7 Reasons Why SLAs Are Crucial for Data Pipeline in 2024
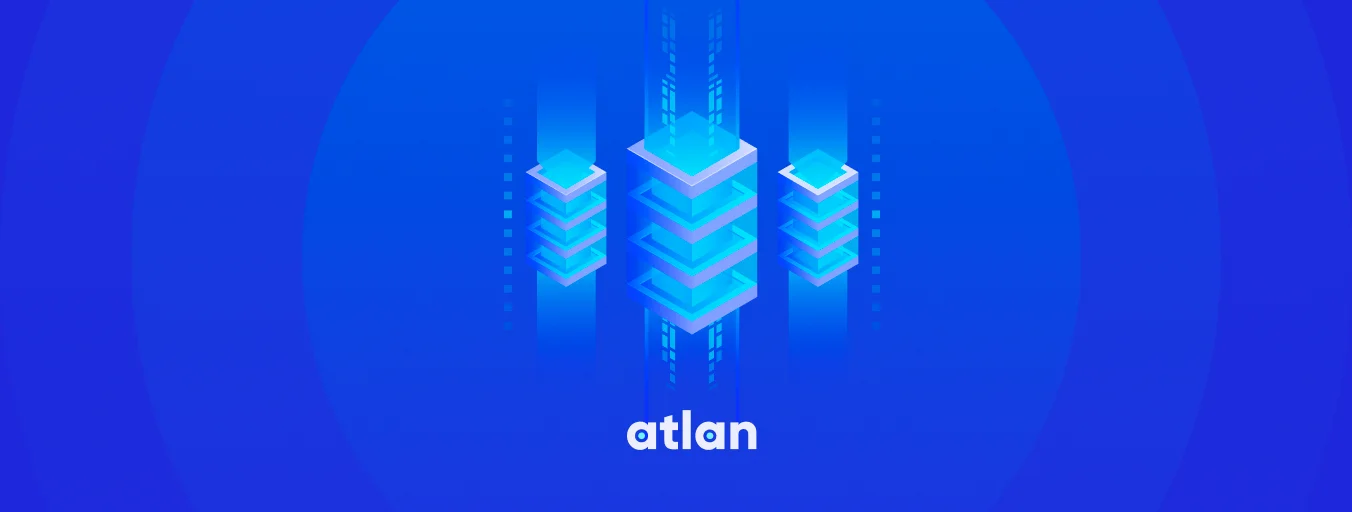
Share this article
In the world of data processing and analytics, ensuring timely and reliable data delivery is of paramount importance. Service level agreements (SLAs) have emerged as a pivotal tool to set clear expectations and standards in this realm.
When it comes to data pipelines, service level agreements ensure that data flows efficiently, timely, and reliably, meeting the needs and expectations of both providers and consumers.
Modern data problems require modern solutions - Try Atlan, the data catalog of choice for forward-looking data teams! 👉 Book your demo today
This article delves into the intricacies of service level agreement specifically tailored for data pipelines. From understanding its basic definition, to the challenges in its implementation and best practices to consider, this comprehensive overview will equip you with everything you need to know about data pipeline SLAs.
Lets dive in!
Table of contents
- What is SLA?
- What is SLA in data pipeline?
- 7 Needs for SLA in data pipelines
- 11 Key metrics to include in a data pipeline SLA
- Monitoring and enforcing data pipeline SLAs
- 10 Challenges in implementing data pipeline SLAs
- 12 Best practices for drafting data pipeline SLAs
- Summary
- Related reads
What is SLA? #
SLA stands for “Service Level Agreement.” It is a contract between a service provider and its customers or clients that specifies the level of service expected. The service level agreement outlines the metrics by which the service is measured, as well as remedies or penalties should agreed-upon service levels not be achieved. It’s commonly used in many industries to define the standard of performance in terms of response time, uptime, and other service-related metrics.
Often, service level agreements serve as a foundational element in building trust between parties, ensuring that expectations are clear and mutual. They provide a framework for handling discrepancies and foster a proactive approach to service delivery and issue resolution.
What is SLA in data pipeline? #
In the context of data pipelines, an service level agreement typically refers to the expected time frame or performance standard within which a particular data processing task or set of tasks should be completed. Data pipelines move and process data from one system to another, and ensuring timely and accurate processing is critical for many business operations.
