What is Cloud Data Migration? A Complete Guide for 2024
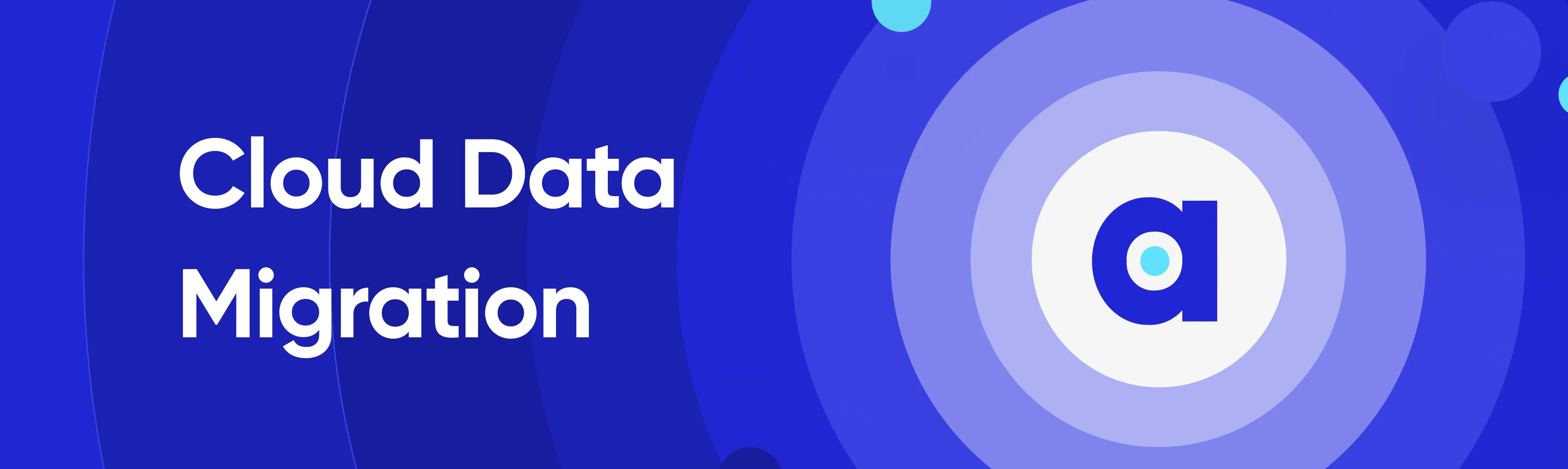
Share this article
Cloud data migration is crucial for organizations because it allows businesses to scale their operations easily by offering unlimited storage and computing resources. This means they can grow without worrying about hardware limitations.
Cloud data migration future-proofs organizations by enabling them to adopt emerging technologies and stay ahead in the market.
Modern data problems require modern solutions - Try Atlan, the data catalog of choice for forward-looking data teams! 👉 Book your demo today
In this article, we will learn the steps of cloud data migration, key challenges, and the major data governance policies essential for it, along with a case study.
So, lets dive in!
Table of contents #
- What is cloud data migration and why is it important?
- 10 Steps for effective cloud data migration
- 8 Challenges to face
- Top 5 tools for 2024
- Cloud data migration strategy: In 10 steps
- Essential data governance policies in cloud data migration
- Cloud data migration: A case study perspective
- Rounding it all up
- Related reads
What is cloud data migration and why is it important? #
Cloud data migration refers to the process of moving data, applications, and other business elements from an organization’s onsite computers to the cloud, or moving them from one cloud environment to another.
This process aims to leverage the cloud’s extensive capabilities to enhance flexibility, scalability, and other vital operational aspects for businesses and organizations.
Importance of cloud data migration #
1. Scalability and flexibility #
- Adaptability: Cloud environments allow businesses to easily scale their IT infrastructure up or down, based on their needs.
- Resource management: Organizations can manage resources more effectively, only paying for the storage and computing power they use.
2. Cost-efficiency #
- CAPEX reduction: By migrating to the cloud, businesses can reduce capital expenditure on physical hardware and infrastructure.
