Data Mart vs Data Lake: What's Right for Your Team in 2024?
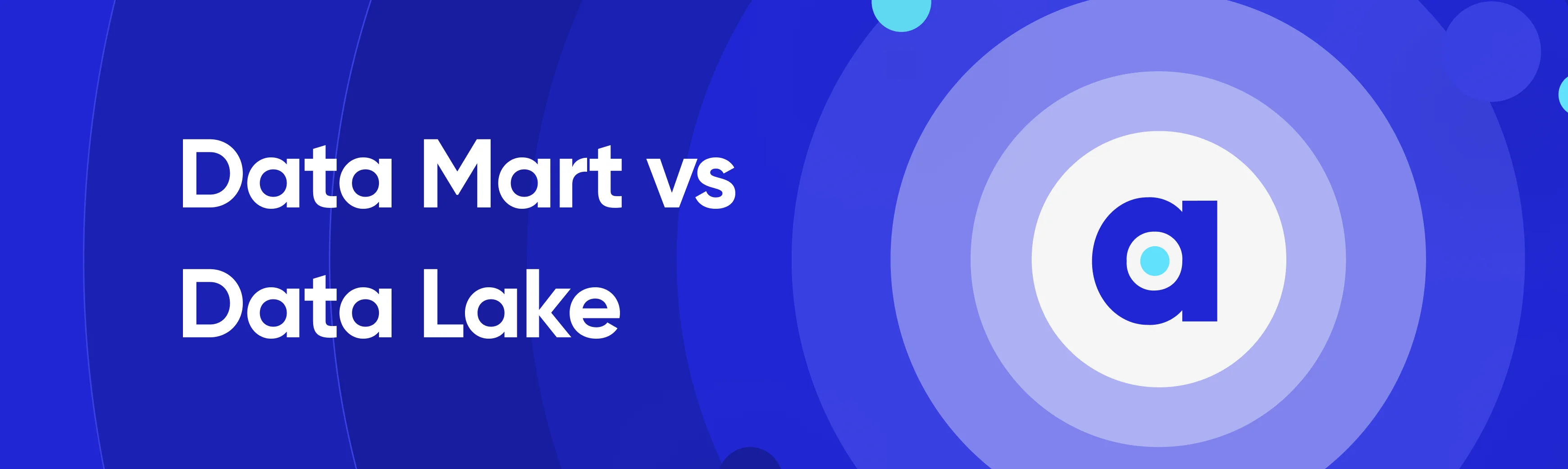
Share this article
A data mart is a subset of a data warehouse, which is designed to cater to the needs of a specific business function. On the other hand, a data lake is a central repository that stores data in its raw, unprocessed format.
Today, as businesses use data to fuel their growth and innovation, data marts vs. data lakes have become prominent storage and analytics frameworks. But, as the volume, variety, and velocity of data continue to expand, it is crucial to understand the characteristics and differences between data marts vs data lakes.
Modern data problems require modern solutions - Try Atlan, the data catalog of choice for forward-looking data teams! 👉 Book your demo today
In this comprehensive guide, we delve into the differences of data marts and data lakes, and how they shape the way modern businesses manage data.
Let’s dive in!
Table of contents
- Data mart vs data lake: 6 Key differences
- Data mart vs. data lake: How do they drive business success?
- Data mart vs. data lake vs. data warehouse: A tabular view
- Data mart vs data lake: Key considerations
- Rounding it up all together
- Related reads
Data mart vs data lake: 6 Key differences
In order to comprehend the differences between data marts and data lakes, it’s essential to grasp the fundamental concepts that underpin these two distinct approaches to data storage and analysis.
In this section, we delve into the essentials of data marts and data lakes, unraveling their core characteristics and functionalities.
What is data mart?
Data marts are optimized to provide data in a ready-for-analysis form for departments within an organization, such as finance, sales, or marketing. Furthermore, they are designed with a specific subject area or business problem in mind.
Data marts can be fed either directly from data sources, or more typically, from an existing data warehouse. They use a highly structured schema optimized for reporting and analysis, commonly dimensional modeling, such as a star schema or a snowflake schema.