For instance, if a company relies on a daily report generated from data processed through a pipeline, the service level agreement might state that the data should be processed and ready by 8:00 AM every day. If the pipeline fails to deliver the data by this time, it’s considered a breach of the service level agreement.
The agreement may then outline the steps to be taken, which could be anything from sending notifications to stakeholders, to applying penalties on the service provider, depending on the nature of the agreement. In essence, the service level agreement in data pipelines ensures data reliability and timeliness, which are essential for maintaining smooth business operations.
7 Needs for SLA in data pipelines #
The need for service level agreements in data pipelines stems from the critical role data plays in modern business operations. Here’s why service level agreements are essential for data pipelines:
- Timeliness of business decisions
- Consistency and reliability
- Accountability
- Resource optimization
- Clear expectations
- Enhanced collaboration
- Risk mitigation
Lets understand each in detail.
1. Timeliness of business decisions #
Many business processes rely on timely data for decision-making. For instance, daily sales data might need processing every night to restock inventory for the next day. An service level agreement ensures that data is available when needed.
Ensuring data is punctually processed and accessible not only bolsters operational efficiency but also gives businesses a competitive edge.
In an era where decisions are made in real-time, a robust service level agreement acts as a safeguard against potential delays, keeping the wheels of commerce turning seamlessly.
2. Consistency and reliability #
Service level agreements provide a measurable benchmark for data pipeline performance. This ensures a consistent and reliable flow of data, which is crucial for maintaining trust in data-driven processes.
By setting clear expectations for data delivery and quality, service level agreements bolster confidence among stakeholders. This assurance empowers teams to make informed decisions without second-guessing the data they rely on.
3. Accountability #
If there’s a breach in the agreed-upon service level agreement, the responsible party (often the service provider) is held accountable. This might involve rectifying the issue, offering compensation, or both.
This level of responsibility strengthens trust between businesses and their service providers. Furthermore, it underscores the importance of maintaining high standards in data processing and delivery.
4. Resource optimization #
Knowing the expected processing time for data allows businesses to optimize resources. For example, if a report is expected by 9 AM, resources can be allocated accordingly.
This proactive approach not only maximizes efficiency but also reduces operational costs by preventing resource wastage. Ensuring resources are used judiciously based on data timelines can greatly improve overall business productivity.
5. Clear expectations #
Service level agreements establish clear performance expectations, which aids in designing precise monitoring and alert frameworks. By having these guidelines, teams can proactively address issues, ensuring data consistency and quality.
This clarity not only bolsters operational efficiency but also fosters trust among stakeholders that the data they rely on is dependable and up-to-date.
6. Enhanced collaboration #
When multiple teams or departments are involved in a data process, an SLA ensures everyone is aligned in terms of expectations and responsibilities.
This unified approach fosters better communication and teamwork, reducing potential friction points and misunderstandings that can arise from differing expectations.
With a clear service level agreement in place, teams can seamlessly work together, prioritizing their tasks and sharing the common goal of maintaining data pipeline performance.
7. Risk mitigation #
In case of a dispute, a well-defined service level agreement provides clarity on agreed-upon standards and remedies. This can help in resolving conflicts and potentially avoid costly litigation.
Furthermore, service level agreements instill a sense of trust and predictability between parties, ensuring smoother operations and collaborations.
They also act as a proactive measure, highlighting potential issues before they escalate, allowing for timely interventions.
In summary, service level agreements in data pipelines provide a framework for ensuring that data-driven processes run smoothly and efficiently, with clear standards and accountability mechanisms in place.
11 Key metrics to include in a data pipeline SLA #
When crafting an service level agreement for a data pipeline, it’s crucial to define key metrics that capture the essence of the performance and reliability you expect from the pipeline. Here are some of the vital metrics to consider:
- Latency
- Throughput
- Uptime/availability
- Data accuracy
- Error rate
- Recovery time objective (RTO)
- Recovery point objective (RPO)
- Order preservation
- Volume scalability
- Notification time
- Resolution time
Lets see the metrics in detail.
1. Latency #
Latency can significantly impact user experience and business decisions, especially in applications where immediate data insights are crucial.
A lower latency ensures smoother data flow, allowing businesses to respond more promptly to emerging trends and challenges. How long it takes for data to pass through the pipeline from the point of entry to its endpoint.
This metric is particularly important for real-time or near-real-time data processing.
2. Throughput #
Throughput is a critical metric to gauge the efficiency and capability of a data pipeline.
