What is Data Modernization? Benefits, Examples & Strategy in 2024!
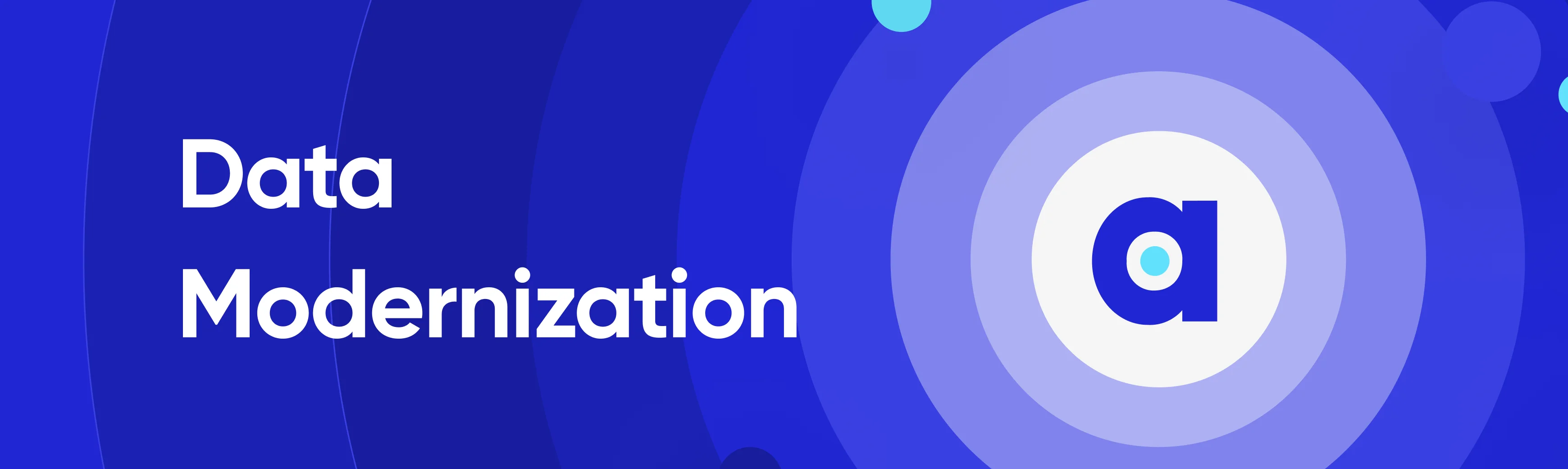
Share this article
Data modernization is the process of updating and improving an organization’s data infrastructure, tools, and practices to meet the evolving needs of data-driven business operations and analytics, ensuring data is accessible, secure, and effectively utilized.
Many organizations accumulate data in isolated, disconnected systems or departments, leading to data silos. Data modernization initiatives typically involve integrating data from various sources into a centralized repository or data lake.
By breaking down data silos and creating a unified data ecosystem, organizations can improve data accessibility and enable cross-functional teams to collaborate effectively.
Modern data problems require modern solutions - Try Atlan, the data catalog of choice for forward-looking data teams! 👉 Book your demo today
In this article, we will understand what data modernization is, reasons why it is important, how to develop a robust data modernization strategy, and more!
Let’s dive in!
Table of contents #
- What is data modernization?
- Benefits
- Components
- Examples
- Data modernization strategy
- Best practices
- Role of data governance in data modernization
- Summarizing it all together
- Related reads
What is data modernization? #
Data modernization is the process of transforming and optimizing large volumes of accumulated data into a more accessible, usable, and valuable form to drive meaningful insights and business results.
It involves adopting contemporary technologies, methodologies, and strategies to address the challenges associated with outdated data management practices and systems.
In practical terms, data modernization encompasses various activities such as
- Data integration
- Data cleansing
- Data consolidation
- Data transformation
- Data migration.
These processes enable organizations to overcome data silos, ensure data consistency and quality, enhance data accessibility and security, and improve the overall efficiency of data management.
