How to Improve Data Literacy? 11 Proven Steps for 2024 & Beyond
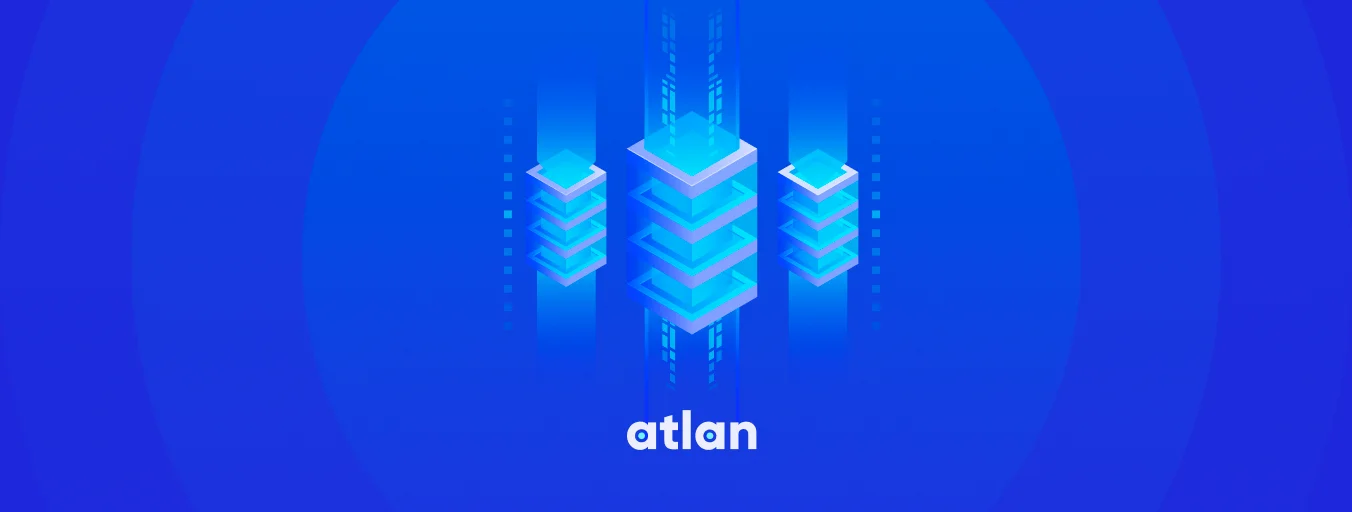
Share this article
Data literacy isn’t just for tech specialists; it’s becoming a crucial skill for professionals across diverse sectors and even for the general public. As we increasingly rely on data to make decisions, from business strategies to policy-making, it’s paramount to understand how to read, comprehend, and act upon this data.
Improve data literacy, and you unlock the power to interpret, analyze, and utilize the vast amounts of information in today’s digital age. Data literacy is the ability to read, understand, analyze, and communicate with data.
Modern data problems require modern solutions - Try Atlan, the data catalog of choice for forward-looking data teams! 👉 Book your demo today
In this article, we will understand:
- Data literacy and its key 9 components
- 11 Steps to improve data literacy
- What are the top 11 data literacy skills?
Ready? Let’s dive in!
Table of contents #
- What is data literacy: A quick sneak peek!
- 11 Steps to improve data literacy
- Top data literacy skills to know in 2024
- 11 Best practices to improve data literacy skills
- Summary
- Improve data literacy: Related reads
What is data literacy: 9 key components to know #
Data literacy is the ability to read, understand, analyze, and communicate with data. It encompasses the skills and knowledge required to work with data effectively, allowing individuals to extract valuable insights, make informed decisions, and communicate their findings in a meaningful way.
Data literacy is not a monolithic skill but a multi-faceted competency composed of several key components. The components are:
- Data identification and sourcing
- Data understanding
- Data cleaning and preparation
- Data analysis
- Statistical literacy
- Data visualization
- Data interpretation and storytelling
- Ethical considerations and data privacy
- Skepticism and critical thinking
Let’s explore each of them in detail:
1. Data identification and sourcing #
- Before any data can be analyzed, it needs to be identified and sourced. Understanding where to find reliable data is crucial.
- Whether it’s public records, social media metrics, or scientific studies, the source of the data is often as important as the data itself.
