6 Ways Metadata Helps You Find Specific Data
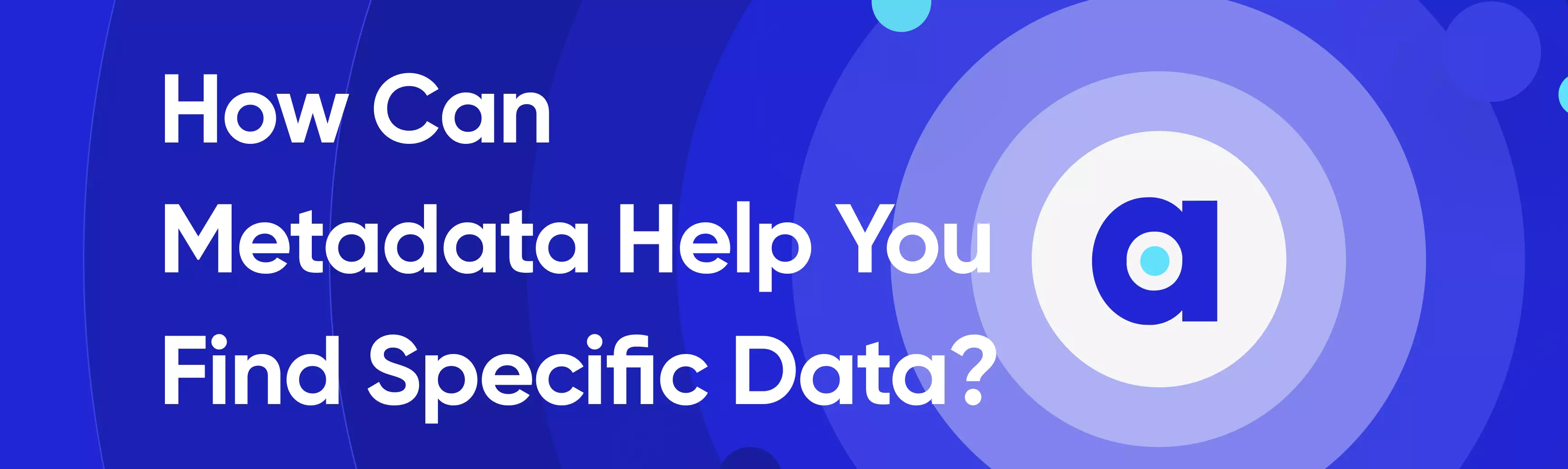
Share this article
Metadata is a powerful tool that enables you to manage and organize your data more effectively by providing context and additional information about your data. It helps you categorize, standardize, and establish relationships between different data sets, making it easier to find the data you need.
In the following sections, we’ll take a closer look at how metadata works to improve data discoverability and help you find specific data sets more quickly and easily.
Table of contents
- How metadata helps find specific data with ease and efficiency
- Understanding the different types of metadata to improve data discovery
- Why is metadata important for efficient data discovery?
- Rounding it all up
- How can metadata help you find specific data: Related reads
How metadata helps find specific data with ease and efficiency
Metadata is often referred to as “data about data” and plays a crucial role in organizing, understanding, and managing data within an organization. It can help your team find specific data more easily and efficiently by providing context and additional information about the data.
Here’s a breakdown of how metadata works to improve data discoverability:
1. Categorization
Metadata helps categorize and classify data by attaching descriptive information such as data type, format, and source. This categorization makes it easier to find specific data sets by filtering or searching based on these attributes.
2. Standardization
Metadata establishes a common language and standards for data, which helps users understand the data and its purpose more clearly. This standardization helps reduce confusion and misinterpretation of data, leading to more efficient data discovery and usage.
3. Relationships
Metadata can also describe relationships between different data sets, helping users understand how data is interconnected. This can help them identify related data sets that may be relevant to their analysis or work.
4. Data lineage
Metadata can provide information about the data lineage, which is the history of the data, including its origin, transformations, and usage. Understanding data lineage helps users trust the data and makes it easier to identify the specific data they need for their analysis.
5. Search and discovery
Metadata is critical for search and discovery purposes, as it enables users to find specific data sets by searching for keywords or attributes. The metadata can be indexed and used by search engines within your metadata management solution, making it faster and easier for users to locate the data they need.
6. Access and security
Metadata can also include information about access and security restrictions, ensuring that users can easily identify if they have permission to access certain data sets or if additional authorization is needed.
By implementing a metadata management solution, your organization can benefit from increased efficiency, better collaboration, and reduced dependency on tribal knowledge. Your data team will be able to locate specific data sets more quickly and easily, which will in turn reduce the number of service requests and improve overall data visibility.
Additionally, having a single source of truth for data will ensure that your organization is working with accurate, up-to-date information, which is essential for making informed business decisions.
Understanding the different types of metadata to improve data discovery
There are several types of metadata that can help you find specific data. Here’s a list of common metadata categories that are particularly useful for data discovery:
1. Descriptive metadata
This type of metadata provides information about the content and context of the data, such as the title, description, keywords, and data owner. Descriptive metadata makes it easier for users to find and understand the purpose of specific data sets.
2. Structural metadata
Structural metadata describes the organization and relationships between data sets or elements within a data set. This can include information about the schema, tables, fields, and hierarchies. Structural metadata helps users navigate complex data systems and understand how data is related.
3. Administrative metadata
This metadata deals with the management aspects of data, including information about data creation, updates, versioning, and access control. Administrative metadata helps users determine if they have the necessary permissions to access specific data sets and understand the history of the data.
4. Technical metadata
Technical metadata provides information about the format, storage, and technical specifications of the data, such as file type, encoding, compression, and data storage location. This metadata helps users understand the technical requirements for accessing and processing the data.
