Data Mesh vs Data Mart - How & Why Are They Different?
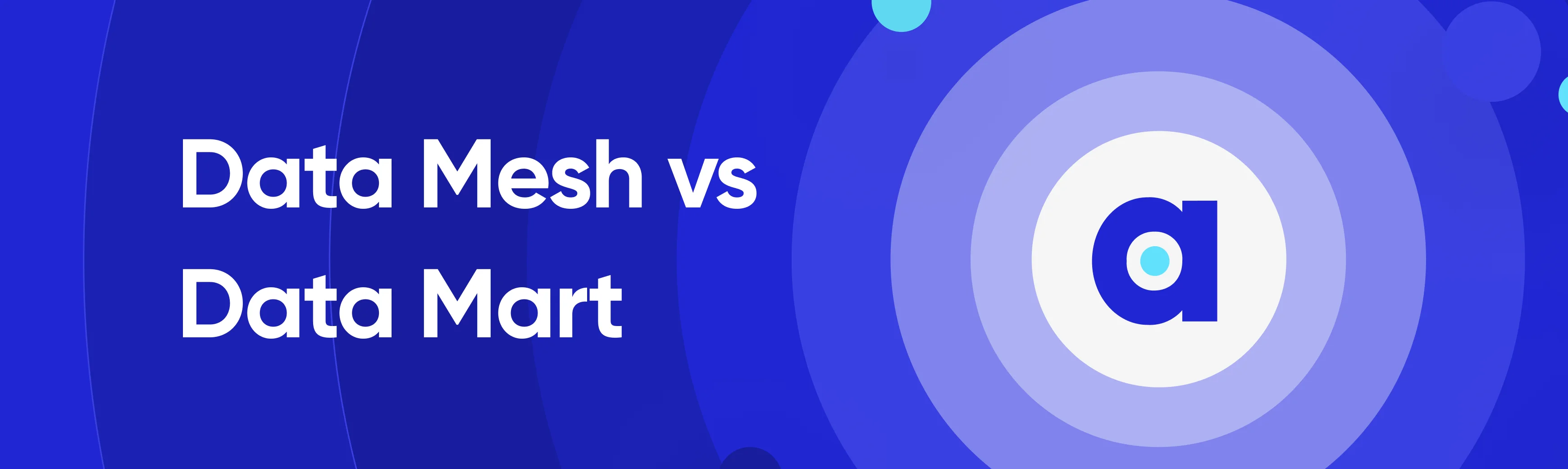
Share this article
A data mesh is essentially an approach where instead of a centralized data team owning the end-to-end data pipeline, every domain team owns its own data, end-to-end. On the other hand, a data mart is essentially a repository of data gathered from a variety of sources, intended to assist a particular community of knowledge workers.
The Ultimate Guide to Data Mesh - Learn all about scoping, planning, and building a data mesh 👉 Download now
In this blog, we will see how both data mesh and data mart are two distinct concepts within the data architecture landscape, each with its specific applications, use cases, advantages, and drawbacks.
Table of contents
- How is data mesh different from data mart?
- How to choose the right tool for your data needs?
- Applications and use cases of data mesh and data mart with real-world examples
- Harnessing synergy: The power of data mesh and data mart integration
- Factors to consider for data mesh and data mart approaches
- Comparing data mesh and data mart: A comprehensive analysis
- Rounding it up all together
- Data mesh vs data mart: Related reads
How is data mesh different from data mart?
The concept of a data mesh is a relatively new approach to dealing with large-scale data management challenges. On the other hand, a data mart is a concept that’s been around for much longer. It’s a subset of a data warehouse oriented towards a specific business line or team.
Let us first begin with data mesh.
What is a data mesh?
A data mesh is especially useful in scenarios where businesses span multiple distinct operational domains. It is a decentralized, domain-oriented, and self-serve design that allows organizations to handle and leverage massive amounts of data more effectively.
An e-commerce example could be inventory management, customer support, billing, sales, marketing, etc.
The data mesh approach will allow each of these teams to operate independently, taking full responsibility for their own data from collection to storage, processing, and providing insights.
Key principles of data mesh
- Domain-oriented decentralized data ownership and architecture
- Data as a product
- Self-serve data infrastructure as a platform
- Federated computational governance
What is a data mart?
On the other hand, the data mart concept fits in a traditional centralized data architecture where a data warehouse team serves the needs of different business units.
Data marts are typically easier to implement than a complete data warehouse because they are smaller and more focused.
Data marts may be the end goal if the organization’s needs are projected to remain relatively static, and the emphasis is on quick retrieval of data.
Key principles of data mart
- Data focus: They are focused on providing relevant data for a specific area of the organization.
- Data aggregation: Data marts involve aggregating data from various sources, such as transactional systems, and operational databases.
- Simplified data structure: Data marts typically have a simplified data structure tailored to specific requirements.
- Business context: Data marts are designed to provide data in a format that aligns with the specific business context.
- Granularity and detail: Data marts can be created at different levels of granularity.