A higher throughput indicates a system’s robustness in managing large data loads swiftly. Evaluating throughput can assist businesses in optimizing processes and ensuring seamless data flow, especially during peak operational periods.
3. Uptime/availability #
Uptime is a critical metric as it directly impacts the reliability and efficiency of data-driven operations. Prolonged downtimes can lead to missed insights, delayed decision-making, and potential revenue losses.
Therefore, ensuring a high uptime percentage is essential for businesses that rely on timely and consistent data processing. The percentage of time the pipeline is operational and available for data processing. For instance, a 99.9% uptime indicates the system is down only 0.1% of the time.
4. Data accuracy #
Measures the correctness of data after processing. It’s crucial to ensure that data is not corrupted, lost, or misinterpreted during the pipeline’s processing.
Ensuring data accuracy is foundational to building trust in any data-driven decision-making process.
Even minor inaccuracies can lead to significant misinterpretations, potentially affecting business outcomes. By emphasizing data accuracy in a pipeline, organizations lay the groundwork for reliable insights and confident actions.
5. Error rate #
The percentage or number of errors encountered for every set number of data transactions or units. This could include data parsing errors, transformation errors, or any other type of processing error.
A high error rate can significantly impact decision-making, as it might lead to inaccurate data insights.
Monitoring and minimizing the error rate ensures the integrity and reliability of data outcomes. Therefore, managing this metric is crucial for businesses that rely heavily on data-driven strategies and actions.
6. Recovery time objective (RTO) #
The targeted duration of time and service level within which a business process must be restored after a disruption to avoid unacceptable consequences.
Essentially, recovery time objective is the maximum tolerable downtime that an organization can endure without significant harm.
It acts as a benchmark for business continuity planning and helps prioritize recovery strategies. By understanding and setting the recovery time objective, businesses can effectively gauge the urgency and resources needed for swift system restoration.
7. Recovery point objective (RPO) #
The maximum age of files that require recovery from backup storage for normal operations to resume if a data disruption occurs. This metric is crucial for businesses to determine how much data they can afford to lose in unforeseen events.
Recovery point objective values help organizations gauge their data backup frequencies, ensuring that vital information remains protected. By setting a clear recovery point objective , companies can strategically align their backup strategies with business objectives and minimize potential operational hiccups.
8. Order preservation #
Ensures that the sequence of data remains consistent from the source to the destination. This is particularly important for time-series data or any use case where the order of data matters.
Maintaining the sequence integrity ensures that insights derived from the data are accurate and relevant.
A break in this consistency could lead to misguided decisions or misinterpretation of trends. Hence, preserving the order is paramount to the overall integrity and reliability of the data pipeline.
9. Volume scalability #
The pipeline’s ability to handle increased data volumes, often measured as a percentage. Volume scalability ensures that as your data grows, the pipeline won’t become a bottleneck or compromise on efficiency.
This adaptability is crucial in today’s ever-evolving digital landscape where data influx can be unpredictable.
Being able to scale seamlessly means that businesses can continue to derive insights and make decisions without delay, even during peak data periods.
10. Notification time #
Notification time is a pivotal metric in ensuring prompt response and action, particularly in mission-critical applications.
By setting clear benchmarks for this metric, organizations can foster accountability, drive rapid problem resolution, and minimize potential impacts on end-users or dependent systems.
A swift notification process empowers teams to be agile, proactive, and more efficient in managing data pipeline hiccups.
11. Resolution time #
The average time taken to resolve a pipeline issue once it’s identified. Resolution time not only reflects the efficiency of the support and technical teams but also impacts user trust and satisfaction. A shorter resolution time ensures that data-driven processes experience minimal disruption, allowing businesses to make decisions without delay.
In the dynamic world of data, where timely insights are invaluable, consistently achieving a swift resolution time demonstrates commitment to service excellence and operational integrity.
Including these key metrics in a data pipeline service level agreement provides a comprehensive understanding of the performance and expectations associated with the pipeline’s operation. This ensures both the service provider and the users are aligned and can trust the system to support their data-driven initiatives effectively.
Monitoring and enforcing data pipeline SLAs #
Monitoring and enforcing service level agreements (SLAs) in data pipelines ensure that data flows reliably and meets the agreed-upon standards set between the data provider and the data consumer. Here’s a detailed breakdown:
- Monitoring
- Enforcing
Lets understand monitoring and enforcing in data pipeline service level agreement in detail.