5. Data quality metadata
This type of metadata assesses the quality and reliability of the data, including information about data accuracy, completeness, and consistency. Data quality metadata helps users evaluate the trustworthiness of the data for their purposes.
6. Data lineage metadata
As mentioned earlier, data lineage metadata provides the history of the data, including its origin, transformations, and usage. This metadata helps users understand how the data has changed over time and can be crucial for regulatory compliance and ensuring data integrity.
7. Data usage metadata
This metadata captures information about how the data is being used within the organization, including information about user access, frequency of use, and common use cases. Data usage metadata can help identify popular data sets and inform data management decisions.
By considering these different types of metadata, you can create a comprehensive metadata management solution that aids users in finding and understanding specific data sets more effectively. This, in turn, will lead to better collaboration, increased efficiency, and more informed decision-making within your organization.
Why is metadata important for efficient data discovery?
Let’s consider three hypothetical examples where different types of metadata can help you discover specific data within your organization.
Example 1: Analyzing customer loan default rates
Suppose a data analyst is tasked with analyzing loan default rates for various customer segments. The analyst needs to find relevant data sets to perform this analysis. Here’s how different types of metadata can help:
- Descriptive metadata 👉 The analyst searches for keywords like “loan,” “customer,” and “default rate,” and finds a data set titled “Customer Loan Default Rates by Segment.”
- Structural metadata 👉 The data set includes information about the schema, tables, and fields, helping the analyst understand the relationships between customer segments, loan amounts, and default rates.
- Administrative metadata 👉 The analyst can see that the data set was last updated a month ago and is maintained by the credit risk department, ensuring its relevance and reliability.
- Technical metadata 👉 The data set is stored in CSV format and is accessible through the company’s data warehouse, allowing the analyst to import it into their preferred analysis tool.
- Data quality metadata 👉 The analyst reviews the accuracy, completeness, and consistency of the data set, confirming its suitability for the analysis.
- Data lineage metadata 👉 The analyst learns that the data is sourced from the company’s loan management system and has undergone specific data cleaning and transformation processes.
- Data usage metadata 👉 The analyst sees that the data set is frequently used for credit risk analysis, suggesting it is a popular and relevant resource for this type of work.
Example 2: Identifying High-Value Customer Segments
Suppose a marketing manager wants to create a targeted campaign for high-value customers. They need to find data sets that help identify these customers. Here’s how metadata can help:
- Descriptive metadata 👉 The manager searches for keywords like “customer,” “revenue,” and “segment” and finds a data set called “Customer Lifetime Value by Segment.”
- Structural metadata 👉 The data set contains information about customer demographics, purchase history, and calculated lifetime value, which helps the manager understand the relationships between these variables.
- Administrative metadata 👉 The manager checks the data set’s access permissions and verifies they have the necessary authorization to view the data.
- Technical metadata 👉 The data set is stored in a cloud-based database and can be accessed through an API, allowing the manager to integrate it with their marketing automation platform.
- Data quality metadata 👉 The manager reviews the data quality information to ensure the data set is accurate and reliable for their campaign targeting.
- Data lineage metadata 👉 The manager learns the data set is updated monthly and sources its data from the company’s CRM and billing systems.
- Data usage metadata 👉 The manager sees that the data set is frequently used for marketing analysis, indicating it is a trusted and relevant resource for their campaign planning.
Example 3: Evaluating loan approval model performance
Suppose a data scientist is tasked with evaluating the performance of a machine learning model used to approve or reject loan applications. They need to find data sets containing historical loan applications and model predictions. Here’s how metadata can help:
- Data quality metadata 👉 The data scientist reviews the data quality information to ensure the data set is accurate, complete, and consistent, allowing for a reliable evaluation of the model performance.
- Data lineage metadata 👉 The data scientist learns that the data set is derived from the company’s loan application system and includes data on the model’s predictions and actual loan outcomes, which helps them understand the data’s origin and transformation processes.
- Data usage metadata 👉 The data scientist sees that the data set is frequently used for model performance evaluations, indicating it is a relevant and trusted resource for their analysis.
By leveraging different types of metadata in these examples, users can efficiently discover the specific data sets they need for their analysis, leading to better decision-making, increased efficiency, and improved collaboration within the organization.
Rounding it all up
- Metadata is essential for data discovery, as it provides context and additional information about the data, helping users find specific data sets more easily and efficiently.
- Different types of metadata can aid data discovery, including descriptive, structural, administrative, technical, data quality, data lineage, and data usage metadata.
- By implementing a metadata management solution and leveraging these different types of metadata, your organization can benefit from increased efficiency, better collaboration, and reduced dependency on tribal knowledge.
- Metadata helps users locate specific data sets more quickly and easily, reducing the number of service requests and improving overall data visibility.
- Having a single source of truth for data ensures that your organization is working with accurate, up-to-date information, which is crucial for making informed business decisions.
- The hypothetical examples provided illustrate how different types of metadata can help users in various scenarios to discover specific data sets, ultimately enabling more efficient and effective decision-making processes within the organization.
By understanding and utilizing metadata, your organization can create a more streamlined data management system that improves data accessibility and discoverability, leading to better decision-making and increased productivity across your data teams.
Robust metadata management is the key for data-driven teams to discover, understand, trust, and collaborate on data assets across your data universe. If you are looking to implement a metadata management tool for your organization, do take Atlan for a spin.
How can metadata help you find specific data: Related reads
- Active Metadata: 101 Guide From Pioneers Of The Concept
- What is Metadata? - Examples, Benefits, and Use Cases
- What Is a Metadata Catalog? — Basics & Use Cases
- What is Metadata Management and Why is it So Important?
- How Operational Metadata Drives Business Success: A Comprehensive Guide
- Mastering Business Metadata: How to Achieve Data Excellence and Streamlined Collaboration
Share this article