Data Quality vs Data Accuracy: 15 Key Differences To Know
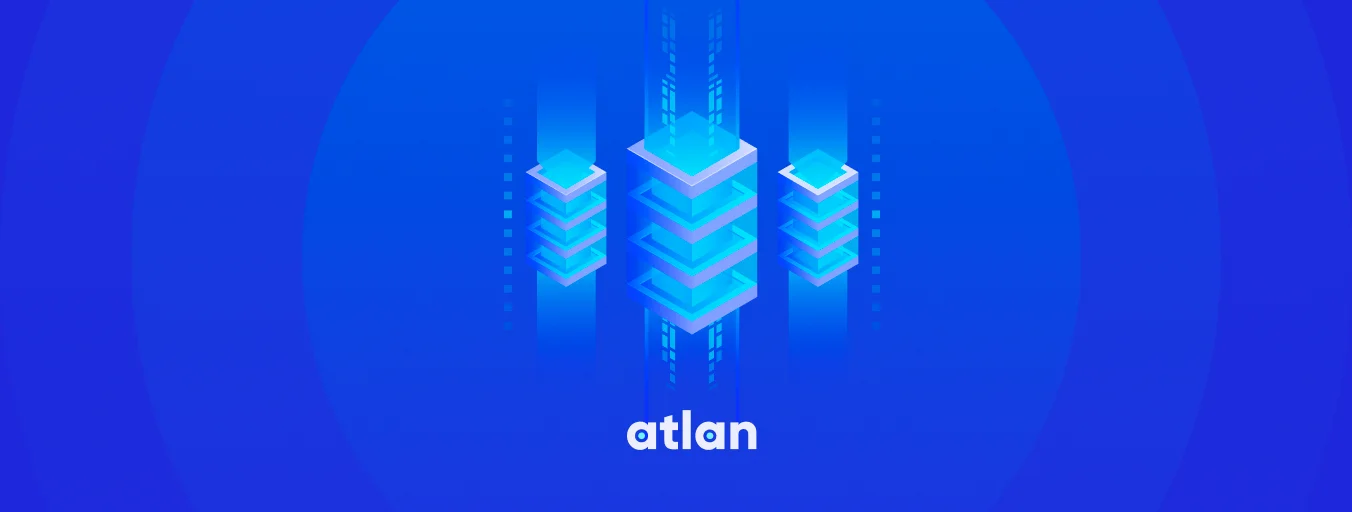
Share this article
Data quality involves assessing various aspects of data, such as reliability, completeness, consistency, and timeliness, to ensure its overall fitness for use, whereas data accuracy specifically relates to the precision and correctness of the information contained in the data, emphasizing the absence of errors or discrepancies.
Amidst a sea of data, a staggering 95% of businesses cite the need to manage unstructured data as a problem for their business. In the realm of data-driven decision-making, grasping the data quality vs data accuracy distinction isn’t just a detail—it’s essential for navigating the digital world successfully.
Understanding the difference between data quality and data accuracy unlocks a crucial understanding necessary for businesses to harness the true power of their data, ensuring not just accuracy in numbers, but excellence in overall data health and applicability.
In this article, we will explore:
- Definitions of data quality and accuracy
- Key differences between them
- Data quality vs integrity vs accuracy
- Real world examples
- Conclusion and related reads
So, let’s dive in!
Table of contents #
- What is data quality?
- What is data accuracy?
- Data quality vs data accuracy: 15 Differences between them
- Is data quality the same as data accuracy?
- Data quality vs data integrity vs data accuracy: A comparative analysis
- Data quality vs data accuracy in practice: 9 Real-world examples
- Conclusion
- Data quality vs data accuracy: Related reads
What is data quality? #
Data quality refers to the measure of data’s fitness for its intended use. It encompasses aspects like accuracy, completeness, consistency, and relevance. High-quality data is crucial for reliable analytics and informed decision-making in businesses, ensuring that strategies are based on solid, dependable information.
The dimensions of data quality include accuracy, completeness, consistency, and relevance. Accuracy ensures data reflects real-world scenarios, while completeness checks for missing elements.
Consistency aligns data across various sources, and relevance assesses its suitability for the context. These aspects collectively determine the usability and reliability of the data.
