How to Implement Master Data Management With Databricks?
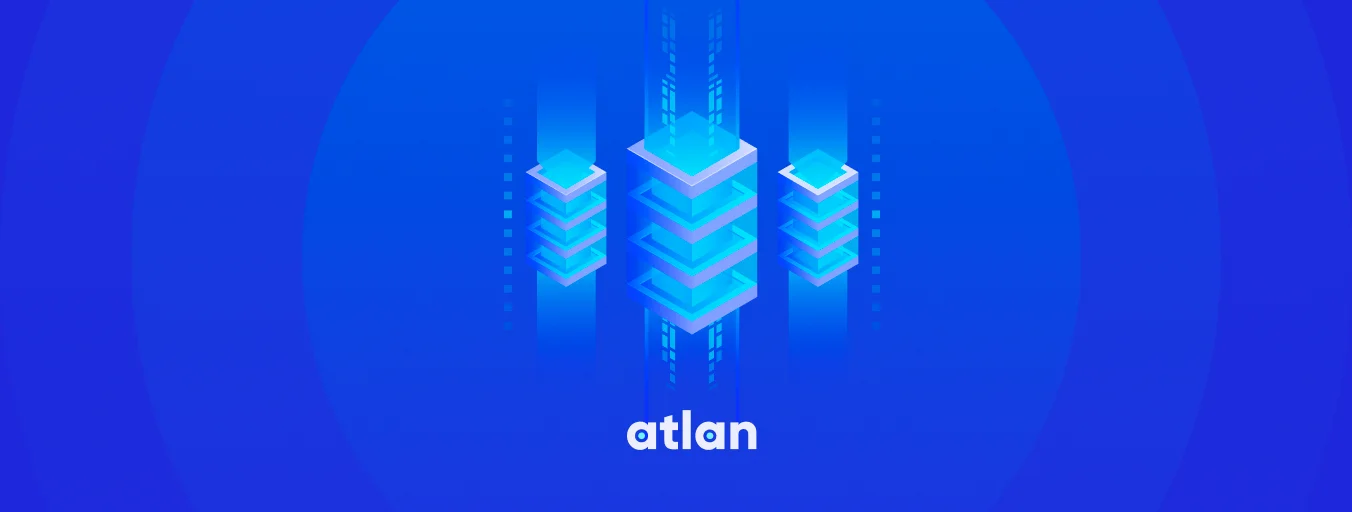
Share this article
Using a master data management (MDM) tool with Databricks is crucial in managing data and dealing with large and diverse data sets in Databricks. With high-quality data from master data management, Databricks can be used to its full potential in advanced analytics and machine learning projects, leading to more innovative and impactful outcomes.
Databricks, as a data intelligence platform, aligns well with master data management use cases. Its capabilities in ETL, data ingestion, business intelligence, AI, and governance support the key aspects of MDM, such as creating a single, reliable source of master data.
Integrating a master data management tool with Databricks enhances data quality, governance, integration, and analysis, leading to more reliable and insightful data-driven decisions.
Modern data problems require modern solutions - Try Atlan, the data catalog of choice for forward-looking data teams! 👉 Book your demo today
Table of contents #
- Why should you use a master data management tool with Databricks?
- Databricks overview
- What is master data management?
- Strategy for implementing a master data management tool with Databricks
- Guidelines for effective implementation
- Databricks Delta as a master data management tool
- Master data management for Databricks: Related reads
Why should you use a master data management tool with Databricks? #
Implementing a master data management tool is crucial for several reasons. Let’s look at some of them.
- Data consistency: Ensures uniformity, accuracy, and consistency of critical data across the organization.
- Improved decision making: Provides a comprehensive and reliable data source, enhancing business decision-making.
- Operational efficiency: Streamlines data management processes, reducing redundancy and errors.
- Compliance and governance: Supports adherence to data governance policies and regulatory compliance by maintaining data integrity and quality.
Databricks overview #
Databricks is a data intelligence platform integrating AI, ETL, data ingestion, business intelligence, and governance, enabling users to own and innovate with their data and AI.
What is master data management? #
Master data management is a process that involves creating a single, authoritative master record for each key entity in a business, such as people, places, or things, by aggregating, de-duplicating, reconciling, and enriching data from various internal and external sources and applications.
This master data becomes a reliable and consistent source that is managed and shared across the organization. The purpose of master data management is to:
- Provide a trusted view of critical business data
- Enhance accuracy in reporting
- Reduce errors and redundancies in data
- Support better-informed business decisions
Strategy for implementing a master data management tool with Databricks #
Implementing a master data management tool with Databricks involves the following strategies:
- Integration compatibility: Ensure the tool seamlessly integrates with Databricks for efficient data processing and analytics.
- Scalability: Assess if the tool can handle increasing data volumes without performance degradation.
- AI and machine learning capabilities: Check for advanced AI features that complement Databricks’ analytics strengths.
- Data governance: Choose tools with robust data governance features to maintain data integrity and compliance.
- Missed aspects: Don’t overlook user-friendliness and support services, which are crucial for smooth implementation and maintenance.
- Business case: Highlight the tool’s potential to enhance data accuracy, decision-making, and operational efficiency. Demonstrate ROI by linking the tool’s capabilities with specific business goals and cost savings.
Guidelines for effective implementation #
Common pitfalls in implementing master data management tool with Databricks include:
- Underestimating data complexity and variety leads to inadequate data standardization.
- Failing to fully leverage Databricks’ AI and machine learning for data harmonization.
- Overlooking the need for rigorous data governance policies in a dynamic data environment.
- Neglecting the integration challenges between Databricks and various data sources, causing data inconsistencies.
Databricks Delta as a master data management tool #
Databricks Delta is a component of the Databricks platform, designed to enhance the capabilities of data lakes with features typically associated with data warehouses. It provides a storage layer that brings reliability, performance, and lifecycle management to big data.
Delta introduces ACID transactions to data lakes, ensuring data integrity by allowing multiple simultaneous read and write operations. It also optimizes data storage and access, enabling faster query performance and efficient handling of large datasets.
In the context of master data management (MDM), Databricks Delta plays a vital role. It supports master data management by ensuring data consistency and integrity, crucial for maintaining reliable master data.
Delta’s ability to handle massive and diverse datasets efficiently, combined with its real-time data processing capabilities, makes it ideal for master data management. By leveraging Delta, businesses can ensure their master data is not only accurate and consistent but also readily accessible and analyzable, thereby enhancing decision-making and operational efficiency.
Master data management for Databricks: Related reads #
- Databricks documentation
- Databricks concepts
- The Databricks Data Intelligence Platform
- Top 15 Benefits of Master Data Management in 2024
- Difference between Master Data Management (MDM) and Metadata Management
- Data Catalogs vs Master Data Management: Decoding Differences, Similarities And Use Cases
- Master Data vs. Transactional Data: Unveiling the Data Symphony
Share this article