- Security and access control: Data marts enforce security measures to ensure that access to data is limited to authorized individuals or groups.
How to choose the right tool for your data needs?
If the organization’s needs are expected to change over time, a data warehouse is usually a better solution, because it is easier to adjust to changing business needs.
To fully understand the applications and implications of these two architectures, you would want to consider factors like :
- The size of your organization
- The scale and diversity of the data you’re dealing with
- The specific needs
- Capacities of the teams that will be using the data
- The expected future growth and evolution of the company’s data needs
For a large, rapidly growing company with diverse operational domains like the e-commerce company described here, a data mesh approach could be beneficial. It allows for a more scalable, resilient, and domain-specific approach to data management.
On the other hand, for more targeted needs or for smaller organizations, data marts might be more suitable and less complex to implement and manage. The decision should be based on a thorough analysis of the business requirements, the available resources, and the strategic direction of the company.
Applications and use cases of data mesh and data mart with real-world examples
In today’s data-driven world, organizations are constantly seeking innovative approaches to leverage their data assets effectively. Two popular strategies that have gained significant attention are data mesh and data mart.
While both serve as essential components of a comprehensive data architecture, they offer distinct approaches to managing and utilizing data.
In this section, we will delve into the applications and use cases of data mesh and data mart. We will be exploring how they can empower organizations to unlock valuable insights and drive informed decision-making.
Let us look into the application of each of the systems one by one:
How is data mesh applied in the real world?
The data mesh architecture is particularly applicable to large, complex organizations where data sources are many and varied.
Let us look into each of these examples one by one:
Multinational corporation
A great example could be a multinational corporation with several independent business units spread across the globe, each producing a large volume and variety of data.
- Take a global retail corporation, for instance. It might have various business units such as online sales, in-store sales, logistics & supply chain, customer service, marketing, etc., each generating its own unique data.
- In a data mesh architecture, each of these business units would act as a separate domain, owning and managing its data as a product.
- The online sales team would have ownership of online customer behavior data, sales data, website analytics, etc. They would ensure that this data is collected, stored, processed, and made available for analytics in an efficient, reliable, and secure manner.
- Similarly, the logistics & supply chain team would manage data regarding inventory, delivery tracking, supplier information, etc.
This approach allows each unit to have control over their data, ensuring it is handled in a way that best serves their specific needs, while also contributing to the overall data ecosystem of the corporation.
How is data mart used in the real world?
Data marts are ideally suited for scenarios where a specific business function needs quick, easy access to a subset of data. This is especially useful when the overall data warehouse is too large and complex, and the team requires only a fraction of that data.
Let us look into some examples one by one:
University
- An example might be a university that has a data warehouse containing data about students, faculty, courses, research, finances, facilities, etc.
- The university’s sports department might only be interested in data about:
- Students (names, age, major)
- Sports participation (which sports they play, their stats), and
- Facilities (availability, usage)
- In this scenario, a specific data mart can be created for the sports department, containing only the data relevant to them.
- This allows the department quick and easy access to the data they need without having to navigate the entire data warehouse.
- It also simplifies queries and reports, as they will be based on a smaller, more manageable data set.
While the use cases of the data mesh and data mart are distinct, it’s crucial to note that they are not mutually exclusive. They can be used in conjunction in a hybrid model, depending on the specific needs of the organization.
Harnessing synergy: The power of data mesh and data mart integration
Now that we’ve understood the basics and use cases of data mesh and data marts, let us understand what happens when they’re integrated. A hybrid model that incorporates both data mesh and data mart architectures can be effective in a large, complex organization.
Here’s how they could work in conjunction:
- Domain-oriented data ownership
- Data marts for specialized use-cases
- Scalability and adaptability
- Federated governance
Let’s learn about each of them one by one:
1. Domain-oriented data ownership
As per the principles of the data mesh, each business unit in the organization owns and manages its own data as a product. This allows for more accurate, efficient, and reliable data management within each domain.
2. Data marts for specialized use-cases
Within these domain-owned data sets, there could be subsets of data that are particularly relevant for specific teams or functions. For these, data marts could be created to allow for quicker, more targeted access to this data.
- For instance, consider a large e-commerce company that has various departments, each generating and managing its own data.
- The marketing department, which owns and manages its own data, might need a specialized view of certain data for its email marketing team.
- A data mart could be set up within the marketing data that contains only data relevant to email campaigns, such as customer email addresses, purchase history, and engagement with previous email campaigns.
- This hybrid approach combines the advantages of both architectures.
The data mesh principle of domain-oriented data ownership ensures that each department has the most complete, accurate, and up-to-date data available for their needs. At the same time, the use of data marts allows for more efficient, targeted access to specific subsets of that data when needed.
3. Scalability and adaptability
One of the key benefits of this hybrid approach is that it is highly scalable and adaptable. As the organization grows and evolves, new domains can be added to the data mesh, and new data marts can be created within these domains as needed.
