Data Anomaly & Quality Monitoring: Importance, Impact & Roadmap
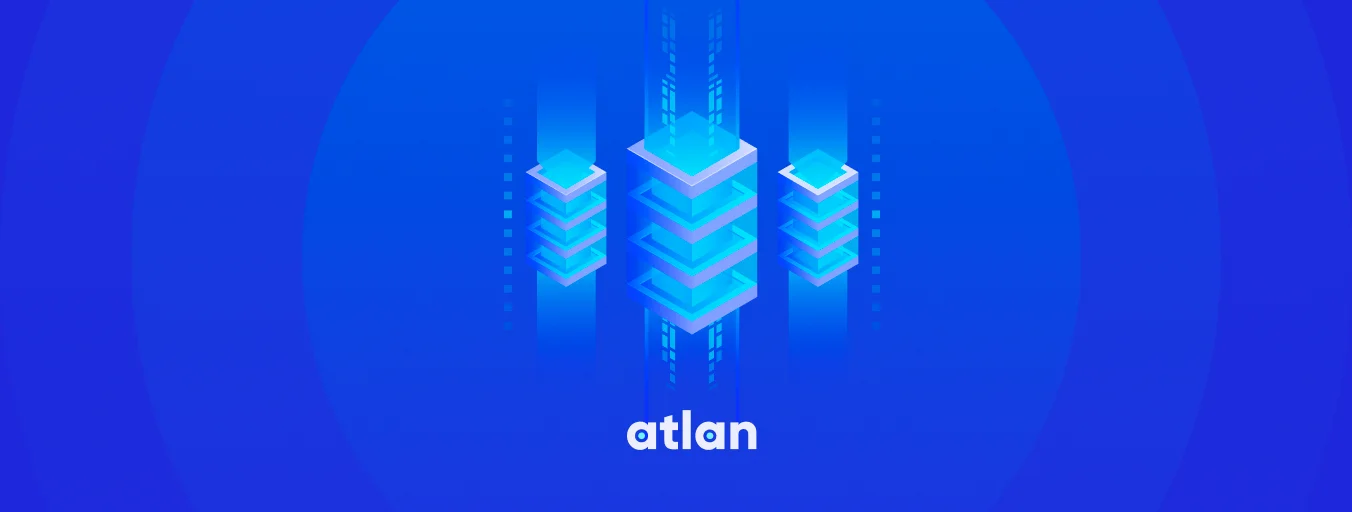
Share this article
In the modern business landscape, the role of data in shaping decisions and strategies is undeniable. To ensure the reliability and effectiveness of these data-driven processes, vigilant monitoring of data anomalies and quality is essential.
This practice goes beyond technicalities; it’s a strategic necessity for organizations seeking accurate insights, efficient operations, and competitive advantage.
In this article, we delve into the practical world of data anomaly and quality monitoring, uncovering its significance, methods, and real-world impacts. As businesses increasingly rely on data, understanding how to effectively monitor its quality and detect anomalies becomes a roadmap to success.
Let’s begin!
Table of contents #
- What is a data anomaly?
- What is data quality?
- The top 10 reasons why monitoring data anomalies and quality is critical
- Unknown impacts of poor data quality and data anomalies
- Roadmaps to monitoring data anomaly and data quality
- Bottom line
- Related reads
What is a data anomaly? #
A data anomaly is a data point or a set of data points that deviate significantly from the expected patterns or behaviors within a dataset. Anomalies can arise due to various reasons, including system malfunctions, human errors, or rare events.
They can sometimes indicate significant incidents or problems that need attention. In different contexts, anomalies might be referred to as outliers, exceptions, aberrations, or discrepancies.
Detecting data anomalies: Why is it important? #
- Detecting anomalies is crucial for a range of applications, including fraud detection, system health monitoring, fault detection, and network security.
- Various techniques, both statistical and machine learning-based, have been employed to detect anomalies. Methods like standard deviation, clustering algorithms, classification algorithms, and neural networks can be used depending on the nature and volume of the data.
- However, it’s essential to note that not all anomalies indicate problems. Some might be one-off occurrences or rare events that are still within the realm of possibility. Therefore, understanding the context and the domain in which the data resides is crucial when interpreting anomalies.
What is data quality? #
Data quality refers to the condition or state of data in terms of its suitability to serve its intended purpose in a given context. Good data quality means that the data is accurate, reliable, and fit for its intended uses in operations, decision-making, and planning.
On the other hand, poor data quality can lead to inaccurate insights, misguided strategies, and bad decision-making.
Data quality: Why does it matter? #
- Data quality is of paramount importance because poor quality data can lead to wrong decisions, inefficiencies, increased costs, and decreased trust in systems.
- Ensuring data quality often requires a combination of manual processes, automated tools, and policies to manage, cleanse, and validate data throughout its lifecycle.
- Organizations often implement data governance and stewardship programs to monitor and maintain the quality of their data over time.
