Stale Data Explained: Your Ultimate Guide in 2024!
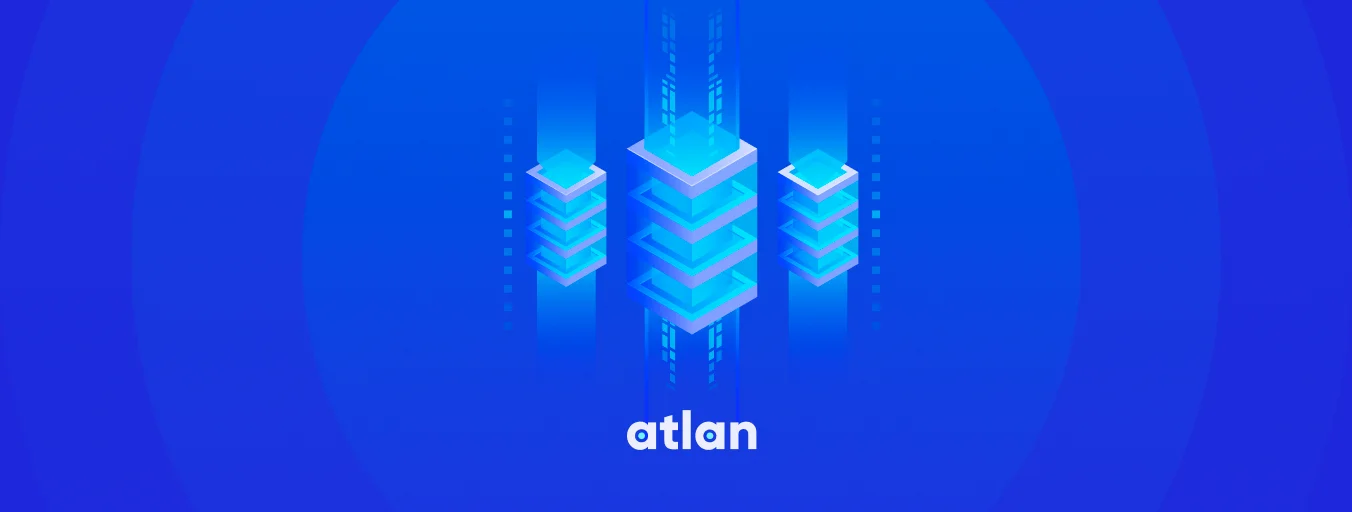
Share this article
Stale data, a silent disruptor in today’s data-driven landscape, has the potential to wreak havoc on decision-making processes and operational efficiency.
Understanding the potential for stale data and the impact it can have is crucial for designing and maintaining reliable and accurate systems.
In this blog, we will delve into the concept of stale data, its far-reaching impacts, and the essential steps organizations can take to mitigate its detrimental effects.
Let’s dive in!
Table of contents
- What is stale data? A quick sneak peek!
- 6 Most popular examples of stale data
- 8 Essential root causes of stale data
- 8 Deadly impacts of stale data you need to know
- How is stale data measured? Here are 8 simple steps!
- How can you prevent stale data? 10 Strategic steps!
- Bottom line?
- Stale data: Related reads
What is stale data? A quick sneak peek!
Stale data is data that is out-of-date, obsolete, or no longer accurate. In the context of databases, software applications, and computing systems, stale data often occurs when an update or refresh operation fails, is delayed, or is not performed regularly.
This can happen for a variety of reasons, including network latency, system outages, synchronization issues, or simply because the data source is not being actively maintained.
And what isn’t stale data?
Data that is current, accurate, and up-to-date information that accurately reflects the most recent state of the subject or entity it represents.
In computing systems, databases, and applications, this means the data has been successfully updated, synchronized, or refreshed to align with the latest changes. This ensures that decisions and actions based on the data are relevant and reliable.
6 Most popular examples of stale data
Stale data can occur in various domains, ranging from technology to business and public services. Below are some popular examples where stale data can have significant implications:
- Financial markets
- E-commerce inventory
- Traffic and navigation systems
- Social media feeds
- Healthcare systems
- Network and cybersecurity monitoring
Let’s understand each example in detail.
1. Financial markets
In the financial world, traders rely heavily on real-time data to make buy or sell decisions. If the data is stale, even by a few seconds, it could lead to poor decisions and financial losses.
