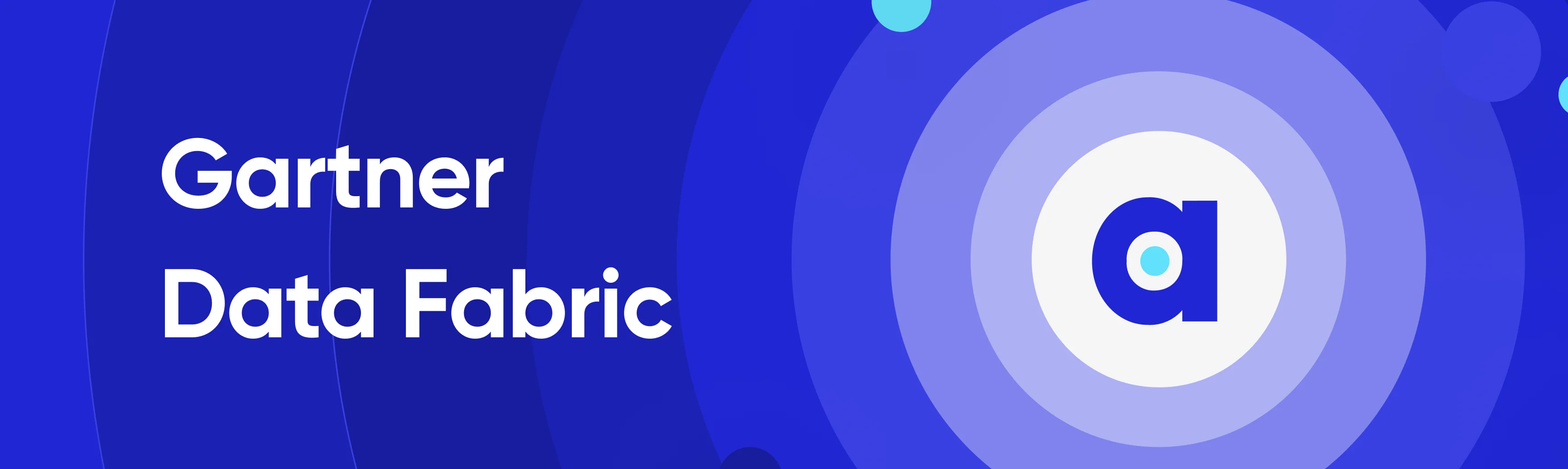
Share this article
In today’s data-driven world, organizations are grappling with the daunting task of managing vast and complex data landscapes. Recognizing this challenge, Gartner, a global research and advisory firm, believes the data fabric architecture is a key solution.
Here we present the intricacies of a data fabric, its strategic importance according to Gartner, the potential challenges of adoption, and crucially, the implementation principles recommended by Gartner.
Table of contents #
- Understanding a data fabric: Gartner’s perspective
- Gartner’s strategic take on data fabric
- Gartner’s view of challenges in adopting data fabric
- Gartner’s recommendations for data fabric Implementation
- Gartner data fabric: Related reads
Understanding a data fabric: Gartner’s perspective #
At the heart of Gartner’s philosophy lies the idea that a data fabric is not a single tool or technology, but rather an “emerging data management design for attaining flexible, reusable, and augmented data integration pipelines, services, and semantics.”
Data fabric is a holistic approach that incorporates various facets of data management under a unified architecture.
The analogy that Gartner uses to describe a data fabric is a “self-driving car”. Just as an autonomous vehicle uses advanced technology to perceive its environment and navigate without human intervention, a data fabric employs machine learning capabilities to autonomously access, consolidate, and manage data.
Gartner’s strategic take on data fabric #
Gartner’s strategic perspective on data fabric is predicated on the efficiencies it can deliver. They propose that a well-implemented data fabric can “quadruple” efficiency levels, effectively reducing human-intensive data management tasks by half.
For instance, data scientists and analysts typically spend approximately 60% of their time on mundane tasks such as cleaning and organizing data. By dramatically reducing this percentage using a data fabric, organizations can allocate more time to value-adding tasks such as data analysis and interpretation.
Gartner strategically positions data fabric as a critical solution that streamlines the time-intensive processes of data management. In addition, Gartner says a data fabric also expedites the transition towards data-driven decision-making across businesses.
The value of data fabric to stakeholders #
The benefits of implementing a data fabric approach are becoming increasingly evident, with many businesses across industries already incorporating this strategic framework. Companies such as IBM, and Dell EMC have leveraged the data fabric model to facilitate data access and analysis across their varied data environments.
One of the most significant values offered by data fabric to stakeholders lies in breaking down data silos. Data silos - isolated islands of data inaccessible to other parts of the organization - can hinder information flow and lead to inefficiencies.
Data fabric addresses this issue by providing a unified, integrated view of data across the organization, irrespective of where the data resides or its format.
Gartner’s view of challenges in adopting data fabric #
Gartner recognizes that adopting a data fabric is not a straightforward process and comes with its unique set of challenges.
One challenge is obtaining support from key stakeholders. Implementing a data fabric entails a shift in both technology and culture, necessitating buy-in from all levels of an organization.