The top 10 reasons why monitoring data anomalies and quality is critical #
Monitoring data anomalies and quality is integral for a variety of reasons, spanning from operational efficiency to strategic decision-making. Here’s a quick look at why these aspects are crucial:
- Informed decision-making
- Operational efficiency
- Trustworthiness
- Compliance and regulatory needs
- Risk management
- Enhanced customer experiences
- Cost savings
- Competitive advantage
- Enhanced data usability
- Support for advanced analytics and AI
Let’s understand each reason briefly.
1. Informed decision-making #
High-quality data ensures that decisions made by organizations or individuals are based on accurate, relevant, and timely information. When anomalies go unnoticed or data quality is poor, the resulting insights can be misleading. This can lead to strategic missteps, financial losses, and missed opportunities.
2. Operational efficiency #
Anomalies in data can sometimes indicate system malfunctions or errors in data entry processes. By promptly detecting these anomalies, businesses can identify and rectify inefficiencies, leading to smoother operations and reduced costs.
3. Trustworthiness #
Stakeholders, whether they are internal (like employees) or external (like customers or investors), place trust in data-driven organizations. If data quality is compromised, it erodes that trust, which can have wide-ranging implications, from decreased employee morale to reduced customer loyalty.
4. Compliance and regulatory needs #
Many industries have specific regulations around data quality and accuracy, especially in sectors like finance, healthcare, and real estate. Monitoring data for anomalies and ensuring its quality is paramount to adhering to these regulations and avoiding potential legal penalties.
5. Risk management #
Anomalies, especially in sectors like finance, can be indicative of fraud or malicious activities. Timely detection of these anomalies can help in proactively managing risks. Similarly, poor data quality can lead to erroneous risk assessments, which can expose businesses to unforeseen vulnerabilities.
6. Enhanced customer experiences #
In customer-centric industries, data quality plays a pivotal role in understanding customer behaviors, preferences, and needs. Poor data can lead to misinformed marketing strategies or customer service mishaps. Monitoring data quality ensures that interactions with customers are based on accurate and relevant information, leading to better customer experiences.
7. Cost savings #
Addressing issues arising from poor data quality after they’ve caused problems can be expensive. Monitoring allows for proactive management, reducing the potential costs associated with rectifying mistakes or addressing issues arising from poor-quality data.
8. Competitive advantage #
Organizations that ensure high data quality and actively monitor for anomalies often have a competitive edge. They can derive more accurate insights from their data, innovate faster, and respond more agilely to market changes.
9. Enhanced data usability #
High-quality data is easier to use and interpret. Analysts and data scientists can spend less time cleaning and preprocessing data and more time deriving actionable insights. This increases the productivity and effectiveness of data teams.
10. Support for advanced analytics and AI #
Advanced analytics, machine learning, and AI models are highly sensitive to data quality. Poor data can result in models that perform badly or offer incorrect predictions. Monitoring data quality is therefore crucial for organizations that rely on these advanced technologies.
In summary, monitoring data anomalies and quality is not just a technical necessity but a strategic imperative. The accuracy, reliability, and relevance of data directly influence an organization’s operational efficiency, decision-making capabilities, and overall success in today’s data-driven landscape.
Unknown impacts of poor data quality and data anomalies #
Data plays a pivotal role in contemporary organizations, driving decision-making and strategic planning. However, the benefits drawn from this data-centric approach are only as good as the quality of the data used.
So, what if we don’t monitor the quality and anomalies? Well, the poor data quality and data anomalies can significantly hinder an organization’s endeavors, leading to a range of adverse outcomes. Let’s delve into the impacts of both of these phenomena.
Impacts of poor data quality #
1. Misguided decision-making #
Poor data quality can lead to inaccurate insights and analyses. When decision-makers rely on this flawed information, it often results in decisions that do not align with the organization’s goals or market realities.
2. Operational inefficiencies #
Inaccurate or incomplete data can disrupt daily operations. This can manifest in various ways, from incorrect orders being shipped to wrong customer details leading to failed communications.
3. Decreased trust #
Stakeholders, be it employees, customers, or partners, expect data-driven organizations to possess accurate data. When they encounter discrepancies, their trust in the organization diminishes.
4. Increased costs #
Rectifying mistakes arising from poor data quality can be expensive. These costs may come in the form of operational hiccups, remedial actions, or even regulatory fines in regulated industries.
5. Compromised customer experience #
Inaccurate data can lead to misinformed marketing campaigns, billing errors, or improper customer service, all of which degrade the customer experience.
6. Regulatory non-compliance #
Industries such as finance, healthcare, and real estate often have stringent data regulations. Poor data quality can result in non-compliance, leading to penalties and reputational damage.
Impacts of data anomalies #
1. Masked real trends #
Anomalies can overshadow actual trends in data. This makes it challenging to derive genuine insights, leading organizations down incorrect strategic paths.
2. False alarms #
Not all anomalies indicate problems. Sometimes, they might be genuine one-off occurrences. However, if they’re treated as errors or threats, they could lead to unnecessary panic or wastage of resources.