Data modernization gained significant traction in the late 2000s and early 2010s when organizations started to recognize the potential of big data, cloud computing, and advanced analytics. With the exponential growth of data and the advent of technologies like artificial intelligence and machine learning, data modernization has become a critical aspect of staying competitive and leveraging data effectively for business outcomes.
7 Critical benefits of data modernization for organizations #
Understanding the benefits of data modernization is crucial for organizations looking to stay competitive in today’s rapidly evolving business landscape.
Here are seven critical benefits of data modernization:
- Improved decision-making
- Enhanced operational efficiency
- Increased agility and innovation
- Better customer experience
- Regulatory compliance
- Scalability and flexibility
- Competitive advantage
Now let us understand each of them in bit detail:
1. Improved decision-making #
Modernizing data allows organizations to leverage accurate, timely, and comprehensive information for making data-driven decisions. By analyzing and interpreting data effectively, teams can gain valuable insights, identify emerging trends, and make informed choices that drive business growth and success.
2. Enhanced operational efficiency #
Outdated data management systems often result in fragmented and inconsistent data across different departments and systems.
Data modernization streamlines :
- Data integration
- Data cleansing
- Data consolidation processes, ensuring data consistency and quality.
This leads to improved operational efficiency, streamlined workflows, and reduced costs associated with manual data handling and reconciliation.
3. Increased agility and innovation #
Modern data platforms enable organizations to adapt quickly to changing market dynamics and customer needs. By harnessing real-time data and leveraging advanced analytics and AI capabilities, companies can identify new opportunities, develop innovative products and services.
They can stay ahead of competitors in rapidly evolving industries.
4. Better customer experience #
By centralizing and analyzing customer data, organizations can gain a holistic view of their customers:
- Enabling personalized experiences
- Targeted marketing campaigns
- Improved customer service.
Data modernization enables the delivery of relevant, timely, and personalized offerings that meet customer expectations, resulting in higher customer satisfaction and loyalty.
5. Regulatory compliance #
In the pharmaceutical industry, compliance with regulatory standards is paramount. Data modernization ensures
- Data integrity
- Data security
- Data traceability
It facilitates compliance with regulations such as the FDA guidelines and data privacy laws. It enables organizations to demonstrate data transparency, maintain audit trails, and respond effectively to regulatory inquiries.
6. Scalability and flexibility #
As data volumes continue to grow exponentially, traditional data systems may struggle to handle the increasing data demands. Data modernization allows organizations to scale their data infrastructure to accommodate growing data volumes and diverse data sources.
It also provides the flexibility to adopt cloud-based solutions, enabling easier access, collaboration, and integration across teams and locations.
7. Competitive advantage #
Organizations that effectively modernize their data gain a significant competitive advantage. By harnessing data-driven insights, they can identify:
- Market trends
- Customer preferences
- Emerging opportunities faster than their competitors.
This enables them to make proactive business decisions, innovate, and respond swiftly to changing market dynamics.
In short, data modernization is not just a technological upgrade; it is a strategic initiative that can positively impact various aspects of an organization’s operations. By understanding and harnessing these benefits, organizations can position themselves for success in a data-driven and dynamic business environment.
What are the components of data modernization and what is their role in modern B2B enterprises? #
Data modernization for modern B2B (Business-to-Business) enterprises typically involves several key components. Here are some of the essential elements:
- Data integration
- Data cleansing and quality
- Data warehousing
- Advanced analytics and business intelligence (BI)
- Cloud computing and big data technologies
- Data governance and security
- Data visualization
Let us now understand each of the components and its role in B2B enterprises in bit of detail:
1. Data integration #
B2B enterprises often deal with data from multiple sources, including internal systems, external partners, suppliers, and customers. Data integration involves bringing together data from various sources, such as :
- CRM systems
- ERP systems
- Supply chain databases
- External APIs, to create a unified view of the business.
This enables comprehensive analysis and reporting, enabling better decision-making and improved operational efficiency.
