How to Implement Data Mesh From a Data Governance Perspective?
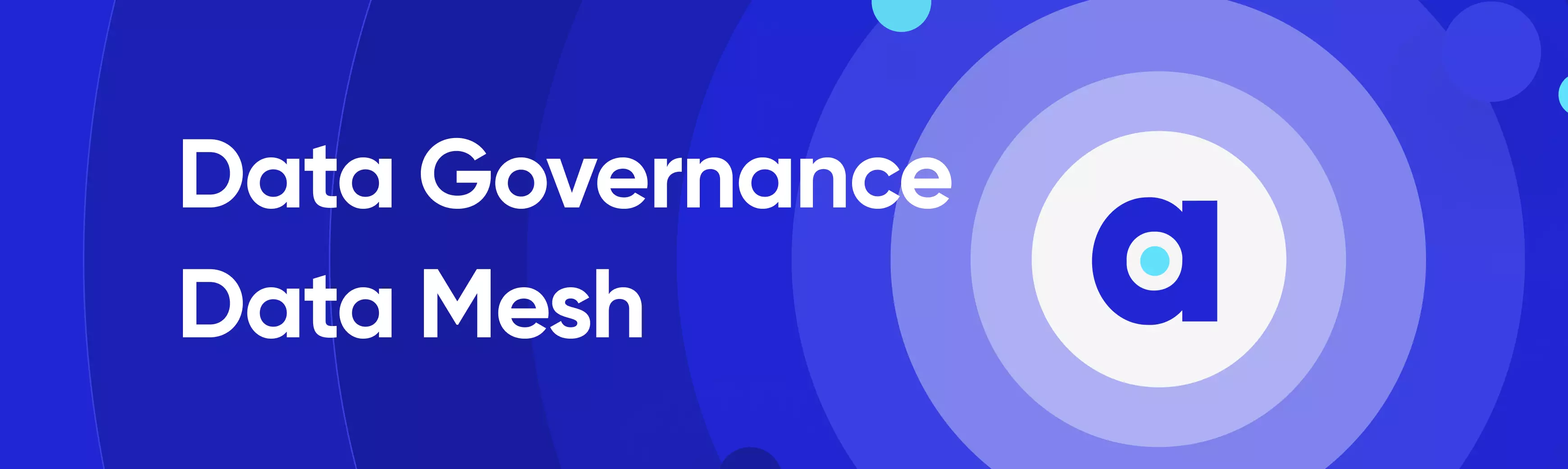
Share this article
Data mesh is a data architecture approach that emphasizes domain-oriented decentralized data ownership and management. While it brings numerous benefits, implementing data mesh can be complex, especially when it comes to data governance. Effective data governance is crucial for ensuring the quality, security, and compliance of data products generated and consumed by different teams.
The Ultimate Guide to Data Mesh - Learn all about scoping, planning, and building a data mesh 👉 Download now
This blog is a comprehensive guide to building a robust governance framework that supports data mesh architecture.
But, before we move in that direction, let us take a moment to understand the connection between data mesh and data governance.
Table of contents #
- How is data mesh connected with data governance?
- Implementing data mesh: A data governance perspective
- Implementing federated data governance: Best practices and tools
- Tools for federated data governance
- Why Atlan is perfect for implementing federated data governance and designing a data mesh for your organization
- Rounding it all up
- Data governance and data mesh: Related reads
How is data mesh connected with data governance? #
Data mesh is a decentralized approach to data architecture and organizational structure, promoting domain-oriented ownership of data products. Data governance is essential in a data mesh to ensure consistency, quality, and security across domains, enabling seamless collaboration and data-driven decision-making throughout the organization.
Now, let’s dive into the steps to implement data mesh!
Implementing data mesh: A data governance perspective #
In this section, we’ll explore the steps to implementing data mesh solely from the perspective of data governance.
- Assess the current data landscape
- Identify domain teams
- Establish data product ownership
- Implement federated data governance
- Create a data catalog
- Define data access policies and mechanisms
- Automate data quality checks and monitoring
- Develop cross-functional collaboration
- Measure and iterate
Let us look into each of the above steps in detail.
1. Assess the current data landscape #
Begin by assessing the company’s current data landscape, infrastructure, and team structure. Understand the data sources, data quality, data cataloging practices, and the existing data governance framework.
