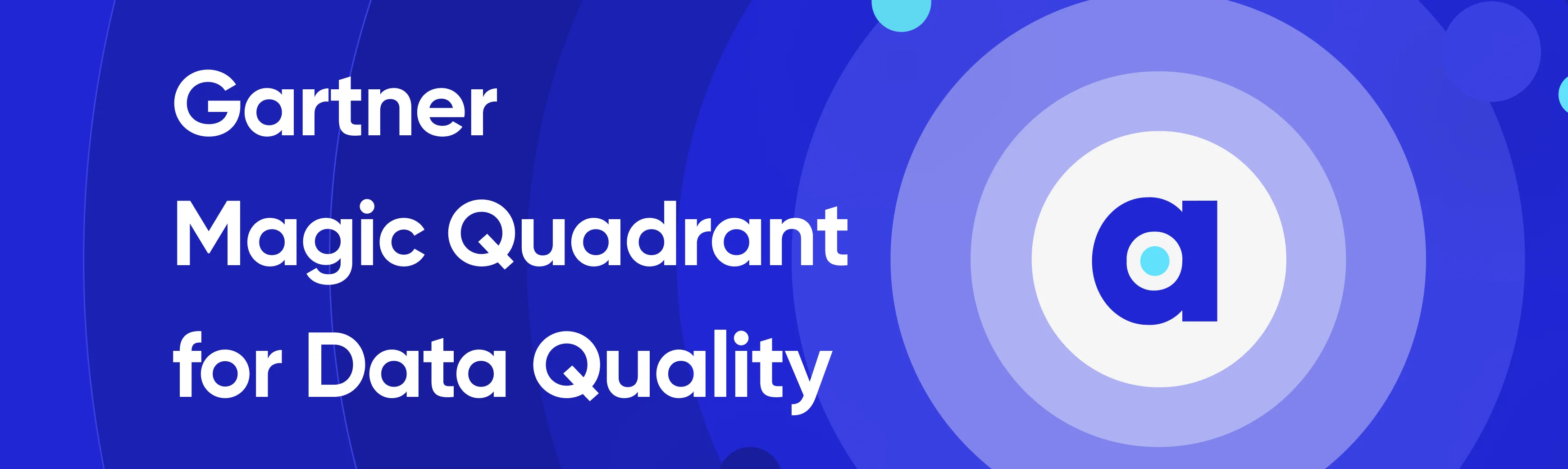
Share this article
In today’s data-driven world, data quality is crucial to an organization’s success. However, the prevalence of data quality issues has been rising, leading to disruptions in operational efficiency.
That’s why Gartner developed the Gartner Magic Quadrant for Data Quality Solutions, a classification criterion for data quality tools. It assesses each tool’s strengths and weaknesses in vision and execution.
In this article, we’ll summarize how Gartner assesses data quality tools and the trends it sees in the market. We’ll also cover what you should look out for when evaluating data quality tools for purchase.
Table of contents #
- Gartner on the state of the data quality market
- Gartner Magic Quadrant for Data Quality Solutions: An overview
- Gartner on improving data quality
- Concluding thoughts
- Related reads
Gartner on the state of the data quality market #
According to Gartner, data quality solutions are “the set of processes and technologies for identifying, understanding, preventing, escalating and correcting issues in data that supports effective decision making and governance across all business processes.”
Data quality tools are crucial in managing and ensuring data accuracy for many use cases. These use cases include analytics and data science, master data management, artificial intelligence, and machine learning.
These data quality improvements lead to:
- Improved data quality
- More reliable insights
- Improved decision-making processes
- Improved workflows
Gartner Magic Quadrant for Data Quality Solutions: An overview #
The Gartner Magic Quadrant for Data Quality Solutions - Source: Gartner.
The Gartner Magic Quadrant for Data Quality Solutions is a graphical representation of the rankings of a number of data quality tools.
In general, Magic Quadrants evaluate the various platforms available in the market. According to Gartner, a Magic Quadrant is “a snapshot in time that assesses the vendors’ vision and Ability to Execute.”
The Magic Quadrant is a snapshot in time of a vendor’s vision and the ability to execute - Source: Gartner.
Gartner determines their rankings based on the tool’s vision, market understanding, and demonstrated ability to execute.
Here are the four quadrants and what they stand for:
- Leader: Exhibits all of the essential qualities required for the platform to rank.
- Challenger: Has shown the ability to execute but lacks a clear vision of their future and market understanding.
- Visionary: Has a clear vision of the current market but has not demonstrated its ability to execute
- Niche Player: Focuses on a sub-section of the market but has an unclear vision and a lack of demonstrated execution.
These quadrants provide valuable insights into the alignment between a tool’s future aspirations, understanding of its market, and its proven track record.
Gartner Magic Quadrant for Data Quality Solutions: Essential capabilities #
According to Gartner, a core capability of data quality solutions is augmented data quality (ADQ). The platform employs advanced techniques such as metadata analytics, knowledge graphs, and artificial intelligence to automate labor-intensive repetitive or manual data quality processes.
For example, you can establish statistical processes to identify a range of numeric values to flag when the incoming values are out of bounds. This workflow streamlines quality workflows and minimizes the possibility of human error.
