Data Trust Value Chain 101: From Creation to Analysis
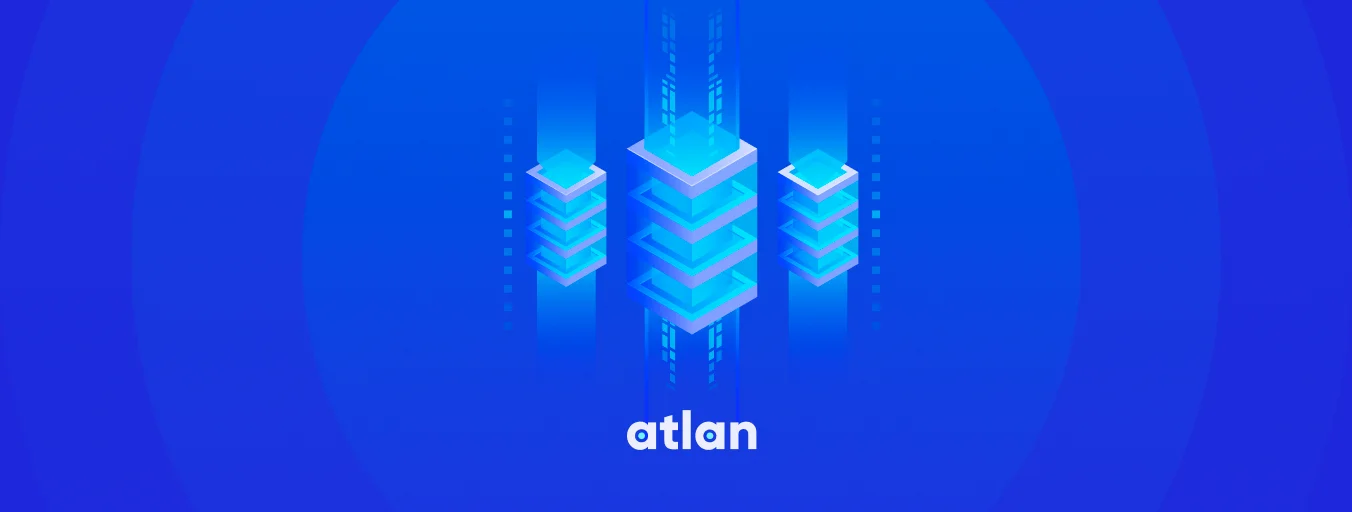
Share this article
A data trust value chain can be thought of as a sequential set of activities and interactions that generate value from data, from its creation and collection to its final use, all underpinned by a framework of trust.
This model aims to ensure data integrity, security, and ethical use, thereby enhancing both the reliability and the value of data in various applications and settings.
In this article, we will learn the sequential stages of generating, collecting, analyzing, and exchanging data, all under a framework of trust.
Table of contents #
- What is a data trust value chain?
- Purpose of the data trust value chain
- 4 steps to build a data trust value chain
- Data trust value chain model
- How can you perform data trust value chain analysis?
- Summary
- Related reads
What is a data trust value chain? #
The data trust value chain is a structured framework that outlines the sequential stages of data management—from its generation and collection to analysis and exchange—while emphasizing the role of trust at each phase.
Edward Curry truly said, “A well-functioning working data ecosystem must bring together the key stakeholders with a clear benefit for all.”
Essentially, this chain ensures that data moves seamlessly, securely, and ethically through different stages and stakeholders involved in a data ecosystem. It adds layers of trust and governance at each stage, making sure that the data is reliable, accurate, and used responsibly.
This chain comprises various actors such as data providers, processors, consumers, and regulators, each playing a unique role in adding value to the data.
If you want to read how data governance is bringing trust in data then read → Objectives of Data Governance: Trust In Data
Purpose of the data trust value chain: Why do you need them? #
The purpose of a data trust value chain is multifaceted.
Firstly, it aims to instil confidence among all stakeholders by offering transparent governance and compliance frameworks. These frameworks can define the legal, ethical, and technical norms that dictate how data should be handled, thereby enhancing the integrity of the data space.
Secondly, the trust value chain is designed to break down the so-called “data silos,” promoting a more open and collaborative data ecosystem. It encourages the sharing and reuse of data, offering the potential to unlock new insights or create new services that might not have been possible within isolated data repositories.
Lastly, the data trust value chain serves as an enabler for innovation. Establishing clear rules and trust mechanisms, lowers the barriers for smaller players to enter the space and interact with data. This results in the democratization of data, making it easier for innovators to create value-added services.
In summary, a data Trust Value Chain not only governs how data is managed and exchanged but also acts as a catalyst for innovation and value creation in the larger data ecosystem.
