Data Swamp: Is It Sinking You In?
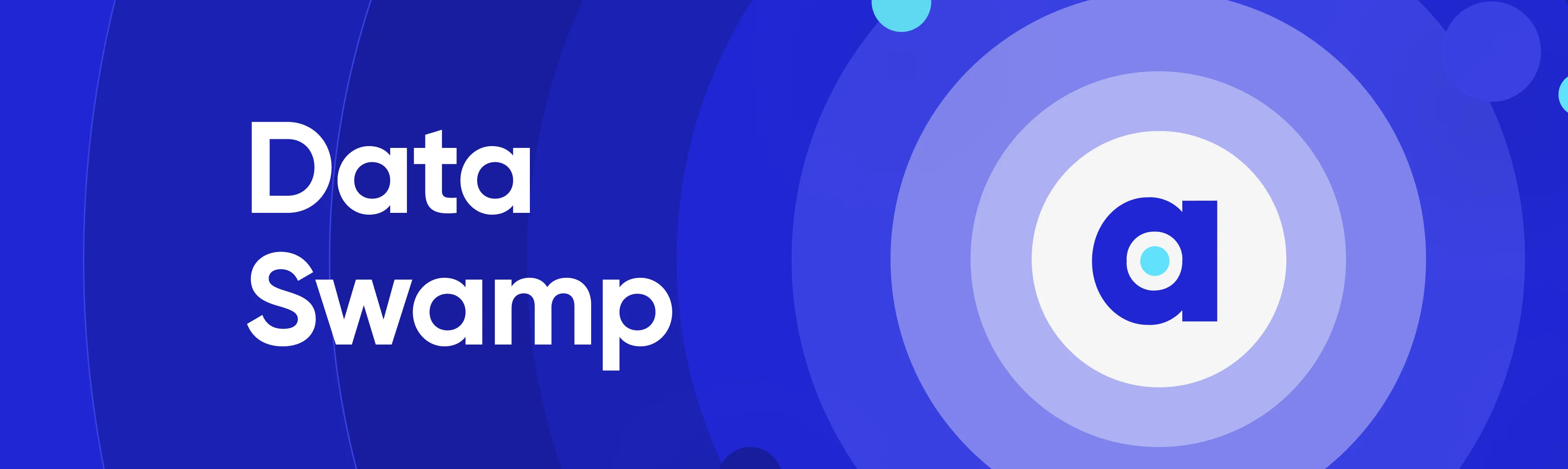
Share this article
A data swamp is a deteriorated and unmanageable data lake. When a data lake becomes unorganized, cluttered, and difficult to navigate due to the lack of proper data management practices, it is called a data swamp.
While data lakes are designed to store vast amounts of raw data in their natural form to be processed and structured as needed, they can become data swamps when data is stored without proper organization or metadata.
In a data swamp, data scientists and other users who need to access the data, spend considerable time on tasks such as data discovery, cleaning, integration, and management.
They need to find the relevant datasets, ensure the data is clean and usable, integrate the data into their work, and manage the overall data storage system. When these tasks are properly addressed, finding and utilizing the data becomes manageable.
Modern data problems require modern solutions - Try Atlan, the data catalog of choice for forward-looking data teams! 👉 Book your demo today
In this article, we will explore:
- The emergence of the term data swamp
- Differences between data swamp and data lake
- The issues that convert data lake into data swamp
- Impact of data swamp on an organization
- Ways to prevent data swamp
Ready? Let’s dive in!
Table of contents #
- How did the term ‘data swamp’ emerge?
- Data swamp example: When can a data lake turn into a data swamp?
- What is the difference between a data lake and a data swamp?
- What are the problems that turn data lake into a data swamp?
- What is the impact of data swamp on organizations?
- How can you prevent data swamping?
- Rounding it all up together
- Related reads
How did the term ‘data swamp’ emerge? #
The term ‘data swamp’ is relatively recent and emerged as an extension of the ‘data lake’ metaphor. While there isn’t a single accepted origin or an individual who is universally credited with coining the term, it generally appears in industry discussions. It came into literature as a way to describe the challenges that have arisen with the advent and management of data lakes.
The term “data lake” itself was first introduced around 2010 by James Dixon, CTO of the software company Pentaho. Dixon used the metaphor of a data lake to describe a large storage repository that holds a vast amount of raw data in its native format until it’s needed.
This concept was an answer to data silos and provided an efficient way to store unstructured, semi-structured, and structured data.
However, as the adoption of data lakes grew, organizations began facing issues with :
- Data governance
- Data quality
- Data security
- A lack of metadata
All of this made data difficult to find, understand, or use effectively. These poorly managed and governed data lakes became referred to as “data swamps”. It illustrates the problems when the volume, variety, and velocity of data outpace an organization’s ability to make that data useful and accessible.