- Operational costs: Organizations often experience lower operational costs due to the pay-as-you-go pricing models offered by cloud providers.
3. Business continuity #
- Disaster recovery: Cloud environments often provide robust disaster recovery capabilities, safeguarding business data against physical or technical failures.
- Data backups: Regular and automated data backups in the cloud ensure that critical business data is always recoverable.
4. Enhanced collaboration #
- Accessibility: Cloud data can be accessed from anywhere with an internet connection, facilitating remote work and global collaboration.
- Real-time collaboration: Multiple users can work on the same datasets and applications in real-time, enhancing teamwork and productivity.
5. Innovation and competitiveness #
- Rapid deployment: Businesses can quickly deploy new applications and services in the cloud, accelerating time-to-market and innovation.
- Technological edge: Employing cloud technologies can provide a competitive edge by enabling companies to adopt the latest technologies without hefty upfront investments.
6. Security and compliance #
- Data protection: Reputable cloud providers invest heavily in security protocols to protect stored data.
- Regulatory compliance: Cloud services often offer solutions to help businesses remain in compliance with regional and industry-specific data protection regulations.
7. Sustainability #
- Energy efficiency: Cloud data centers are typically more energy-efficient than traditional data centers.
- Reduced carbon footprint: By sharing resources in a cloud environment, businesses can reduce their overall energy consumption and carbon footprint.
Navigating through the complexity of migration requires a clear understanding, meticulous planning, and an adept execution strategy to ensure seamless migration, optimal functionality, and organizational growth in the digital landscape.
10 Steps for effective cloud data migration #
Migrating data to the cloud is a major decision for any company, especially those dealing with large volumes of sensitive data such as a financial technology company.
There are 10 steps in cloud migration, which are as follows:
Step 1: Define your strategy
Step 2: Identify and assess your data
Step 3: Choose a cloud provider
Step 4: Define your architecture
Step 5: Choose a migration approach
Step 6: Security and compliance review
Step 7: Perform a migration test
Step 8: Migrate data
Step 9: Validate and optimize
Step 10: Plan for continuous improvement
Now, let us look into each of the above steps in brief.
Step 1: Define your strategy #
Understand your key business drivers for migration.
This could be :
- Cost-efficiency
- Scalability
- Improving data security
- Disaster recovery
- Driving faster insights
Articulating clear goals for migration, aligning various stakeholders, and determining budget and timeline constraints.
Strategic alignment workshops with executives, business, IT, and data teams. SWOT analysis to identify strengths, weaknesses, opportunities, and threats of the migration. Cost-benefit analysis.
Finalized migration strategy with agreed-upon objectives, budget, and timeline.
Step 2: Identify and assess your data #
Assess the data that you need to migrate. This can be challenging when dealing with legacy systems. Tools for data cataloging can help you discover and classify data.
Difficulties in understanding data scattered across various legacy systems, identifying sensitive or regulated data, assessing data quality, and defining data migration priorities.
Data cataloging tools, data quality assessment tools, cross-functional team to analyze the data, existing system documentation, and data dictionary.
Thorough understanding of data landscape with data catalog, identified sensitive data, and prioritized data migration list.
Step 3: Choose a cloud provider #
Evaluate and select a cloud provider that best suits your needs. Consider factors such as cost, services offered, security measures, and compliance standards. The big three are:
- Amazon Web Services (AWS)
- Google Cloud Platform (GCP)
- Microsoft Azure
Difficulties in evaluating cloud providers based on pricing, performance, security, services offered, and compliance with relevant regulations.
RFP (Request for Proposal) documentation, responses from potential cloud vendors, vendor evaluation matrix.
Selection of cloud provider that matches business, security, and compliance needs within budget.
Step 4: Define your architecture #
Define the architecture of your cloud setup. This could be public, private, or hybrid depending on your needs. Also, define how the components will interact with each other and with on-premises systems.
Choosing between speed and resource efficiency (rehosting), moderate change for more benefits (replatforming), or full redesign for maximum benefit but high cost (refactoring).