2. Identify domain teams #
Divide the organization into domain teams, which should be aligned with the business functions, such as product categories, marketing, or supply chain. Domain teams should be responsible for their data products and should have data engineers, data scientists, and analysts as part of their teams.
3. Establish data product ownership #
Each domain team should have clear ownership of the data products they generate. Establish data product managers who are responsible for the quality, security, and accessibility of their data products, as well as for ensuring that these products meet the needs of the consumers.
4. Implement federated data governance #
Empower domain teams to manage their data products while ensuring that overarching policies and guidelines are adhered to. Implement a federated governance model that maintains the right balance between central governance and domain autonomy. This includes defining standard data security and privacy policies, data cataloging and lineage practices, data quality metrics, and access controls.
We will look into the steps to implement federated data governance in the next section of this blog.
5. Create a data catalog #
Develop a centralized data catalog that indexes all the data products available across the organization. This catalog should contain metadata, including data lineage, data quality scores, and ownership information. It should also provide a user-friendly interface for teams to discover, understand, and access relevant data products.
6. Define data access policies and mechanisms #
Develop data access policies and mechanisms that provide secure, role-based access to data products. This includes defining data-sharing agreements between domain teams, implementing data anonymization techniques, and setting up data access control mechanisms such as API gateways.
7. Automate data quality checks and monitoring #
Implement automated data quality checks, validation, and monitoring processes to ensure the quality of the data products. This includes setting up quality metrics, defining thresholds, and creating alerts for potential data quality issues.
8. Develop cross-functional collaboration #
Encourage collaboration between domain teams and central data governance bodies to continuously improve data governance practices. Set up regular reviews, workshops, and training sessions to share best practices, lessons learned, and new insights.
9. Measure and iterate #
Establish key performance indicators (KPIs) to measure the effectiveness of the data governance framework. Monitor these KPIs and continuously iterate on the governance model to adapt to changing business needs, technology advancements, and regulatory requirements.
By implementing these steps, you will be able to build a robust data governance framework that supports a data mesh architecture, empowering teams with relevant data and insights, and driving data-driven decision-making across the organization.
Now, we will learn why it is important to know about federated data governance in the context of data mesh.
Implementing federated data governance: Best practices and tools #
Federated data governance is a model where the responsibility and authority for managing data are distributed across different domains or teams within an organization, while still adhering to overarching policies and guidelines set by a central governance body. This model balances autonomy and control, empowering domain teams to manage their data products while ensuring consistency and compliance.
Here’s a comprehensive guide to implementing federated data governance:
- Central governance body
- Domain teams
- Data governance framework
- Data product ownership
- Standardization and collaboration
- Data access and sharing
- Data catalog
- Automated data quality and monitoring
- Continuous improvement
Let us look into each of these steps in more detail:
1. Central governance body #
Establish a central data governance body responsible for defining and enforcing organization-wide policies, standards, and guidelines. This body should include representatives from different domains, such as data management, IT, security, privacy, and legal teams.
2. Domain Teams #
Identify and create domain teams that align with business functions (e.g., marketing, supply chain, product categories). Each team should include data product managers, data engineers, data scientists, and analysts responsible for managing their data products.
3. Data governance framework #
Develop a data governance framework that outlines the roles, responsibilities, and policies for data management across the organization. This framework should encompass data quality, privacy, security, cataloging, lineage, access control, and data lifecycle management.
4. Data product ownership #
Assign clear data product ownership to domain teams. Data product managers should be responsible for ensuring that data products adhere to established governance policies, and for liaising with the central governance body to address any issues or concerns.
5. Standardization and collaboration #
Establish standard data models, metadata management, and data lineage practices to enable seamless collaboration between domain teams. Encourage knowledge sharing and collaboration by organizing workshops, training sessions, and regular communication between domain teams and the central governance body.
6. Data access and sharing #
Define data access policies and mechanisms, including role-based access controls, data-sharing agreements, and data anonymization techniques. Implement API gateways or similar technologies to facilitate secure and controlled access to data products.
7. Data catalog #
Create a centralized data catalog that indexes all data products, including metadata, lineage, ownership, and data quality scores. This catalog should provide a user-friendly interface for discovering and understanding data products across the organization.
8. Automated data quality and monitoring #
Implement automated data quality checks, validation, and monitoring processes to ensure compliance with governance policies. Establish data quality metrics, thresholds, and alerting mechanisms to proactively identify and address data quality issues.