Stale exchange rates or outdated stock prices can misguide investors and traders, making them believe they are making profitable moves when, in fact, they are not.
2. E-commerce inventory
Imagine you’re shopping online for a limited-edition item. The website shows it’s in stock, but by the time you proceed to checkout, the item is no longer available.
This happens when the inventory data is stale and not updated in real-time, leading to a poor customer experience and potentially lost sales for the retailer.
3. Traffic and navigation systems
GPS-based navigation apps that use stale traffic data could direct you onto congested routes or areas with ongoing construction.
In this case, the user experience is significantly diminished, and people could be late for important appointments.
4. Social media feeds
Stale data in a social media feed can mean you’re seeing old posts or updates that have already been commented on or resolved.
For instance, a breaking news article that has been updated or corrected might still appear in its original form, causing misinformation.
5. Healthcare systems
In healthcare databases, stale data can have life-or-death consequences.
For example, if a patient’s medication data is not updated in a timely fashion across all systems, medical professionals might administer the wrong medications or dosages based on outdated information.
6. Network and cybersecurity monitoring
In the realm of network security, stale data can be misleading and dangerous. If a system administrator is looking at old logs or outdated threat intelligence data, they may miss current threats or vulnerabilities, thereby compromising the organization’s cybersecurity posture.
Understanding the risks and potential consequences of stale data is crucial for both organizations and individuals. Timely, accurate data is often essential for making informed decisions, and failing to update or refresh data can lead to various kinds of setbacks and complications.
8 Essential root causes of stale data
Stale data occurs when information becomes outdated, inaccurate, or inconsistent, leading to potential errors or poor decision-making. Several factors contribute to the occurrence of stale data across different domains, from technology and finance to healthcare and public services.
Understanding these causes is crucial for implementing strategies to mitigate the risks associated with stale data. Below are some common causes:
- Latency in data transmission
- System outages
- Data synchronization issues
- Caching mechanisms
- Human error
- Software bugs
- Resource limitations
- Policy and permission issues
Let’s explore these causes sequentially.
1. Latency in data transmission
One of the primary causes of stale data is latency in transmitting updates from the data source to the system where the data is being used.
This delay can be due to network congestion, slow servers, or other bottlenecks in data transmission. In real-time systems like stock trading platforms, even milliseconds of latency can cause data to become stale.
2. System outages
Unexpected downtime on servers or databases can result in stale data. During the outage, new data cannot be written or existing data cannot be updated.
Once the system is back online, there may be a lag in syncing the data, causing stale or inconsistent information to appear.
3. Data synchronization issues
In distributed systems where data is stored in multiple locations, synchronization is crucial to ensure that all nodes have the same, most recent data.
Failures or delays in synchronization processes can lead to stale data appearing on one or more nodes.
4. Caching mechanisms
Caches are designed to speed up data retrieval by temporarily storing a copy of the data. However, if the cache is not updated in line with the primary data source, it can serve stale data to users.
This is particularly common in web services and database queries.
5. Human error
In systems that rely on manual updates, human error can be a significant factor in causing stale data.
Whether due to forgetfulness, oversight, or a lack of training, individuals who are responsible for updating data may not do so in a timely or accurate manner.
6. Software bugs
Software glitches or bugs in the code can also lead to stale data. For example, a bug might prevent a database from saving changes, or a flawed algorithm might not recognize that new data is available for updating.
7. Resource limitations
In some cases, systems may have limited computational resources, causing them to prioritize certain operations over data updates.
This can result in delayed updates and, consequently, stale data.
8. Policy and permission issues
Data governance policies and permission settings can also contribute to stale data. For instance, if only specific users are authorized to update certain data fields, and those individuals are unavailable or unaware of the need for an update, the data will become stale.
Understanding the root causes of stale data helps organizations and individuals take proactive steps to prevent it, thereby improving decision-making and operational efficiency.
8 Deadly impacts of stale data you need to know
Stale data, or outdated and inaccurate information, can have wide-ranging negative impacts across various sectors, including business, healthcare, finance, and public services. Such impacts can range from minor inconveniences to serious consequences that can affect an organization’s bottom line or even put lives at risk.
Here are some of the significant impacts of stale data:
- Poor decision-making
- Financial loss
- Compromised user experience
- Reduced operational efficiency
- Impaired data integrity
- Risk of non-compliance
- Loss of competitive edge
- Life-threatening situations in healthcare
Let’s look into each impact closely.
