Lexikon: Spotify’s Efficient Solution For Data Discovery And What You Can Learn From It
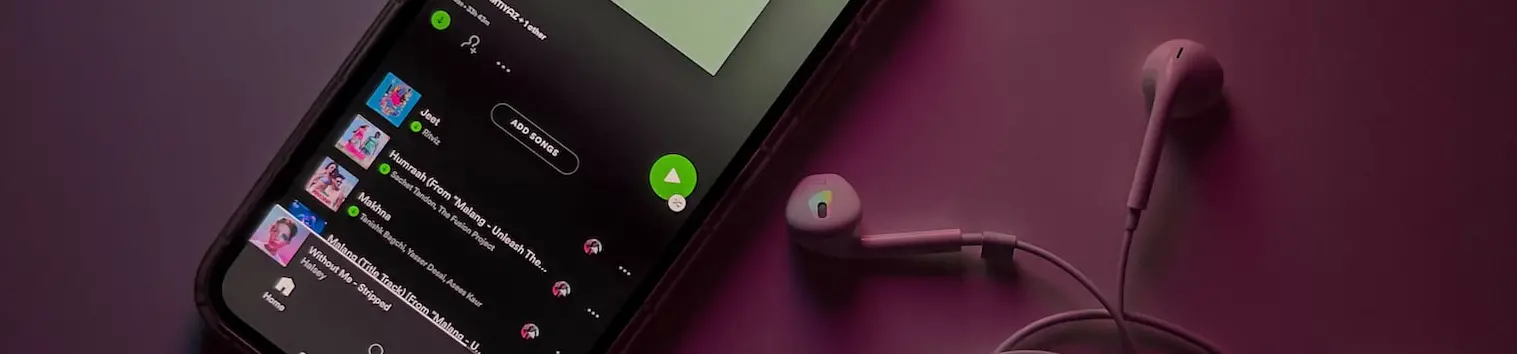
Share this article
Facilitating data discovery, breaking data silos, and enabling knowledge sharing - these are all hard problems. Here is how Spotify addressed these challenges with Lexikon. Also, get insights into real use cases and practical strategies you can use to accelerate the performance of your data team.
Is Open Source really free? Estimate the cost of deploying an open-source data catalog 👉 Download Free Calculator
What is Lexikon? #
Lexikon is Spotify’s in-house solution for facilitating data discovery. It allows data teams to find, share, and use data and knowledge easily, allowing them to unlock business insights more quickly and effectively.
So why all the fuss? And why is data discovery important you may ask? In Q3 of 2022, Spotify reported having over 456M active monthly users. You can imagine how important it is to gather business insights around user behavior, recommended content, creator metrics, advertising revenue and targeting, infrastructure performance, user churn, revenue generated, forecasts, and much, much more.
However, unlocking these insights is no easy challenge. Data teams need an effective way to find and use the datasets they need, share and access internal resources, and build on top of existing knowledge. Lexikon is Spotify’s in-house solution for enabling data discovery at scale.
What problems does Lexikon solve? #
You may be wondering, how exactly did Lexikon help Spotify? And what problems did Spotify face in the beginning? Let’s look at common challenges that Lexikon helped Spotify solve.
- Data discovery was hard and inefficient
- Data teams and members worked in silos
- Difficulty in using datasets after they were found
Data discovery was hard and inefficient #
On average, a data scientist at Spotify uses 25-30 datasets every month. With hundreds of data users and tens of thousands of datasets, one of the main problems reported by data scientists was their inability to find the datasets they needed. Lexikon enabled Spotify’s data scientists to navigate this sea of data and quickly find the datasets they were looking for.
Data teams and members worked in silos #
With different teams working on different projects and people working in different time zones and locations, breaking silos becomes a challenge. How do you know what knowledge or datasets already exist? How do you leverage work done by one team for another team? And how do you promote knowledge sharing across hundreds of data producers and consumers? Lexikon was Spotify’s solution for breaking data silos, enabling teams to share data and knowledge at scale.
Difficulty using datasets after they were found #
Even when datasets were found, it was difficult to quickly make use of their contents. Datasets often had hundreds of fields, so it was not apparent which fields were most useful or how to query the dataset effectively. On top of this, datasets had a lack of metadata describing them, there was lack of ownership and documentation, and it was hard to assess whether a dataset was valuable or not, let alone put it to use.
The result of all this? A painful data discovery process, chaos, wasted time and resources, frustrations, and increased time to unlock business value. Something had to change.