For businesses, high data quality is fundamental. Poor quality data can lead to flawed decisions and inefficiencies. For example, in marketing, it drives targeted campaigns, while in supply chain management, it optimizes logistics. Therefore, maintaining data quality is essential for efficient and effective business operations.
What is data accuracy? #
Data accuracy refers to the degree to which data correctly represents the true values, attributes, or events it is meant to depict. Accurate data closely aligns with the real-world scenarios or conditions it describes, making it a critical component of effective data management.
Accuracy is essential for ensuring that the insights and decisions derived from data are valid and reliable. The accuracy of data directly influences the quality of decision-making in organizations. Inaccurate data can lead to inaccurate conclusions, affecting everything from strategic business decisions to everyday operational processes.
Maintaining data accuracy involves rigorous validation and verification processes. This includes cross-checking data against reliable sources, employing error-checking algorithms, and regularly updating data to reflect the most current information.
Organizations must prioritize data accuracy to safeguard against the risks associated with misinformation, which can have far-reaching consequences on business performance and reputation.
Data quality vs data accuracy: 15 Differences between them #
In the complex world of data management, the terms ‘data quality’ and ‘data accuracy’ are often used interchangeably, yet they represent distinct concepts with unique implications. Understanding these differences is crucial for professionals who handle, analyze, or make decisions based on data.
Let us look at 15 key differences between them in a tabular format:
Aspect | Data quality | Data accuracy |
---|---|---|
1. Definition | Encompasses the overall utility of data, including various attributes like consistency, reliability, and completeness. | Refers specifically to the closeness of data to true, real-world values or standards. |
2. Scope | Broad and multifaceted, considering various dimensions of data. | Narrower, focusing primarily on the correctness of data. |
3. Dimensions | Includes accuracy, completeness, consistency, reliability, and relevance. | Solely focused on the precision and correctness of data. |
4. Objective | To ensure data is fit for its intended use across various applications. | To ensure data correctly represents what it is supposed to. |
5. Measurement | Assessed through a range of criteria depending on the intended use of the data. | Measured against a known standard or true value. |
6. Impact on decision-making | Affects the overall effectiveness and reliability of data-driven decisions. | Directly influences the correctness of specific data points used in decisions. |
7. Importance in data processing | Critical for the overall integrity and usability of data in different contexts. | Essential for the precision of data, particularly in analytics and reporting. |
8. Relevance in data integration | Ensures consistency and usability of data merged from different sources. | Important for verifying the correctness of individual data points from various sources. |
9. Relation to data integrity | A component of data quality, ensuring data is accurate, complete, and reliable. | A subset of data quality, focused on accuracy alone. |
10. Approach to improvement | Involves enhancing various aspects like data entry processes, validation rules, and data management practices. | Primarily involves correcting errors and inconsistencies in data. |
11. Tools and techniques | Utilizes a variety of tools including data profiling, cleansing, and standardization. | Often involves error detection and correction algorithms. |
12. Influence on user trust | High data quality builds trust in the overall data set and its applications. | High data accuracy builds trust in the specific correctness of data points. |
13. Impact on analytics | Influences the overall reliability and effectiveness of analytics. | Affects the precision and validity of analytical results. |
14. Industry examples | In healthcare, affects patient records’ usability; in finance, influences transaction data’s reliability. | In scientific research, crucial for experimental data; in manufacturing, vital for measurements and specifications. |
15. Long-term goals | Aims for comprehensive data management and governance strategies. | Focuses on maintaining and improving the specific accuracy of data over time. |
Understanding the nuances between data quality and data accuracy is pivotal for any data-driven business or individual. While data quality is a broad concept encompassing various dimensions including accuracy, data accuracy is a specific aspect focusing on the correctness of data.
Both play critical roles in the realm of data management, influencing decision-making, analytics, and overall data integrity.
By distinguishing these two concepts, organizations can better strategize their data management practices, ensuring not only the accuracy of their data but also its overall quality and suitability for diverse applications. This distinction empowers businesses to utilize data more effectively, driving informed decisions and fostering trust among users and stakeholders.
Is data quality the same as data accuracy? #
In the complex landscape of data management, a common query often arises: Is data quality the same as data accuracy? While they are interrelated and often confused with one another, they are distinctly different concepts.
