9 Essential Data Lake Use Cases You Must Know
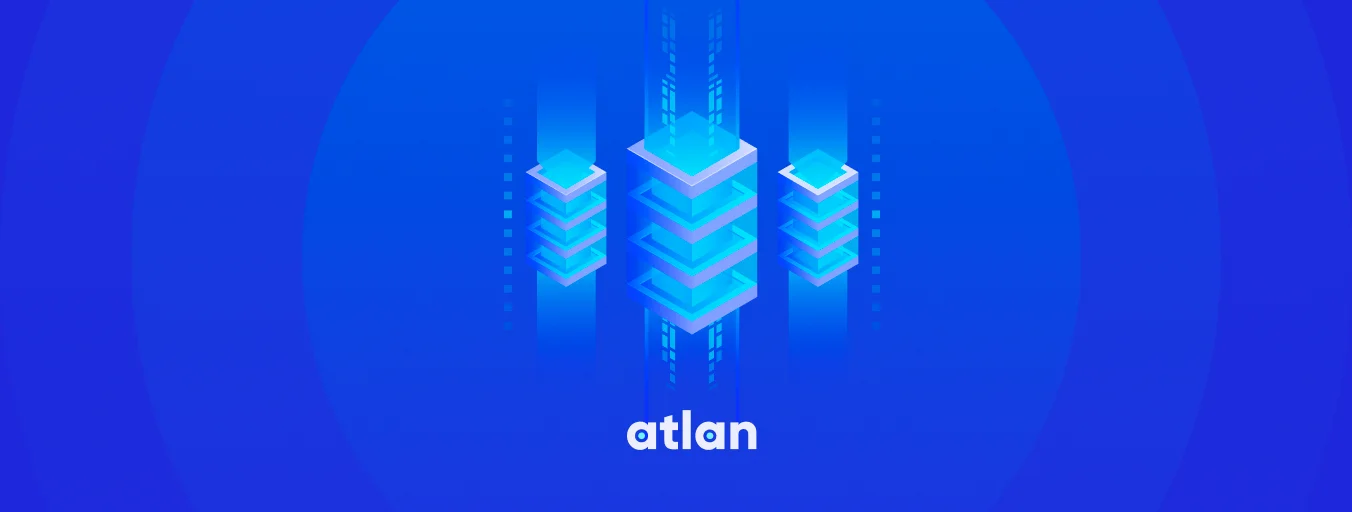
Share this article
As organizations collect more and more data, traditional databases can become overwhelmed, leading to performance issues. Data lake use cases provide the ability to efficiently and cost-effectively manage and analyze vast volumes of diverse data from various sources.
In a nutshell, data lakes offer a powerful solution to address the challenges associated with managing and deriving insights from large and diverse datasets. They empower organizations to overcome the limitations of traditional data storage and processing systems, leading to more informed decision-making and better competitive positioning.
Modern data problems require modern solutions - Try Atlan, the data catalog of choice for forward-looking data teams! 👉 Book your demo today
In this article, we delve deep into the world of data lakes, breaking down their essence, advantages, and diverse data lake use cases.
Let’s dive in!
Table of contents #
- What is a data lake?
- Data lake use cases
- Challenges and considerations
- Real-time examples
- Data lake in comparison
- To summarize
- Related reads
What is a data lake? #
A data lake is a centralized storage system where you can store vast amounts of raw data. This data can come from different sources and can be in various formats - like text, images, or videos.
Think of it as a big virtual pond where all your data flows into and gets stored in its natural, raw state, waiting to be analyzed.
4 Key advantages of data lakes #
In today’s business landscape, data is more than just numbers and facts; it’s the foundation for informed decisions, strategies, and growth.