9. Continuous improvement #
Regularly review and refine the data governance framework to adapt to changing business needs, technology advancements, and regulatory requirements. Measure the effectiveness of the governance model using key performance indicators (KPIs) and iterate as needed.
Tools for federated data governance #
- Data catalog
- Data quality and validation
- Data access and security
- Data lineage and metadata management
Implementing federated data governance in a data mesh architecture requires a combination of organizational structure, governance policies, collaboration, and tooling. By adopting this model, organizations can ensure data consistency, quality, and security while empowering domain teams to manage their data products effectively.
Why Atlan is perfect for implementing federated data governance and designing a data mesh for your organization #
Atlan is an active metadata platform that can be an excellent choice for implementing federated data governance and designing a data mesh for your organization. Some of its features that can support these goals include:
- Data catalog
- Metadata management
- Data quality and validation
- Data access and security
- Collaboration and knowledge sharing
- Integration
- Scalability
Let us look into each of these features in more detail:
1. Data catalog #
Atlan allows you to create a centralized data catalog that indexes all data products across your organization. It enables users to discover, understand, and access data products with ease, facilitating collaboration between domain teams.
2. Metadata management #
Atlan manages metadata automatically, helping you maintain a comprehensive understanding of your data assets. It captures metadata from various data sources, tools, and pipelines, allowing users to trace data lineage and understand the relationships between different data assets.
3. Data quality and validation #
Atlan integrates with data quality tools and provides a platform to define and track data quality rules and metrics. This helps you ensure that your data products adhere to your organization’s data governance policies.
4. Data access and security #
Atlan allows you to set up granular, role-based access controls to manage who can access different data products. You can also implement data anonymization and masking techniques to protect sensitive information.
5. Collaboration and knowledge sharing #
Atlan fosters collaboration among domain teams by providing features such as data asset annotations, data dictionaries, and collaborative workflows. This encourages knowledge sharing and helps maintain a common understanding of your organization’s data assets.
6. Integration #
Atlan supports integration with a wide range of data sources, data processing tools, and data visualization platforms, making it easier to implement a data mesh architecture that spans across your organization’s data ecosystem.
7. Scalability #
Atlan is designed to scale with your organization as it grows, ensuring that it remains a suitable choice for managing your data governance needs even as the complexity and volume of your data products increase.
By using Atlan as an active metadata platform, you can effectively implement federated data governance and design a data mesh that meets your organization’s data management and collaboration requirements. This will enable your organization to harness the full potential of its data assets and drive data-driven decision-making across all business functions.
Rounding it all up #
In this blog, we discussed the importance of data mesh and data governance for a rapidly growing e-commerce company. Data mesh is a decentralized approach to data architecture and organizational structure, which promotes domain-oriented ownership of data products. Data governance is essential for ensuring consistency, quality, and security of data across domains in a data mesh.
We also explored the concept of federated data governance, a model that balances domain autonomy and central control. This model distributes responsibility for managing data across domain teams while adhering to overarching policies and guidelines set by a central governance body.
In summary, adopting a data mesh architecture with a focus on federated data governance can help your organization effectively manage its data assets, ensure data quality and security, and empower teams with relevant data and insights for data-driven decision-making.
If you are evaluating and looking to deploy best-in-class data access governance for the modern data stack without compromising on data democratization, do give Atlan a spin.
Atlan is a third-generation data catalog built on the premise of embedded collaboration that is key in today’s modern workplace, borrowing principles from GitHub, Figma, Slack, Notion, Superhuman, and other modern tools that are commonplace today.
Data governance and data mesh: Related reads #
- What is Data Governance?;Its Importance, Principles & How to Get Started?
- Key Objectives of Data Governance: How Should You Think About Them?
- Data Governance Framework — Examples, Templates, Standards, Best Practices & How to Create One?
- What is Data Mesh? - Examples, Case Studies, and Use Cases
- Snowflake Data Mesh: Step-by-Step Setup Guide
- Snowflake Data Governance — Features, Frameworks & Best Practices
- Enterprise Data Governance — Basics, Strategy, Key Challenges, Benefits & Best Practices
- Data Governance and Compliance : Act of Checks & Balances
- How to implement data governance? Steps, Prerequisites, Essential Factors & Business Case
- How to Improve Data Governance? Steps, Tips & Template
- 7 Steps to Simplify Data Governance for Your Entire Organization
- Automated Data Governance: How Does It Help You Manage Access, Security & More at Scale?
Share this article