Understanding this distinction is crucial for anyone dealing with data, whether it’s for analytical, operational, or strategic purposes.
Here are some nuances between data quality and data accuracy:
- Conceptual foundation
- Scope and breadth
- Impact on decision making
- Measurement and evaluation
- Relevance in different industries
- Strategies for improvement
- Relationship with data integrity
- Long-term organizational impact
- Evolution and adaptation
Let’s look into each of the above nuances in brief:
1. Conceptual foundation #
Data quality is an umbrella term that includes various attributes of data, including accuracy, but extends beyond it. It refers to the overall suitability of data for its intended use, considering factors such as completeness, consistency, relevance, and timeliness.
On the other hand, data accuracy is a singular component of data quality, specifically focusing on the correctness and precision of data in representing real-world values or conditions. Understanding this fundamental distinction is crucial as it sets the stage for how data is evaluated and managed within an organization.
2. Scope and breadth #
Data quality addresses a broader spectrum of characteristics that determine the usability and effectiveness of data in various contexts. It’s not just about whether the data is correct, but also whether it is appropriate, up-to-date, and presented in a consistent manner.
In contrast, data accuracy zeroes in on one specific aspect: the truthfulness and exactness of the data. While accuracy is critical, it’s just one piece of the larger data quality puzzle.
3. Impact on decision making #
The influence of data quality extends to the overall reliability and applicability of data in decision-making processes. High-quality data ensures that decisions are based on comprehensive and contextually relevant information.
Conversely, data accuracy directly affects the correctness of those decisions. Inaccurate data, even if it’s high in other quality dimensions, can lead to flawed conclusions and misguided strategies.
4. Measurement and evaluation #
Measuring data quality involves assessing multiple dimensions using a variety of criteria, which can vary depending on the data’s intended use. This multifaceted evaluation ensures that the data meets diverse needs and expectations.
Data accuracy, however, is more straightforward to measure as it involves comparing data points to a known standard or truth to ascertain their correctness.
5. Relevance in different industries #
Different industries emphasize different aspects of data quality based on their specific needs. For example, in healthcare, the completeness and confidentiality of patient data are as important as its accuracy. In financial sectors, the consistency and timeliness of data are crucial.
Data accuracy, while universally important, takes on varying degrees of emphasis depending on the sector’s reliance on precision.
6. Strategies for improvement #
Improving data quality is a holistic process that includes enhancing data collection methods, validation processes, and ongoing data management practices. It requires a comprehensive approach to address all facets of quality.
Improving data accuracy, in contrast, often involves more targeted initiatives like error correction, data verification, and implementing precision-focused technologies.
7. Relationship with data integrity #
Data integrity and data quality are closely related, with integrity often considered a component of quality. Data integrity ensures data is accurate, consistent, and reliable over its lifecycle.
Data accuracy is a subset of both integrity and quality, focusing specifically on precision and correctness without necessarily encompassing broader integrity concerns like security and compliance.
8. Long-term organizational impact #
The long-term impact of data quality encompasses the entire spectrum of data-driven activities within an organization. It influences customer satisfaction, operational efficiency, and strategic planning.
Data accuracy, while impactful, primarily affects specific areas like reporting accuracy, analytical precision, and operational correctness.
9. Evolution and adaptation #
As the field of data management evolves, the understanding and importance of data quality continue to expand, embracing new dimensions like user-friendliness and interoperability.
Data accuracy also evolves but within a narrower scope, adapting to advancements in measurement techniques and standards of correctness in various fields.
Data quality and data accuracy, while closely related, are not the same. Data quality is a broad concept encompassing various dimensions, including accuracy, while data accuracy is a specific aspect of data quality focusing on the correctness and precision of data. Recognizing this distinction is essential for effective data management and utilization.
By understanding and addressing both the comprehensive nature of data quality and the specific focus of data accuracy, organizations can make more informed decisions, achieve higher operational efficiency, and leverage data as a strategic asset. This clarity not only enhances data practices but also fosters a more nuanced approach to the ever-evolving challenges of data management.
Data quality vs data integrity vs data accuracy: A comparative analysis #
In the complex domain of data management, three terms often arise in professional debate: data quality, data integrity, and data accuracy. While these concepts are interlinked, understanding their unique characteristics and differences is crucial for effective data handling and analysis.