As we amass increasing amounts of data, it’s crucial to have a system that can manage and utilize it effectively. This is where data lakes come into play. The key advantages are:
- Scalability
- Flexibility
- Cost-effectiveness
- Advanced Analytics
Let’s delve into the core advantages of data lakes that make them an attractive solution for businesses:
1. Scalability: Handling vast amounts of data of all kinds #
Imagine your business’s data as water. In the beginning, you might only have a bucketful, easy to manage and store. But as your business grows, so does the amount of water, and soon you’ll need a pond, then a lake to store it all.
A data lake acts as this expansive storage space, designed to handle enormous volumes of data, whether it’s unstructured (like emails), semi-structured (like spreadsheets), or structured (like databases).
No matter how much data your business accumulates, a data lake can scale to accommodate it, ensuring you never run out of room.
2. Flexibility: Adapting to various data sources and types #
Different businesses have different types of data. A retail store might gather data from sales transactions, while a hospital collects patient records, and a tech company accumulates user data.
A data lake’s beauty lies in its ability to accept data from a wide array of sources without needing to format it first. Whether you’re feeding it sales figures, medical histories, or user interactions, a data lake can handle it all without flinching.
3. Cost-effectiveness: Leveraging affordable technologies #
Starting a business is expensive, and as decision-makers, you’re always on the lookout for ways to cut costs without compromising quality. One of the significant advantages of data lakes is that they often rely on open-source technologies. This means you’re not paying hefty fees for proprietary software.
Additionally, with the rise of cloud storage solutions, data lakes can be hosted on the cloud, eliminating the need for expensive on-site hardware and reducing maintenance costs. In short, you get top-tier data management without the top-tier price tag.
4. Advanced analytics: Tapping into deeper insights #
Now, having vast amounts of data is great, but what’s even more essential is the ability to analyze and derive insights from it. Data lakes come equipped with the capability to integrate with powerful analytic tools.
This means you can sift through your data, identify patterns, and gain insights that might have otherwise gone unnoticed. For a business owner, this translates to understanding your customers better, optimizing operations, and foreseeing market trends, giving you a competitive edge.
For business owners and decision-makers, understanding the value of data is crucial. By leveraging the advantages of data lakes, businesses can ensure they’re not just collecting data, but harnessing it to drive growth, efficiency, and innovation.
With scalability, flexibility, cost-effectiveness, and advanced analytics on its side, a data lake is more than just a storage system—it’s a strategic asset.
9 Data lake use cases #
Data lakes are transforming the way businesses store, analyze, and leverage their vast pools of information. To illustrate their versatility, here are nine data lake use cases that showcase their potential in various sectors and applications. They are:
- Advanced analytics and machine learning
- IoT (Internet of Things) data storage and analysis
- Data exploration and research
- Centralized data repository
- Real-time dashboarding and reporting
- Data archive and data lake-as-a-service
- Data lake use cases in insurance
- Data lake use cases in banking
- Data lake in manufacturing
Let’s understand these use cases in detail.
1. Advanced analytics and machine learning #
One of the most compelling reasons businesses are turning to data lakes is the potential for advanced analytics and machine learning.
- Predictive analytics
This involves analyzing current and historical data to forecast future events. For instance, a retail business might use past sales data to predict which items will be in demand next season.
By having a clear forecast, businesses can manage inventory, plan marketing strategies, and make informed decisions about future investments.
- Personalized customer experiences
With machine learning, companies can study individual customer behaviors and preferences. This means they can offer tailor-made recommendations or services, enhancing the customer experience.
For example, streaming platforms suggest shows based on viewers’ past choices.
- Natural language processing (NLP) for sentiment analysis:
Imagine knowing how your customers feel about your product without conducting a survey. With sentiment analysis, businesses can scan online reviews, social media mentions, and other digital feedback to gauge customer sentiments. It’s like having a pulse on customer feelings in real-time.
2. IoT (Internet of Things) data storage and analysis #
In our increasingly connected world, everyday objects, from fridges to street lamps, are becoming “smart”.
- Handling high-velocity and high-volume data streams
Smart devices send vast amounts of data constantly. Data lakes can capture this flow, offering a place to store, manage, and analyze it.