2. Data cleansing and quality #
Data modernization involves cleansing and ensuring the quality of data. This process includes:
- Removing duplicates
- Correcting errors
- Standardizing formats
- Validating data accuracy.
Clean and high-quality data is crucial for generating reliable insights and maintaining data integrity across the organization.
3. Data warehousing #
Data warehousing involves creating a centralized repository where structured and organized data is stored for analysis and reporting purposes. Modern B2B enterprises often employ data warehousing solutions that can handle large volumes of data and support various data types.
Data warehousing enables efficient data storage, retrieval, and analysis, providing a foundation for data-driven decision-making.
4. Advanced analytics and business intelligence (BI) #
Data modernization involves leveraging advanced analytics techniques and BI tools to derive actionable insights from the accumulated data. B2B enterprises can utilize techniques like:
- Data mining
- Predictive analytics
- Machine learning to identify patterns, trends, and anomalies in their data.
This enables them to make informed business decisions, optimize operations, identify new revenue opportunities, and drive growth.
5. Cloud computing and big data technologies #
Modern B2B enterprises often adopt cloud computing and big data technologies as part of their data modernization strategy. Cloud-based solutions offer
- Scalability
- Flexibility
- Cost-efficiency in handling large volumes of data.
Big data technologies, such as Hadoop, Spark, and NoSQL databases, enable processing and analysis of diverse and unstructured data types. These includes social media data, sensor data, and customer interactions.
6. Data governance and security #
Data modernization requires implementing robust data governance practices to ensure data privacy, security, and compliance with regulations. B2B enterprises need to define
- Data ownership
- Establish data access controls,
- Monitor data usage to protect sensitive information.
Data governance also includes establishing data policies, standards, and procedures to maintain data consistency, accuracy, and compliance.
7. Data visualization #
Data visualization plays a vital role in data modernization by presenting complex data in a visual format that is easy to understand and interpret. Modern B2B enterprises use data visualization tools and dashboards to communicate insights effectively across the organization.
Interactive visualizations enable stakeholders to explore data, identify trends, and make data-driven decisions quickly.
By incorporating these components into their data modernization strategy, B2B enterprises can unlock the full potential of their data, drive operational excellence, gain competitive advantage, and foster innovation in their business processes.
Top 10 examples of data modernization #
Data modernization is a critical step for businesses aiming to thrive in today’s fast-paced digital landscape. Let’s delve into some prominent examples of data modernization that illustrate this transformative journey.
They are:
- Migrating to cloud-based storage and processing
- Implementing data lakes
- Adopting big data technologies
- Integrating advanced analytics and business intelligence tools
- Automating data governance and quality control
- Utilizing IoT (Internet of Things) for data collection
- Applying AI and machine learning for data processing
- Modernizing data architecture for scalability and flexibility
- Enhancing data security and privacy measures
- Adopting a data-as-a-service (DaaS) approach
Let’s understand these examples shortly.
1. Migrating to cloud-based storage and processing #
Moving data storage and computing from on-premises servers to cloud services. This shift not only provides scalability and flexibility but also often enhances data security and accessibility.
2. Implementing data lakes #
Creating data lakes allows organizations to store structured and unstructured data in a single, centralized repository. This approach improves data accessibility and analytics capabilities, enabling more sophisticated data processing techniques like machine learning.
3. Adopting big data technologies #
Utilizing tools and technologies designed for big data, such as Hadoop or Spark, allows organizations to efficiently process and analyze large volumes of data in various formats.
4. Integrating advanced analytics and business intelligence tools #
Incorporating modern analytics and BI tools helps businesses to derive actionable insights from their data. These tools often feature advanced capabilities like predictive analytics and data visualization.
5. Automating data governance and quality control #
Implementing automation in data governance helps in maintaining data quality, compliance, and security. Automated workflows can ensure data integrity and streamline the management process.
