Enterprise Data Governance Tools: How to Find Tools That Scale
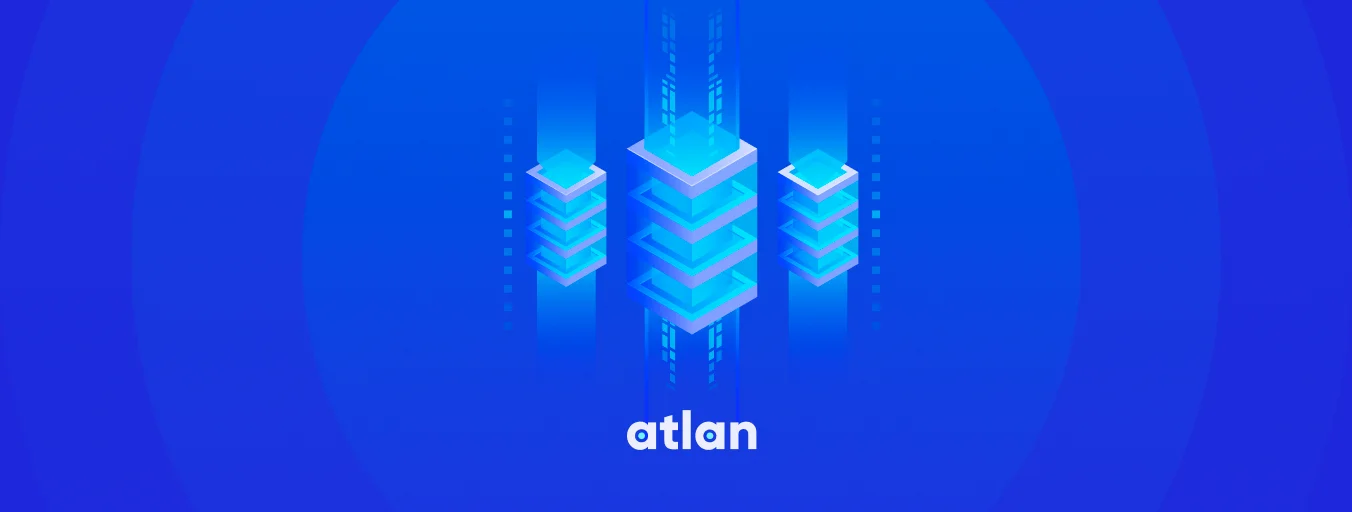
Share this article
At the enterprise scale, data governance poses unique challenges. The larger an enterprise grows, the more data sources it will have, potentially leading to mismatched systems and ballooning costs. Advanced data governance tools become necessary to handle this growing data architecture.
In this article, learn what challenges enterprise data governance tools can address and which of these tools are best suited to address each problem.
Modern data problems require modern solutions - Try Atlan, the data catalog of choice for forward-looking data teams! 👉 Book your demo today
Table of contents #
- The challenge of enterprise data governance management
- What to look for in enterprise data governance tools
- Examples of enterprise data governance tools
- Conclusion
- Related reads
The challenge of enterprise data governance management #
Enterprises data governance faces challenges on three fronts: scalability, complexity, and usability.
The scalability of software & processes #
The global DataSphere is a metric that tracks the amount of data created and used by organizations. According to the International Data Corporation, it is on track to double from 2022 to 2026. Enterprises are driving the bulk of this growth.
Another survey by Matillion and the International Data Group found that, as of 2022, companies have an average of 400 data sources, with 20% having over 1,000 data sources.
The complexity of large data catalogs #
At this scale, even a simple workflow like documentation poses a challenge. Standard tools like data lineage visualization can fail to provide the insight they do for smaller data systems.
As an enterprise creates solutions to the friction created by its expanding data environment, standardization becomes a problem. When different data silos are using different methods to manage their data, the enterprise as a whole can lose track of what data is where.
If those silos are using different methods to organize their data, this can create inconsistencies in the data structures. In an age of expanding regulations around data privacy and access permission, these inconsistencies can lead to significant fines.
The usability of enterprise data governance tools #
At the enterprise scale, complex organizational structure can pose a serious obstacle to collaboration between different groups in the organization. Robust inline collaboration tools become necessary for teams to handle growing data volume properly.
Just giving people tools, however, is not enough. They also need training and support to use these sophisticated tools properly. An enterprise needs to develop strategies that prioritize training as an ongoing commitment so it can sustain its data governance systems into the future.
Addressing these problems requires significant investment from the enterprise, both in terms of time and money. As the data environment grows, these costs can become a serious drag on the budget. To keep data governance costs from hindering their growth, enterprises need to choose tools that can automate as many of these processes as possible.
What to look for in enterprise data governance tools #
Now that we have a sense of the data governance problems enterprises face, let’s consider the features of effective solutions to these issues:
- Automation
- DataOps support
- AI tooling
- Scalable usability features
- Inline collaboration
- Ease of use and explanation
Automation #
It may be possible to manage data governance for a small organization manually. But at scale, automation is crucial to making sure the large data environment is properly governed.