- Analyzing patterns for smart devices and cities
Data from these devices can show patterns. For instance, city planners could use traffic light data to manage congestion, or energy companies might analyze smart meter data to predict usage spikes.
3. Data exploration and research #
Delving into vast amounts of data can uncover hidden gems of information.
- Real-time data exploration for businesses
With a data lake, businesses can instantly dive into their data, searching for insights or patterns. It’s like having a powerful magnifying glass for your business data.
- Academic and scientific research use cases
Universities and research institutions can store vast amounts of research data in one place, making it easier to conduct groundbreaking studies.
4. Centralized data repository #
Having data scattered in different places is not only inefficient but can lead to missed opportunities.
- Integrating data from different business units for a holistic view
By pooling data from various departments into one place, companies can see the bigger picture. This central overview can lead to better decision-making and more cohesive strategies.
- Historical data storage and archiving
Storing past data is essential for trend analysis, compliance, and more. With a data lake, businesses can keep years of data accessible and organized.
5. Real-time dashboarding and reporting #
Immediate insights are crucial in our fast-paced business environment.
- Aggregating data from various sources for real-time insights
With data lakes, companies can pull data from multiple sources and display it in easy-to-understand dashboards. These live updates can help decision-makers respond to changing situations swiftly.
- Business intelligence (BI) integration for visualization
By integrating with BI tools, data lakes can turn complex data into visual reports, making it easier for stakeholders to understand and act on.
6. Data archive and data lake-as-a-service #
Even if businesses don’t need their data now, they might in the future.
- Long-term data retention for compliance
Some industries need to keep records for years due to regulatory requirements. Data lakes offer a secure and efficient way to meet these demands.
- Offering data lake services to third-party organizations
As more companies see the value in data lakes, there’s a growing market for providing data lake services. This offers a new revenue stream for those equipped to provide these services.
7. Data lake use cases in insurance #
- Enhanced customer profiling
Data lakes allow insurers to compile comprehensive customer profiles by integrating data from various sources. This holistic view enables better risk assessment, personalized policy offerings, and improved customer service.
- Efficient claims processing
When a customer submits a claim, there’s a lot of background work involved. Verification, cross-referencing with policy details, and more.
Data lakes can simplify this process by consolidating all the necessary information in one place, ensuring quicker and more accurate claim settlements.
- Predictive analytics for risk management
Data lakes can store vast amounts of historical data. By analyzing past events, trends, and patterns, insurers can better predict future risks.
For example, by analyzing past car accident data in a particular region, an insurer can better assess the risks of providing auto insurance in that area.
- Real-time fraud detection
Unfortunately, insurance fraud is a costly problem. By pooling data from various sources in a data lake and analyzing it in real-time, insurers can quickly spot suspicious activities or inconsistencies, leading to faster fraud detection and prevention.
- Streamlined regulatory compliance
The insurance industry is often subject to strict regulations. Data lakes can help insurers maintain comprehensive records, ensuring that they are always ready for audits and in compliance with industry standards.
8. Data lake use cases in banking #
- Enhanced customer experiences
Banks are collecting more data about their customers than ever before. From transaction histories to online interactions, every piece of data is valuable.
With a data lake, banks can analyze this data comprehensively to gain insights into individual customer behaviors and preferences.
As a result, they can tailor their services, like offering personalized loan rates or recommending specific banking products, to suit individual needs.
- Fraud detection and prevention
Fraud is a significant concern in banking. By analyzing patterns and anomalies in vast amounts of transaction data stored in data lakes, banks can identify and flag potentially suspicious activities in real-time.
This swift response can prevent unauthorized transactions and protect customers’ hard-earned money.
- Streamlined operations
With multiple departments and operations, banks often grapple with scattered and siloed data. Data lakes help centralize this data, allowing for smoother cross-departmental collaboration and more efficient operational decisions.
- Regulatory compliance and reporting
Banks are subject to stringent regulations. They are required to maintain records and generate periodic reports for compliance. Data lakes simplify this process by providing a centralized location for all required data, making it easier to retrieve and compile necessary information.