4. Federated governance
As per the data mesh principles, governance of this data infrastructure would be federated. This means that while each domain would have ownership and control over its data, there would still be overarching principles.
Moreover, there may be standards, and procedures in place to ensure the overall coherence, compatibility, and security of the organization’s data ecosystem.
Remember, the key to a successful data strategy is understanding your organization’s specific needs and circumstances, and designing a data architecture that best serves those. The choice between data mesh, data mart, or a combination of the two should be informed by a careful analysis of the above factors.
Factors to consider for data mesh and data mart approaches
In today’s data-driven world, organizations are constantly seeking the most effective and efficient ways to manage and utilize their data assets.
In this section, we delve into the key factors that organizations should consider when evaluating and selecting between data mesh and data mart approaches. By understanding these factors, you can make informed decisions that align with your organization’s goals and maximize the value of your data.
Here are a few more considerations for both the data mesh and data mart approaches:
Key factors to consider for data mesh
- Technological capabilities
- Implementing a data mesh architecture requires substantial technological capabilities.
- Each team or domain must be equipped with the necessary tools and skills to manage their data, including collection, storage, processing, and analytics.
- Data product thinking
- A data mesh treats data as a product, with its own lifecycle and value proposition.
- This may require a cultural shift in many organizations, where data has traditionally been seen as a byproduct of operations, not a product in its own right.
- Decentralized governance
- Governance in a data mesh is federated, meaning that each domain has a degree of autonomy in managing its data.
- This requires careful balancing to ensure that while domains have the necessary independence.
- There’s still coherence across the organization regarding data quality, security, and privacy.
Key factors to consider for data mart
- Dependency on data warehouse
- Data marts are typically subsets of a larger data warehouse.
- This means that the quality and completeness of the data mart are heavily dependent on the quality and completeness of the data warehouse.
- Limited scope
- A data mart serves a particular community of knowledge workers.
- Its scope is limited, and it may not be suitable for broader, cross-functional analytics.
- Maintenance and updates
- Data marts require regular updates from the data warehouse to stay current.
- This means they are not real-time and there could be a delay in data availability.
Factors to consider for both data mesh and data mart
A hybrid approach, combining a data mesh and data marts, can potentially balance out some of these issues.
- The data mesh can provide real-time, domain-specific data management, while data marts can offer focused, efficient access to specific data subsets.
- The key is to carefully evaluate your organization’s specific needs, capabilities, and strategic direction before deciding on a data architecture.
- Lastly, remember that technology and data management practices are rapidly evolving fields.
There are always new approaches and tools being developed, so it’s important to stay informed and be open to adapt your data architecture as needed.
Comparing data mesh and data mart: A comprehensive analysis
In this section, we delve into the world of data architecture by exploring the key differences and similarities between two prominent approaches: data mesh and data mart.
Here’s a detailed comparison table that highlights the distinct applications, use cases, and characteristics of these data management methodologies.
Features | Data mesh | Data mart |
---|---|---|
Architecture | Decentralized | Centralized |
Data Ownership | Owned by individual business units/domains | Owned by a central data team |
Data scope | Entire domain-specific data | A subset of data warehouse for specific business needs |
Data governance | Federated, each domain governs its data | Centralized, governed by a central data team |
Data management | Managed by individual business units/domains | Managed by a central data team |
Implementation complexity | High, requires substantial technological capabilities across domains | Lower, focused on a specific subset of data |
Use case | Large, complex organizations with varied data across different domains | Specific business functions needing quick, easy access to a subset of data |
Real-time updates | Possible, as each domain manages its data | Depends on how frequently data is updated from the data warehouse |
Autonomy | High, each domain has independence in managing its data | Low, dependent on the central data warehouse |
Remember, these are broad generalizations and the specifics can vary greatly depending on the organization’s specific needs and circumstances.
Both a data mesh and a data mart can be valuable tools for data management and can even be used in conjunction with a hybrid model.
Rounding it up all together
Data mesh and data mart are two different approaches to managing data in an organization, each with its own specific applications and advantages.
A data mesh is a decentralized approach where each domain or business unit owns and manages its own data. Whereas a data mart is a centralized approach where a specific subset of data is managed by a central data team for a specific business function.
In essence, it’s not about choosing one over the other but choosing the right tool for the right job. Even combining these approaches in a hybrid model if that is what serves the organization best.
Data mesh vs data mart: Related reads
- What is Data Mesh? - Examples, Case Studies, and Use Cases
- Data Mesh Principles — 4 Core Pillars & Logical Architecture
- Data Mesh Architecture: Core Principles, Components, and Why You Need It?
- Data Mesh Setup and Implementation - An Ultimate Guide
- How to Implement Data Mesh from a Data Governance Perspective?
- Snowflake Data Mesh: Step-by-Step Setup Guide
- Data Mesh Vs. Data Lake — Differences & Use Cases For 2023
- Data Mart vs. Data Lake: why are they different?
- Data Mesh vs. Data Fabric: How do you choose the best approach for your business needs?
Share this article