1. Monitoring #
- Tools and Software: Use specialized tools to keep a watchful eye on the health, performance, and reliability of the data pipeline. These tools can track metrics, send alerts for anomalies, and generate reports.
- Regular Audits: Conduct systematic reviews of the data pipeline to confirm compliance with the service level agreement. This involves checking the timeliness, accuracy, and reliability of data movement and processing.
- Dashboard Visualization: Implement real-time dashboards that visually represent the state of the pipeline, making it easier to identify issues or performance dips.
2. Enforcing #
- Alerts: Set up automatic notifications that trigger when a metric falls below the agreed-upon standard, indicating a potential service level agreement breach.
- Escalation Procedures: Create clear protocols to escalate any service level agreement breaches to the right team or individual, ensuring swift resolution.
- Penalty Clauses: In some service level agreements, there might be clauses that stipulate penalties or consequences if the service doesn’t meet the agreed-upon standards. These can serve as a deterrent and ensure that the service provider maintains high-quality service.
- Regular Review and Updates: Periodically review the SLA and adjust metrics or terms based on the evolving needs of the business and advancements in technology.
By diligently monitoring and strictly enforcing service level agreements, businesses can maintain trust with stakeholders, ensure consistent data quality, and optimize the performance of data-driven processes.
10 Challenges in implementing data pipeline SLAs #
Implementing service level agreements for data pipelines presents a unique set of challenges, which can arise due to the dynamic nature of data flows, the complexity of modern data architectures, and the varying needs of businesses. Here are some of the notable challenges:
- Complexity of data ecosystems
- Defining clear metrics
- Dynamic data volumes
- Change management
- Alert fatigue
- Technical limitations
- Dependency on external systems
- Ensuring accurate reporting
- Balancing performance and costs
- Evolution of service level agreement metrics
1. Complexity of data ecosystems #
Modern data systems often involve numerous tools, platforms, and technologies. Ensuring service level agreement compliance across such a heterogeneous environment can be daunting.
Navigating the intricate web of integrations requires deep expertise and continuous monitoring.
The interdependencies between these various components can lead to unforeseen challenges. Therefore, a robust strategy and proactive approach are crucial to maintain data integrity and system functionality.
2. Defining clear metrics #
Deciding on what precisely to measure and how to measure it can be challenging. Metrics need to be meaningful, actionable, and relevant to both the data provider and the consumer.
Balancing precision with comprehensibility ensures that metrics resonate with all stakeholders.
Furthermore, consistency in data collection methods guarantees that comparisons over time remain valid. Lastly, timely reviews and adjustments to chosen metrics ensure they evolve with the changing demands of the business landscape.
3. Dynamic data volumes #
Data pipelines can experience varying loads, with spikes in data volume that can unexpectedly stress the system. Ensuring consistent performance during these times can be challenging.
Adapting to these fluctuations requires a robust and scalable infrastructure.
Proactive monitoring and analytics tools are essential for timely response. Balancing system resources and optimizing data flow can help maintain peak efficiency.
4. Change management #
As businesses evolve, their data needs change. Adjusting service level agreements to accommodate new data sources, formats, or processing requirements can be a continuous challenge.
A proactive approach to these shifts ensures operational efficiency and reduces disruptions.
Leveraging insights and feedback from various departments can inform better decisions. Furthermore, regular reviews and audits of data management strategies foster resilience in a rapidly-changing environment.
5. Alert fatigue #
Too many alerts can lead to “alert fatigue,” where teams become desensitized and may miss critical warnings. Striking the right balance between alerting and over-alerting is crucial.
Overwhelming staff with constant notifications can diminish the urgency of genuine threats.
It’s essential to refine and prioritize alerts to ensure the most critical ones are immediately noticeable. An effective alert system focuses on quality over quantity, safeguarding team responsiveness and operational integrity.
6. Technical limitations #
Not all tools and platforms provide the necessary granularity or real-time capabilities for effective service level agreement monitoring. Some may offer superficial insights, lacking in-depth analysis vital for proactive problem-solving.
In addition, integration challenges can arise when trying to synchronize multiple monitoring tools.
Finally, outdated systems or lack of regular updates can hinder the accuracy and relevance of the captured data.
7. Dependency on external systems #
Data pipelines often rely on third-party tools or external data sources. Any disruptions or changes in these can impact the service level agreement compliance of the pipeline.
Furthermore, integration complexities can introduce unforeseen errors or data inconsistencies.
