What is Data Cataloging & Why Its 6 Components Matter?
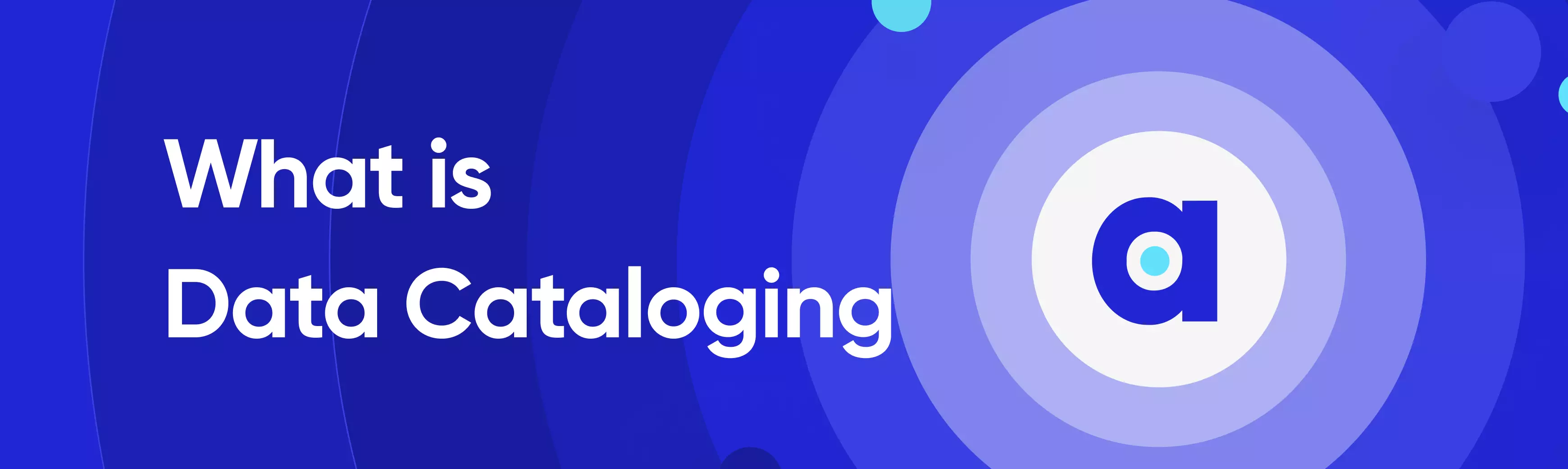
Share this article
What is data cataloging? #
Data cataloging is the process of creating and maintaining a centralized and organized repository of metadata and information about the data assets within an organization.
A data cataloging tool helps users find, understand, and use the data more effectively by providing a comprehensive view of all available datasets, their relationships, and their properties.
Modern data problems require modern solutions - Try Atlan, the data catalog of choice for forward-looking data teams! 👉 Book your demo today
In this article, we will learn essential components of data cataloging, how it unifies metadata across different systems, and how it can benefit different teams in your organization.
Let’s dive in!
Table of contents #
- What is data cataloging?
- Understanding the six essential components of data cataloging
- 5 Key benefits of data cataloging
- 10 Essential features of data cataloging
- From chaos to order: How data cataloging unifies metadata across different systems
- User personas and use cases: How data cataloging can benefit IT teams, business analysts, and end users
- Challenges in data cataloging
- 10 Data cataloging examples
- Rounding it all up
- What is Data Cataloging? Related reads
Understanding the six essential components of data cataloging #
Data cataloging typically includes the following six essential components:
- Metadata
- Data lineage
- Data quality indicators
- Data governance policies
- Data usage analytics
- Collaboration tools
Let’s look at them in detail:
1. Metadata #
This includes information about the data, such as table names, column names, data types, descriptions, and other relevant details. Metadata helps users understand the structure and meaning of the data.
2. Data lineage #
This shows the origin and history of the data, including how it was transformed, processed, or combined with other datasets. Data lineage helps users trace data back to its source and understand how it has changed over time.
3. Data quality indicators #
These provide insights into the accuracy, completeness, and consistency of the data, helping users assess its reliability for their purposes.
4. Data governance policies #
These outline the rules and processes for accessing, modifying, and using the data, ensuring that data is used appropriately and securely.
5. Data usage analytics #
This offers insights into how frequently and by whom the data is being used, helping organizations identify popular datasets and prioritize improvements.
6. Collaboration tools #
These enable users to share insights, ask questions, and discuss the data, promoting a more data-driven culture within the organization.