Cost-benefit analysis of different approaches, insights from similar migration projects, pilot migration projects.
Chosen migration approach that aligns with strategic objectives, resource availability, and risk tolerance.
Step 5: Choose a migration approach #
The approach can vary based on the amount and type of data, downtime tolerances, and migration window. The common approaches are:
Re-hosting (Lift and Shift): This involves moving applications and data to the cloud with little to no modification. This approach is fast and less resource-intensive but doesn’t take full advantage of cloud-native features.
Re-platforming (Lift, Tinker, and Shift): This involves making some cloud-optimized modifications during the migration process to benefit from cloud capabilities without a complete redesign.
Repurchasing: This involves moving to a different product, typically a cloud-based service.
Refactoring/Re-architecting: This involves redesigning the application to fully exploit cloud-native features. This is the most resource-intensive approach but can yield more long-term benefits.
Step 6: Security and compliance review #
Review the security features provided by the cloud service provider. For a fintech company, compliance with regulations like PCI DSS, GDPR, and regional data laws is essential.
Ensuring the chosen cloud provider, architecture, and processes comply with all relevant regulations and provide adequate data protection.
Information on relevant regulations and standards, cybersecurity experts, and third-party auditors.
Compliance audit report, risk assessment report.
Step 7: Perform a migration test #
Before fully migrating, perform a migration test. This allows you to assess the effectiveness of the migration process, estimate the downtime, and troubleshoot any potential problems.
Ensuring the test accurately replicates the final migration and interpreting results to identify potential issues.
Test data sets, data migration tools, test environments, data validation tools.
Successful test migration, documented insights about potential issues and their solutions, accurate estimate of migration time.
Step 8: Migrate data #
After successful testing, plan the migration. This might be a single event or staggered over a period of time, based on the business needs and the downtime acceptable.
Managing downtime, ensuring data integrity during transfer, and dealing with unexpected issues during migration.
Data migration tools, migration team including project managers and engineers, coordinated communication plan.
]Successful migration within agreed downtime, no loss of data integrity, a post-migration report documenting any issues and their resolutions.
Step 9: Validate and optimize #
After the migration, perform validation tests to ensure everything is working as expected. Also, look for optimization opportunities like cost-saving, performance improvement, etc.
Verifying data integrity post-migration, and tuning new systems for optimal performance.
Data validation tools, performance monitoring, and management tools, technical team for system tuning.
Achieving or exceeding initial performance benchmarks, favorable feedback from users, stable system operation with minimal downtime or errors.
Step 10: Plan for continuous improvement #
Data migration is not a one-time process. The business and data landscape will continue to evolve, so there should be a plan in place for continuous improvement and maintenance.
Establishing processes for ongoing optimization and adaptation to evolving business and data needs. Staying up-to-date with cloud technology advancements.
Regular system performance review, user feedback mechanisms, continued learning and training for the team, innovation budget.
Implementation of the improvement plan, evidence of iterative improvements in system performance, team adaptability, and user satisfaction over time.
Additional considerations #
- Team structure: Decentralizing your data team could help reduce bottlenecks. You could consider adopting a DataOps approach, where cross-functional teams collaborate to deliver data, insights, and analytics at speed.
- Data governance: Establish robust data governance procedures to ensure data quality and security. This is especially important for a self-serve analytics environment.
- Training: Ensure your team is trained in new technologies and methodologies. This is important for the success of the migration and the ongoing operation of the new infrastructure.
Remember that every migration is unique and these steps should be adapted based on your business context and technical environment.
Cloud data migration: 8 Challenges to face #
In the process of cloud data migration, there are several areas that organizations often underestimate:
- Data cleanup
- Training and change management
- The complexity of legacy systems
- Security and compliance
- Time and cost overruns
- Post-migration optimization
- Integration with existing systems
- Managing downtime
Let us understand these challenges in detail:
1. Data cleanup #
Prior to cloud data migration, it’s essential to cleanse and organize data. This step ensures that only relevant and accurate data is transferred to the cloud, increasing efficiency and reducing migration costs.
