Data Quality Dimensions: Do They Matter in 2024 & Beyond?
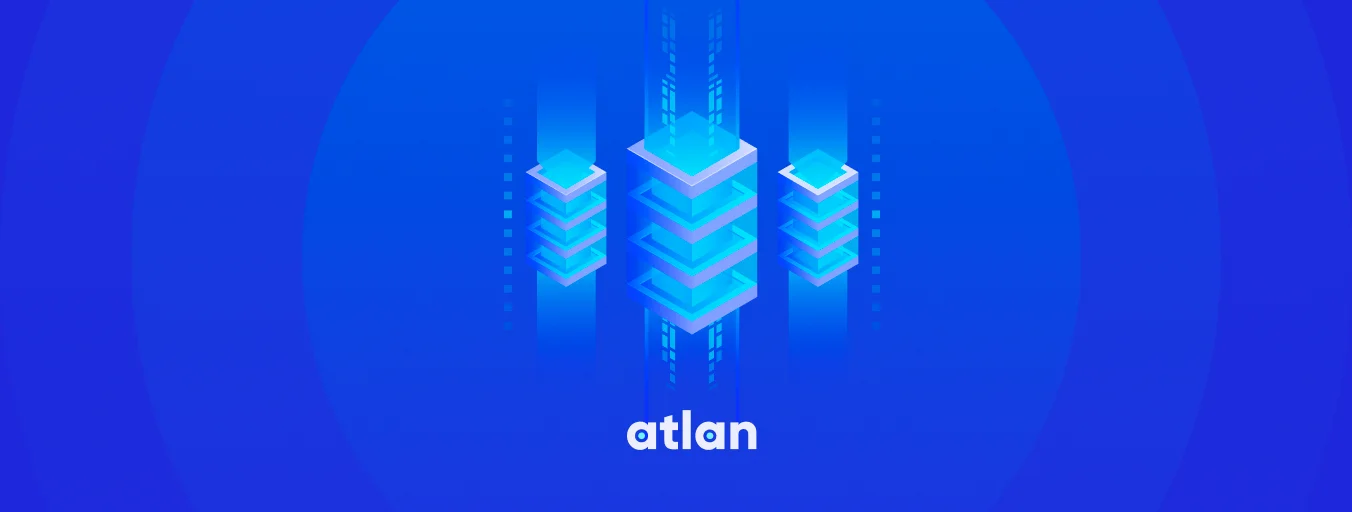
Share this article
According to Gartner, poor data quality is responsible for an average of $12.9 million per year in losses for businesses. This startling statistic underscores the critical importance of understanding the various dimensions of data quality for any organization aspiring to effectively leverage this invaluable asset.
Data quality dimensions are foundational metrics that evaluate how trustworthy, reliable, and actionable your data is. For professionals, these dimensions aren’t just concepts; they’re the lifeblood of insightful, effective decision-making.
Modern data problems require modern solutions - Try Atlan, the data catalog of choice for forward-looking data teams! 👉 Book your demo today
To assess the integrity and utility of data, we turn to a set of foundational metrics known as “data quality dimensions.” These dimensions serve as the yardstick by which we measure the reliability, trustworthiness, and actionability of our data.
Whether you’re a data analyst, a business executive, or an information enthusiast, understanding these dimensions is essential for unlocking the full potential of your data resources.
In this article, we will explore these dimensions and how keeping them in check will help optimize your data architecture.
Let’s dive in!
Table of contents #
- What are data quality dimensions and what are their types?
- Data quality dimensions framework
- Why are data quality dimensions essential for a modern data governance architecture?
- 10 Challenges in maintaining data quality dimensions
- Summary
- Data quality dimensions: Related reads
What are data quality dimensions and what are their types? #
Data quality refers to the condition of a set of values of qualitative or quantitative variables. It captures how well the data represents the real-world constructs, entities, or conditions it is designed to measure.
Data quality dimensions provide a framework for assessing the quality of a dataset. Various models and frameworks identify different dimensions, but some commonly cited dimensions include:
- Accuracy
- Completeness
- Consistency
- Timeliness
- Relevancy
- Validity
- Uniqueness
Let us understand these dimensions in detail:
1. Accuracy #
Accuracy is about ensuring that the data truly reflects reality. Professionals understand that even minor inaccuracies can have major repercussions. Imagine the implications of a financial error in a large transaction or a minor misrepresentation in a clinical trial dataset. Accuracy ensures that decisions made based on the data will be sound.
2. Completeness #
In a professional context, missing data can be costly. Consider a scenario in predictive maintenance where missing data can lead to machine failures. Completeness ensures that every required piece of data is available, giving a full picture and enabling confident decisions.
