Apache Atlas: Origins, Architecture, Capabilities, Installation, Alternatives & Comparison
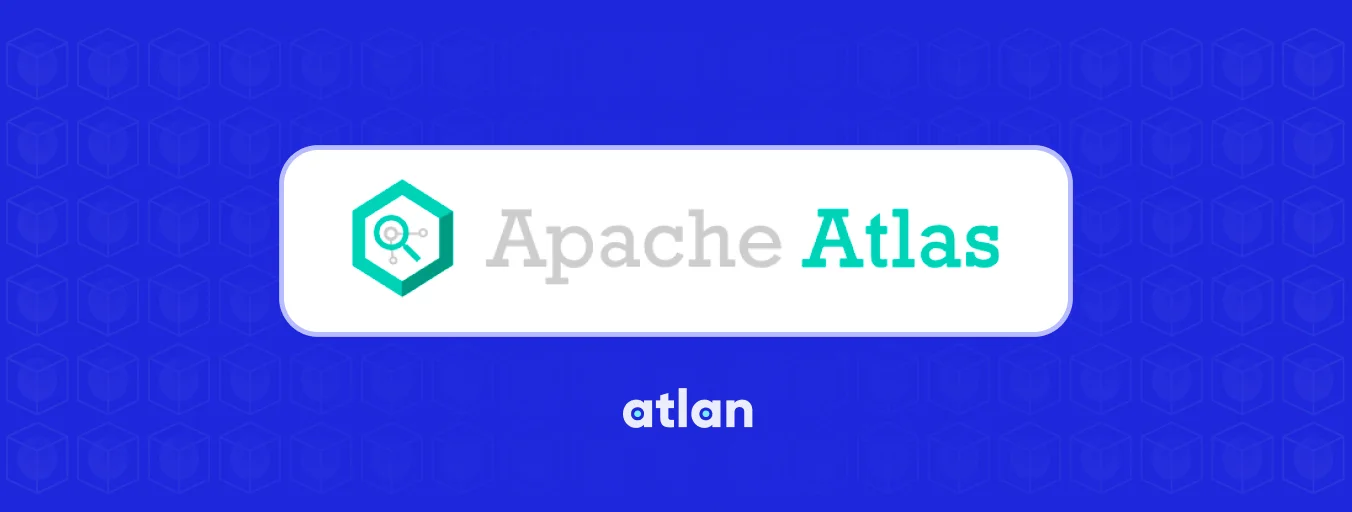
Share this article
Apache Atlas is an open-source metadata and big data governance framework which helps data scientists, engineers, and analysts catalog, classify, govern and collaborate on their data assets.
What is Apache Atlas? #
By representing metadata as types and entities, Apache Atlas provides metadata management and governance capabilities for organizations to build, categorize, and govern their data assets on Hadoop clusters.
These “entities” are instances of metadata types that store details about metadata objects and their interlinkages.
Are you interested in Apache Atlas as a metadata management framework? Then you should get familiar with Active Metadata. Active Metadata is becoming increasingly popular because it helps data teams save time and money. Here is a basic introduction to the concept, as well as how to get started using it. Download our Free Report for more information.
“In Atlas, Type is the definition of metadata object, and Entity is an instance of metadata object,” ~ IBM Developer
Apache offers a state-of-the-art “atlas-modeling” service to help you outline the origins of your data, in tandem with all of its transformations and artifacts. This service takes away the hassle of managing metadata by utilizing labels and classifications to add metadata to the entities. Although anyone can create and assign labels to entities, classifications are under the control of system administrators through the action of Atlas policies.
Atlas Labels and Classifications. Source: Cloudera.
The best of Atlas, without the pain of deployment & maintenance.
Try Atlan's Product Tour
Apache Atlas Origins #
Apache Atlas was initially incubated by Hortonworks in 2014 as the Data Governance Initiative (DGI). This initiative aimed to implement comprehensive data governance practices at enterprises.
Five months later, the endeavor was officially handed over to the Apache Foundation as an open-source project, after which it continuously proved itself as a top-tier project till its graduation in mid-2017.
Since 2015, the project has been maintained by the community for the community, and currently, version 2.2 is in operation.
From DGI to Apache Atlas: A Timeline. Source: Hadoop Summit.