9. Data lake use cases in manufacturing #
- Enhancing production efficiency
One of the primary goals for any manufacturer is to ensure that their production lines run efficiently. With a data lake, you can gather information from different stages of the production process and analyze it in one unified space.
This could include data from machinery sensors, worker productivity metrics, or even environmental factors. By studying this data, manufacturers can identify bottlenecks, optimize processes, and improve overall production efficiency.
- Improved inventory management
Managing inventory is a delicate balancing act. Overstocking can lead to increased holding costs, while understocking can result in missed sales opportunities. Data lakes can store vast amounts of sales, procurement, and inventory data.By analyzing these, manufacturers can get insights into demand patterns, optimize stock levels, and reduce wastage, leading to more profitable operations.
- Predictive maintenance
Machinery breakdown can be a costly affair, not just in terms of repair costs but also in the potential halt it can cause in production. Through the data stored in a data lake, manufacturers can track the performance and health of machinery over time.This allows them to predict when a machine might break down or need maintenance, ensuring timely intervention and minimizing disruptions.
- Enhancing product quality
Data lakes can store quality check data from various stages of production. By analyzing this data, manufacturers can identify which stages or batches have quality issues and take corrective measures.
This ensures that the final product meets the desired standards, leading to increased customer satisfaction and reduced return rates.
- Supply chain optimization
For manufacturers, the supply chain is a critical component that can heavily influence costs and delivery times. Data lakes can house data from suppliers, transporters, and other partners.
Analyzing this data can offer insights into potential delays, cost overruns, or inefficiencies, allowing manufacturers to optimize their supply chains for better performance and profitability.
Challenges and considerations in data lake use cases #
In the world of data, data lakes offer promising solutions. However, as with any technology, there are challenges to be aware of. For business owners and decision-makers considering implementing a data lake, it’s essential to understand these challenges.
Being informed will help in making strategic choices and maximizing the benefits of the data lake. Here are some key challenges and considerations to be aware of:
1. Data security and privacy concerns #
The first and foremost concern for any business is ensuring the safety and privacy of its data. With the increasing number of data breaches reported worldwide, it’s more important than ever to ensure that the data you collect, store, and analyze is secure.
In a data lake, where vast amounts of data from various sources come together, ensuring robust security measures becomes a challenge. It’s crucial to implement advanced encryption techniques, access controls, and regular audits to ensure data remains safe.
2. Ensuring data quality and avoiding a “data swamp” #
Data lakes can store immense amounts of data. However, simply dumping all the data into a data lake can lead to what many terms a “data swamp” - a place where data is messy, unorganized, and of little value.
To avoid this, it’s vital to maintain the quality of the data that goes into the data lake. This means regularly cleaning, refining, and updating the data. Having a clear strategy for data intake, processing, and maintenance is crucial to avoid turning your data lake into a data swamp.
3. Managing metadata and ensuring discoverability #
In simple terms, metadata is data about data. For instance, metadata can tell you when a particular set of data was collected, its source, and how it has been processed. Managing metadata is essential in a data lake because it ensures that users can find the data they need quickly.
Without proper metadata management, a data lake can become a vast ocean where finding the right data becomes nearly impossible. Properly tagging, categorizing, and indexing data will ensure that it’s easily discoverable.
4. The importance of governance and management #
A data lake without governance is like a city without laws. Governance refers to the set of rules, policies, and standards that guide how data is collected, stored, accessed, and used. Implementing a strong governance framework ensures that the data in the data lake is consistent, reliable, and used responsibly.
Regular reviews, updates to policies, and staff training are essential components of data governance. Proper management ensures that the data lake remains an asset and doesn’t turn into a liability.
While data lakes offer a myriad of opportunities for businesses, it’s crucial to approach them with an understanding of the associated challenges. Being proactive in addressing these challenges ensures that your data lake remains a valuable resource for your business.