2. Training and change management #
Cloud data migration requires personnel to learn new systems and adapt to new processes. Adequate training and change management strategies are crucial for a smooth transition.
3. The complexity of legacy systems #
Migrating from legacy systems to the cloud can be daunting. These systems are often complex and may not be readily compatible with modern cloud services.
4. Security and compliance #
Maintaining data security and meeting regulatory compliance are paramount during cloud data migration. This involves a thorough understanding of cloud security practices and compliance standards.
5. Time and cost overruns #
Cloud data migration projects can easily exceed initial timeframes and budgets. It’s important to set realistic expectations and continuously monitor migration costs.
6. Post-migration optimization #
After cloud data migration, it’s necessary to optimize the new cloud environment to ensure that it operates efficiently and cost-effectively.
7. Integration with existing systems #
Ensuring that the migrated data works seamlessly with existing systems is a significant challenge. This requires careful planning and testing of integrations.
8. Managing downtime #
Minimizing downtime during cloud data migration is essential to avoid disrupting business operations. This requires meticulous planning and the use of migration tools that can facilitate a swift transition.
Thoroughly considering these often underestimated aspects during the planning stage can make your migration process more predictable and effective.
Cloud data migration: Top 5 tools for 2024 #
These are some of the important cloud data migration tools.
- AppDynamics
- AWS migration services
- AvePoint migration
- Azure migrate
- Carbonite migrate
Let’s understand each of them in details.
1. AppDynamics #
Parameters:
- Real-time performance monitoring: Provides insights into application performance, user experiences, and business outcomes with real-time analytics.
- Anomaly detection: Identifies performance anomalies and pinpoints root causes to mitigate issues before they affect users.
- End-user monitoring: Tracks user journeys to understand the user experience and enhance it post-migration.
Key features:
- Application mapping: Offers visualizations to understand application dependencies and structure, which aids in planning migrations.
- Performance baselining: Establishes performance benchmarks, which helps in comparing pre and post-migration performance.
2. AWS migration services (AWS DMS, Server Migration Service, Migration Hub) #
Parameters:
- Homogeneous & heterogeneous migration: Supports the migration of various database types, including migrating between different database engines.
- Continuous replication: Allows data synchronization between source and target databases during migration.
- Server migration: Assists in migrating on-premises server workloads to the AWS cloud.
Key Features:
- AWS database migration service (DMS): Focuses on easy and secure database transfers with minimal downtime.
- AWS server migration service: Streamlines the migration of on-premise servers to AWS.
- AWS migration hub: Provides a single location to track migration progress across AWS and partner solutions.
3. AvePoint migration #
Parameters:
- Unified platform: Migrates a wide array of data types including files, emails, and collaborative platforms’ data to the cloud.
- Mapping and scheduling: Allows for detailed migration mapping and scheduling to tailor the process as per business needs.
Key Features:
- Detailed reporting: Offers in-depth reports and analytics on migration progress and potential issues.
- Minimal downtime: Ensures data is continually synchronized between source and destination, minimizing migration-related downtime.
- Content analysis: Gains insights into existing content, helping to determine what to migrate.
4. Azure migrate #
Parameters:
- Assessment capabilities: Facilitates in evaluating on-premises workloads for migration to Azure.
- Migrating servers and databases: Aids in migrating servers, applications, and databases with minimized risk and downtime.
Key features:
- Dependency visualization: Illustrates dependencies of applications and workloads, aiding in strategizing migration phases.
- Cost estimation: Provides cost estimates and performance recommendations for running resources in Azure.
- Unified migration platform: Offers tools and services to help migrate servers, databases, web apps, and virtual desktops to Azure.
5. Carbonite migrate #
Parameters:
- Automated migration: Enables automated processes for migrating physical, virtual, and cloud workloads to and from any environment.
- Minimal interruption: Assures virtually no downtime during the migration process.
Key features:
- Continuous replication: Ensures data consistency by continuously replicating changes from the source to the target.
- Flexibility: Supports migration between disparate systems, eliminating vendor lock-in and facilitating transitions between platforms.