The need for constant monitoring and updates to accommodate these external changes can strain resources. Additionally, the opacity of third-party systems might leave teams in the dark about potential performance bottlenecks or data vulnerabilities.
8. Ensuring accurate reporting #
To maintain trust, it’s essential to provide stakeholders with accurate and transparent reports on service level agreement compliance. This requires robust reporting tools and processes.
Regular audits can help verify the authenticity of data presented.
Streamlined communication channels ensure that discrepancies are addressed promptly. Emphasizing a culture of transparency encourages teams to uphold their commitments and maintain stakeholder confidence.
9. Balancing performance and costs #
Meeting stringent service level agreements might require additional resources, leading to increased costs. Balancing the trade-off between performance and financial implications is crucial.
However, a thoughtful and strategic approach can optimize both. By leveraging efficient tools and methodologies, organizations can achieve high performance without breaking the bank. Ultimately, it’s about smart investments that drive consistent, cost-effective results.
10. Evolution of service level agreement metrics #
As technology and business needs change, the metrics that were once relevant can become outdated, requiring regular review and adjustment of service level agreements.
Additionally, with the rise of digital transformation and cloud computing, there’s a pressing need to ensure service level agreement metrics align with contemporary infrastructural demands.
Customer expectations are also evolving rapidly, necessitating service level agreements that resonate with their current requirements. By proactively adapting these metrics, businesses can foster trust, ensure efficiency, and maintain a competitive edge in the marketplace.
Addressing these challenges requires a combination of the right tools, well-defined processes, and a collaborative approach between data teams and stakeholders.
12 Best practices for drafting data pipeline SLAs #
When drafting service level agreements (SLAs) for data pipelines, it’s important to ensure clarity, precision, and a focus on the user’s needs to guarantee data quality, reliability, and timely delivery. Here are some best practices to consider:
- Clearly define metrics and terms
- Set realistic expectations
- Prioritize data quality
- Monitor performance
- Include flexibility clauses
- Detail issue response and resolution times
- Plan for failovers and redundancies
- Specify maintenance windows
- Establish communication protocols
- Incorporate penalties and rewards
- Confidentiality and data security
- Regularly review and update service level agreements
Lets understand the best practices for drafting data pipelines in detail.
1. Clearly define metrics and terms #
Every service level agreement starts with clear definitions. Define what constitutes data latency, uptime, data completeness, and other critical metrics. Avoid ambiguous language to ensure both parties understand their commitments.
It’s also essential to provide real-world examples or scenarios for context, making the terms more relatable and comprehensible.
Glossaries or appendices can be valuable additions, offering deeper insights into specific terminologies. Regularly revisiting and updating these definitions ensures that they remain relevant as technology and processes evolve.
2. Set realistic expectations #
Ensure that the service level agreement commitments are realistic based on the current technology stack, resources, and operational capabilities. Overpromising can lead to service level agreement breaches and damage trust.
Aligning expectations with capabilities not only fosters a transparent relationship but also streamlines performance management.
It’s crucial to keep stakeholders informed about any potential limitations or constraints. This proactive approach aids in collaborative problem-solving and strengthens partnerships for the long run.
3. Prioritize data quality #
Define metrics around data accuracy, completeness, and consistency. Specify the expected error rates and how data quality issues will be resolved once identified.
Furthermore, highlight the importance of regular data audits to maintain optimal data integrity.
As data becomes increasingly pivotal in decision-making, maintaining its pristine state is paramount. Implementing robust validation checks and routine clean-up procedures will also play a significant role in upholding the highest standards of data quality.
4. Monitor performance #
Use monitoring tools to measure the actual performance against service level agreement commitments. Regular monitoring helps in early identification of potential issues and aids in proactive remediation.
Incorporating real-time alerts can further expedite response times, ensuring that stakeholders are instantly aware of any discrepancies.
Moreover, historical performance data aids in refining the data pipeline processes and optimizing for future throughput. With consistent oversight, businesses can achieve enhanced reliability and foster greater trust with their end-users.
5. Include flexibility clauses #
Data pipelines might need to adapt to changing business and technology landscapes. Include provisions for regular reviews and potential updates to the service level agreement terms.
As the digital ecosystem continues to evolve, it’s crucial that service level agreements remain dynamic and adaptable to maintain service excellence.
Regular reassessment ensures that both parties remain aligned in their expectations and deliverables. By incorporating flexibility, organizations can pivot quickly in response to unforeseen challenges or advancements, ensuring continued efficiency and collaboration.
