Data Governance vs. Data Management: What's the Difference?
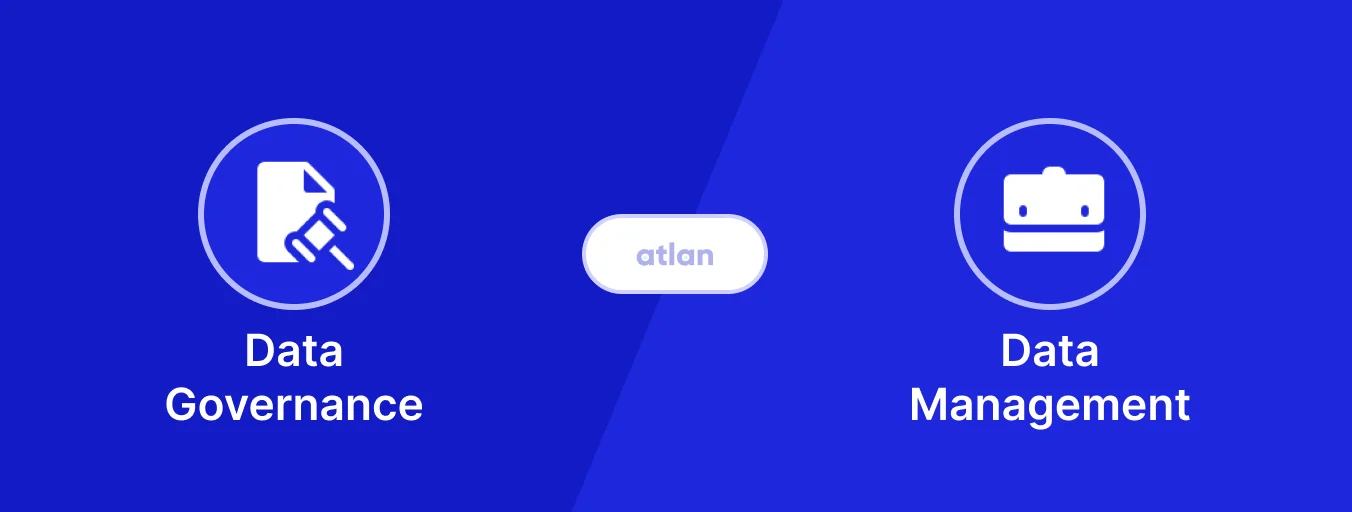
Share this article
Difference between data governance and data management #
The main difference between data governance and data management is data governance is a set of procedures and guidelines on data access, use, and management. Meanwhile, data management includes processes and tools to ingest, store, catalog, prep, explore, and transform data, so that you can use it for decision-making.
Here’s a quick comparison table between data governance and data management to help you out.
Data governance | Data management | |
---|---|---|
What is it? | A practice to increase the business value of data without compromising its security, integrity, or privacy | Processes and tools to support data consumption by following the data governance guidelines |
What does it do? | Defines policies and controls for storing, manipulating, and consuming data | Defines tools, procedures, and methods to manage the lifecycle of each data asset |
What questions does it answer? | 1. Which data sources should you use for your business? 2. How will you classify the data originating from various sources? 3. How will you store sensitive data? 4. Who owns a data asset? 5. Who can access that asset? Who can make changes to it? 6. What are your data quality metrics? | 1. What tools will you use to store data? 2. How will you make data from various domains or applications interoperable? 3. How will you facilitate data sharing and collaboration? 4. How will you ensure the quality of data and keep it relevant, accurate, and updated? |
Who owns it? | The accountability lies with everyone, but primarily the business heads. For example, in a decentralized model, the data domains are responsible for governing the data they create. | The accountability lies with the engineers and other technical members of your data team. |
Here’s an analogy that’s often cited to explain the difference between data governance vs. data management. Data governance can be seen as the blueprint for constructing a new building, whereas data management is the act of construction.
Read more → Data management 101
Table of contents #
- Difference between data governance and data management
- Data governance vs. data management: In action
- Data governance vs. data management: 3 core differences
- Difference #1: The processes
- Difference #2: The people
- Difference #3: The technology
- How do data governance and data management work together?
- #1: Regulatory compliance
- #2: Role-based access
- #3: Data cataloging
- Data governance and data management: Are they really that different?
- Data governance vs. Data management: Related reads
Data governance vs. data management is a frequent comparison as they are two similar practices that help you leverage data to its fullest potential.
Here let’s understand where the comparison stems from, understand the fundamentals of data governance and data management, and then explore how they’re related.
The author of the book titled “Non-Invasive Data Governance” Robert S. Seiner compares data governance vs. data management as follows:
Data governance focuses on what I refer to as the Bill of “Rights”. It’s all about getting the “right” people with the “right” knowledge working with the “right” data in the “right” way at the “right” time resulting in the “right” decision. Meanwhile, data management is the delivery of practices and processes targeted at successful business outcomes.
The overlap between the disciplines of data governance, data management, and information security Source: TDAN
Meanwhile, the International Organization for Standardization (ISO) highlights the difference between data governance vs. data management as follows:
Data governance specifies which decisions are to be made in data management and who makes such decisions. However, data management ensures these decisions are made and actions are taken place appropriately.
Data governance vs. data management: In action #
For instance, when you use AWS S3 to store your data or Fivetran to set up data pipelines, that’s an example of data management in action.
However, when you set up a business glossary with definitions of the data assets in AWS S3 or a platform to define S3’s access rules, you’re implementing data governance.
