Data Fabric vs Data Lake: What's Right for You and When?
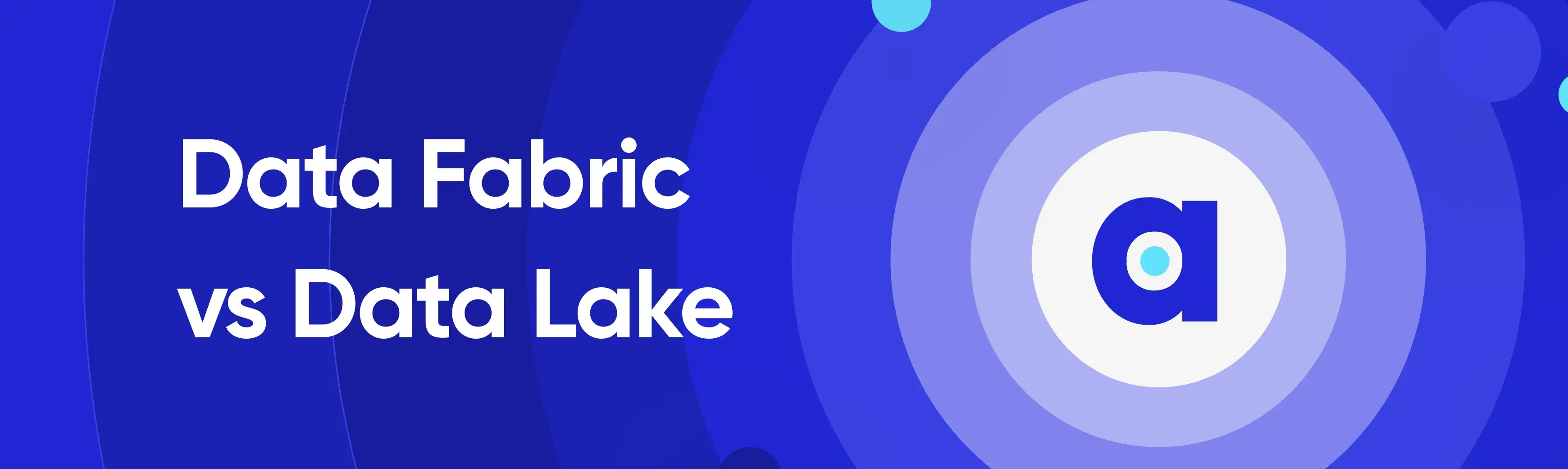
Share this article
A data lake is a large storage repository that holds raw data in its native format until it’s needed. It stores structured, semi-structured, and unstructured data, which makes it great for big data and real-time analytics. On the other hand, a data fabric is an architecture involving the management, integration, transformation, and governance of data.
In order to get value out of a data lake, it needs to be combined with other technologies such as data warehouses, data catalogs, and advanced analytics tools. Also, while data lakes can provide enormous flexibility and scalability, they can also pose challenges in data management, governance, and maintaining data quality.
A data fabric is an architecture and set of data services that provide consistent capabilities across a range of endpoints spanning both on-premise and multiple cloud environments. It can help in handling diverse data, supporting real-time and dynamic data-driven applications, and can also deal with privacy and security concerns.
Table of contents #
- Data fabric and data lake: Can they coexist together?
- How is data fabric different from data lake?
- Data fabric vs data lake: A tabular view
- Data maturity survey: Questions to ask to know what is best for you
- Data maturity survey: Acting on the insights
- Use cases for both data lake and data with practical examples
- Bringing it all together
- Data fabric vs data lake: Related reads
Data fabric and data lake: Can they coexist together? #
Yes, of course, they can. Both the data fabric and data lake serve different purposes and can coexist in a larger data ecosystem.
Let us take an example and understand this better.
Let us assume that your business is experiencing explosive growth. That means there is a lot of data generated from different business units. That’d also mean you’d need to provide timely data-driven insights to your teams.
So, here’s how you could do it:
- Step 1: Use a data lake to store the raw, granular data coming from different sources like web logs, transaction data, and user behavior data.
- Step 2: Then, using a data fabric, you could integrate data from this data lake and your existing databases
- Step 3: Apply the necessary transformations, enforce governance and security rules, and provide a unified view of data to your business users.
Besides, you’d need a data warehouse or a data mart for your analytical needs. This data warehouse can be part of your data fabric, pulling in data from the data lake and the database, transforming it into a suitable format, and serving it to your business users.
Remember that transitioning to a data fabric architecture would involve rethinking your data workflows and possibly changes to your data teams’ structure and skillsets. It’s important to have a clear roadmap and to make sure that your team is aligned with these changes.