Delhivery’s learnings from implementing Apache Atlas and Amundsen
Download Case Study
What is Apache Atlas used for? #
Apache Atlas is used for the following 5 broad use cases:
- Exerting control over data across your data ecosystem
- Mapping out lineage relationships via metadata
- Providing metadata “bridges”
- Creating and maintaining business ontologies
- Data masking
Let us look into each of the above use cases in detail:
1. Exerting complete control over data #
The data charting ability that the Apache Atlas architecture provides to businesses helps both blue chips and startups to navigate their data ecosystems. It helps in mapping and organizing metadata representations, allowing you to stay attuned to your operational and analytical data usage.
“Apache Atlas is designed to exchange metadata with other tools and processes within and outside of the Hadoop stack, thereby enabling platform-agnostic governance controls that effectively address compliance requirements.”
~ Hortonworks Data Platform: Data Governance
Defining a Complex Process Evolution on Apache Atlas. Source: Hashmap.
2. Mapping out lineage relationships via metadata #
By assigning business metadata, Atlas promotes the genesis of entities that help you devise business vocabulary to keep track of your data assets. More importantly, a lineage map is generated automatically once query information is received. The query itself is noted and its inputs and outputs are used to visualize how and when data transformations took place. Consequently, you can follow the changes and envisage impacts.
Mental Model of a Metadata Structure on Apache Atlas. Source: Marcelo Costam.
3. Providing metadata “bridges” #
Atlas also enables the collection of metadata to be automated through the use of “bridges,” whereby this information could be imported from different data assets in a given source through the use of APIs.
Apache Atlas Providing a Metadata Bridge to the Hortonworks Data Platform. Source: Apache Atlas.
4. Creating and maintaining business ontologies #
By managing classifications and labels, Apache Atlas helps you empower your metadata. Its dashboard aids in annotating the labeled entities, thereby creating an infrastructure specific to your use case and business ontology. The classifications themselves are arranged in a hierarchy and the addition of a single term generates a report of all the entities associated with the query.
Apache Atlas Dashboard. Source: Cloudera.
5. Data masking #
Once data has been organized into an inventory, a data catalog is formed. Based on the classifications that act as the backbone of any given data catalog, Apache Atlas helps mask data access once integrated with Apache Ranger. This feature is critical for securing access to operations and entity instances.
Creation of a Personally Identifiable Information tag for Data Masking. Source: Cloudera
Apache Atlas architecture #
The Apache Atlas architecture is divided into 4 main parts, and it is the reason behind both its popularity and capability. These include:
- Metadata sources & Integration: The categories used in Apache Kafka to organize messages, called Kafka message topics [Integration], typically receive metadata through Atlas add-ons [Metadata Sources].
- Core: Atlas, therefore, has to read each message, which is subsequently stored in JanusGraph [Core]. In turn, JanusGraph is used to visualize the relationships between entities, and the datastore utilized in this case is HBase. A search index by the name of Solr is also employed to reap the benefit of its search functionalities.
- Apps: All of these components allow Atlas to manage metadata, which is eventually used by various governance-oriented use cases [Applications].
The Architecture of Apache Atlas. Source: Apache Atlas.
All the power of open source, with none of the hassle.
Ask Atlan Demo
Capabilities of Apache Atlas #
As a framework for metadata and governance, the Apache Atlas architecture imbues the following capabilities:
- Defining metadata via a Type and Entity system
- Storage of metadata through a Graph repository (JanusGraph)
- Apache Solr search proficiency
- Apache Kafka notification service
- Querying and populating metadata via APIs (Rest API)
1. Defining metadata via a Type and Entity system #
One of the main modules of the tool is the Type System, whose action takes inspiration from how OOP (object-oriented programming) employs instances (i.e., entities) and classes (i.e., types). In the form of Apache Atlas data catalog, we obtain an easy and intuitive tool that models various “types” and then stores information about them as “entities” (instances). Such systemization allows users to address many of the challenges associated with data governance today via the classification and use of data catalogs.
Apache Atlas Types and Entities. Source: Cloudera.
2. Storage of metadata through a Graph repository (JanusGraph) #
After data is extracted by Atlas’ ingesting and export system, the information is discovered and indexed through the Graph Engine Module. Powered by the open-source graph database called Janus, this module enables Apache Atlas to not only highlight interlinkages between various entities in the data catalog but also locate the meta-information of entities according to information sources, which would be stored in a column-oriented database called HBase. Apache Atlas can thus easily and resiliently handle a plethora of very large tables with sparse data.
Vertex (Types) and Edges (Relationships) in a JanusGraph. Source: IBM.
3. Apache Solr search proficiency #
The Solr indexing technology (i.e., an indexing-oriented database) is used by Atlas to boost search proficiency as it facilitates discovering actions. With the help of three collections (i.e., full-text index, edge index and vertex index), users can search for data on the Atlas UI efficiently. It acts as a full-text search engine that is user-friendly and flexible.