- Comprehensive management: Manages the entire migration lifecycle, from planning and testing to execution and validation.
These tools provide various approaches to cloud data migration, offering businesses the capability to choose solutions that align closely with their requirements, scale, and the complexity of their migration project.
Cloud data migration strategy: In 10 steps #
Creating a robust cloud data migration strategy is essential for any organization looking to leverage the advantages of cloud computing. A successful strategy ensures that the transfer of data to the cloud is smooth, secure, and aligned with business goals. These include:
- Understand your data landscape
- Set clear objectives
- Choose the right cloud environment
- Plan for security and compliance
- Design a phased migration approach
- Prepare for the migration
- Test your migration
- Execute the migration
- Monitor and optimize post-migration
- Train your team
Here’s how to craft a comprehensive cloud data migration strategy.
1. Understand your data landscape #
Begin by thoroughly assessing the data you plan to migrate. Understand its structure, sensitivity, and dependencies. This knowledge will inform every other aspect of your cloud data migration strategy.
2. Set clear objectives #
Define what you aim to achieve with cloud data migration. Whether it’s to enhance business agility, reduce costs, or improve data analytics capabilities, your goals should guide the strategy.
3. Choose the right cloud environment #
Decide on a public, private, or hybrid cloud environment based on your specific needs. This choice will impact your cloud data migration strategy, especially concerning security and compliance requirements.
4. Plan for security and compliance #
Develop a plan to ensure that your data is secure and that the migration complies with all relevant regulations. This includes choosing a cloud provider that meets industry standards.
5. Design a phased migration approach #
Consider breaking down the migration into manageable phases. A phased approach allows you to troubleshoot issues as they arise and reduces the risk of business disruption.
6. Prepare for the migration #
This involves setting up the cloud environment, configuring storage and databases, and establishing a backup and recovery process. It’s also the stage to clean and organize your data.
7. Test your migration #
Before going live, conduct thorough testing to ensure data integrity and application performance. Testing should be done in a controlled environment to minimize the impact of any issues.
8. Execute the migration #
Carry out the cloud data migration according to the planned phases. Monitor the migration closely to detect and address any issues quickly.
9. Monitor and optimize post-migration #
After the migration, continue to monitor the cloud environment. This allows you to optimize resources, manage costs, and improve performance.
10.Train your team #
Ensure that your team is well-trained on the new cloud environment. Effective use and management of the cloud are crucial to realizing its benefits.
By following these steps, your cloud data migration strategy will align with your business objectives, ensuring a seamless transition to the cloud.
Cloud data migration: Essential data governance policies #
Data governance is an important aspect of any data management strategy, especially when considering a cloud data migration. It provides an overarching structure to ensure the security, availability, usability, and integrity of data in an organization.
Data governance includes various policies, procedures, and practices. In the context of a self-serve analytics environment and cloud data migration, these include:
- Data quality
- Metadata management
- Data access and security
- Data lifecycle management
- Data integration
- Compliance
Let us understand these policies in detail:
1. Data quality #
This includes standardizing, cleaning, and ensuring the accuracy and completeness of data.
Bad data can lead to incorrect analysis and poor decision-making, so it’s crucial to establish procedures to continuously check and improve data quality.
Tools for data profiling and data cleaning can be used to automate some of these tasks.
2. Metadata management #
Metadata provides context about data, making it easier for users to find and understand data. This is particularly important in a self-serve analytics environment.
Implementing metadata management involves creating a metadata repository and establishing procedures for maintaining it.
3. Data access and security #
Defining who can access what data and under what conditions is crucial for data security. This includes setting up role-based access control, implementing data masking for sensitive data, and establishing audit logs to track data usage.
4. Data lifecycle management #
This involves establishing policies for data archiving, retention, and deletion. This not only helps manage storage costs in the cloud but also ensures compliance with various data protection regulations.
5. Data integration #
Ensuring that data from various sources can be seamlessly integrated is key for a comprehensive analysis. This might involve:
- Establishing data naming standards
- Defining a common data model
- Implementing a data integration tool
6. Compliance #
Depending on your industry, you might need to comply with various data protection regulations, such as GDPR or CCPA. This involves establishing procedures for data privacy, data residency, and data handling practices.