Gartner highlights augmented data quality as a key market driver. Other essential capabilities include:
- Connectivity with a wide range of data sources
- Comprehensive profiling to give business users insight into the quality of data and empower them to identify data quality issues
- Interactive visualization and analytics to help business and IT users identify, understand, and monitor data quality issues and discover patterns and trends
- Monitoring based on preconfigured, custom-built monitoring rules, or adaptive rules, and alert violations
- Auto-detection of outliers, anomalies, patterns, and drifts
- Parsing, standardizing, and cleansing capabilities
- Matching, linking, and merging of data entries across your data estate
- Metadata discovery and collection to support lineage, root cause analysis, data discovery, cataloging, and more
- Multidomain support
- Data curation and enrichment to improve completeness and add value.
- Rule management and data validation
- Business-driven workflow and issue resolution in a collaborative manner
- DataOps support to improve the communication, integration, and automation of data flows between data managers and data consumers across an organization
- Deployment environment
- Architecture and integration
- Automation and augmentation by leveraging advanced technologies such as AI/ML, knowledge graph, active metadata, or NLP to minimize manual effort
- Multi-person usability to engage and support the various roles (especially nontechnical business roles)
Next, let’s look at Gartner’s inclusion criteria for the Magic Quadrant for Data Quality Solutions.
Gartner Magic Quadrant for Data Quality Solutions: Inclusion criteria #
To qualify for inclusion, Gartner specifies the following inclusion criteria:
- Offers a stand-alone data quality solution
- Delivers critical data quality functions, such as data profiling, monitoring and detection, rule management and data validation, and parsing, among others
- Supports augmentation of the critical data quality functions
- Enables large-scale deployment, along with support for the cloud-based/SaaS versions
- Supports multiple data domains and diverse use cases across different industries
- Maintains an installed base of at least 100 production paying customers (different companies/organizational entities); alternatively, makes at least $5 million in total recognized revenue for software (license, maintenance, and subscription and not any services)
- Includes customers in multiple countries and in at least two of these regions — North America, South America, EMEA, and Asia/Pacific
- Supports data quality functionality with packaged capabilities to process data in at least two different languages
- Includes a complete solution for four or more of the following types of users — data steward, data architect, data quality analyst, data engineer, data integration analyst, data scientist, business intelligence analyst, and a casual user
- Provides out-of-box (OOTB) and prebuilt data quality rules for data profiling and monitoring, cleansing, standardization, and transformation
- Supports integrability and interoperability with other data management solutions
- Offers direct sales and support operations, or a partner providing sales and support operations in at least two of the following regions — North America, South America, EMEA and Asia/Pacific
Gartner Magic Quadrant for Data Quality Solutions: Ranking criteria #
As mentioned earlier, Gartner looks at the Ability to Execute by considering the following criteria:
- Product or service capabilities, quality, feature sets, skills, and more; solutions are rated based on their ability to address current market needs
- Overall viability that shows the likelihood of a vendor continuing to offer and invest in the product
- Sales execution/pricing methods — deal management, pricing and negotiation, presales support, and the overall effectiveness of the sales channel (direct and indirect sales)
- Market responsiveness/record demonstrating the vendor’s ability to respond, change direction, be flexible, capitalize on opportunities, and adapt as needs evolve or market dynamics change
- Marketing execution that uses messaging capable of influencing the market, boosting brand awareness, and cultivating a positive brand image
- Customer experience and the level of satisfaction they express
- Operations in terms of the quality of the organizational structure, skills, experiences, programs, the stability of key staff, and more
Gartner Magic Quadrant for Data Quality Solutions evaluation criteria - Source: Gartner.
Gartner on improving data quality #
Gartner also defines a data quality maturity scale. Organizations can determine the level of data quality maturity they have attained and identify the next steps to enhance their data quality practices.
Gartner’s Data Quality Maturity scale defines Low, Medium, and High Data Quality as the following:
- Low Quality: An organization’s actions are restricted to defining the impact of data quality improvements on business outcomes via KPIs and establishing data quality standards.
- Medium Quality: An organization prioritizes data quality. They set up and utilize dashboards and reporting to track data quality metrics and establish data quality responsibilities.
- High Quality: An organization integrates data quality throughout its processes.
Once you’ve assessed the current standing of your data quality, you can take the necessary steps to improve it. These may include:
- Defining clear data governance policies
- Defining data standardization standards and data cleansing processes
- Monitoring data quality
- Training users on the importance of data quality and the processes necessary to achieve it
Concluding thoughts: Build data quality into your data projects #
Gartner predicts that by 2024, 90% of data quality technology buying decisions will focus on ease of use, automation, operational efficiency, and interoperability as critical decision factors.
Data quality is vital in facilitating quality decision-making and is crucial to effective data governance practices.
Organizations can build and incorporate data quality standards and processes into their organizational culture regardless of the data stack used.
Regardless of the toolset, every organization can revamp its data quality guidelines and processes. The road to good data quality, as always, starts with people.
Gartner Magic Quadrant for Data Quality: Related reads #
- Gartner Data Catalog Research Guide — How To Read Market Guide, Magic Quadrant, and Peer Reviews
- A Guide to Gartner Data Governance Research — Market Guides, Hype Cycles, and Peer Reviews
- Gartner Active Metadata Management: Concept, Market Guide, Peer Insights, Magic Quadrant, and Hype Cycle
- Active Metadata: Your 101 Guide From People Pioneering the Concept & It’s Understanding
- The G2 Grid® Report for Data Governance: How Can You Use It to Choose the Right Data Governance Platform for Your Organization?
- The G2 Grid® Report for Machine Learning Data Catalog: How Can You Use It to Choose the Right Data Catalog for Your Organization?
Share this article