Now as we are conceptually clear about the data trust value chain and its purpose, we can head towards the process of building one for your organization.
4 steps to build a data trust value chain #
Let us delve into each of these four main steps of the Data Trust Value Chain to better understand their significance and functionality.
- Data generation: Capture and record data
- Data collection: Collect, validate, and store data
- Data analysis: Process and analyze data to generate new potential insights
- Data exchange: Expose the data outputs to use, whether internally or externally with partners
1. Data generation: Capture and record data #
The first step in the data trust value chain is data generation, where raw data is captured and recorded from various sources.
These could range from:
- Sensors and devices in an IoT environment
- User interactions on a website
- Transactional data in a retail setting
- Numerous other sources depending on the domain
In this phase, trust is essential to ensure that the generated data is accurate, reliable, and collected in compliance with legal and ethical standards.
Ensuring that the data is of high quality at the point of generation sets the stage for the subsequent phases in the value chain. If trust is compromised at this stage, it could propagate errors or uncertainties throughout the entire chain.
2. Data collection: Collect, validate, and store data #
Once data is generated, the next step is to collect it for further use. This involves not just the aggregation of data but also its validation to confirm its quality and integrity. Inconsistent, inaccurate, or incomplete data can lead to misleading insights down the line.
Trust is built into this phase through rigorous validation algorithms, security measures, and compliance checks. Data is then stored in databases, data lakes, or other storage solutions, where it’s critical to have robust security measures to ensure data integrity and confidentiality.
Essentially, this stage prepares the data for analysis while maintaining its quality and safeguarding it from potential risks.
To know more about the best practices to ensure data integrity, check out the following article →7 Data Integrity Best Practices You Need to Know
3. Data analysis: Process and analyze data to generate new potential insights #
Data that has been collected and stored is processed and analyzed in this phase. Techniques can range from basic statistical analysis to more complex machine learning models. The aim is to extract valuable insights that can be acted upon.
The trust element in this phase involves ensuring that the analytical models are transparent, fair, and free from biases.
Data governance and auditing practices often come into play here, ensuring that data is used responsibly and that the derived insights are both reliable and ethical. Depending on the complexity and the type of data, multiple iterations might be required to refine the models and insights.
4. Data exchange: Expose the data outputs to use, whether internally or externally with partners #
The final phase is data exchange, where the analyzed data or insights are made available for internal or external use. This could involve sharing data with other departments within the same organization, or with external partners, clients, or even open data initiatives.
The trust aspect here is paramount, as this is where data often leaves the controlled environment of its original repository. Governance policies, data masking, encryption, and secure APIs are techniques used to maintain trust.
Explicit consent, privacy agreements, and contractual obligations often come into play in this stage to regulate how the data can be used by the third parties.
To know more about best tools on data masking check out → 11 Best Data Masking Tools and Software
In summary, each phase of the Data trust value chain builds upon the trust established in the previous stages, ensuring that the data is reliable, secure, ethical, and valuable from generation to its final use. By carefully managing each step, organizations can maximize the value derived from data while minimizing risks and ensuring compliance with relevant regulations and ethical standards.
Now let us learn about the data trust value chain models with their respective examples.
Data trust value chain model: Explained with examples #
Applying the concept of a Data trust value chain to a real-world example can help clarify how each of these four main steps—data generation, data collection, data analysis, and data exchange—function in practice.
Let’s consider a healthcare scenario involving the use of wearable devices for patient monitoring.
Example: Wearable Health Monitors for Diabetes Management #
Data generation
- In this example, patients wear a device that continuously monitors their blood sugar levels, physical activity, and other relevant health metrics. The device captures this raw data in real time.
- Trust in this phase is crucial; patients need to be confident that the device is accurately measuring their vitals. Manufacturers, therefore, adhere to stringent quality control and calibration processes.
- Trust mechanism applied: Regulatory approval (e.g., FDA approval in the U.S.) for the wearable device assures users that the data generated meets specific accuracy and reliability standards.
Data collection
- The wearable device transmits the gathered data to a secure cloud storage system managed by a healthcare provider. Here, validation algorithms ensure that the received data is within expected ranges to rule out device malfunctions or data corruption. This stored data is then securely encrypted to protect patient confidentiality.
- Trust mechanism applied : Security protocols, such as end-to-end encryption and multi-factor authentication, ensure that patient data is stored securely. Compliance with healthcare data regulations like HIPAA in the U.S. further strengthens trust.
Data analysis
- Healthcare providers and data scientists may run sophisticated algorithms on this collected data to identify patterns or trends, such as the best time of day for each patient to take their medication or triggers that may cause blood sugar spikes.
- Trust mechanism applied: Transparent algorithms, peer reviews, and ethical guidelines help ensure that the analysis is accurate and unbiased. Patients can view summaries of their health trends, thereby validating the insights generated and building trust.