1. Poor decision-making
One of the most immediate impacts of stale data is that it leads to poor decision-making. Whether in business analytics, financial trading, or public policy, decisions based on outdated information are likely to be ineffective or even counterproductive.
2. Financial loss
In sectors like finance and stock trading, decisions are often made in fractions of a second based on real-time data.
Stale data can mislead traders and financial analysts, leading to substantial monetary losses. Similarly, businesses relying on stale data may allocate resources inefficiently, resulting in wasted capital.
3. Compromised user experience
Stale data can significantly degrade the user experience on digital platforms and services. For example, in e-commerce, if inventory levels are not updated in real-time, customers might add items to their cart only to find out at checkout that the product is out of stock.
This not only frustrates the user but also risks losing customers to competitors.
4. Reduced operational efficiency
In any organization, accurate data is crucial for maintaining efficient operations. Stale data can lead to delays, redundancies, and errors in processes ranging from supply chain management to customer service, affecting the organization’s overall performance.
5. Impaired data integrity
Data integrity is vital for the reliability of databases and systems, especially in sensitive sectors like healthcare, law enforcement, and national security.
Stale data can severely compromise the integrity of these systems, leading to incorrect analyses, reporting errors, and ultimately a loss of trust in the data.
6. Risk of non-compliance
Particularly in regulated industries like healthcare and finance, using stale data can lead to non-compliance with laws and regulations.
This could result in legal repercussions, hefty fines, and damage to an organization’s reputation.
7. Loss of competitive edge
For businesses operating in highly competitive markets, stale data can mean missed opportunities and a loss of competitive edge.
Accurate, real-time data is often the key to identifying new market trends, consumer behaviors, or emerging threats and opportunities.
8. Life-threatening situations in healthcare
Perhaps the most critical impact of stale data can be seen in healthcare settings, where outdated patient records, medication lists, or treatment plans can lead to incorrect diagnoses, ineffective treatments, and, in the worst cases, life-threatening situations.
Understanding the potential impacts of stale data is essential for both individuals and organizations. Awareness of these risks prompts the implementation of strategies to prevent or minimize the occurrence of stale data.
How is stale data measured? Here are 8 simple steps!
Measuring stale data involves evaluating the age, accuracy, and relevancy of the information in a given system. Effective measurement can help organizations identify the extent to which stale data is affecting their operations and make informed decisions for mitigation.
Various methods and metrics are used to quantify the state and impact of stale data, and these are often tailored to the specific needs and contexts of the systems in question. Here are some common ways to measure stale data:
- Time-based metrics
- Versioning
- Data freshness indicators
- Quality assessment
- User activity and audit logs
- Performance metrics
- Cross-reference checks
- Automated monitoring and alerts
Let’s understand each step quickly.
1. Time-based metrics
The simplest way to measure stale data is through time-based metrics like timestamps. By comparing the timestamp of the last update with the current time, one can calculate the age of the data and determine if it’s stale according to predefined criteria.
2. Versioning
Version control systems can also be used to measure staleness. In this approach, every update to a data item increments its version number. By comparing the version number of data across different systems or nodes, one can identify if any instance is outdated.
3. Data freshness indicators
Some systems use a metric known as data freshness, which is a measure of how current the data is compared to the source. Data freshness indicators might include flags or counters that get updated whenever the data is refreshed, providing an easy way to measure the age or currency of the data.
4. Quality assessment
Data quality tools can be employed to assess the extent of stale data in a system. These tools often use a range of metrics, including accuracy, completeness, and timeliness, to give a holistic view of data quality, thereby helping to identify stale data.
5. User activity and audit logs
Monitoring user activity and audit logs can also help measure stale data. Frequent reads and minimal writes could indicate that the data is being accessed often but not updated, suggesting that it might be stale.
6. Performance metrics
Stale data often leads to operational inefficiencies. By closely monitoring performance metrics like response times, error rates, or customer satisfaction scores, organizations can indirectly measure the impact of stale data on their operations.
7. Cross-reference checks
Comparing data with other reliable sources or systems is another method to measure staleness. If significant discrepancies are found, it’s likely that one or both sets of data are stale.
8. Automated monitoring and alerts
Some advanced systems have built-in monitoring and alerting mechanisms to notify administrators when data becomes stale. These mechanisms often use a combination of the above methods to measure and track data staleness over time.