5 Key benefits of data cataloging #
By implementing a data catalog tool, your company can:
- Improve data discovery
- Enhance data understanding
- Foster collaboration
- Streamline data governance
- Monitor data usage
Let’s look at them in detail:
1. Improve data discovery #
Business users can quickly find relevant datasets without having to rely on technical staff or dig through multiple systems.
2. Enhance data understanding #
Users can easily access metadata, lineage, and quality information to better understand the context and trustworthiness of the data.
3. Foster collaboration #
Data cataloging promotes knowledge sharing and collaboration among business users, leading to better data-driven decision-making.
4. Streamline data governance #
Centralizing metadata and governance policies helps ensure that data is used responsibly and securely.
5. Monitor data usage #
Understanding how data is being used can help prioritize improvements and identify areas where additional training or support may be needed.
Data cataloging can empower your business users to leverage data more effectively and make better-informed decisions without needing deep technical expertise.
10 Essential features of data cataloging #
Data cataloging is a critical component of modern data management strategies, enabling organizations to organize, discover, and govern their data assets effectively. Here are some of the key features that characterize data cataloging systems:
- Metadata management
- Data discovery and classification
- Data governance and compliance
- Data quality management
- Data lineage visualization
- Collaborative features
- Search and query capabilities
- Integration with data sources and tools
- User access and security management
- Customizability and scalability
Let’s look at them in detail:
1. Metadata management #
Metadata management is a core feature of data cataloging that involves the extraction, storage, and maintenance of metadata. This process is crucial because metadata provides detailed information about data, including its origin, format, content, and relationships with other data sets.
Effective metadata management enhances the understanding of data, helping organizations to maintain a structured and well-organized data environment. This feature is instrumental in simplifying the complexity of data landscapes, allowing for better organization and accessibility of data assets.
2. Data discovery and classification #
Data discovery and classification is a pivotal feature of data cataloging systems. This capability automatically identifies and categorizes data across various sources, using algorithms to scan, tag, and classify data according to predefined rules or patterns.
This feature is particularly beneficial for handling large datasets, as it facilitates easier data retrieval and management. By enabling quick identification and categorization of data, it helps organizations to streamline their data management processes and ensures that data assets are efficiently utilized.
3. Data governance and compliance #
Data governance and compliance are integral to data cataloging. This feature supports data governance policies and ensures compliance with various regulations. It includes setting data access controls, auditing data usage, and tracking data lineage.
The importance of this feature lies in its ability to ensure responsible use of data and adherence to legal and regulatory standards. It provides a framework for managing data assets in a way that aligns with organizational policies and external regulatory requirements, thereby safeguarding data integrity and trust.
4. Data quality management #
Data quality management is a critical feature that ensures the accuracy, completeness, and reliability of data. It involves validating and cleansing data, managing data quality rules, and monitoring data quality metrics.
The significance of this feature cannot be overstated, as high-quality data is foundational to effective decision-making and operational efficiency. By maintaining stringent data quality standards, data cataloging tools help improve the trustworthiness and usefulness of data across the organization.
5. Data lineage visualization #
Data lineage visualization offers a visual representation of the data’s lifecycle. It shows the data’s origins, movements, and transformations, tracing it from its source through various transformations to its final form.
This feature is essential for understanding complex data relationships, impact analysis, and troubleshooting. It provides transparency and clarity on how data is used and transformed within an organization, thereby enhancing data accountability and governance.
6. Collaborative features #
Collaborative features in data cataloging tools enable users to share insights, annotations, and recommendations about data. This may include user ratings, comments, and shared views, promoting a collaborative data culture within the organization.
The benefits of these features include improved data literacy, leveraging collective knowledge, and fostering an environment where data is a shared asset that benefits from the contributions and insights of various users.
7. Search and query capabilities #
Search and query capabilities in data cataloging tools allow users to easily find and access data using advanced search tools. This includes full-text search, filtering options, and natural language queries.
These features save time and improve productivity by making data easily accessible to all users, regardless of their technical expertise. Efficient search and query capabilities are fundamental in a data-rich environment, enabling quick access to relevant information and facilitating data-driven decision-making.
8. Integration with data sources and tools #
Integration with various data sources and existing data tools and platforms is a key feature of data cataloging systems. This capability ensures seamless connectivity with databases, cloud storage, data lakes, and business intelligence tools.
The benefit of this feature is the creation of a unified view of all data assets, regardless of where they are stored. This integration is critical for ensuring that data cataloging tools work effectively within the broader data ecosystem of an organization.