A Guide to Building a Business Case for a Data Catalog
Download free ebook
3 Innovations to Lexikon: How Spotify iterated and improved their data discovery solution #
Spotify released Lexikon in early 2017. The first version allowed users to search and browse datasets, as well as discover knowledge generated through past research and analysis. Despite this effort, data scientists still reported data discovery as being a major pain point. To address this weakness in Lexikon, Spotify improved its data discovery platform using three innovative strategies.
- Understanding user intent
- Enabling knowledge exchange across data teams and people
- Helping users get started with a dataset they found
Understanding user intent #
Understanding user intent is important when designing any product. Hours of research were invested to understand typical use cases of Lexikon – how Spotify data scientists were searching the tool and what information they were looking for or might find useful. Research indicated that users were usually looking for low-intent data (think broad, discovery mode), or for high-intent data (think specific, relevant searches). Using these insights, they were able to redesign their product to facilitate these modes of searching.
Enabling knowledge exchange across data teams and people #
In the first version of Lexikon, Spotify added more metadata and descriptions to datasets. The goal was to reduce the need for person-to-person knowledge sharing. While this aided in data discovery, research showed that data scientists still valued connecting with one another to find datasets and learn how to use them.
Spotify embraced this finding by making it easier to share knowledge and filter datasets based on specific teams and data members.
Helping users get started with a dataset they found #
Once a dataset was found, it was still challenging to put it to use. Datasets often had hundreds of fields and it was not apparent which ones were most useful. Data scientists wanted to see common ways to query the table, most popular fields used, and which tables the datasets were commonly joined with. Spotify improved Lexikon to facilitate these use cases, making it as simple as possible to get started with a dataset as soon as it was found.
Data Catalog 3.0: The Modern Data Stack, Active Metadata, and DataOps
Download ebook
Lexikon Use Cases - Tactical Deep Dive #
What are some use cases of Lexikon within Spotify? If you enjoy getting tactical and want to look at real strategies you can implement within your own data teams, then follow along.
Enabling Low Intent Data Discovery #
Low intent data discovery is a mode of searching for users that have a broad set of goals and may not know what they’re looking for exactly. With initial testing of Lexikon, Spotify found this mode of discovery particularly useful for new employees or people starting on new teams or projects, as they had little familiarity with existing datasets.
To enable this mode of discovery for Lexikon users, Spotify created personalized dataset recommendations. Data scientists were shown algorithmically-generated datasets based on what they might need or find useful. Common applications of low intent data discovery include:
- Finding popular datasets across the company
- Finding datasets relevant to your team or project
- Finding datasets you haven’t used before, but you may find useful
- Recently used datasets
Lexikon intelligently suggests data sources that are commonly used by teams across Spotify, hence, resulting in improved data discovery efficiency. Source: Spotify Engineering
The result of this? 20% of data scientists at Spotify reported using personalized recommendations instead of relying on the search functionality. What a fantastic win!
Enabling High Intent Data Discovery #
High intent data discovery is ideal when you know what you’re looking for or have specific query filters or restraints. This can be useful for tenured data scientists who are more familiar with datasets and have a good idea of what they’re looking for. In the newer version of Lexikon, Spotify enabled the following capabilities to enable high intent data discovery:
- Finding datasets by name
- Finding datasets with a specific field
- Finding datasets related to a specific topic
- Finding datasets owned by a team
- Finding datasets used by specific colleagues
Drilldown and search data assets by data sources, teams that own the data, and by specific topics. Source: Spotify Engineering
In addition to adding the above search capabilities to Lexikon, Spotify also improved the tools’s search ranking algorithms. While there were tens of thousands of datasets, research showed that the majority of consumption was related to a few datasets. Data scientists with high intent were usually searching for one of these popular datasets. As such, Spotify modified Lexikon’s ranking algorithm to weigh results more heavily based on dataset popularity.
Following these changes, data scientists reported search results being more relevant to their queries and it was easier to find popular datasets. On top of that, 44% of Lexikon’s active monthly users reported using high intent searches for data discovery.
Mapping Expertise Within The Data Community #
Data scientists that struggled to find the datasets they were looking for often approached other data experts in the community for help. Sometimes however, it was difficult to identify who to approach regarding a specific topic or dataset. This was commonly the case for new employees who had little familiarity with datasets and had not built connections with fellow colleagues.
To address this limitation in Lexikon, Spotify created a feature allowing users to search for data team members using specific keywords. The search results would also show an individual’s relationship to the data such as someone who owns/queries the data, someone who views/owns dashboards, or someone that runs test experiments on the data for example.