This topic aims to unravel these terms, offering clarity on how each one distinctively contributes to the overall value and reliability of data.
Let us look at the differences between data quality, data integrity, and data accuracy:
Aspect | Data quality | Data integrity | Data accuracy |
---|---|---|---|
1. Definition | A broad concept includes the overall utility and fitness of data for its intended use. | Ensures data is accurate, consistent, and reliable throughout its lifecycle. | Specifically pertains to the correctness and precision of data in representing real-world values. |
2. Scope | Encompasses various attributes including accuracy, completeness, consistency, reliability, and relevance. | Focused on maintaining and assuring the accuracy and consistency of data over its entire lifecycle. | Narrowly focused on the exactness and correctness of individual data elements. |
3. Objective | To make data suitable for a specific purpose or use. | To safeguard data against corruption, unauthorized access, and ensure its consistency. | To ensure data precisely represents the intended information. |
4. Measurement | Assessed using a variety of criteria relevant to the intended use of data. | Measured by the absence of corruption and adherence to data models and rules. | Measured against known standards or true values to ascertain correctness. |
5. Importance | Critical for ensuring the overall effectiveness and reliability of data-driven decisions. | Essential for ensuring data remains unaltered from its original source and consistent throughout its use. | Key for the precision and validity of specific data points. |
6. Techniques | Utilizes methods like data cleansing, profiling, and standardization. | Involves techniques like data encryption, backup, and access controls. | Involves error detection and correction methods. |
7. Impact on business | Affects decision-making, customer satisfaction, and operational efficiency. | Influential in maintaining regulatory compliance and preventing data breaches. | Directly impacts the accuracy of reports, analyses, and operational processes. |
8. Industry examples | In marketing, ensures campaign data is relevant and complete; in healthcare, ensures patient data is reliable and accurate. | In banking, crucial for maintaining transaction consistency; in software development, vital for code and database integrity. | In scientific research, critical for precise measurements; in manufacturing, essential for accurate product specifications. |
9. Relationship with data management | A comprehensive aspect of data management strategies. | A component of data security and compliance in data management. | A specific focus within the broader data quality framework. |
10. Long-term goals | Aims for comprehensive data management and governance to ensure data is fit for various applications. | Focuses on preserving data's state and data consistency throughout its lifecycle. | Concentrates on maintaining and enhancing the correctness of data over time. |
Understanding the distinctions between data quality, data integrity, and data accuracy is essential in the data management landscape. Data quality is a comprehensive concept encompassing the overall fitness of data for use, including aspects like integrity and accuracy.
Data integrity focuses on the consistent and unaltered maintenance of data, while data accuracy zeroes in on the precision and correctness of data. Grasping these differences is key for businesses to manage their data effectively, ensuring not only its accuracy and security but also its overall utility and reliability for informed decision-making and operational success.
Data quality vs data accuracy in practice: 9 Real-world examples #
In the dynamic world of data management, understanding the practical implications of data quality and data accuracy is vital. While both are critical components of effective data handling, they manifest differently in real-world scenarios.
By examining these cases, readers can gain a deeper understanding of how these concepts play out in various industries and applications, and why distinguishing between them is crucial for successful data-driven operations.
Here are the real-world examples of data quality and data accuracy:
- Healthcare records management
- Financial reporting
- E-commerce inventory management
- Marketing campaign analysis
- Real estate property listings
- Manufacturing process control
- Academic research
- Transportation and logistics
- Environmental monitoring
Let’s look into each of the above examples in brief:
1. Healthcare records management #
In healthcare, data quality refers to the comprehensive accuracy, completeness, and accessibility of patient records. High data quality ensures that healthcare providers have a full picture of a patient’s medical history, leading to better diagnosis and treatment plans.
Conversely, data accuracy in this context would specifically refer to the correctness of individual patient details like test results or medication dosages. Inaccurate data can lead to harmful medical errors, while poor data quality can result in incomplete patient care.
2. Financial reporting #
For financial institutions, data quality covers the reliability and consistency of financial data across different reports and systems. High-quality financial data provides a trustworthy foundation for decision-making and regulatory compliance.
Data accuracy, on the other hand, specifically involves the correctness of individual data points, such as transaction values or account balances. Accurate data is crucial for preventing fraud and ensuring precise financial statements.