3. Consistency #
For professionals managing large datasets from various sources, consistency is key. It avoids situations where the same entity is represented differently across systems. For instance, a CRM system might show a client’s address differently from an invoicing system, leading to confusion and potential missed opportunities.
4. Timeliness #
Especially in industries like finance or healthcare, data needs to be up-to-date. Outdated stock prices can lead to financial losses, while old patient records might not reflect recent diagnoses or treatments. Timely data means that professionals can make decisions that are relevant right now.
5. Relevancy #
Data can be accurate, complete, and timely, but if it’s not relevant, it’s just noise. For a sales professional, data about unrelated market sectors can distract from key insights. Relevancy ensures that every piece of data serves a purpose and adds value.
6. Validity #
This is about ensuring that data fits the intended format and constraints. It might sound basic, but consider the complexities of date formats between countries or the varied ways products might be categorized in an e-commerce database. Valid data is standardized and structured, ensuring it can be effectively used in analyses.
7. Uniqueness #
For professionals dealing with vast databases, duplicate records can be a nightmare. They skew analysis, waste resources, and can lead to conflicting insights. Ensuring data uniqueness means that each piece of information stands on its own merit.
Understanding and ensuring data quality dimensions are met is fundamental for any organization aiming for successful data-driven operations. Proper data quality ensures reliable, actionable insights, building a strong foundation for effective decision-making.
Data quality dimensions framework: The 6Cs of data quality #
The 6Cs of data quality is a framework that some professionals use to define and assess the quality of data. Here’s a breakdown of the 7Cs
- Correctness
- Completeness
- Consistency
- Currency
- Conformity
- Cardinality
Let us understand them in detail:
1. Correctness #
At its core, correctness emphasizes the importance of data reflecting reality without errors. In practical scenarios, data incorrectness can lead to a multitude of issues: think about a medical record misstating a patient’s allergies or a finance system logging incorrect transaction amounts.
The cascading impact of such inaccuracies could be significant, from health hazards to financial discrepancies. Ensuring data correctness is the foundation upon which all other quality dimensions rest.
2. Completeness #
This isn’t merely about having all records filled but ensuring that the data provides a comprehensive picture. For instance, if a retailer is tracking sales transactions but fails to record all sales channels, they might miss significant insights about customer behaviour.
Completeness doesn’t just fill gaps; it ensures that the entire narrative the data is supposed to tell is intact.
3. Consistency #
In a complex organization, data flows through multiple systems, platforms, and teams. Consistency is about ensuring that this data remains harmonized across touchpoints. Imagine the confusion if a customer’s profile shows different purchasing histories in the sales and customer service databases.
Beyond just avoiding contradictions, consistency ensures that data remains a single source of truth no matter where it’s accessed from.
4. Currency #
The value of some data depreciates over time. Stock prices from a month ago or yesterday’s weather data won’t be much help in making decisions today. Currency is about ensuring data is not just available but relevant to the current context.
This is crucial in industries like finance, marketing, and healthcare where staleness of data can lead to missed opportunities or even risks.
5. Conformity #
Every field in a database is usually designed with specific standards in mind. Whether it’s the format of dates, the structure of email addresses, or constraints on numerical values. Conformity ensures that the data aligns with these pre-set criteria.
For instance, an “email” field shouldn’t accept values that don’t resemble an email format. Conformity, thus, acts as a gatekeeper, ensuring data entered aligns with expected standards.
6. Cardinality #
In data systems, especially large databases, there’s a risk of duplicate entries. These duplicates can skew analytical results, inflate figures, or cause redundancy in communications (like sending two copies of the same promotional email to a customer).
Cardinality emphasizes that each record should be unique, ensuring clean, lean, and efficient databases.
The 6Cs framework provides a comprehensive approach to data quality, ensuring that the data in an organization’s possession is both reliable and fit for its intended use.
Why are data quality dimensions essential for a modern data governance architecture? #
For companies committed to becoming data-driven organizations, weaving data quality dimensions into the fabric of their data governance architecture isn’t just an option; it’s a necessity. These dimensions offer practical ways to translate data governance policies into tangible outcomes.
Some of the benefits of data quality dimensions include
- Improved decision-making
- Operational efficiency
- Regulatory compliance
- Enhanced customer experience
- Competitive advantage
Let’s understand them in detail:
1. Improved decision-making: #
Accurate, timely, and relevant data form the backbone of sound decision-making. For example, a retail company that ensures the ‘accuracy’ and ‘timeliness’ of its sales data can better predict inventory needs, thereby optimizing stock levels and reducing carrying costs.