6. Detail issue response and resolution times #
Clearly define the timeframe within which issues need to be acknowledged and resolved. This helps in setting clear expectations for incident response.
Furthermore, by stipulating clear timelines, it ensures accountability, promotes proactive intervention, and minimizes potential disruptions.
Both parties can then operate with confidence, knowing there’s a structured approach in place for unforeseen challenges. Such transparency not only builds trust but also ensures smooth collaboration between service providers and clients.
7. Plan for failovers and redundancies #
Detail backup plans and redundancy measures in case of pipeline failures. Mention the expected recovery times and recovery point objectives. In an era where data drives decisions, ensuring uninterrupted access is paramount.
The strategy should also encompass periodic testing of these failover mechanisms to validate their effectiveness. By having a robust redundancy plan in place, organizations can safeguard against unforeseen data interruptions and bolster their operational resilience.
8. Specify maintenance windows #
Regular maintenance is essential for the smooth functioning of data pipelines. Specify the scheduled maintenance windows and how clients will be informed of any planned downtime.
Effective communication during these periods can bolster client trust, ensuring they feel valued and in-the-know.
Transparency about the reasons for maintenance can also aid in understanding its importance. It’s imperative to offer alternatives or solutions, such as low-activity time slots, to minimize disruptions to the client’s operations.
9. Establish communication protocols #
Clearly define who should be contacted in case of an service level agreement breach or any other issues. Have an escalation matrix to ensure timely resolution of problems. Additionally, maintain an up-to-date list of stakeholders with their roles and contact information to facilitate swift communication.
Incorporate feedback loops to understand the root causes and prevent future incidents. Lastly, utilize standardized communication channels and tools to ensure transparency and consistent information dissemination across the board.
10. Incorporate penalties and rewards #
Consider including clauses that stipulate penalties for non-compliance and potential rewards for consistently exceeding service level agreement standards. This aligns incentives for the service provider. Such a balanced approach not only ensures accountability but also motivates the service provider to always strive for excellence.
Additionally, this fosters a collaborative environment where both parties work towards a common goal of efficiency and high performance. In the long run, this can enhance the relationship between the client and the service provider, solidifying a partnership built on trust and mutual respect.
11. Confidentiality and data security #
Emphasize the importance of data privacy and security. Define standards and protocols for data encryption, storage, and transmission, ensuring adherence to legal and industry-specific regulations.
In today’s interconnected digital landscape, potential breaches can have far-reaching implications, making a robust data protection strategy paramount.
Regular training and awareness programs should be in place to educate team members on the latest security threats and best practices. Additionally, implementing regular audits and assessments can ensure consistent compliance and readiness against emerging vulnerabilities.
12. Regularly review and update service level agreements #
As with any agreement, service level agreements for data pipelines should be reviewed regularly to account for evolving needs, technological changes, or learnings from past experiences.
Furthermore, periodic assessments ensure that both parties remain aligned in their objectives and expectations.
Incorporating feedback from stakeholders and users can refine and enhance the agreement’s effectiveness. By staying proactive in these revisions, organizations can preemptively address potential challenges and continue to optimize data flow and accessibility.
By following these best practices, organizations can draft service level agreements that are both effective and beneficial for all parties involved, ensuring the smooth and efficient operation of data pipelines.
Summary #
Service level agreements (SLAs) for data pipelines play a pivotal role in ensuring the consistent, efficient, and secure flow of data within an organization. They act as a binding contract, delineating the expectations and responsibilities of both service providers and recipients.
In the dynamic landscape of data management and digital transformation, these service level agreements serve as a beacon, guiding teams towards operational excellence. To maintain their relevance and effectiveness, such agreements must be adaptable and responsive to the evolving needs of technology and business objectives.
By diligently crafting, monitoring, and updating these service level agreements, organizations can safeguard the integrity of their data processes and ensure a seamless data-driven decision-making environment.
Related reads: #
- Data Pipeline Monitoring: A Necessity for Modern Data Teams
- What is Data Governance? Its Importance, Principles & How to Get Started?
- What is Data Driven Decision Making & Why Its Crucial?
- Data Catalog Guide: Examples, What to Look For, and Where They’re Going
- Cloud Data Migration: A 10-Step Ultimate Guide
- Atlan + Airflow: Better Pipeline Monitoring and Data Lineage with Our Newest Integration
Share this article