What is data lake?
Data lakes are highly flexible and scalable, able to store massive volumes of data. The data can be structured, semi-structured, or unstructured, and comes from multiple disparate sources.
The data stored in a data lake is not processed until it is needed. They are schema-on-read, which means the schema is applied only when the data is read.
It helps in giving you flexibility in the kinds of data that can be stored. However, without appropriate governance, data lakes can become unmanageable data swamps. This happens because data is dumped into them without a clear structure or metadata.
What is the difference between data mart and data lake?
Data marts differ from data lakes based on different factors such as:
- Level of structures
- Purpose
- Data types
- Users
- Schema
- Scalability
Let us dive into each of the above factors in brief:
1. Level of structure
Data marts are highly structured and tailored for a specific business use case, whereas data lakes store raw data without a predefined structure.
2. Purpose
Data marts are designed for specific business needs, providing ready-to-use data for analysis and reporting. On the other hand, data lakes serve as a central repository for all data, serving multiple use cases.
3. Data types
Data marts typically store structured data that has been cleaned and prepared for analysis. Whereas data lakes can store any type of data - structured, semi-structured, or unstructured.
4. Users
Data marts are primarily used by business users who need to perform analysis on specific business areas. Data lakes are used by data scientists and engineers who need raw data for exploratory analyses, machine learning, and other data experiments.
5. Schema
Data marts use schema-on-write (data is structured upon entry into the system), while data lakes use schema-on-read (data is structured when it is accessed for reading).
6. Scalability
Data lakes are more scalable than data marts because of their ability to store vast amounts of raw data. Each storage type has its purpose and place in the data landscape of an organization, depending on the specific needs and capabilities of the business.
By unraveling the key disparities between these two data architectures, we aim to provide you with a comprehensive understanding of their strengths, and limitations.
Data mart vs. data lake: How do they drive business success?
In the realm of data-driven decision-making, the power of data marts and data lakes is exemplified by real-life success stories. Businesses across various industries have harnessed the potential of these data storage and analytics frameworks to drive remarkable business outcomes.
In this section, we explore inspiring examples of how data marts and data lakes have transformed organizations. We will also unlock valuable insights and enable informed decision-making.
Data mart example: Driving business success
Data marts enable users within a specific business function to analyze the data most relevant to them. They can provide insights and drive decision-making within a particular subject area.
- Data mart for sales teams
For instance, a sales data mart might be used by the sales department of a company to understand product performance, sales trends, customer buying behaviors, etc.
They could answer questions like:
- Which products are bestsellers?
- Which regions have the highest sales volume?
- Is there a seasonal pattern in sales?
These insights can then drive sales strategy, customer engagement, and marketing efforts.
- Data mart for finance department
Similarly, a finance data mart might help the finance department track revenue, expenses, profitability, and financial ratios. This enables them to manage budgets, forecast financial performance, and report to stakeholders.
Data lake example: Driving business success
Data lakes, storing raw and diverse data, serve as a foundational layer for different types of analytics and AI use cases.
- Telecom industry
A telecommunication company could use a data lake to store call detail records (CDRs), network data, customer data, and more.
Data scientists and engineers could access this data to develop predictive models for customer churn, analyze network performance, and personalize customer offerings.
By leveraging the raw data in the data lake, they could unearth insights not immediately obvious in a more structured data mart environment.
- Retail industry
A retail company could use a data lake to combine structured sales data with semi-structured clickstream data and unstructured social media data. This could enable comprehensive customer 360-degree views and personalized marketing, thereby enhancing customer experience and boosting sales.
In both of these examples, data lakes enable advanced analytics, machine learning, and AI capabilities that can drive significant business outcomes.
Data mart vs. data lake vs. data warehouse: A tabular view
In order to gain a clear understanding of the distinctions between data marts and data lakes, a comprehensive and easily digestible comparison is essential.
So, here’s a tabular view that offers side-by-side analysis of the key characteristics, functionalities, and use cases of data marts vs. data lakes vs. data warehouse.