3 Case studies: Real-time examples of data lakes #
Data lakes, while technical in nature, have found practical applications in various industries, making a significant difference in how organizations operate. By looking at real-world implementations, we can gain a clearer understanding of the tangible benefits and potential of data lakes.
Here are three case studies of data lakes from different industries, showcasing their approaches and outcomes:
1. Coca-Cola Andina: #
- Built a data lake on AWS which led to an 80% increase in analytics team productivity.
- Unified 95% of the data from different business areas into a single data lake.
- Adopted AI, machine learning, and other advanced capabilities, enabling data-driven decision-making and increased profitability.
2. A multinational energy corporation: #
- Faced challenges due to data spread across various systems and lacked a singular view of enterprise data.
- Implemented a cloud data lake to integrate data from over 140 countries, supporting various data types and structures.
- Achieved comprehensive enterprise data visibility, operational excellence, and robust data governance, resulting in effective enterprise-wide data management.
Want to know more? Check this out → Multinational Energy Corporation Case Study by Emtec Digital
3. TTEC and Neal Analytics: #
- Built the Humanify Insights Platform, a cloud-based data platform, to unify customer data on Azure.
- Enhanced the end-to-end customer experience with AI-powered insights, providing a 360-degree view of the customer.
- Resulted in a 5-10% increase in sales and a 20% reduction in customer churn, demonstrating the effectiveness of their unified data ecosystem.
The case studies of Coca-Cola Andina, a multinational energy corporation, and TTEC with Neal Analytics showcase the effective use of data lakes to enhance analytics productivity, streamline data visibility, and improve customer experience, leading to increased sales and operational efficiency across diverse industries.
Data lake in comparison to traditional databases and data warehouses #
To better understand the concept of a data lake, it’s useful to compare it with traditional databases and data warehouses, which you might be more familiar with.
1. Nature of data #
Traditional databases usually store structured data. This means the data is organized, much like an Excel spreadsheet with rows and columns.
On the other hand, data warehouses can handle a bit more variety but often require data to be processed before storing. A data lake, however, accepts every type of data in its raw form.
2. Flexibility #
Databases and data warehouses often need a defined structure or schema to store data. If you want to change this structure later on, it can be quite challenging.
Data lakes offer more flexibility, allowing you to store the data now and decide how to use or analyze it later.
3. Cost #
Traditional databases and data warehouses can sometimes be expensive to set up and maintain, especially if you’re dealing with vast amounts of data.
Data lakes, often built on more modern and open-source technologies, can provide a more cost-effective solution.
4. Purpose #
Traditional databases are excellent for specific, ongoing tasks like managing customer information or transaction records. Data warehouses are built to process and report on large datasets.
However, a data lake is your best bet if you want a system that simply stores vast amounts of varied data for potential future analysis.
A data lake is a modern solution for businesses that deal with massive and varied amounts of data. While traditional databases and data warehouses have their place, a data lake offers versatility and scalability that can be crucial for businesses looking to harness the power of their data effectively.
To summarize #
A data lake is a modern solution for storing vast amounts of diverse data, offering businesses flexibility, cost savings, and advanced analytical capabilities. From enhancing customer experiences through predictive analytics to streamlining operations with real-time dashboards, its applications are vast and transformative.
However, while the benefits are clear, it’s vital to address potential challenges like data security and effective management. Leading companies are already harnessing the power of data lakes to gain insights and stay ahead. For business owners and decision-makers, understanding and integrating data lakes can be a strategic move towards informed, data-driven decision-making.
Data lake use cases: Related articles #
- What Is a Data Lake? Definition, Architecture, and Solutions
- Data Lake & Data Governance: Unifying Disparate Data Sources for Business Success
- What is a Data Lake in the Cloud? A 2023 Guide
- Data Mesh Vs. Data Lake: Differences & Use Cases For 2023
- Data Lake vs. Data Warehouse: 7 Key Differences!
- Data Mart vs Data Lake: What’s Right for Your Team in 2023?
Share this article