9. User access and security management #
User access and security management is a vital feature of data cataloging systems. This includes managing who can access what data and how they can use it, incorporating role-based access controls, authentication mechanisms, and audit logs.
This feature is crucial for protecting sensitive data and ensuring that data is used in a secure and controlled manner. By enforcing strict access controls and security measures, data cataloging tools help maintain the confidentiality and integrity of data assets.
10. Customizability and scalability #
Customizability and scalability are important features of data cataloging tools. These tools allow customization according to specific organizational needs and can scale to accommodate growing data volumes.
The adaptable architecture and the ability to handle increasing amounts of data ensure that the data catalog can evolve with the organization. This flexibility and scalability are essential for maintaining the relevance and effectiveness of the data cataloging system in a dynamic and evolving data landscape.
Data cataloging tools, equipped with these features, empower organizations to handle the challenges of big data by making data more organized, understandable, and actionable. Whether it’s for compliance, strategic decision-making, or operational efficiency, these features are fundamental in unlocking the true potential of an organization’s data assets.
From chaos to order: How data cataloging unifies metadata across different systems #
Metadata and data cataloging are closely related concepts but have distinct roles in organizing and managing data. Data cataloging can utilize a variety of metadata from your tech stack, including Snowflake, Power BI, SQL on-prem server, Power BI on-prem, SAP HANA, ThoughtSpot, and even Microsoft Teams.
Here are some examples of metadata that a data catalog can collect and integrate from these systems (note that this list cites popular systems and is not an exhaustive list):
- Snowflake
- Power BI (cloud and on-prem)
- SQL 0n-prem server
- SAP HANA
- ThoughtSpot
- Microsoft Teams
Let’s discuss them in detail:
1. Snowflake #
- Table and schema names
- Column names, data types, and descriptions
- Data warehouse, database, and schema sizes
- Query history and usage statistics
- Access control policies and roles
2. Power BI (cloud and on-prem) #
- Dashboard and report names, descriptions, and creators
- Dataset names, sources, and refresh schedules
- Table and column names, data types, and relationships
- Measures, calculated columns, and DAX expressions
- Visualization types, chart titles, and axis labels
3. SQL on-prem server #
- Database, table, and schema names
- Column names, data types, and descriptions
- Indexes, primary keys, and foreign keys
- Stored procedures, functions, and triggers
- Query history, execution plans, and performance statistics
4. SAP HANA #
- Database, schema, and table names
- Column names, data types, and descriptions
- Calculation views, analytic views, and attribute views
- Stored procedures, functions, and triggers
- Data provisioning methods and replication status
5. ThoughtSpot #
- Search index names and descriptions
- Data source connection details
- Table and column names, data types, and relationships
- Synonyms and search keywords
- Worksheet and pinboard names, creators, and descriptions
6. Microsoft Teams #
- Team names, descriptions, and members
- Channel names and purposes
- Conversations, messages, and file attachments
- Meeting and call details (e.g., date, time, participants, duration)
- Apps, bots, and custom connectors used within the teams
By collecting metadata from these various systems, your data catalog can provide a unified view of your organization’s data assets, making it easier for business users to discover and understand the data they need for their analysis and decision-making.
User personas and use cases: How data cataloging can benefit IT teams, business analysts, and end users #
We can categorize the users of a data catalog into three main groups, each with its specific use cases:
- IT Team
- Business analysts
- End users
Let’s look at them in detail:
1. IT Team (Data engineers, DevOps, etc.) #
a. Data lineage
Understand the flow and transformation of data across various systems, allowing them to trace data back to its source and analyze its history. This helps with debugging issues, ensuring data accuracy, and maintaining compliance.
b. Impact analysis
Assess the potential effects of changes to data sources, schemas, or processes on downstream systems and reports. This helps them make informed decisions when planning and implementing changes, minimizing unintended consequences and reducing the risk of data-related issues.
2. Business analysts (Data analysts, information analysts, etc.) #
a. Tagging
Annotate datasets, tables, and columns with relevant tags or labels to improve data discovery and understanding. This enables analysts to quickly find and understand the data they need for their analysis.
b. Categorizing
Organize data assets into meaningful categories or groups, making it easier for users to navigate and discover relevant datasets.
c. Data dictionary
Create and maintain a data dictionary, which is a centralized repository of definitions, descriptions, and metadata for all data elements. This helps analysts and other users to understand the meaning and context of the data.
d. Data governance
Collaborate with IT and other stakeholders to develop and enforce data governance policies, ensuring that data is used responsibly, securely, and in compliance with regulations.