Another challenge is that you can’t purchase a data fabric off the shelf. Data fabrics are built to suit the specific needs of an organization.
Fortunately, you can use tools like data catalogs to speed up the process. Data catalogs serve as a single source of truth for all data assets, making data discovery and governance more efficient and promoting the adoption of a data fabric.
A further obstacle is the lack of data, particularly metadata. Metadata provides context and meaning to data assets, making them easier to find and understand. Collecting, managing, and integrating metadata is a significant task that needs to be addressed while building a data fabric.
Lastly, creating a data fabric requires a specialized knowledge set, including skills in areas like NoSQL, GraphQL, and others.
Gartner’s recommendations for data fabric Implementation #
In their comprehensive guide, “From Data and Analytics Essentials: How to Define, Build, and Operationalize a Data Fabric,” Gartner presents a detailed outline of recommendations for effectively implementing a data fabric:
- Gather and scrutinize all metadata types
- Shift from static to dynamic metadata
- Develop and manage knowledge graphs
- Create a Robust Data Integration Backbone
- Establish and use protocols and standards
- Allocate resources to enriched data catalogs
Gather and scrutinize all metadata types #
The collection and analysis of all forms of metadata form a crucial part of implementing a data fabric. In the context of a data fabric, metadata acts as the framework that enables the system to understand, organize, and manage data effectively.
Shift from static to dynamic metadata #
In the domain of data management, there’s an increasing shift from passive to active metadata.
Passive metadata involves simple documentation of data, such as its source, date of creation, or format. Meanwhile, active metadata provides context, relationships, and lineage, acting as a living, breathing part of the data landscape.
Gartner emphasizes the transformation of passive metadata to active metadata as a critical step in effectively leveraging a data fabric.
Read more → Active metadata 101
In addition, Gartner highlights the value of visualizing active metadata and deriving metrics from it.
Visual representation makes data more understandable and digestible, aiding in better decision-making. You can also feed active metadata metrics to artificial intelligence and machine learning algorithms, improving their performance over time.
Develop and manage knowledge graphs #
A powerful tool in data management, particularly within the realm of data fabric, is the use of knowledge graphs. A knowledge graph is a model of a knowledge domain created by connecting and defining relationships between pieces of data.
Creating and curating knowledge graphs can provide an organization with a holistic view of its data, offering significant insights into how different data points relate to one another. This becomes particularly valuable in the context of data fabric, as it facilitates a deep understanding of data in an interconnected and meaningful way.
Create a robust data integration backbone #
A strong data integration backbone is a vital component in the implementation of a successful data fabric. While it might be easy to confuse data integration with data ingestion, the two have distinct roles in the data management ecosystem.
Data ingestion involves importing data into a system. But data integration goes a step further. It includes gathering data from various sources, consolidating it, and then finally importing it in a way that creates a unified, holistic view of data.
Different data integration methods such as Extract, Transform, Load (ETL) - and its increasingly more popular alternative, Extract, Load, Transform (ELT) - streaming, messaging, and replication should all be supported within a robust data integration backbone.
Gartner advises that creating a robust data integration backbone is crucial for effective data fabric implementation.
Establish and use protocols and standards #
In the development of a comprehensive data fabric, adhering to established protocols and standards is essential. For instance, embracing protocols like Open Data Discovery can help streamline the discovery of data across an organization. It aids in creating a unified view of data, thereby enhancing its accessibility and usability.
Additionally, it’s pivotal to ensure that the tools used within your data fabric support an Open API architecture. Open APIs facilitate seamless integration and interoperability between different software applications, promoting greater collaboration and efficiency.
Invest in augmented data catalogs #
Investing in augmented data catalogs is a strategic move for organizations implementing a data fabric design approach. Augmented data catalogs serve as centralized repositories, facilitating the collection of all types of metadata, thereby fostering effective data management.
In the context of data fabric, a data catalog plays a crucial role in maintaining the coherence and usability of the data. It helps in managing and understanding data across the organization by capturing metadata and providing a searchable repository.
Read more → Modern data catalogs
Summing up #
According to Gartner, a data fabric is an innovative design approach for data management, characterized by flexibility, reusability, and augmented data integration. It’s not a singular tool or technology, but an overarching strategy that leverages machine capabilities to accomplish tasks like data profiling, schema discovery, and self-healing datasets.
The cornerstone of a successful data fabric strategy lies in having a robust, open data catalog. It is this crucial component that ties together the disparate threads of data across an organization, providing a unified, searchable, and actionable view of data.
Gartner data fabric: Related reads #
- Gartner Data Catalog Research Guide — How To Read Market Guide, Magic Quadrant, and Peer Reviews
- A Guide to Gartner Data Governance Research — Market Guides, Hype Cycles, and Peer Reviews
- Gartner Active Metadata Management: Concept, Market Guide, Peer Insights, Magic Quadrant, and Hype Cycle
- Active Metadata: Your 101 Guide From People Pioneering the Concept & It’s Understanding
- The G2 Grid® Report for Data Governance: How Can You Use It to Choose the Right Data Governance Platform for Your Organization?
- The G2 Grid® Report for Machine Learning Data CatalogHow Can You Use It to Choose the Right Data Catalog for Your Organization?
Share this article