How is data fabric different from data lake? #
Let us now understand the fundamental differences and relationship between data lakes and data fabrics.
What is data lake? #
A data lake is a storage system or repository that holds an enormous amount of raw data in its native format until it’s needed.
This is especially useful when dealing with big data, as it lets you collect data from different sources and analyze it later using various tools. Data lakes are suitable for machine learning, data discovery, and data exploration.
But, since data isn’t transformed before it’s stored in a data lake, it can lack the structure that can make data retrieval and analysis more complex. This is often referred to as a “data swamp.”
What is data fabric? #
Data fabric isn’t a storage system, but rather a way of managing and integrating data across different environments (which could include data lakes, data warehouses, databases, and even real-time data streams).
It allows data to be accessed, shared and managed across distributed architectures, and it can deal with different types of data (structured, semi-structured, and unstructured).
The interrelationship between a data lake and a data fabric #
The relationship between a data lake and a data fabric is that they can be complementary parts of a data strategy. You can have a data fabric that manages data across several storage systems, one of which could be a data lake.
When should you consider a data lake and a data fabric? #
A data lake is a good option when your organization has vast amounts of raw data that it wants to store for future exploration, big data analytics, or machine learning purposes. They’re useful when you aren’t yet sure what insights or use cases you might want to extract from the data.
On the other hand, a data fabric is important when you have a complex data landscape that spans multiple systems, platforms, and locations. It can be helpful when you’re looking for ways to manage, integrate, and make data accessible in a unified manner.
Data fabric vs data lake: A tabular view #
Now, let us understand their relationship and differences in a table, so that it is easy to process it.
Data Lake | Data Fabric | |
---|---|---|
Nature | Storage System | Architecture & Set of Data Services |
Data | Holds raw data (structured, semi-structured, unstructured) in its native format | Manages, integrates and transforms data across different environments (Data Lakes, Data Warehouses, Databases, Real-Time Data Streams) |
Purpose | Designed for storing large volumes of raw data for later analysis | Designed to manage and unify data across various platforms, systems and locations |
Used for | Big data analytics, data exploration, machine learning | Real-time data access, data integration, data governance, privacy and security |
Challenges | Risk of becoming a 'Data Swamp' if not managed properly | Complexity in managing diverse types of data across multiple platforms |
When to consider | When you have vast amounts of raw data that you want to store for future use | When you need to manage and integrate data across complex, distributed architectures |
Relationship with other | Can be one of the components managed by a Data Fabric | Can manage and integrate data from a Data Lake as part of a larger data landscape |
Remember that the above differences and relationships are generalized. In specific cases, the exact nature of these systems could vary based on the specific use cases, technologies used, and the way these systems are implemented.
Data maturity survey: Questions to ask to know what is best for you #
Now, which approach is best for you depends on how mature your organization is while dealing with data. You can determine it using a data maturity survey. Performing a data maturity survey is a great first step towards identifying which approach would be more beneficial for your organization.
Here are some key questions that you could ask to understand your organization’s data maturity:
1. Data collection and storage #
- What types of data are we currently collecting (structured, unstructured, semi-structured)?
- What volume of data does the organization handle?
- How do we store and manage our data?
- Is our data storage scalable and flexible?
2. Data quality and governance #
- How do we ensure the quality and accuracy of the data we collect?
- Do we have data governance policies in place?
- How do we handle data privacy and security?
3. Data integration and accessibility #
- How is data integrated from different sources within the organization?
- How accessible is the data to the teams that need it?
- How are changes to data structures and schemas handled?
4. Data usage and analytics #
- How often is data used in decision-making processes?
- How advanced are the data analytics capabilities (descriptive, predictive, prescriptive)?
- Do we use data for machine learning or AI initiatives?
5. Data culture #
- Is there a data-driven culture within the organization?
- Are employees encouraged and trained to use data in their roles?
- How is data literacy across different teams and roles?
6. Data strategy and future goals #
- Is there a clear data strategy in the organization?
- How is the organization planning to evolve its data capabilities?
- What are the future data needs and objectives of the organization?
Your responses to these questions can help you understand your organization’s data maturity and can guide your decision between a data lake, a data fabric, or some other data architecture.
In the next section, we will see how you can use the insights from your survey to make key decisions.
Data maturity survey: Acting on the insights #
The insights you gather from the data maturity survey will help you understand your organization’s current data capabilities, culture, and needs. Based on these insights, you can align the choice of a data infrastructure approach to your current state and future goals.
Here’s how the insights can guide your decision:
1. Data collection and storage #
If your organization collects a large volume of diverse data, a Data Lake could be beneficial for storing this raw data. If your data is already stored across different systems and environments, a Data Fabric could help integrate and manage this data.