3. Potential system malfunctions #
Data anomalies, especially in automated systems, can be symptoms of underlying system malfunctions or failures. If not addressed, these can escalate into larger operational issues.
4. Risk exposure #
Especially in sectors like finance, anomalies might be indicative of fraud or security breaches. If undetected, this exposes the organization to potential losses and risks.
5. Resource misallocation #
Addressing anomalies, especially those that are false positives, can divert resources from more pressing matters, leading to inefficiencies.
6. Impaired machine learning models #
For organizations that utilize machine learning or AI, anomalies can distort the training of models, leading to incorrect predictions or analyses.
In conclusion, while data promises immense potential for insights and efficiency, the presence of poor data quality and anomalies can significantly impede an organization’s progress. Understanding these impacts underscores the importance of rigorous data quality management and anomaly detection procedures.
Roadmaps to monitoring data anomaly and data quality #
The proliferation of data-driven decision-making in organizations necessitates the continuous monitoring of data quality and anomalies. Data quality ensures that the information used for analysis and decision-making is accurate, complete, and reliable.
On the other hand, monitoring data anomalies helps in detecting unusual patterns which might indicate issues or outliers. Both are vital components for ensuring the integrity and reliability of datasets. Here’s a detailed look at how one can monitor these aspects of data:
How to monitor data quality? #
1. Establish a data governance framework #
This involves creating a set of procedures, policies, and standards to manage and ensure the quality of data. It designates roles and responsibilities, setting clear expectations on who oversees various aspects of data quality.
Detail: A robust data governance framework will define data owners, data stewards, and detail the processes on data acquisition, storage, processing, and usage. This organized approach provides a foundation for all subsequent data quality monitoring efforts.
2. Implement data validation rules #
Create checks and rules to ensure the data entered or imported into systems is accurate and adheres to predefined standards.
Detail: These checks might involve format validations (e.g., email formats), range checks (e.g., age should be between 0-100), or referential integrity in databases.
3. Regularly audit the data #
Periodically review datasets to ensure they maintain their quality over time. This helps in identifying any deterioration in data quality at early stages.
Detail: Audits can be done through random sampling or using automated tools that scan datasets and report inconsistencies or errors.
4. Use automated data quality tools #
There are many tools available that can automate the process of monitoring data quality. These tools can detect issues like duplicates, missing values, and inconsistencies.
Detail: These tools operate autonomously, scanning datasets for anomalies within the information.
5. Feedback loops #
Establish mechanisms where end users can report data quality issues they encounter. This provides a real-world check and can be instrumental in catching issues that might be missed in automated processes.
Detail: This can be as simple as a reporting button or a dedicated communication channel where anomalies or inconsistencies in data can be flagged by users.
How to monitor data anomalies? #
1. Statistical analysis #
Techniques like the Z-score or the IQR (Interquartile Range) can help in identifying data points that deviate significantly from the norm.
Detail: For instance, a Z-score measures how many standard deviations a data point is from the mean. If it’s significantly high or low, that point can be considered an anomaly.
2. Visual inspection #
Plotting data on graphs can sometimes make anomalies visually evident. Scatter plots, time series plots, or box plots can be particularly useful.
Detail: For instance, a sudden spike in a time series plot might indicate an anomaly.
3. Machine learning #
Algorithms like Isolation Forest, One-Class SVM, or autoencoders can be trained to detect anomalies in large datasets.
Detail: Machine learning models can be trained on “normal” data, and then they can detect data points that deviate from this pattern as anomalies.
4. Threshold-based checks #
Set predefined limits or thresholds, and monitor the data for any values that cross these limits.
Detail: For example, in a temperature monitoring system, a sudden reading above 100°C might be considered anomalous and trigger an alert.
5. Real-time monitoring tools #
Use software solutions that monitor data streams in real-time for any unusual patterns or deviations.
Detail: Tools like Elasticsearch combined with Kibana, or specialized monitoring platforms, can continuously analyze data and alert users to potential anomalies as they occur.
In a nutshell, as organizations rely more heavily on data-driven insights, ensuring the fidelity of this data becomes paramount. By implementing structured monitoring processes for data quality and anomalies, businesses can make more informed decisions, reduce risks, and ensure operational efficiency.
Bottom line #
Effective monitoring of data quality and anomalies is essential for accurate decision-making, operational efficiency, risk management, customer satisfaction, cost savings, competitive advantage, and support for advanced analytics. By implementing structured monitoring processes and utilizing appropriate techniques, organizations can navigate the challenges of data anomalies and poor data quality, ensuring success in a data-driven landscape.
Data anomaly and quality monitoring: Related reads #
- Data Quality Explained: Causes, Detection, and Fixes
- Data Quality Metrics for Integrity & Consistency
- What is Anomaly Detection, and Why is It Significant for You?
- How to Improve Data Quality in 10 Actionable Steps?
- Data Integrity vs Data Quality: Nah, They Aren’t Same!
- 6 Reasons Why Data Quality Needs a Data Catalog
Share this article