Lexikon allows you to find experts, users, and owners of a data asset. Source: Spotify Engineering
Creating a Slackbot to Facilitate Data Discovery #
Data scientists frequently talked about datasets over slack. To support this communication channel, Spotify built a Lexikon Slackbot that improved chat discussions over Slack. When a user shared a link to a dataset, the slackbot automatically displayed helpful metadata such as the dataset’s name, owner, description, usage stats, most commonly used fields, as well as links to view more information through Lexikon directly.
While this strategy provided value in the moment of the discussion, it also raised awareness and increased the adoption of Lexikon at Spotify. The result of this was a 25% increase in datasets being shared over Slack as well as increased usage of Lexikon.
Displaying and Ranking Schema Field Stats #
Datasets typically had hundreds of schema fields. Which fields should you use once you found the desired dataset? To aid in this last mile of data discovery, Spotify added features to display consumption stats at the schema level. For a given dataset, data scientists could see the total number of times a field was used in a query as well as the number of unique people that used a field in their queries. Data scientists could then sort a dataset’s fields by popularity allowing them to find commonly used or potentially useful fields for their data discovery.
Lexikon surfaces consumption statistics for a data asset — total queries that use this data, and the number of users who use the data asset. Source: Spotify Engineering
Demonstrating Sample Queries #
Once a dataset was found, how could it be easily used? In the first instance of Lexikon, Spotify required data owners to submit sample queries to give data scientists an idea of how to use the datasets. This posed two problems however. First, it was cumbersome to have data producers share sample queries for all their datasets (there are tens of thousands). Second, queries could easily become outdated and it was impractical to continuously monitor and update them.
To address these issues, Spotify created a feature that allowed users to search/view all recent queries made on a dataset. That way, data scientists could see up-to-date queries and also filter results such that only queries with a specific schema field are shown for example. 25% of users that visited a dataset page reported using the queries feature and finding it valuable.
Lexikon surfaces commonly used SQL queries related to a data asset. Source: Spotify Engineering
Tables Commonly Joined #
It is often unlikely the case that a dataset alone will contain all the information a data scientist needs. In most cases, the dataset needs to be joined with another dataset to uncover actionable insights. In the updated version of Lexikon, Spotify developed a feature which listed all tables that were commonly joined with a given dataset. This feature was used by 15% of Lexikon users.
Lexikon surfaces all the joins between the tables, this helps track the relationships and dependencies between various data assets. Source: Spotify Engineering
Is Lexikon open source? #
You might be tempted to get your hands on Lexikon after reading the use cases above. At the moment, Lexikon is not open-source, but this may be an idea that Spotify entertains in the future. For updates on Lexikon, Spotify has an engineering blog you can subscribe to, or you may want to read Spotify’s original post about Lexikon.
Lexikon: Accomplishments and Results #
You might be wondering if the improved version of Lexikon helped Spotify? What were the results exactly? Below are key accomplishments Spotify achieved once they focused on user intent, enabled knowledge sharing, and facilitated the use of datasets.
- Adoption of Lexikon at Spotify increased from 75% to 95% among all data scientists. This made it one of the 5 most utilized tools by data scientists. More people reported using Lexikon at Spotify vs Python, BigQuery or Tableau!
- Lexikon’s user base grew organically from 550 to 870+ monthly active users.
- Data scientists used Lexikon at Spotify more frequently. Users reported using the tool an average of 9 times per month vs 3 times per month when the tool was initially launched.
Quite fantastic results, right? Perhaps the most notable achievement however, was that data scientists no longer identified data discovery as a major pain point in their work.
If you feel inspired by these results, then there’s no reason to feel left out. Enabling data discovery is at the heart of what we do at Atlan. Explore our data discovery solutions and see how we can remove barriers and accelerate performance for your data teams.
Are you looking to implement a data discovery and data catalog solution for your organization — you might want to check out Atlan.
A demo of Atlan for data discovery
Spotify Lexikon: Related Resources #
- Evaluating a data catalog? Here are the 5 essential features to look for in a modern data catalog
- What are the benefits of a data catalog? 5 key reasons why you need one
- What Is a Data Catalog? & Do You Need One?
- Best Alation Alternative: 5 Reasons Why Customers Choose Atlan
- Data catalogs are going through a paradigm shift! Here is everything you need to know about the Third-Generation Data Catalog.
- Learn more about Atlan: The pioneering third-generation data catalog for modern data teams.
Share this article