The term “data swamp” gained prominence as more businesses and organizations adopted big data strategies and began grappling with the challenges of managing their vast stores of data. The discussions about these problems in industry forums, blogs, and research papers brought the term into broader use.
The main issue with a data swamp is that while the data is stored, it’s not usable for discovery or analysis until it’s processed. The data can be so disorganized and unmanageable that automated tools struggle to provide useful insights, making it difficult for data scientists and other end-users to extract value from the data.
This lack of organization and structure often results in significant delays and inefficiencies in data analysis and decision-making processes.
The concept of a data swamp underscores the need for robust data management strategies, including data cataloging, metadata management, data governance, and data cleaning, to ensure that the potential value of data lakes doesn’t degrade into an inaccessible, unmanageable data swamp.
Data swamp example: When can a data lake turn into a data swamp? #
The concept of a “data swamp” is often used to describe a data environment where the management, governance, and quality of data are either lacking or entirely absent. Unlike a “data lake,” which is a well-organized and easily navigable repository of raw data, a data swamp is chaotic and challenging to navigate.
Let us look at an example of a retail company’s data swamp.
The scenario
Let’s consider a mid-sized retail company named “RetailGenius” that started collecting data five years ago. Initially, the company maintained a clean data lake designed to centralize all of its data, including customer transactions, inventory, web analytics, social media interactions, and sensor data from its brick-and-mortar stores.
They hoped to use this data to derive insights on customer behavior, optimize stock levels, and even predict sales trends.
Initial steps
In the beginning, a small data team was in charge of importing data into the data lake. They followed a basic schema, tagged the datasets, and documented what each dataset contained. ETL (Extract, Transform, Load) processes were set up to ingest new data at regular intervals.
Transition to a data swamp
Over time, things started to fall apart for several reasons, such as:
-
Lack of data governance 👉 With company growth, more departments and teams started dumping data into the data lake without any standardized format or metadata.
-
Inconsistent data 👉 Over time, the ETL processes broke or became outdated, leading to duplicate or incorrect data being loaded.
-
Complex, unmaintained code 👉 The original data team moved on to different projects, and the new team didn’t fully understand the existing data ingestion and transformation codebase.
-
Lack of documentation 👉 Metadata, the “data about data,” was not kept up-to-date, making it difficult to understand the context, quality, or even the relevance of datasets.
-
No version control 👉 Old and new data were mixed together without any version control, making it difficult to track changes over time.
So, what are the symptoms that led to a data swamp?
-
Poor data quality 👉 Sales teams found discrepancies in sales reports. Analysts found different numbers for the same KPIs, depending on which datasets they used.
-
Unreliable insights 👉 Machine learning models that were initially built to forecast stock levels started to produce increasingly inaccurate predictions because they were trained on low-quality data.
-
Inefficiency 👉 Data scientists spent more time cleaning and finding the right data than doing actual analysis or modeling
-
Compliance risks 👉 The company was not sure if all the data it stored complied with data protection regulations like GDPR or CCPA because data lineage was not clearly mapped.
And, what are the technical complications?
-
Schema drift 👉 Datasets that were initially well-structured now have additional fields or missing fields, causing schema drift issues.
-
High cardinality and skew 👉 Data skew, especially in transactional data, made it difficult to partition data effectively, leading to inefficient data processing.
-
Orphaned datasets 👉 Numerous datasets had no “owners,” and it was unclear what processes generated them or who used them.
-
Data silos 👉 Although the intention was to break down data silos by having a data lake, the swampy nature of the environment created ‘virtual silos’ where only specific teams understood or had access to particular subsets of data.
RetailGenius’s data lake turned into a data swamp due to a lack of data governance, data quality, and effective management. Turning this swamp back into a lake—or even better, into a well-managed, secure, and compliant data environment—would require a significant investment in time, resources, and tooling.
But first, the company must recognize the severity of the situation; otherwise, the data swamp will continue to inhibit business agility, introduce risk, and increase operational costs.
What is the difference between a data lake and a data swamp? #
The comparison table outlining the key differences between a data lake and a data swamp:
Aspect | Data lake | Data swamp |
---|---|---|
Data organization | Data is organized, cataloged, and easily accessible. | Data is disorganized, uncataloged, and hard to access. |
Data quality | High-quality data that's clean and well-maintained. | Poor data quality due to lack of cleaning and maintenance. |
Metadata | Rich metadata exists to help with data discovery. | Insufficient or missing metadata, making data discovery hard. |
Data governance | Strong data governance strategies are in place. | Weak or non-existent data governance strategies. |
Accessibility | Data is accessible and easy to find and use. | Data is inaccessible and difficult to find and use. |
Usability | Useful for deriving insights and decision-making. | Difficult to derive insights or make decisions due to chaos. |
Security and compliance | Strong security measures and compliance controls. | Lax security and compliance measures leading to risks. |
User efficiency | Users can focus on analysis and gaining insights. | Users spend much time on data discovery, cleaning, and management. |
Data integration | Data can be integrated effectively for analytics. | Difficulty in integrating data for analytics due to poor organization. |
These differences are important considerations for data practitioners in their data management practices. They underline the need for proper data organization, quality control, governance, and security in maintaining a functional data lake. It prevents it from devolving into a data swamp.