Feature | Data mart | Data lake | Data warehouse |
---|---|---|---|
Data type | Structured data | Structured, semi-structured, unstructured data | Structured data |
Schema | Pre-defined schema | Schema-on-read | Pre-defined schema |
Storage | Optimized for specific business areas or departments | Raw storage of data in its native format | Optimized for historical data storage |
Data integration | Subset of data from data warehouse or other sources | Ingests data from various sources without transformation | Integrates data from multiple sources with ETL processes |
Flexibility | Limited flexibility due to pre-defined structure | High flexibility due to schema-on-read approach | Moderate flexibility with a structured schema |
Data processing | Typically used for reporting and analytics specific to a business area | Supports a wide range of data processing, including analytics, data science, and exploration | Primarily used for reporting and business intelligence |
Scalability | Scalable for specific business areas or departments. | Highly scalable to accommodate large volumes of data | Scalable for historical data storage |
Data quality | Easier to maintain data quality since it's specific to a business area | Requires robust data governance and quality controls. | Requires data cleansing and quality assurance processes |
The above comprehensive comparison summarizes the main characteristics and differences between data marts, data lakes and data warehouse and help you understand when and why to use each in your data strategy.
Data mart vs data lake: Key considerations
Data marts and data lakes are both important components of a comprehensive data strategy. Here are some additional considerations to keep in mind:
What are the key considerations for data marts?
- Design
- It’s crucial to properly design your data marts to serve the specific needs of each business function. This involves understanding the unique data requirements of different departments.
- For example how they will use the data, and which metrics are most important to them etc.
- Performance
- Because data marts typically contain less data than a full data warehouse or data lake, they can often deliver faster query performance. This can be particularly important for business users who need quick insights.
- Agility
- Data marts provide a degree of agility, as they can be built and adjusted relatively quickly in response to changing business requirements.
- Dependency
- While data marts are convenient and optimized for specific uses, they can also create data silos if not properly integrated. Further, they can also be managed within the broader data architecture.
What are the key considerations for data lakes?
- Data governance
- Implementing a strong data governance strategy is crucial for managing data lakes. Without proper data cataloging, metadata management, and access control, a data lake can quickly turn into a data swamp.
- Data quality
- Since data lakes store raw data, it’s important to have mechanisms in place to ensure and improve data quality.
- Security & privacy
- Given that data lakes often store a variety of data, including sensitive information, security and privacy concerns become paramount. It’s essential to have strong access controls and data encryption methods.
- Costs
- Data lakes can handle large volumes of data and are generally cost-effective at scale. But maintaining them and the required infrastructure, particularly if not used efficiently, can be costly.
- Skills
- Extracting value from a data lake requires a team with the right skills, including data engineers to manage the data and data scientists to perform complex analyses.
In essence, while both data marts and data lakes can provide valuable insights, they each have their own use cases, benefits, and considerations.
Understanding these will help in designing and implementing an effective data strategy.
Rounding it up all together
In summary, data marts and data lakes are integral components of a modern data architecture, each serving unique purposes and catering to specific needs.
Data marts provide targeted, ready-to-use data for business users to drive decision-making within specific domains, while data lakes offer a flexible and scalable environment for data scientists and engineers to explore raw data and develop innovative solutions.
By knowing the fundamental disparities and key considerations, you can leverage data marts and data lakes effectively for informed decision-making, innovation, and business growth.
Data mart vs data lake: Related reads
- Data Mesh vs Data Mart - How & Why Are They Different?
- Data Mart vs. Data Warehouse: Should You Use Either or Both?
- What is a data lakehouse: Definition, architecture, components, and use cases.
- What is a data lake: Definition, examples, architecture, and solutions.
- Data mesh vs data lake: What are the differences in architecture, use cases, and benefits?
- Data Warehouse vs Data Lake vs Data Lakehouse: What are the key differences?
- Top data catalog use cases intrinsic to data-led enterprises
- What is Data Fabric: Components, Use Cases & More
- What Is a Data Lake and Why Does It Need a Data Catalog?
- Data Warehouse vs Data Lake vs Data Lakehouse
- Data Mesh vs. Data Lake — Differences & Use Cases
- Data Fabric Explained Like You Know Nothing
Share this article