6. Utilizing IoT (Internet of Things) for data collection #
Leveraging IoT devices for data collection provides real-time, high-velocity data from a variety of sources, which can be used for enhanced analytics and decision-making.
7. Applying AI and machine learning for data processing #
Utilizing artificial intelligence and machine learning algorithms can transform data processing, enabling more sophisticated analysis and predictive modeling.
8. Modernizing data architecture for scalability and flexibility #
Redesigning data architecture to be more modular and scalable, allowing for easier integration of new technologies and accommodating growing data volumes.
9. Enhancing data security and privacy measures #
Upgrading security protocols and privacy measures to protect sensitive data against emerging cyber threats and to comply with new regulations like GDPR.
10. Adopting a data-as-a-service (DaaS) approach #
Offering data and analytics capabilities as a service to internal and external stakeholders, facilitating easier access and collaboration.
These examples reflect the shift towards more agile, scalable, and sophisticated data management practices that can support the dynamic needs of modern businesses.
Data modernization strategy: How can you create it in 10 steps? #
Developing a data modernization strategy is crucial for organizations looking to leverage data more effectively in today’s fast-paced and data-driven business landscape. A well-thought-out data modernization strategy can help improve data quality, accessibility, and agility, leading to better decision-making and competitive advantages.
Here are the steps in a data modernization strategy:
- Define objectives and goals
- Assess current state
- Identify business drivers
- Formulate a data strategy
- Prioritize initiatives
- Technology selection
- Data governance
- Data integration and migration
- Data security and compliance
- Data analytics and insights
Here are the steps to develop a data modernization strategy in detail:
1. Define objectives and goals #
Start by clearly defining your organization’s objectives and goals for data modernization. Understand what you aim to achieve, such as improving data analytics, enabling data-driven decision-making, reducing data silos, or enhancing data security.
2. Assess current state #
Conduct a comprehensive assessment of your organization’s current data infrastructure, processes, and capabilities. This includes:
- Identifying existing data sources, data types, and data storage systems.
- Evaluating data quality, accuracy, and completeness.
- Assessing data governance practices and compliance with data regulations.
- Analyzing data integration, data warehousing, and data analytics tools and technologies in use.
3. Identify business drivers #
Understand the specific business drivers that motivate your data modernization efforts. Common drivers include improving customer experience, optimizing operations, and uncovering new revenue opportunities.
4. Formulate a data strategy #
Based on your objectives, assessment, and business drivers, formulate a comprehensive data strategy. This strategy should outline:
- The desired future state of your data architecture.
- Key performance indicators (KPIs) to measure success.
- Data governance policies and procedures.
- Data security and compliance measures.
- Data integration and data management methodologies.
5. Prioritize initiatives #
Identify and prioritize specific data modernization initiatives based on their potential impact and alignment with your data strategy. Prioritization may consider factors like cost, complexity, and the expected return on investment.
6. Technology selection #
Choose the right technologies and tools to support your data modernization efforts. This might involve selecting data integration platforms, data lakes, data warehouses, data quality tools, analytics tools, and more. Ensure that the selected technologies align with your strategy and can scale as needed.
7. Data governance #
Develop a robust data governance framework to ensure data quality, security, compliance, and accountability. Define roles and responsibilities, data ownership, and data stewardship processes. Implement data governance policies and standards.
8. Data integration and migration #
Plan and execute data integration and migration strategies. This may involve extracting data from legacy systems, transforming it to fit the new architecture, and loading it into modern data storage systems. Data integration should ensure data consistency and quality.
9. Data security and compliance #
Implement robust data security measures to protect sensitive information. Ensure compliance with relevant data privacy regulations (e.g., GDPR, CCPA) and industry-specific standards (e.g., HIPAA for healthcare).
10. Data analytics and insights #
Build or enhance data analytics capabilities to extract actionable insights from your data. Invest in data visualization tools, machine learning, and artificial intelligence to derive value from your data assets.
Data modernization is an ongoing process, and as technology and business requirements evolve, your strategy should evolve with them. By following these steps and maintaining a commitment to data modernization, your organization can harness the full potential of its data assets to gain a competitive advantage.