What are the problems that turn data lake into a data swamp? #
The main reasons that can lead to the formation of data swamps:
- Lack of data governance
- Inconsistent data formats
- Lack of metadata
- Insufficient access controls and security
- Data volume, velocity, and variety
- Lack of maintenance and upkeep
Let us delve into each of the above reasons one by one:
1. Lack of data governance #
Data governance involves the overall management of the availability, usability, integrity, and security of data used in an enterprise.
- It includes establishing processes, responsibilities, and guidelines for data use and management.
- Without strong data governance, a data lake can quickly turn into a data swamp.
For example, if there are no clear guidelines for how data should be entered into a system, different users may input data in inconsistent formats. This can lead to confusion and make data difficult to analyze and use.
2. Inconsistent data formats #
Data lakes are designed to handle a variety of data types, including structured, semi-structured, and unstructured data. However, if data isn’t ingested and stored in a consistent manner, it can become difficult to manage and analyze.
For instance, one team may store date information as ‘DD-MM-YYYY’, while another uses ‘MM-DD-YYYY’. This inconsistency can create confusion and lead to incorrect analysis.
3. Lack of metadata #
Metadata provides information about other data, helping users understand the context, source, and characteristics of the data.
- Without sufficient metadata, finding and using data effectively can become a difficult task.
- This results in making a data lake feel more like a data swamp.
As an example, if a dataset does not have clear metadata indicating what each column represents. A data analyst might spend considerable time just figuring out what the data actually means before any meaningful analysis can occur.
4. Insufficient access controls and security #
Proper access controls and security measures are crucial for managing data. Without them, sensitive data could be exposed, or data could be accidentally (or intentionally) modified, leading to data integrity issues.
For example, if everyone in an organization has ‘Write’ access to a central data repository, it increases the chance of data being accidentally altered or deleted.
5. Data volume, velocity, and variety #
The 3Vs of big data - Volume, Velocity, and Variety - pose a significant challenge.
- The sheer volume of data can overwhelm traditional data management practices.
- The speed at which data is generated (velocity), and the variety of data types and sources, can also contribute to a data lake becoming a data swamp.
For example, a company might collect terabytes of social media data, customer transaction data, sensor data, and more, each with different formats and characteristics. If this diverse data isn’t effectively managed, it can lead to a chaotic, swamp-like environment.
6. Lack of maintenance and upkeep #
Data lakes need regular maintenance and upkeep, including tasks like:
- Data cleaning
- Updating outdated information
- Checking for data duplication, and so on.
If these tasks are neglected, the data lake can quickly become a data swamp. For instance, without regular cleaning, duplicate or outdated data can accumulate in the data lake, leading to inefficiencies and potential inaccuracies in analytics.
These reasons highlight the importance of having robust data management strategies and practices in place when working with data lakes. Proper attention to data governance, data formats, metadata, security, and regular maintenance can help prevent a data lake from turning into a data swamp.
What is the impact of data swamp on organizations? #
Data swamps can have a significant impact on organizations, affecting both their operational efficiency and their financial performance. The impacts of data swamps on your organization are considerable and worth taking a look:
- Decreased productivity
- Poor decision making
- Increased costs
- Regulatory risks
- Missed opportunities
- Reduced customer satisfaction
- Security risks
Here’s a detailed look at the potential impacts:
1. Decreased productivity #
In a data swamp, data scientists and analysts often spend a large proportion of their time trying to find, understand, and clean the data they need. They don’t get time to analyze and derive insights. This can significantly reduce their productivity and slow down data-driven projects.
2. Poor decision-making #
If data is disorganized, incomplete, outdated, or otherwise of poor quality, it can lead to inaccurate analytics and insights, which in turn can lead to poor decision-making.
For instance, if sales data is not up-to-date or comprehensive, forecasts and strategies based on this data may be incorrect. This leads to lost sales opportunities (affecting the top line).
3. Increased costs #
Managing a data swamp can be costly. Resources may be wasted on redundant or obsolete data. Additionally, significant costs can be incurred in trying to clean up the data swamp.
For example, the cost of data cleaning tools, or the time cost of manual data cleaning by employees. This increased operational expense can eat into the organization’s bottom line.