- Knowing how to identify reputable sources and extract data for analysis is the first step in becoming data literate.
2. Data understanding #
- Once you have the data, the next step is understanding what the data represents.
- This includes familiarity with different types of data (qualitative vs quantitative, structured vs. unstructured) and the units of measurement involved.
- Recognizing the limitations and scope of the data is also crucial; not all data is created equal or suitable for every kind of analysis.
3. Data cleaning and preparation #
- Dirty or poorly formatted data can significantly impact the quality of insights derived from it.
- Learning how to clean and prepare data for analysis is essential. This may include dealing with missing values, removing outliers, or transforming data into a format suitable for analysis.
4. Data analysis #
- Data analysis is often what people first think of when they hear “data literacy,” but as we’ve seen, it’s just one component.
- Analysis involves using statistical tools or software to interpret the data. This can range from calculating simple averages to more complex tasks like predictive modelling.
5. Statistical literacy #
- Understanding basic statistical concepts like mean, median, variance, standard deviation, and correlation is essential for interpreting data effectively.
- A grasp of more complex statistical methods like regression analysis or machine learning algorithms can be advantageous but is not essential for basic data literacy.
6. Data visualization #
- The ability to represent data visually is invaluable for both understanding and communication.
- Well-designed charts, graphs, and dashboards can make complex data easily understandable.
- Knowing how to use tools for data visualization, and understanding the best ways to visualize different types of data, is a key aspect of data literacy.
Check out the following articles about the benefits of data visualization.
7. Data interpretation and storytelling #
- Data by itself is often not impactful until it tells a story or supports a decision. Being able to interpret the data and articulate its implications clearly to others is an essential skill.
- This can involve explaining the significance of a data trend, the ROI (Return on Investment) of a business decision, or the social implications of a public health statistic.
8. Ethical considerations and data privacy #
- In our enthusiasm to derive insights from data, it’s easy to overlook ethical considerations.
- Understanding the ethical implications of data usage, including issues of privacy, consent, and bias, is critical in today’s data-centric world.
9. Skepticism and critical thinking #
- Being data literate doesn’t just mean knowing how to work with data; it means being skeptical of data when warranted.
- Understanding the context in which data was collected and being aware of any potential biases or inaccuracies is crucial for making sound decisions based on data.
By mastering these components, one becomes truly data literate, capable of navigating the complex data landscape we all live in. Each component not only complements the others but is a necessary part of a holistic understanding of what it means to be adept with data.
11 Steps to improve data literacy #
Improving data literacy is essential in a world where data-driven decisions are becoming the norm. Being data literate means having the ability to read, analyze, interpret, and make informed decisions based on data.
Below are 11 steps to improve your data literacy:
Step 1. Understand the basics #
Familiarize yourself with basic statistical concepts like mean, median, mode, standard deviation, and correlation. You don’t have to become a statistician, but a basic understanding of these terms will help you interpret data more effectively.
Step 2. Learn basic data-handling skills #
Learn how to handle data using spreadsheets like Microsoft Excel or Google Sheets. These platforms have built-in functionalities for sorting, filtering, and performing basic analyses.
Step 3. Know your data sources #
Understand where your data comes from. Is it a reliable source? How was it collected? Knowing the source of your data can help you gauge its reliability and validity.
Step 4. Acquire basic data visualization skills #
Learn how to visualize data using simple tools like charts, graphs, and dashboards. Visualization aids in understanding the story that the data is telling. Platforms like Tableau or Power BI can be useful.
Step 5. Start small #
Begin by analyzing small datasets and gradually work your way up to larger, more complex ones. Practice is key to improving your skills.
Step 6. Question the data #
Always be critical of the data you are presented with. Ask questions like, “What does this mean?”, “Is this a significant change?”, “What are the implications?”, etc.
Step 7. Get hands-on experience #
Practical application solidifies understanding. Engage in projects or exercises that require you to analyze and interpret data. You can find various datasets related to different domains online for practice.
Step 8. Learn basic scripting #
Understanding the basics of scripting languages like SQL for databases or Python for data manipulation can be highly advantageous. Libraries like Pandas for Python make data manipulation more efficient.