Key Elements of the Solr Search Process. Source: Apache Solr.
4. Apache Kafka notification service #
Another notable feature of the Apache Atlas architecture is how real-time data imports and exports can be integrated with various catalogs through Kafka. The Apache Kafka notification service permits messages to be pushed as a single Kafka topic, enabling integration with other data governance tools and the reading of permissions, together with real-time change notifications.
Kafka - A Message Queue Software on Steroids. Source: Sipios.
5. Querying and populating metadata via APIs (Rest API) #
The HTTP Rest API is the primary method for integrating with Apache Atlas. In addition to the four main storage functions (i.e., creating, reading, updating, and deletion), Apache also offers advanced exploration and querying as it exposes a multitude of REST endpoints to work with types, entities, lineage, and data discovery.
Kafka - Atlas Populating Metadata across Multiple Clusters. Source: Sipios.
Built on open source, with Open APIs driving every action. Tour Atlan’s data catalog.
Access Guided Tour
How to install Apache Atlas? #
The entire process to set up Apache Atlas can be broken down into five simple steps.
Step 1: Understanding the Prerequisites #
A cloud VM running Docker and Docker Compose, relevant GitHub repositories, Docker images, and Maven would be required to successfully initiate the installation sequence.
Step 2: Cloning the Repository #
After accessing the root of the cloned repository, the docker-compose up command is used to begin the setup procedure.
Step 3: Executing Docker Compose #
A Docker Compose would trigger a Maven build of Atlas only after the Docker images are extracted from Hive, Hadoop, and Kafka. Thereafter, the Apache Atlas Server is installed.
Step 4: Loading Metadata #
After logging into the admin UI of Atlas, following a successful status verification, the metadata is populated.
Step 5: Navigating the UI #
Loading of the metadata allows you to access entities, formulate classifications, and determine business-specific vocabularies for data contextualization.
For a more in-depth guideline: check out this step-by-step installation guide.
Apache Atlas alternatives #
Although Atlas is a popular data cataloging software backed by an active open-source community, a few noteworthy competitors, such as Lyft’s Amundsen, LinkedIn’s DataHub, and Netflix’s Metacat, deserve to be mentioned.
GitHub Star History of the Top Contenders. Source: Twitter.
Lyft’s Amundsen : Similar to Atlas, Amundsen has also enjoyed high adoption due to its simple text searches, ease of context sharing, and data usage facilities.
LinkedIn’s DataHub : Started in 2016, DataHub represents LinkedIn’s second attempt to solve its cataloging problem through contextual understanding, automated metadata ingestion, and simplified data asset browsing.
Netflix’s Metacat: Metacat ensures interoperability and data discovery at Netflix via change notifications, defined metadata storage, and seamless aggregation and evaluation of data from diverse sources.
A more detailed description of each tool can be found here.
Apache Atlas vs Amundsen #
The capabilities of Amundsen in relation to Apache Atlas are frequently asked.
Taking this opportunity, we would like to highlight that Amundsen focuses on supporting multiple backend environments, ensuring ease of use, and providing sophisticated previews for better data contextualization.
Whereas Atlas prioritizes bestowing upon its users greater control over their data while enabling them to employ glossaries to add business-specific contextual information.
Amundsen vs. Atlas. Image by Atlan.
Click here for a comprehensive comparison between Amundsen and Atlas.
Conclusion #
The greater the control of a company over its data, the better its data governance. Apache Atlas was designed to address this need and concentrates its efforts and architecture to formulate a tool that allows data practitioners to do just that.
Still, deploying such a solution is an energy- and skill-intensive process. Be prepared to go all in once a team of battle-hardened and trustworthy engineers is by your side.
If you’re in the build vs buy mind frame, it’s worth taking a look at off-the-shelf alternatives like Atlan. What you’ll get is a ready-to-use tool from the get-go, that is built for drawing the most out of open-source systems like Apache Atlas.
A Demo of Atlan Data Catalog Use Cases
Apache Atlas: Related Resources #
- A comprehensive guide to help install and set up Apache Atlas.
- Popular open source data catalog platforms to consider in 2024
- Apache Atlas Alternatives: Amundsen, DataHub, Metacat, and Databook
- Amundsen Vs Atlas: A deep dive into how both the open source data discovery tools compare and contrast.
- Understanding AWS Glue data catalog: Use cases, benefits, and more
- How do you evaluate a data catalog for a modern data stack: 5 key considerations and an evaluation guide to help you out.
Share this article