3. E-commerce inventory management #
In e-commerce, data quality involves ensuring that inventory records are complete, up-to-date, and consistent across all platforms. This ensures efficient order processing and customer satisfaction.
Data accuracy, in contrast, focuses on the correct representation of individual inventory items, like the exact number of units available. Inaccuracies can lead to over-selling or stock shortages, directly impacting sales and customer trust.
4. Marketing campaign analysis #
Data quality in marketing analytics involves having complete, relevant, and timely data for campaign analysis. This enables marketers to make informed decisions about future campaigns.
Data accuracy in this field refers to the precision of individual metrics, like click-through rates or engagement statistics. Accurate data is essential for assessing the true performance of marketing efforts.
5. Real estate property listings #
In real estate, data quality refers to the overall completeness, consistency, and reliability of property listings. High-quality data ensures that listings are comprehensive and useful for potential buyers.
Data accuracy, specifically, would involve the correctness of listing details such as property dimensions or amenities. Accurate data is crucial for maintaining credibility and avoiding misleading buyers.
6. Manufacturing process control #
In manufacturing, data quality pertains to the holistic reliability and usability of data across various production processes. This includes ensuring data consistency and data completeness for efficient operation.
Data accuracy in manufacturing is about the exactness of measurements and specifications, which is critical for product quality and safety.
7. Academic research #
For academic researchers, data quality involves ensuring that research data is complete, relevant, and consistently formatted for analysis. High-quality data enables robust research findings.
Data accuracy in academic research refers to the precision of collected data points, such as experimental measurements, which are essential for validating research hypotheses.
8. Transportation and logistics #
In the transportation and logistics sector, data quality refers to the comprehensive accuracy, timeliness, and consistency of logistics data. This ensures efficient routing, scheduling, and resource allocation.
Data accuracy specifically involves the correctness of individual data elements like package locations or delivery times, which are crucial for operational efficiency and customer satisfaction.
9. Environmental monitoring #
In environmental monitoring, data quality means having reliable, complete, and timely data to assess environmental conditions accurately. This is vital for informed policy-making and public awareness.
Data accuracy in this context refers to the precision of individual environmental readings, such as pollution levels or temperature readings, which are critical for accurate assessments and interventions.
These real-world examples demonstrate that while data quality and data accuracy are closely related, they address different aspects of data management and have distinct impacts across various fields. Data quality is a broader concept encompassing the overall utility of data, whereas data accuracy focuses on the correctness of individual data points.
Understanding and distinguishing between these two is crucial for effective data handling, leading to more informed decision-making and improved operational outcomes in diverse sectors. Recognizing their specific roles and implications helps organizations and professionals optimize their data strategies for maximum efficiency and reliability.
Conclusion #
Understanding the difference between data quality and data accuracy is like knowing the ingredients in a recipe versus how they blend together.
Data accuracy is about getting each detail right - it’s like making sure you have the exact amount of sugar for a cake. But data quality is the bigger picture; it’s making sure your cake has all the right ingredients and is baked well. It’s not just about the sugar, but also the flour, eggs, and how you mix them.
In our daily work, this matters a lot. Good data helps companies make smart decisions. It’s like using a well-tested recipe for success. If the data is accurate but lacks other quality aspects, it’s like having the right amount of sugar but forgetting the eggs. You need everything to work together.
So, as we wrap up, remember: data quality and accuracy are both important. They’re part of the same team but play different roles. Just like in baking, every ingredient counts. Keep this in mind, and you’ll make better, more informed decisions - in data and maybe in the kitchen, too!
Data quality vs data accuracy: Related reads #
- Data Quality Explained: Causes, Detection, and Fixes
- Data Quality Fundamentals: Why It Matters in 2023
- Data Accuracy in 2023: A Roadmap for Data Quality
- Data Quality vs Data Governance: Learn the Differences & Relationships!
- Data Integrity vs Data Quality: Nah, They Aren’t Same!
- How to Improve Data Quality in 12 Actionable Steps?
- Data Quality is Everyone’s Problem, but Who is Responsible?
- Data Quality in Data Governance : The Crucial Link That Ensures Data Accuracy and Integrity
- Data Quality Dimensions: Do They Matter in 2023 & Beyond?
Share this article