4. Regulatory risks #
Data swamps often lack robust data governance and security measures, which can lead to regulatory compliance risks.
For example, if an organization is unable to accurately track where sensitive customer data is stored because of poor data management. It could potentially fall foul of regulations such as GDPR, leading to financial penalties.
5. Missed opportunities #
The difficulty in finding and accessing relevant data in a data swamp can mean that organizations fail to capitalize on potential opportunities.
For instance, they might miss out on cross-selling or up-selling opportunities because they don’t have a comprehensive view of their customer’s behaviors and preferences.
6. Reduced customer satisfaction #
In today’s digital age, customers expect personalized and efficient interactions. If a company’s data is disorganized and inaccessible, it can hinder its ability to provide these personalized experiences.
This may lead to decreased customer satisfaction and potentially reduced customer loyalty.
7. Security risks #
Lack of proper access controls and security measures in a data swamp can increase the risk of data breaches. This can lead to financial loss, reputational damage, and potential regulatory penalties.
All these factors can have a significant impact on an organization’s top line (revenue) and bottom line (profits). Thus, it’s crucial for organizations to invest in robust data management practices to avoid the formation of data swamps.
How can you prevent data swamping? #
Preventing the formation of a data swamp or mitigating an existing one involves several strategic steps which include:
1. Establish strong data governance #
Data governance involves creating guidelines and processes for how data is to be handled. This includes aspects such as:
- Data collection
- Data storage
- Data access
- Data usage
- Data disposal
It also involves establishing roles and responsibilities for data management within the organization.
2. Consistent data formats #
Standardize on the data formats across your organization to reduce inconsistencies. The use of a common data model can be helpful in this regard. This ensures that different types of data can be understood and used effectively by everyone.
3. Implement metadata management #
Metadata provides crucial information about the data and aids in its discovery and use. Implement systems to automatically capture and manage metadata. Clear metadata should be created for all data stored in the data lake.
4. Data quality checks #
Implement automated data quality checks at the point of ingestion into the data lake. This can include checks for:
Invalid or poor-quality data should be corrected or rejected.
5. Access controls and security #
Implement robust access controls to ensure that only authorized users can access or modify the data. Data security measures, such as encryption, should also be applied to protect sensitive data.
6. Data cataloging #
Implement a data cataloging system to organize and index the data in the data lake. A data catalog provides an inventory of data available and makes it easy for users to find the data they need.
7. Regular maintenance and cleanup #
Regularly remove obsolete or redundant data from the data lake to keep it clean and manageable. Implement automated systems for data cleanup and de-duplication.
8. Invest in data lake management tools #
Numerous tools and platforms are available that can aid in managing data lakes and preventing data swamps. These tools can automate many of the tasks involved in data management, such as:
- Data ingestion
- Metadata management
- Data quality checks
- Data cleanup.
9. Training and culture #
Cultivate a modern data-driven culture within your organization. Train staff on the importance of good data management practices and the role they play in preventing data swamps.
10. Human-in-the-loop interaction #
Involve data practitioners in the data management process to bring in their expertise and judgment, especially in ambiguous or complex situations.
If a data swamp has already formed, these steps can still be applied to remediate the situation. It might also be necessary to undertake a significant one-time cleanup effort to bring the data into a manageable state.
This could involve tasks like data cleaning, reformatting, metadata creation, and reorganizing the data. This process may be time-consuming and costly, but it’s an essential investment to restore the value of the data lake.
Rounding it all up together #
Data lakes are meant to store large volumes of raw data in its native format until it’s needed. They can turn into data swamps if they’re not properly managed. Data swamps are characterized by poor data quality, lack of data governance, inadequate metadata, and lack of a data catalog, among other issues.
Data swamps can form due to various reasons, such as lack of data governance, inconsistent data formats, lack of metadata, etc. They can decrease productivity, lead to poor decision-making, increase operational costs, and pose regulatory risks.
If a data swamp has already formed, a good remedial step can be applied to remediate the situation, alongside a significant one-time cleanup effort.
However, prevention is better than cure. Implementing good data management practices from the start is the best way to prevent a data swamp from forming.
Overall, while data lakes can provide significant benefits in terms of flexibility, speed to insight, and simplicity of implementation, careful and ongoing management is necessary to prevent them from turning into data swamps.
Data swamp: Related reads #
- Data Lake vs Data Swamp: Differences & Cautionary Steps
- What is a data lakehouse: Definition, architecture, components, and use cases.
- What is a data lake: Definition, examples, architecture, and solutions.
- Data Warehouse vs Data Lake vs Data Lakehouse: What are the key differences?
- What is Metadata? - Examples, Benefits, and Use Cases
- Metadata Management: Benefits, Automation & Use Cases
Share this article