2. Operational efficiency #
The ‘consistency’ and ‘completeness’ dimensions are crucial here. A manufacturing company might integrate data from suppliers, internal processes, and distributors into a single system. If this data is consistent and complete, the company can optimize its supply chain from end to end, reducing lead times and costs.
3. Regulatory compliance #
For businesses in highly regulated industries like healthcare or finance, data validity and ‘uniqueness’ are crucial for compliance. Failing to meet data quality standards can result in hefty fines. For instance, healthcare organizations must ensure that patient records are accurate and unique to comply with regulations like HIPAA.
4. Enhanced customer experience #
Data ‘relevance’ and ‘accuracy’ dimensions enable companies to offer more personalized experiences. A media streaming service, for example, can curate better content suggestions if it accurately understands user preferences and behaviour.
5. Competitive advantage #
Companies that have data governance architectures emphasizing all quality dimensions can derive insights faster and more reliably than competitors. The ‘timeliness’ and ‘relevancy’ dimensions can help a financial trading firm make quicker and more informed trades, thereby outperforming competitors.
The benefits of incorporating data quality dimensions into data governance are multifaceted and far-reaching. From internal operations to customer-facing activities, and from compliance to gaining a competitive edge, these dimensions help companies navigate the complex landscape of today’s data-driven world. Data quality isn’t just a technical issue; it’s a business imperative.
10 Challenges in maintaining data quality dimensions #
Maintaining high data quality dimensions is essential for any organization that relies on data for decision-making and operational efficiency. However, ensuring that data quality dimensions are upheld can be a complex and ongoing challenge. Here are some of the key obstacles faced:
- Volume of data
- Data source diversity
- Data decay
- Human error
- Complex data relationships
- Evolving data compliance and regulation
- Integration of new technologies
- Lack of standardization
- Resource constraints
- Prioritization of data quality
Let’s explore the challenges in maintaining the data quality dimensions in detail.
1. Volume of data #
As the amount of data collected by organizations grows exponentially, maintaining quality becomes increasingly difficult. The sheer volume can overwhelm traditional data management systems, leading to potential issues with accuracy and consistency.
2. Data source diversity #
With data coming from various sources, including internal systems, social media, IoT devices, and more, ensuring that all data adheres to the same quality standards is a significant challenge. The diverse nature of data sources often leads to inconsistencies in data types and formats.
3. Data decay #
Over time, data can become outdated or irrelevant—what is known as data decay. Ensuring that data remains accurate and timely requires ongoing review and updates, which can be resource-intensive.
4. Human error #
Manual data entry and handling are prone to errors. Incorrect data can propagate through an organization’s systems, affecting all data quality dimensions, particularly accuracy and reliability.
5. Complex data relationships #
In many cases, data is not standalone but has complex relationships and dependencies. Maintaining the integrity of these data relationships during updates and migrations is a challenge.
6. Evolving data compliance and regulation #
With regulations such as GDPR and CCPA, there are stringent requirements for data privacy and handling. Keeping up with these evolving regulations and ensuring compliance adds another layer of complexity to maintaining data quality dimensions.
7. Integration of new technologies #
As new technologies are adopted, integrating them into existing data ecosystems without compromising data quality requires careful planning and execution.
8. Lack of standardization #
Without company-wide standardization, each department may have different practices for data handling, leading to challenges in maintaining uniform data quality dimensions across the organization.
9. Resource constraints #
Allocating enough resources, including tools and skilled personnel, to maintain data quality is a challenge, especially for smaller organizations or those with limited budgets.
10. Prioritization of data quality #
Often, organizations may prioritize other initiatives over data quality, not recognizing the long-term benefits of investing in high-quality data.
Addressing these challenges requires a strategic approach that includes the implementation of robust data governance policies, continuous monitoring of data quality dimensions, and the use of advanced data management tools.
By recognizing and proactively managing these challenges, organizations can significantly improve their data quality, leading to better business outcomes.
In summary #
Attaining to industry’s data quality standards has become a competitive necessity for companies relying largely on their data warehouse and data analytics for both their operations and decision-making process.
The concept of data dimension gives data managers and architects a checklist to follow; eliminating the need for complex data quality solutions or standards that may not work in the long run.
Data quality dimensions: Related reads #
- Data Quality Measures : Best Practices to Implement
- Data Quality Explained : Causes, Detection, and Fixes
- 9 Components to Build the Best Data Quality Framework
- How To Improve Data Quality In 12 Actionable Steps?
- 6 Popular Open Source Data Quality Tools To Know in 2024: Overview, Features & Resources
- Data Governance Roles and Their Responsibilities
- Data Governance Policy — Examples & Templates
- Data Dictionary — Examples, Templates, Best Practices, and How To Create a Data Dictionary
Share this article