Step 9. Follow industry experts #
Follow blogs, podcasts, or social media accounts of professionals who specialize in data analysis or data science. This can keep you up-to-date with the latest trends and tools in the field.
Step 10. Take formal courses #
There are many online courses and certifications in data analysis and data science. Taking such courses can formalize your understanding and give you a structured way to improve your skills.
Step 11. Collaborate and share knowledge #
Sharing knowledge helps you solidify your own understanding. Collaborate with others, join online forums, and participate in discussions. Teaching is one of the best ways to learn.
By following these steps, you’ll find that data starts to make more sense and becomes a tool you can use to make better decisions, whether in your personal life, in business, or in scientific research.
Top data literacy skills to know in 2024 #
Data literacy is not just a concept or a set of abstract understandings; it’s a hands-on skill set that allows individuals to interact with data in meaningful ways.
The following are some of the top data literacy skills to know:
- Data collection
- Data organization
- Data cleaning
- Basic statistical analysis
- Advanced statistical analysis and modelling
- Data visualization
- Data interpretation
- Software proficiency
- Ethical reasoning
- Communication skills
Here’s a deep dive into the specific skills that make up data literacy:
1. Data collection #
- The ability to gather data from various sources, whether it be through surveys, data scraping, or manually observing and recording information.
- Data collection is the foundation upon which any form of data analysis is built. Understanding how to collect data accurately and ethically is crucial for obtaining reliable results.
2. Data organization #
- This involves sorting, categorizing, and structuring the data in a manner that facilitates analysis.
- Organized data is easier to work with and reduces the chances of errors during the analysis phase. It helps in saving time and creating a workflow that is easier to understand and replicate.
3. Data cleaning #
- The process of identifying and correcting (or removing) errors and inconsistencies in data to improve its quality.
- Dirty data can lead to misleading results. Data cleaning ensures that the dataset is accurate, complete, and formatted correctly, thus making the subsequent analysis more reliable.
4. Basic statistical analysis #
- Understanding fundamental statistical measures like mean, median, mode, standard deviation, and variance.
- These basic statistics provide initial insights into the dataset and can guide the direction of more advanced analyses.
5. Advanced statistical analysis and modeling #
- Going beyond basic statistics to perform more complex analyses like regression analysis, hypothesis testing, and predictive modeling.
- Advanced statistical techniques allow for a deeper understanding of data, enabling predictive insights and data-driven decision-making.
6. Data visualization #
- The ability to represent data in a visual format, such as graphs, charts, or dashboards.
- Visualization can make complex data more accessible, understandable, and actionable for both technical and non-technical audiences.
7. Data interpretation #
- Translating the findings from the data analysis into actionable insights.
- The whole point of dealing with data is to derive insights that can lead to effective actions or decisions. Data interpretation is the skill that bridges data analysis and decision-making.
8. Software proficiency #
- Familiarity with data analytics tools and software like Excel, SQL, Python, R, and specialized analytics software like Tableau.
- Software tools expedite the process of data analysis and offer functionalities that manual methods cannot achieve.
9. Ethical reasoning #
- Understanding the ethical implications related to data collection, storage, and analysis.
- Misuse of data can lead to a host of ethical and legal issues. Ethical reasoning is crucial for responsible data use.
10. Communication skills #
- The ability to communicate your findings clearly and effectively to various stakeholders through reports, presentations, or conversations.
- Data analysis is only as useful as your ability to communicate its implications. Effective communication ensures that the insights gained from data are understood and actionable.
Each of these skills forms a vital piece of the data literacy puzzle. By honing these skills, one can navigate the increasingly data-driven world with confidence and insight.
11 Best practices to improve data literacy skills #
Improving data literacy is no longer a luxury but a necessity in today’s data-driven world. Whether you’re a professional seeking to make informed decisions based on data or a student looking to bolster your analytical skills.
The following 11 best practices aim to provide a structured path for enhancing your data literacy skills:
- Educational workshops and training
- Online courses and tutorials
- Real-world practice
- Use analytical tools
- Understand data ethics
- Peer learning and discussion
- Analytical thinking exercises
- Read widely on data-related topics
- Consult experts
- Stay updated
- Regular assessment and feedback
Let us understand each of them in detail:
1. Educational workshops and training #
- Workshops provide interactive and specialized learning experiences, often guided by experts in the field.