Data exchange
- The analyzed data and insights might be shared with different healthcare professionals (e.g., doctors, and pharmacists) for better treatment plans. They might also be used in anonymized, aggregated forms for academic research or to improve healthcare policies.
- Trust mechanism applied: Data sharing agreements specify the conditions under which data can be used or shared, ensuring it aligns with the patient’s consent and privacy laws. Secure APIs ensure that data transfer occurs safely.
In this example, each stage of the Data trust value chain builds on the trust and value generated in the previous stages. The system is designed not only to manage data effectively but also to generate actionable, trustworthy insights that contribute to better healthcare outcomes. By implementing trust mechanisms at every step, from device calibration to data-sharing agreements, the entire chain aims to maximize both utility and trustworthiness.
Analysing data trust value chain is as important as preparing one, let us know the tips to analyse your value chain.
How can you perform data trust value chain analysis? #
Performing a data trust value chain analysis involves a structured evaluation of each phase of the chain, from data generation to data exchange. This type of analysis aims to identify gaps, risks, and opportunities to improve trust and add value to data-driven operations.
Here are detailed steps to conduct a Data trust value chain:
1. Scope definition #
Before diving into the analysis, clearly define the scope of the data activities you’re examining. Are you focusing on a specific department, the entire organization, or a multi-partner data ecosystem? Knowing the scope will set the stage for a more focused analysis.
2. Stakeholder identification #
Identify all the stakeholders involved in each phase of the data trust value chain. This could range from data generators and collectors to processors, analysts, and end-users. List out their roles, responsibilities, and how they interact with each other.
3. Data flow mapping #
Create a visual map or diagram of how data flows through the value chain. Include sources of data generation, collection points, storage locations, analysis tools, and points of data exchange. This provides a high-level view that helps in identifying bottlenecks or vulnerabilities.
4. Trust mechanism audit #
For each phase, audit the existing trust mechanisms. These can include security protocols, validation checks, legal frameworks, ethical guidelines, and so forth. Evaluate their effectiveness in ensuring data integrity, quality, and security.
5. Risk assessment #
Perform a comprehensive risk assessment at each phase, focusing on potential areas where trust could be compromised. This might include vulnerabilities in data storage, risks of bias in data analysis, or gaps in compliance with legal and ethical standards.
6. Value assessment #
Simultaneously, assess how much value each stage is adding to the data. Are the collection mechanisms robust enough to capture high-quality data? Is the analysis sophisticated enough to extract meaningful insights? Are there opportunities to derive more value at each stage?
7. Gap identification #
Based on the trust and value assessments, identify gaps or areas for improvement. These could be weak links that require strengthened trust mechanisms, or stages where additional value could be generated with different tools, algorithms, or practices.
8. Recommendations and action plan #
Develop a set of recommendations aimed at improving both the trustworthiness and the value generated by the data activities. Prioritize these actions based on their impact and feasibility.
9. Implementation and monitoring #
Execute the recommended actions and establish KPIs to monitor their effectiveness. This should be a continuous process, periodically re-evaluating the trust and value in the chain as technologies, regulations, and business needs evolve.
10. Documentation and reporting #
Finally, document all findings, actions, and outcomes of the analysis. Share this documentation with relevant stakeholders to maintain transparency and continue building trust within the ecosystem.
Performing a data trust value chain analysis is not a one-time task; it is an ongoing process. As technologies advance and business objectives change, it’s essential to periodically reassess the data trust value chain to ensure it remains resilient, ethical, and valuable.
Summarizing it all together #
Sanctity of data doesn’t merely lie in its volume or complexity, but in the trustworthiness and value it provides to stakeholders at each stage. From the initial capture of raw data to its transformation into actionable insights, data trust value chain serves as the glue that binds the entire process.
By rigorously assessing and optimizing each phase of the data lifecycle, organizations can not only mitigate risks but also unlock unprecedented opportunities for innovation and growth.
Remember, in a data-driven world, trust isn’t just an optional add-on; it’s a foundational pillar that empowers us to extract the maximum value from our data assets.
Data trust value chain: Related reads #
- How to Drive Business Value With Data Governance?
- Key Objectives of Data Governance: How Should You Think About Them?
- Data Quality Explained: Causes, Detection, and Fixes
- Data Quality Metrics: Understand How to Monitor the Health of Your Data Estate
- Data Quality in Data Governance: The Crucial Link that Ensures Data Accuracy and Integrity
- Data Governance 101: Principles, Examples, Strategy & Programs
- Data Governance Policy — Examples & Templates
- Data Governance Roles and Responsibilities: A Quick Round-Up
Share this article