Measuring stale data is a critical step in managing its impact on an organization or system. The appropriate measurement techniques often depend on the nature of the data, the architecture of the system, and the specific operational requirements.
Regardless of the method used, the goal is to accurately identify stale data so that corrective actions can be taken to refresh the data and mitigate negative consequences.
How can you prevent stale data? 10 Strategic steps!
Preventing stale data is crucial for maintaining the integrity, accuracy, and effectiveness of various systems, ranging from databases and applications to real-time services and operations. Ensuring data freshness helps in making informed decisions and provides a reliable user experience.
Here are some strategies and techniques for preventing stale data:
- Data refresh policies
- Use of timestamps and versioning
- Real-time synchronization
- Implement caching strategies with care
- Use consistency algorithms in distributed systems
- Monitoring and alerts
- User and admin manual overrides
- Regular audits and quality checks
- Redundancy elimination
- Training and awareness
Now, let’s dive into each step sequentially.
1. Data refresh policies
Implement data refresh policies that automatically update data at regular intervals. Whether it’s real-time, near-real-time, or batch updates, having a consistent refresh policy ensures that data stays current.
2. Use of timestamps and versioning
Employ timestamps and versioning to keep track of when data was last modified or accessed. Systems can be configured to alert administrators when data exceeds a certain age or when discrepancies in versions are detected.
3. Real-time synchronization
In distributed systems, ensure real-time or near-real-time data synchronization across all nodes or databases. Employing real-time synchronization methods can significantly reduce the risk of data becoming stale.
4. Implement caching strategies with care
Caching can speed up data retrieval but can also lead to stale data if not managed properly. Implement cache eviction policies and set appropriate Time-to-Live (TTL) values to ensure that the cached data is refreshed periodically.
5. Use consistency algorithms in distributed systems
In distributed computing environments, algorithms like Paxos, Raft, or Two-Phase Commit can help maintain consistency across different nodes, thereby reducing the chance of stale data.
6. Monitoring and alerts
Implement monitoring systems to track the age, quality, and freshness of data. Automated alerts can notify administrators about potential stale data, allowing for immediate corrective action.
7. User and admin manual overrides
Allow users and administrators to manually refresh the data. This is particularly useful in systems that can’t be updated in real-time and ensures that users can access the most current data when needed.
8. Regular audits and quality checks
Perform regular data audits and quality checks to identify and resolve issues related to stale data. This is essential for maintaining high data integrity and is particularly crucial in sectors like healthcare and finance, where data accuracy is imperative.
9. Redundancy elimination
Eliminate unnecessary copies of data that might become stale and are not being used for specific purposes. The more copies of data there are, the higher the chances of inconsistencies arising.
10. Training and awareness
Educate users and system administrators about the risks of stale data and the importance of keeping information updated. A well-informed team is less likely to ignore or overlook the significance of maintaining data freshness.
Preventing stale data involves a multi-faceted approach that combines technology, processes, and awareness. Tailoring these strategies to fit the specific needs and constraints of your system will go a long way in maintaining data accuracy and reliability.
Bottom line?
- Understanding the impacts of stale data is crucial, as it can lead to misguided decisions, wasted resources, and eroded trust in data-driven processes. We’ve seen how the consequences can ripple through businesses, affecting their efficiency and competitiveness.
- To mitigate the risks associated with stale data, we’ve discussed the importance of measuring data freshness and ensuring data quality.
- In a data-driven world where information is a valuable asset, staying vigilant against the persistence of stale data is imperative.
- By recognizing its existence, understanding its origins, and implementing proactive strategies, organizations can ensure that their data remains a reliable and valuable resource, facilitating more informed and effective decision-making.
Stale data: Related reads
- Data Quality Explained: Causes, Detection, and Fixes
- Data Quality Issues? Here Are 6 Ways to Fix Them!
- Data Quality Measures: Best Practices to Implement
- Data Quality Metrics for Integrity, Consistency & More
- How to Improve Data Quality in 12 Actionable Steps?
- Automated quality control of data pipelines
- Bad Data: How It Sneaks in & How to Manage It in 2024
- Hidden Costs of Bad Data Explained: 12 Ways to Tackle Them
- Dark Data: Concept, Challenges, and Ways to Manage It
Share this article