Data governance vs. data management: In action - A Demo of Atlan
Does data management include data governance? #
Yes. Data governance is all about how you manage your data. So, data management includes data governance as you must document the guidelines on dealing with data and how they affect your business goals.
Data governance vs. data management: 3 core differences #
We can compare data governance vs. data management in terms of:
- Processes
- People
- Technology
Difference #1: The processes #
Data governance dictates “how organizations decide about using data”. So, the processes involved can be:
- Setting up data quality checks
- Defining data access policies
- Complying with global, local, and organizational regulations
- Setting up and maintaining a business glossary
Meanwhile, data management is all about “how organizations use data”. These processes follow the guidelines outlined in the data governance framework.
The processes involved can be:
- Data transformations to maintain data in consistent formats
- Data storage in warehouses, lakes, and more
- Data exploration to solve operational and analytics use cases
Difference #2: The people #
Data governance was traditionally seen as a function that concerned business and IT teams. So, implementing data governance would involve business managers, domain data owners, and other such business stakeholders.
Meanwhile, data management would be all about execution — implementing the governance framework and influencing the organization’s business goals. This involved everything from defining rules for data storage to setting up access rights and controls.
So, it required purely technical roles, such as a data engineer, architect, or database administrator (DBA).
Difference #3: The technology #
So, data governance tools are used to document these rules and incorporate them for data assets across the organization. This includes data dictionaries and glossaries, data catalogs, etc.
Meanwhile, data management tools are more concerned with data storage, processing, and exploration.
How do data governance and data management work together? #
Data management without governance is like constructing something without a blueprint. Meanwhile, governance without management is just documentation. It’s crucial for data governance and data management to work in tandem so that you can extract value from your data.
Here are some examples of how that would look:
- Regulatory compliance
- Role-based access
- Data cataloging
Example #1: Regulatory compliance #
GDPR guidelines help you define your data governance policy. For instance, the GDPR expects you to maintain records for at least seven years from the end of your tax year/accounting period. So, your data governance policy must ensure that you know which data should be retained for seven years, the format in which it must be stored, and the people who can access it.
In this scenario, data management would involve extracting the necessary data and performing the required transformations to organize, classify, and store it in the right format. Data management would also involve ensuring that this data is easy to discover and access for the right people.
Example #2: Role-based access #
Another example is that of role-based access. The data governance program should identify user roles and their data access rights.
Data management would involve the execution and monitoring of role-based access within your organization.
Example #3: Data cataloging #
Data governance requires you to ensure that all of your data assets are well-defined and offer the necessary context, such as asset origins, ownership, transformations, etc.
Data governance would involve setting up a data cataloging platform that leverages metadata from all data sources to surface asset descriptions, 360-degree data profiles, lineage mapping, and more. Meanwhile, data management would focus on organizing the data from various sources into data warehouses and lakes and connecting these sources with the cataloging tool.
Data governance and data management: Are they really that different? #
Traditionally, identifying data governance and data management as two separate concepts made sense because governance was merely all about compliance. So, the tooling focused on access control and security.
Meanwhile, data management was all about executing the various processes involved in collecting, storing, and using data.
Today, those lines have blurred as data governance is crucial to helping data teams work better. It’s not a “data governance vs. data management” situation anymore.
Let’s look at how various organizations define it:
”Data governance is about managing data as a strategic asset. Data governance practices are essential in helping ensure that data is optimized for any use—enabling deeper insights across our organizational and functional boundaries.”
“Data governance reflects an organization’s strategic direction and desired outcomes in areas related to data management, including quality and metadata management, information security, architecture, and data modeling.”
“The foundation of data governance is — balancing central oversight, proper prioritization, and consistency while ensuring that the employees creating and using data are the ones leading its management.”
“Data governance drives an understanding of the challenges data teams face. Ultimately, data governance leads to a data-driven organization that will continue to compete in the modern marketplace.”
“Data governance is an organization’s management of its data availability, usability, consistency, and data integrity and data security.”
That’s why data governance and data management can be seen as different ways of looking at the same problems — two sides of the same coin. Better governance will lead to better management of your data assets, and effective data management is only possible when you achieve true governance.
Ready to make data governance effortless? #
Try Atlan — Say goodbye to the complex, bureaucratic version of data governance. Say hello to data enablement — a simpler, community-centered approach, with privacy at its core.
Data governance vs. Data management: Related reads #
- Data Governance in Action: Community-Centered and Personalized
- Data Governance and Its Importance in the Modern Data Stack
- Data Governance Framework — Examples, Templates, Standards, Best practices & How to Create One?
- Snowflake Data Governance — Features, Frameworks & Best practices
- Open Source Data Governance Tools - 7 Best to Consider in 2023
- Data Governance Policy: Examples, Templates & How to Write One
- 7 Best Practices for Data Governance to Follow in 2023
- Benefits of Data Governance: 4 Ways It Helps Build Great Data Teams
- Data Governance Roles and Responsibilities: A Quick Round-Up
- Key Objectives of Data Governance: How Should You Think About Them?
- The 3 Principles of Data Governance: Pillars of a Modern Data Culture
- A Guide to Gartner Data Governance Research — Market Guides, Hype Cycles, and Peer Reviews
- 5 Popular Data Governance Certifications & Trainings in 2023
- 8 Best Data Governance Books Every Data Practitioner Should Read in 2023
- Automated Data Governance: How Does It Help You Manage Access, Security & More at Scale?
- Data Governance and Compliance: Act of Checks & Balances
- Data Governance vs. Data Management: What’s the Difference?
- Enterprise Data Governance — Basics, Strategy, Key Challenges, Benefits & Best Practices
Share this article