3. End users (Business users, decision-makers, etc.) #
a. Data discovery
Leverage the metadata, tags, categories, and data dictionary created by the other user groups to easily find and understand relevant data for their specific needs.
b. Collaboration
Use the data catalog’s collaboration tools to ask questions, share insights, and discuss data with colleagues, fostering a data-driven culture and improving decision-making.
c. Trust and confidence
Access data quality indicators, lineage information, and governance policies to assess the reliability and trustworthiness of the data, enabling them to make more informed decisions.
d. Self-service
Empower end users to explore and analyze data on their own without relying on technical staff, improving their efficiency and productivity.
By understanding the different user groups and their unique use cases, you can tailor the implementation of a data catalog to meet the specific needs of your organization and drive maximum value from your data assets.
Challenges in data cataloging #
Data cataloging, while immensely beneficial for managing and leveraging data assets within an organization, comes with its own set of challenges. These challenges can range from technical complexities to organizational hurdles. Understanding these challenges is crucial for organizations aiming to implement effective data cataloging practices.
- Data volume and complexity
- Ensuring data quality and consistency
- Integration with existing systems
- Data governance and compliance
- User adoption and training
- Maintaining data security and privacy
- Scalability and performance
- Metadata management and lineage
- Cost and resource allocation
- Keeping up with technological advances
Let’s understand them in detail:
1. Data volume and complexity #
The sheer volume and complexity of data that organizations collect can make cataloging a daunting task. As data sources and types proliferate, cataloging systems must keep pace, which can be technically challenging.
If not managed properly, the overwhelming amount of data can lead to inefficient data cataloging, making it difficult for users to find the data they need.
2. Ensuring data quality and consistency #
Ensuring the quality and consistency of data in the catalog is a major challenge. Poor quality data can lead to incorrect analysis and business decisions.
The presence of inaccurate, incomplete, or outdated data in the catalog can undermine trust in the entire data ecosystem.
3. Integration with existing systems #
Integrating a data cataloging tool with existing data systems and workflows can be complex. It involves technical challenges related to compatibility, data formats, and maintaining data integrity during integration.
Failure to properly integrate can lead to data silos, where information is not properly shared across the organization.
4. Data governance and compliance #
Ensuring that data cataloging adheres to data governance policies and compliance regulations is challenging, especially as these regulations evolve and vary across regions.
Non-compliance can result in legal issues and fines, as well as damage to an organization’s reputation.
5. User adoption and training #
Encouraging user adoption and providing adequate training can be difficult. Users may resist changing their existing workflows or may not understand how to effectively use the data catalog.
Without proper user adoption, the full benefits of data cataloging cannot be realized, leading to underutilization of the tool.
6. Maintaining data security and privacy #
Balancing data accessibility with security and privacy is a critical challenge. Data catalogs must protect sensitive information while making data accessible to authorized users.
Insufficient security measures can lead to data breaches, while overly restrictive access can hinder data usability.
7. Scalability and performance #
As data volume grows, maintaining the performance and scalability of the data cataloging system can be challenging.
Scalability issues can lead to slow performance, impacting user experience and reducing efficiency.
8. Metadata management and lineage #
Accurately tracking and managing metadata, especially data lineage (the data’s origin and lifecycle), is complex but crucial for effective cataloging.
Poor metadata management can lead to confusion about data sources, history, and usage, impacting data trustworthiness.
9. Cost and resource allocation #
Implementing and maintaining a data cataloging system can be costly, requiring significant investment in both technology and skilled personnel.
Limited budgets and resources can constrain the scope and effectiveness of data cataloging initiatives.
10. Keeping up with technological advances #
The rapid pace of technological change means that data cataloging tools and practices must continually evolve.
Organizations may struggle to keep their data cataloging capabilities up-to-date, potentially leading to inefficiencies and competitive disadvantages.
In conclusion, while data cataloging is a powerful tool for harnessing the potential of data, it comes with a range of challenges that require careful planning, robust technology, and strategic foresight to overcome. Organizations must acknowledge these challenges and actively work towards addressing them to fully leverage the benefits of data cataloging.