- Attendees can ask questions, receive personalized guidance, and get hands-on experience with actual data sets.
- Workshops are excellent venues for collaborative learning and networking, allowing participants to also learn from one another’s experiences and perspectives.
2. Online courses and tutorials #
- Online platforms offer the benefit of self-paced learning. Many come with assignments, quizzes, and certifications to validate your learning.
- They cover a wide range of skill levels, from beginners looking to understand the basics of data visualization, to advanced students seeking to master machine learning algorithms.
- The interactive nature of these platforms, often featuring forums and mentor support, enriches the learning experience.
3. Real-world practice #
- Engaging with real-world data sets adds context and relevance to theoretical knowledge.
- By applying what you’ve learned to actual data, you encounter the complexities and nuances of real-world data scenarios.
- This could be in a professional setting, a personal project, or even a community service project where data-driven insights can make a meaningful impact.
4. Use analytical tools #
- Tools or programming languages like R and Python, are standard in data analytics.
- Proficiency in these tools is practically a requirement for any form of in-depth data work.
- Beyond mere tool operation, understanding their capabilities and limitations ensures that you can select the appropriate tool for each analytical task.
5. Understand data ethics #
- Ethical considerations are critical in data handling and interpretation.
- This includes understanding data privacy laws, gaining informed consent when required, recognizing potential biases in data sets, and being transparent about the limitations of your data.
- Being ethical not only guards against misinformation but also builds trust with your audience.
6. Peer learning and discussion #
- Peer-to-peer learning environments foster discussion, clarify doubts, and deepen understanding through shared experiences and insights.
- This could be a study group, an online forum, or even a workplace discussion.
- Diverse perspectives from peers can challenge your understanding and help you consider aspects of data literacy that you might not have encountered otherwise.
7. Analytical thinking exercises #
- Activities like logic puzzles, brain teasers, or even strategy games that require careful planning and problem-solving can sharpen your analytical skills.
- Analytical thinking is foundational to understanding and interpreting data correctly. The stronger your analytical skills, the better you will be at drawing accurate conclusions from data.
8. Read widely on data-related topics #
- Reading books, scholarly articles, or even relevant blog posts expands your understanding of the field.
- It helps you stay updated on the latest methodologies, debates, and breakthroughs. This continuous learning is crucial because the field of data is dynamic and ever-evolving.
9. Consult experts #
- Experts have in-depth knowledge and real-world experience that can provide invaluable insights.
- A single conversation with an expert can sometimes be as enlightening as reading an entire book.
- They can guide you on best practices, common pitfalls, and resources that could significantly accelerate your learning journey.
10. Stay updated #
- Data literacy is an evolving field with new tools, methodologies, and best practices emerging regularly.
- Following reputable journals, attending conferences, and participating in webinars are excellent ways to keep abreast of the latest developments.
11. Regular assessment and feedback #
- Regularly assessing your skills through tests, quizzes, or peer reviews provides tangible metrics on your progress.
- Feedback from these assessments identifies your strengths and weaknesses, guiding you on what areas to focus your learning efforts.
- It acts as a form of quality control for your ongoing education.
Check out this post by Atlan to know how it helped Octane improve its data literacy .
Summary #
Improving data literacy is a multi-faceted endeavour that involves both formal education and practical experience.
Educational platforms like workshops and online courses offer foundational knowledge and specialized skills, often with the benefit of expert guidance. These formal settings are complemented by real-world practice, where theoretical understanding is put to the test in actual data scenarios.
Ethical considerations in data usage are also crucial, emphasizing the importance of responsible data handling and interpretation. Regular assessments offer a way to gauge progress and receive valuable feedback, which helps in fine-tuning learning objectives.
Staying updated with the latest trends and consulting experts can provide additional layers of knowledge and expertise. Overall, the journey to data literacy is continuous and evolves with the rapidly changing landscape of data science and analytics.
Improve data literacy: Related reads #
- What is Data Literacy and Why is It Important?
- Why Data Literacy Matters for Your Business?
- 8 Popular Problems With Data Literacy & How to Overcome Them
- Measure Data Literacy: How to Assess Your Organization’s Understanding and Usage of Data
Share this article