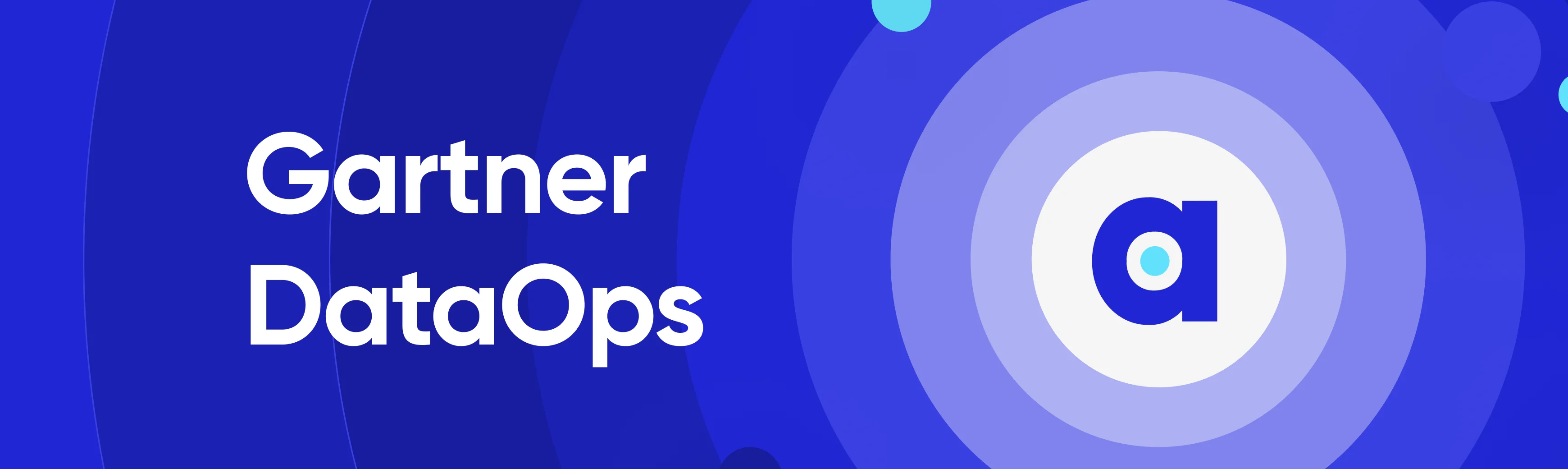
Share this article
DataOps is a data management practice that combines the best of Lean, Product Thinking, Agile, and DevOps to get value from data while saving time and reducing wasted effort.
Global research and advisory firm Gartner identifies DataOps as a way to automate and optimize data flows while improving collaboration for its various stakeholders.
In this article, we’ll look into Gartner’s take on DataOps and its recommendations for introducing DataOps within organizations.
Gartner’s Inaugural Magic Quadrant for D&A Governance is Here #
In a post-ChatGPT world where AI is reshaping businesses, data governance has become a cornerstone of success. The inaugural report provides a detailed evaluation of top platforms and the key trends shaping data and AI governance.
Read the Magic Quadrant for D&A Governance
Table of contents #
- Understanding DataOps
- Gartner’s insights on adapting DataOps
- Gartner’s recommendations for introducing DataOps
- Gartner on DataOps tools
- Future of DataOps
- Related reads
Understanding DataOps: Gartner’s perspective #
According to Gartner, DataOps is “a collaborative data management practice focused on improving the communication, integration, and automation of data flows between data managers and data consumers across an organization.”
The goal is to optimize data-driven decision-making processes and deliver value faster.
Here’s how Ted Friedman, Distinguished VP Analyst, Gartner puts it:
“The point of DataOps is to change how people collaborate around data and how it is used in the organization.” Gartner on DataOps: Rather than simply throwing data over the virtual wall (where it becomes someone else’s problem), the development of data pipelines and products should be a collaborative exercise.
Changing how you collaborate around data requires developing a shared understanding of the value proposition of DataOps. It also necessitates a change in the way you work with data.
This means eliminating traditional waterfall delivery setups where data teams are fragmented, data is siloed, and processes are riddled with bottlenecks.
Read more → The 9 principles of DataOps
Why is DataOps important? #
With DataOps, you can operationalize data in a timely, consistent, and repeatable manner. According to Gartner Research titled Data and Analytics Essentials: DataOps, this leads to benefits, such as:
- Improved organizational speed in delivering trusted data
- Elimination of unwanted data delivery efforts by focusing on value flows
- Greater collaboration across data, business, and technical personas
- Management of interdependencies across business processes
- Increased reusability of data engineering work product through automation
- Reliable data-delivery meeting service levels
Also, read → Building a business case for DataOps
Who benefits from DataOps? #
Even though DataOps is primarily seen as an engineering practice, its impact goes far beyond.
Gartner highlights how data engineers, data consumers, and organizations benefit from DataOps — greater productivity, improved trust in data, and a culture of self-service and collaboration.
With DataOps, every data practitioner can get more value from data.
For data engineers, it’s when the data pipelines are observable so that whenever they break, they can be fixed right away. For business managers, it’s when they have access to ready-to-use data, without any bottlenecks.
So, embracing DataOps is beneficial to all data practitioners and organizations seeking to derive maximum value from their data assets.
How DataOps affects the end users - Source: Towards Data Science.
Gartner’s insights on adapting DataOps to three core value propositions #
Demonstrating the value of DataOps is easier when it is tied to business outcomes. Succeeding with DataOps also becomes more likely when the organization as a whole is invested in making it work.