10 Best practices to adopt an effective data migration strategy #
When undertaking a data modernization exercise, it’s important to follow best practices to ensure a successful and efficient transformation.
Here are some key best practices to keep in mind:
- Define clear objectives
- Establish data governance
- Focus on data quality
- Adopt agile methodology
- Plan for data security and privacy
- Foster data collaboration
- Embrace scalable and cloud-based solutions
- Invest in data literacy and training
- Monitor and measure success
- Seek external expertise
Let us understand each of them in bit of detail:
1. Define clear objectives #
Clearly define the goals and objectives of your data modernization initiative. Understand the specific business problems you aim to address and the outcomes you expect to achieve. This clarity will help guide your strategy and implementation approach.
2. Establish data governance #
Implement robust data governance practices to ensure data quality, security, and compliance. Define data ownership, establish data standards, and enforce data policies and procedures. Data governance provides the foundation for effective data management throughout the modernization process.
3. Focus on data quality #
Prioritize data quality during data modernization. Invest in data cleansing, validation, and standardization processes to improve data accuracy, consistency, and reliability. High-quality data is essential for meaningful insights and confident decision-making.
4. Adopt agile methodology #
Apply agile principles to your data modernization project. Break the project into smaller, manageable tasks and iterations. Embrace flexibility, collaboration, and regular feedback to adapt and iterate as needed. Agile practices promote efficiency and reduce risks associated with large-scale transformations.
5. Plan for data security and privacy #
Incorporate robust security and privacy measures into your data modernization initiative. Ensure that sensitive data is protected through encryption, access controls, and data masking techniques. Comply with relevant data protection regulations and establish protocols for data privacy and consent.
6. Foster data collaboration #
Encourage collaboration and cross-functional teamwork throughout the data modernization process. Involve stakeholders from various departments to ensure their needs are addressed, and their insights are incorporated. Collaboration fosters a shared understanding of data and promotes a data-driven culture within the organization.
7. Embrace scalable and cloud-based solutions #
Consider adopting scalable and cloud-based technologies for data storage, processing, and analytics. Cloud platforms offer flexibility, scalability, and cost-effectiveness. Leverage modern data storage and processing capabilities to handle growing data volumes and support advanced analytics.
8. Invest in data literacy and training #
Promote data literacy within your organization by providing training and education on data concepts, tools, and best practices. Ensure that teams have the necessary skills to effectively analyze and interpret data. This empowers employees to leverage data insights and make data-driven decisions.
9. Monitor and measure success #
Establish metrics and key performance indicators (KPIs) to measure the success of your data modernization initiative. Regularly monitor and assess the impact of your efforts against defined objectives. Use the insights gained to refine your approach and continuously improve your data modernization processes.
10. Seek external expertise #
Consider engaging external experts, consultants, or partners with experience in data modernization. Their expertise can provide valuable insights, guidance, and support throughout the process, helping you navigate challenges and accelerate your modernization journey.
By adhering to these best practices, you can optimize your data modernization exercise and maximize the value of your data assets. A thoughtful and strategic approach will enable you to drive actionable insights, improve operational efficiency, and achieve your business goals in the data-driven era.
What is the role of data governance in data modernization? #
Data governance plays a critical role in data modernization efforts. It provides a framework for managing and ensuring the availability, integrity, security, and compliance of an organization’s data assets throughout their lifecycle.
These are the following roles:
- Data quality and consistency
- Data ownership and accountability
- Data privacy and security
- Data compliance and regulatory requirements
- Data cataloging and metadata management
- Data integration and interoperability
- Data lifecycle management
Here’s a detailed explanation of the role of data governance in data modernization:
1. Data quality and consistency #
Data governance establishes data quality standards, guidelines, and processes to ensure that data is accurate, complete, consistent, and reliable.