Access controls, for instance, can be handled on a person-by-person basis for a small team. But when hundreds of people are working with a data system, the enterprise needs a way to specify each person’s permissions precisely and have their data tools enforce those permissions automatically.
The same goes for data lineage. For complex data systems, tracking lineage by hand will be tedious and error-prone. Tools that track and display lineage automatically let engineers know how their updates will interact with tables and dashboards down the line, without having to refresh data through manually run CSV imports or pipelines.
A properly automated data governance system will also handle policy applications. When new data is brought into the environment, it enforces the correct data access policies without manual intervention. This is critical so that enterprise expansion doesn’t lead to a breakdown in role-based access controls, potentially exposing sensitive information and resulting in fines.
DataOps support #
DataOps is a mindset in data governance that emphasizes a holistic approach to data engineering by breaking the silos that separate data engineers and analysts. Rather than having these teams work separately, DataOps-based data governance enables continuous collaboration between these groups in a shared system.
When you achieve such collaboration, you can ship data products like they are software products. You can leverage agile and lean methodologies in the data management and analytics side of the enterprise, just like organizations do for software development. This allows the enterprise to adapt to a changing data environment, continuously improving its data products.
AI tooling #
Integrating AI tools in data governance allows an enterprise to automate the processes that limit data catalog scalability. Properly implemented AI tools can automatically give suggestions for data documentation, provide suggestions for data tags, update those tags at scale, and check data quality. It can also raise alerts proactively if it detects any issues, such as potentially untagged sensitive information.
Scalable usability features #
Robust data governance requires tools that allow data to be seen and searched. For an enterprise, these tools must scale to fit a large data environment.
Visual tools like data lineage need to support zooming and panning to give teams a proper sense of the scale and structure of the data system, as well as filtering so that users can focus their investigation.
Why is data lineage important? - Source: Atlan on Youtube.
At scale, however, simple filters can lead to conflicting definitions and miscommunication. Enterprise data governance tools should support enhanced options like persona-based filtering to coordinate understanding across the organization properly.
All of this needs to connect with robust search functionality that can handle large data sets to narrow down searches by use case properly.
Inline collaboration #
The key to any data governance program is sharing and collaboration. Inline collaboration breaks through data silos, creating a united culture and democratizing the maintenance of data quality and classification. This allows people to bring diverse perspectives to the data, minimizing bias in data sets and analysis.
Ease of use and explanation #
With a collaborative mindset of data governance, tools must be usable by many users – from data engineers to analysts, from managers to leaders. The easier the onboarding process is for using these tools, the faster an enterprise can improve its data governance and expand its personnel and operations.
Any tool an enterprise adapts, therefore, should come with a sufficient baseline of training and documentation that enables new users to engage with the data system smoothly.
Examples of enterprise data governance tools #
Now that we have seen what effective tools look like, let’s look at a few examples of companies that implemented these tools effectively.
In the process of updating their data governance tools, Nasdaq decided to add Atlan’s active metadata management to their data systems, building a Google for their data.
One Fortune 500 company used Atlan to add data governance to their data lake, managing 62 external data connections and 900 workflows. In the process, they finally completed a three-year-old KPI with Atlan’s data governance support.
Contentsquare, a growing digital experience platform, wanted to implement a data governance program. Implementing Atlan allowed them to fully map their data estate, streamline collaboration, and propagate data standards across its entire system.
Elastic, a distributed enterprise built on an open source culture, used Atlan’s active metadata management tools to build a data governance program that aligned with their goals of transparency, accountability, and engagement.
Conclusion #
We have seen that enterprises face unique challenges regarding data governance. Facing these challenges requires the right tools for the job. Proper tools should be automated, support DataOps, have AI tooling, scalable usage, inline collaboration, and ease of use.
Atlan’s active metadata management supports all these features and has already helped multiple enterprises scale their data governance programs in line with their unique needs.
Are you ready to equip your enterprise with data governance tools? Contact Atlan today.
Enterprise data governance tools: Related reads #
- What is Data Governance? Its Importance, Principles & How to Get Started?
- Data Governance Framework — Examples, Templates, Standards, Best Practices & How to Create One?
- Data Governance Policy : Examples, Templates & How to Write One
- Data Governance Roles and Responsibilities: A Quick Round-Up
- Enterprise Data Governance — Basics, Strategy, Key Challenges, Benefits & Best Practices
- Automated Data Governance: How Does It Help You Manage Access, Security & More at Scale?
- How to implement data governance? Steps, Prerequisites, Essential Factors & Business Case
- Open Source Data Governance Tools - 7 Best to Consider in 2023
- 7 Steps to Simplify Data Governance for Your Entire Organization
Share this article