To this end, Gartner proposes three value propositions for better collaboration and extracting greater value from data. These include:
- Adapting the DataOps strategy to a utility value proposition
- Using DataOps to support data’s use as a business enabler
- Supporting the data and analytics driver value proposition
Three value propositions for adapting DataOps and amplifying the value of data - Source: Gartner.
Let’s explore each value proposition further.
1. Adapting the DataOps strategy to a utility value proposition #
Treat data as a utility — a product — that meets specific needs.
A great way to get started is by ensuring that each data asset has an owner or a data product manager. The data product manager is accountable for guaranteeing that the data assets they own deliver value to the intended data consumers.
Read more → How to apply product thinking to data
2. Using DataOps to support data’s use as a business enabler #
Identify use cases — fraud detection, supply chain optimization, etc. — and ensure that data can support these use cases. The right data consumers should have ready access to the data they need for making decisions.
3. Supporting the data and analytics driver value proposition #
Find new opportunities — new revenue streams, markets, etc. — and deliver business value using data assets.
Gartner on DataOps: Use DataOps to link “Can we do this?” to “How do we provide an optimized, governed data-driven product”
Gartner’s recommendations for introducing DataOps #
Bringing about a cultural shift can be challenging as most workplaces are resistant to change. That’s why Gartner recommends a few best practices to maximize the chances of successfully introducing DataOps:
- Choose the right projects
- Focus on collaboration
- Involve the right people
- Assign data asset ownership and accountability
- Track the right metrics
Let’s dig into each recommendation.
Choose the right projects #
Pick data and analytics projects that are struggling due to lack of collaboration, overburdened by the pace of change, or where service tickets from data consumers are piling up. These projects create the best opportunity to show value.
Focus on enabling collaboration across roles, domains, and projects #
Gartner recommends connecting the various data practitioners — DBAs, data engineers, integration architects, data stewards, etc. — by providing an infrastructure for shared metadata, regular communication, and feedback.
They should be involved in decisions that define requirements, develop pipelines, and manage and monitor data flows, among others.
For instance, at Atlan, developing a culture code and using it as a foundation for everything we build has worked well. Our systems are transparent, discoverable, and accessible to the right people. Moreover, we have embraced the diverse nature of data teams and set up mechanisms for effective collaboration.
Read more → DataOps culture code at Atlan
Reach out to the right people #
Gartner recommends reaching out to application leaders who have successfully applied DevOps practices to application development and operations.
They can help you get started on foundational activities, such as version control repository setup, building regression packs, and release pipelines, without reinventing the wheel.
Assign ownership and accountability #
Another Gartner recommendation for success with DataOps is to revisit current data operations ownerships and service level management. DataOps teams should generally own the full development life cycle from inception to production.
Track the right metrics #
Monitor metrics such as time to deploy changes, degree of automation, team productivity, data pipeline quality, pipeline failure rates, and more.
Also, read → The future of the metrics layer and metadata
Gartner on DataOps tools #
Gartner highlights the role of technology in DataOps. Technology can automate the design, deployment, and management of data delivery with appropriate levels of governance.
According to Gartner, DataOps tools should have core capabilities such as:
- Orchestration: Connectivity, workflow automation, lineage, scheduling, logging, troubleshooting and alerting
- Observability: Monitoring live/historic workflows, insights into workflow performance and cost metrics, impact analysis
- Environment Management: Infrastructure as code, resource provisioning, environment repository templates, credentials management
- Deployment Automation: Version control, release pipelines, approvals, rollback, and recovery
- Test Automation: Business rules validation, test scripts management, test data management
What does the future of DataOps look like? #
Gartner suggests extending the idea of DataOps to a set of capabilities labeled as XOps. This would include:
- DataOps (data engineering): Handles building, managing, and scaling data pipelines, along with capabilities for data extraction, integration, transformation, and analysis
- MLOps (machine learning development): Includes activities involved in building and operationalizing the ML model development cycle
- ModelOps (AI governance): An extension of MLOps that governs working with third-party AI models
- Platform Ops (overarching AI platform management): The AI orchestration platform that helps in managing DevOps, DataOps, MLOps, ModelOps, and SecOps; different from AIOps as that refers to using AI for improving IT operations management
Gartner on the XOps frameworks that will shape the future of DataOps - Source: VentureBeat.
Soyeb Barot, research director at Gartner, refers to the XOps as frameworks that can ”help implement a structured process for the people involved to productionalize AI. Think of it as the assembly line of an automobile manufacturing plant, but for data.”
According to Barot’s interview with VentureBeat, XOps are for organizations that already have a strong DataOps framework and need more help in operationalizing their AI processes.
If your organization has already laid the foundations for strong DataOps practices, it’s prudent to watch this space grow as the data stack matures and evolves.
Gartner DataOps: Related reads #
- Gartner Data Catalog Research Guide — How To Read Market Guide, Magic Quadrant, and Peer Reviews
- A Guide to Gartner Data Governance Research — Market Guides, Hype Cycles, and Peer Reviews
- Gartner Active Metadata Management: Concept, Market Guide, Peer Insights, Magic Quadrant, and Hype Cycle
- Active Metadata: Your 101 Guide From People Pioneering the Concept & It’s Understanding
- The G2 Grid® Report for Data Governance: How Can You Use It to Choose the Right Data Governance Platform for Your Organization?
- The G2 Grid® Report for Machine Learning Data Catalog: How Can You Use It to Choose the Right Data Catalog for Your Organization?
Share this article