It involves defining:
- Data validation rules
- Data cleansing procedures
- Data standardization practices
By maintaining high data quality, organizations can trust the insights derived from their data and make informed decisions based on reliable information.
2. Data ownership and accountability #
Data governance defines roles, responsibilities, and accountability for data management. It establishes data ownership, specifying individuals or teams responsible for data assets.
It ensures that there is clear ownership and accountability throughout the organization. Data owners are responsible for data accuracy, security, and compliance, driving data stewardship and data management best practices.
3. Data privacy and security #
With data privacy regulations such as GDPR (General Data Protection Regulation) and CCPA (California Consumer Privacy Act) becoming increasingly stringent, data governance plays a crucial role in ensuring compliance.
It establishes policies, procedures, and controls to:
- Protect sensitive data
- Define data access levels
- Manage data sharing and consent.
Data governance ensures that appropriate measures are in place to safeguard data privacy and mitigate the risk of data breaches or unauthorized access.
4. Data compliance and regulatory requirements #
Data governance helps organizations comply with various regulatory requirements, industry standards, and internal policies.
It involves:
- Establishing data governance frameworks aligned with regulatory guidelines,
- Ensuring adherence to data retention and disposal policies
- Maintaining audit trails and documentation
Data governance provides the necessary controls and mechanisms to demonstrate data compliance during audits or regulatory inspections.
5. Data cataloging and metadata management #
Data governance involves creating and maintaining a data catalog that provides a comprehensive inventory of the organization’s data assets.
A data catalog includes metadata, such as
- Data definitions
- Data lineage
- Data classifications
- Data usage information
Effective metadata management through data governance enables data discovery, promotes data reuse, and facilitates understanding and collaboration across teams and departments.
6. Data integration and interoperability #
Data governance establishes standards and best practices for data integration and interoperability. It ensures that data is exchanged seamlessly and accurately between different systems, applications, and departments within the organization.
Data governance sets guidelines for
- Data mapping
- Data formats
- Data transformation, enabling smooth data integration processes during data modernization initiatives.
7. Data lifecycle management #
Data governance defines processes and policies for data lifecycle management, encompassing data creation, storage, usage, archival, and disposal.
It ensures that data is managed efficiently throughout its lifecycle, reducing
- Data redundancy
- Optimizing storage costs
- Ensuring data availability when needed
Data governance also includes data backup and disaster recovery strategies, ensuring data resilience and business continuity.
Overall, data governance provides the necessary structure, policies, and practices to manage data effectively during data modernization initiatives. It promotes data integrity, trust, compliance, and collaboration across the organization, enabling successful data-driven decision-making, improved operational efficiency, and mitigated risks associated with data management.
Summarizing it all together #
Data modernization is the process of transforming and optimizing accumulated data into actionable insights. It involves adopting contemporary technologies and strategies to overcome data silos and drive results.
Key benefits of data modernization include improved decision-making, enhanced operational efficiency, better customer experiences, regulatory compliance, scalability, and gaining a competitive advantage. Components of data modernization include data integration, cleansing, warehousing, analytics, cloud computing, and data governance.
Atlan, a data catalog, plays a vital role in data modernization by providing centralized data cataloging, governance, collaboration, and insights. This ensures successful data modernization, enabling organizations to harness the full potential of their data for informed decision-making and business growth.
Data modernization: Related reads #
- The Future of the Modern Data Stack in 2024 4 new emerging trends and 6 big trends from last year
- Metadata Management: Benefits, Automation, Best Practices, and Tools
- What is metadata management and why is it so important?
- Top 6 Metadata Management Best Practices for 2024
- Active Metadata: Definition, Characteristics, Use Cases & More
- Enterprise Metadata Management and Its Importance in the Modern Data Stack
- Snowflake Metadata Management: Importance, Challenges, and Identifying The Right Platform
- Databricks Metadata Management FAQs, Tools, Getting Started
- Data Catalog Vs. Metadata Management: Differences, and How They Work Together?
- Difference between Master Data Management(MDM) and Metadata Management
Share this article