10 Data cataloging examples #
Data cataloging is a critical process in data management, which involves organizing, managing, and making data easy to find and use within an organization. Here are some examples of data cataloging that you, as a content writer, might explore:
- E-commerce retail catalog
- Healthcare patient records
- Financial data management
- Public sector - government records
- Academic research and libraries
- Media and entertainment
- Corporate data repositories
- IoT device management
- Supply chain and logistics
- Marketing and customer data
Let’s see them in detail:
1. E-commerce retail catalog #
An e-commerce company might use data cataloging to manage and categorize their vast inventory of products. This would include detailed product information, pricing, supplier details, and stock levels. A well-organized data catalog helps in enhancing the customer shopping experience by enabling effective search and recommendation systems.
2. Healthcare patient records #
In healthcare, data cataloging is used to organize patient records, clinical trials data, and research studies. A healthcare data catalog might include patient demographics, treatment history, test results, and medication records, all categorized in a way that ensures privacy compliance yet allows for efficient retrieval for treatment or research purposes.
3. Financial data management #
Financial institutions rely heavily on data cataloging to manage their vast amounts of financial data, including transaction histories, customer profiles, market data, and regulatory documents. Effective cataloging not only helps in better customer service but also ensures compliance with various financial regulations.
4. Public sector - government records #
Government agencies use data catalogs to manage public records, census data, environmental data, and policy documents. These catalogs are often designed to be accessible to the public, providing transparency and facilitating research and policy analysis.
5. Academic research and libraries #
Universities and research institutions catalog research papers, academic journals, and educational resources. This type of cataloging aids in academic research, helping students and researchers locate necessary documents and studies efficiently.
6. Media and entertainment #
In the media and entertainment industry, data cataloging is used to manage content libraries, including movies, music, and digital assets. This helps in content management, distribution, and in providing personalized recommendations to users.
7. Corporate data repositories #
Large corporations often maintain extensive data catalogs for internal data governance. These catalogs include project documents, employee records, internal research data, and operational data. Such cataloging aids in knowledge management and decision-making processes within the organization.
8. IoT device management #
For companies dealing with IoT (Internet of Things), data cataloging is crucial for managing data from various devices and sensors. This includes data on device performance, environmental data, usage patterns, and maintenance records.
9. Supply chain and logistics #
Data cataloging in supply chain management involves organizing data related to product sourcing, inventory levels, transportation logistics, and customer delivery information. Effective cataloging ensures smoother operations and improved customer satisfaction.
10. Marketing and customer data #
Marketing departments catalog customer data, market research, campaign results, and customer feedback. This organized data helps in crafting targeted marketing strategies and understanding customer preferences.
In conclusion, data cataloging plays a pivotal role in various industries by organizing data in a structured manner, thereby enhancing efficiency, decision-making, and compliance with regulations. As a content writer, delving into these examples can provide rich material for discussions on data management and its impact on different sectors.
Rounding it all up #
Data cataloging involves the systematic organization and indexing of metadata and other related information to create a searchable catalog of data assets within an organization. A data catalog serves as a centralized inventory of available data sources, datasets, databases, files, reports, and other data artifacts.
It acts as a data discovery tool, enabling users to find, explore, and understand the available data assets.
Data cataloging goes beyond mere metadata management by providing a user-friendly interface and search capabilities to locate and access data assets effectively. It may include features such as data profiling, data lineage visualization, user annotations, data usage statistics, and collaborative functionalities.
Data cataloging solutions help data consumers, analysts, and scientists discover relevant data assets, understand their contents and characteristics, and assess their fitness for specific use cases.
Deploying a data catalog tool starts the seeding process of data democratization and data enablement in your organization. It says that your organization is serious about maximizing the value of data.
It also recognizes that we can extract much more from data when we create an even playing field for the diverse data users in an organization. A data catalog is a starting point for that inclusive initiative.
Are you considering data cataloging for your organization — you might want to check out Atlan.
What is Data Cataloging? Related reads #
- Enterprise data catalog: Definition, Importance & benefits
- Data Catalog: The Must-Have Tool for Data Leaders in 2023
- Data catalog benefits: 5 key reasons why you need one
- Open Source Data Catalog Software: 5 Popular Tools to Consider in 2023
- Data Catalog Platform: The Key To Future-Proofing Your Data Stack
- Top Data Catalog Use Cases Intrinsic to Data-Led Enterprises
- AWS Glue Data Catalog: Architecture, Components, and Crawlers
- Airbnb Data Catalog — Democratizing Data With Dataportal
- Lexikon: Spotify’s Efficient Solution For Data Discovery And What You Can Learn From It
- Google Cloud Data Catalog Guide - Everything You Need to Know
Share this article