and productivity challenges.
9. Evolution of data sources #
- With the rapid evolution of technology, data sources continually change and expand.
- Challenge: Adapting to these evolving sources, and modifying collection techniques accordingly, requires agility and foresight.
The challenges associated with data collection techniques are as intricate as the techniques themselves. However, recognizing these challenges is the first step toward addressing them. By actively seeking solutions and maintaining an adaptive mindset, data teams can navigate these obstacles, ensuring that their data collection endeavors remain robust, insightful, and valuable.
Summary #
Data collection lies at the heart of any analytical endeavor. For data teams, employing the right techniques is not just a procedural necessity but the linchpin to unearthing actionable insights and driving meaningful change.
As the digital landscape evolves, so do the methods of data collection, necessitating that data teams remain adaptive, informed, and discerning. Embracing a comprehensive approach to data collection ensures that the derived insights are accurate, relevant, and reflective of the complexities of the real world.
As organizations venture further into the data-driven era, the expertise of data teams in employing optimal data collection techniques will be paramount to their success and innovation. The journey of transforming raw data into impactful strategies begins with effective collection, making it an invaluable skill in the arsenal of modern-day data professionals.
Related reads #
- Data Quality Explained: Causes, Detection, and Fixes
- Data Quality Dimensions: A Primer on Data Quality Measurement
- What is Data Governance? Its Importance, Principles & How to Get Started?
- 8 Data Governance Standards Your Data Assets Need Now
- Data Governance vs Data Security: 7 Key Differences in 2024
- 7 Data Integrity Best Practices You Need to Know
Share this article