2. Data quality and governance #
If you have strong data governance and quality assurance practices, you’re likely ready for a more advanced system like a Data Fabric. If not, you may need to start with building a more structured storage system like a Data Lake and simultaneously work on improving data governance.
3. Data integration and accessibility #
If your data is siloed and hard to access for decision-making, a Data Fabric can help by providing a unified view of data. If your data is already integrated and accessible, you might only need a Data Lake for storing raw data.
4. Data usage and analytics #
If your organization frequently uses data for decision-making, especially with advanced analytics, AI, or machine learning, a Data Lake can provide the raw, detailed data needed for these activities. A Data Fabric can further enhance this by providing real-time access and integration.
5. Data culture #
If your organization has a data-driven culture with high data literacy, you might be ready to adopt a Data Fabric, which requires a good understanding of data management principles. If not, starting with a Data Lake could be more beneficial while you work on building a stronger data culture.
6. Data strategy and future goals #
Your future goals will significantly influence your decision. If your organization aims to have a flexible, real-time, integrated view of data across all systems and environments, a data fabric would align with this goal. If your goal is to enhance storage and analysis of big data, a Data Lake could be the right choice.
Many organizations adopt both a data lake and data fabric as part of their data strategy, using each for its strengths. You could start with a data lake and build towards a data fabric as your data maturity increases.
Use cases for both data lake and data with practical examples #
Now, let us look at some practical use cases that you can drive with a data lake and a data fabric:
Data lake use cases #
Here are a few use cases where you could use a data lake.
Advanced analytics and machine learning #
A financial institution collects vast amounts of structured and unstructured data. They could store all their raw data in a data lake and run advanced analytics and machine learning algorithms on this data to predict stock trends, identify fraud patterns, or personalize banking services.
IoT sensor data analysis #
A manufacturing company with thousands of IoT sensors on their machinery could store the high volume of sensor data in a data lake. Data scientists could then analyze this data to predict equipment failures, optimize maintenance schedules, and improve operational efficiency.
Customer behavior analysis #
An e-commerce company could store detailed clickstream data in a data lake. This data could be used to understand customer behavior, optimize website design, personalize product recommendations, or A/B test different strategies.
Data fabric use cases #
Here are a few use cases where you could use a data fabric.
Real-time business intelligence #
A retail chain has data spread across multiple systems - sales databases, inventory systems, online e-commerce platform, and customer feedback forms. They could use a data fabric to integrate data from all these sources and provide real-time dashboards to their business users. This could help in making timely decisions like restocking inventory, adjusting pricing, or identifying trending products.
Unified customer view #
A telecom company could use a data fabric to combine data from their customer databases, call detail records, network performance logs, and customer service systems. This could provide a unified view of each customer’s experience, which could be used to enhance service, reduce churn, and personalize offerings.
Regulatory compliance and reporting #
A pharmaceutical company could use a data fabric to manage their diverse data - research data, clinical trial results, patient data, manufacturing data, and sales data. The data fabric could enforce data governance rules, ensure data privacy, and automate the generation of regulatory reports, thus simplifying compliance with regulations like HIPAA or GDPR.
The above use cases are simplified examples. In the real-world, actual use cases could be more complex and might require additional technologies or systems.
Besides, a data lake and a data fabric are not mutually exclusive, and many use cases could involve both. For example, raw data from IoT sensors could be stored in a data lake and then integrated with other data via a data fabric for real-time analysis and decision-making.
Bringing it all together #
A data lake is essentially a storage repository for raw data in its native format, while a Data Fabric is an architecture and a set of data services that provide consistent capabilities across various endpoints, spanning on-premise and multiple cloud environments.
A data lake can be highly beneficial for storing and analyzing big data and running advanced analytics and machine learning. But, a data fabric can help in providing real-time insights, integrating data from various sources, and managing data governance and privacy.
Ultimately, your choice isn’t binary and depends on your current data maturity and future data strategy. You could start with a data lake and move towards a data fabric as your data maturity increases.
Data fabric vs data lake: Related reads #
- What Is a Data Lake? Definition, Architecture & Solutions
- What is Data Fabric: Components, Use Cases & More
- What Is a Data Lake and Why Does It Need a Data Catalog?
- Data Warehouse vs Data Lake vs Data Lakehouse
- Data Mesh vs. Data Lake — Differences & Use Cases
- Data Fabric Explained Like You Know Nothing
- Implementing a Data Fabric: A Scalable and Secure Solution for Maximizing the Value of Your Data
- Data Fabric Architecture: Components, Tools, and More
Share this article