For organizations thinking of cloud data migration, here is some specific guidance around data governance:
a. Involve stakeholders: Start by getting all stakeholders onboard – from executives to data users. Their input can help define clear data governance policies and make implementation smoother.
b. Assess current state: Understand your current data governance maturity level and identify areas that need improvement. This could involve auditing your current data quality, security, and compliance practices.
c. Define governance policies: Based on the assessment, define clear data governance policies. This includes policies around data quality, metadata management, data access, data lifecycle, data integration, and compliance.
d. Implement tools: Consider implementing data governance tools that can help automate some of the tasks. This could include tools for data quality management, metadata management, data cataloging, data lineage, etc.
e. Continual monitoring and improvement: Data governance is not a one-time task but an ongoing process. Continually monitor your data governance practices and look for areas of improvement.
By establishing robust data governance, organizations can ensure that their data is secure, reliable, and ready for use in a self-serve analytics environment. Moreover, it can make the cloud data migration process smoother and more efficient.
Cloud data migration: A case study perspective #
There are many publicly available case studies that might provide insights into the process of cloud data migration and the implementation of data governance:
1. Capital One #
Capital One is one of the most notable examples of successful cloud migration. They migrated most of their workloads to AWS, and they went all-in on the cloud.
They emphasized security as a major consideration in their migration process and created a “Cloud Governance Framework” to ensure that security was embedded in all aspects of their cloud deployment. The framework involved a continuous auditing process and an automated system to ensure compliance.
Here is a link to the AWS case study for more information: Capital One - AWS Case Study
2. Dow Jones #
Dow Jones migrated its data centers to AWS. A notable aspect of their migration was their use of AWS’s IAM (Identity and Access Management) for data governance.
IAM allowed Dow Jones to control user and programmatic access to AWS services and resources, ensuring that only authorized services could access their data.
Here is the AWS case study for Dow Jones: Dow Jones - AWS Case Study
3. Telefónica #
Telefónica is a global telecommunications company that migrated to Google Cloud. They employed a strong governance model, ensuring secure data access, implementing Identity and Access Management (IAM), and using tools for data loss prevention.
Here is a related Google Cloud case study: Telefónica - Google Cloud Case Study
Please note that while these case studies provide an overview of their process, the exact details of their data governance policies and procedures might not be disclosed due to the sensitive nature of the subject.
However, they should give you a good understanding of how large companies have approached cloud migration and data governance.
Rounding it all up #
Cloud data migration is crucial for organizations because it allows businesses to scale their operations easily by offering unlimited storage and computing resources.
The need for robust data governance to ensure data quality, security, access control, lifecycle management, data integration, and compliance in a self-serve analytics environment.
Involving stakeholders, assessing the current state, defining governance policies, implementing data governance tools, and continuously monitoring and improving.
This information should serve as a comprehensive guide to understanding cloud data migration, the importance of data governance, and practical insights from industry case studies.
Each organization’s migration journey will be unique, and these guidelines should be adapted as necessary to meet specific circumstances.
Cloud data migration: Related reads #
- Benefits of Moving from On-Premise to Cloud
- Data Migration: On-Premise to Cloud - 10 Steps to Success
- 8 Essential Strategies to Fast-Track Your Cloud Migration Journey!
- Cloud vs On-Premise vs Hybrid: Choosing the Right Data Management Approach
- Benefits of Data Governance: 4 Ways It Helps Build Great Data Teams
- Cloud Data Warehouses: Cornerstone of the Modern Data Stack
- What is data governance & why does it matter?
- 7 Best Practices for Data Governance to Follow in 2024
- Benefits of Data Governance: 4 Ways It Helps Build Great Data Teams
- Data Governance Policy: Examples, Templates & How to Write One
- Key Objectives of Data Governance: How Should You Think About Them?
- Automated Data Governance: How Does It Help You Manage Access, Security & More at Scale?
- What Is a Data Catalog? & Do You Need One?
Share this article