Features of Machine Learning Data Catalog - 2025 Guide
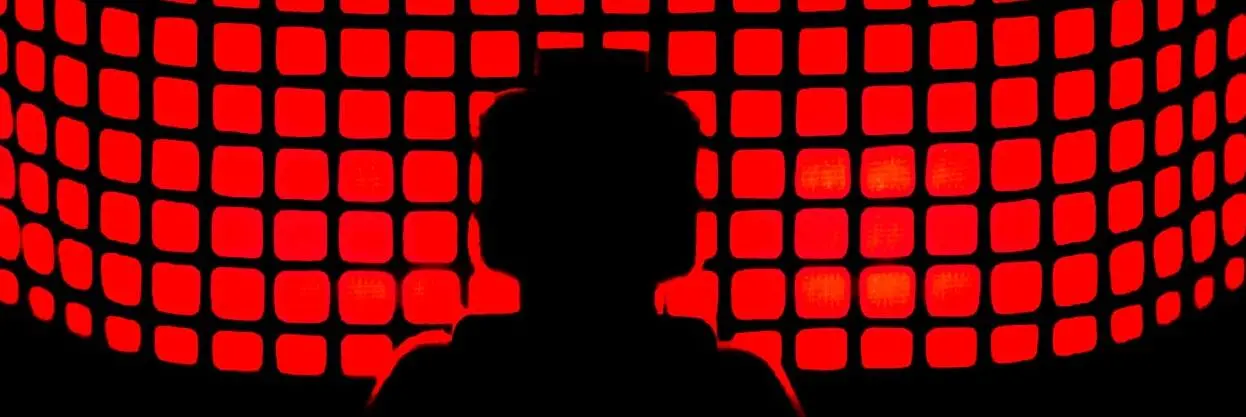
Share this article
What is a machine learning data catalog (MLDC)? #
A machine learning data catalog is a next-generation data catalog that enables real-time data discovery and automates cataloging, crawling of metadata, and classification of PII data.
Machine learning data catalogs are an evolution from traditional data catalogs. Data cataloging or what we at Atlan like to call Data Catalog 1.0 started with simple metadata management for IT Teams right about when data began exploding.
Metadata management in this case included inventorying of data. Then came the concept of data stewardship. With it came the second generation of data catalogs that allowed a few people - data stewards in the organization to manage metadata, set & maintain governance practices, and manually catalog data.
But in an era where metadata is as big as data, machine learning data catalogs are an essential evolution that matches the capabilities of the rest of the modern data stack when it comes to being fast, flexible, and scalable.
Table of contents #
- What is a machine learning data catalog (MLDC)?
- How does machine learning data catalogs work?
- Why do you need machine learning data catalogs?
- What are the key capabilities of a machine-learning data catalog?
- Six essential machine learning data catalog features
- Machine learning data catalog: Related reads
Before we get into machine learning data catalogs, let’s revise:
What is a data catalog? #
Data catalogs are a neatly organized inventory of all data assets across an organization. They seek to solve data discovery, quality & governance issues.
Read our 101 on data catalogs to get the complete lowdown on data catalogs.
“Modern machine-learning-augmented data catalogs automate various tedious tasks involved in data cataloging, including metadata discovery, ingestion, translation, enrichment, and the creation of semantic relationships between metadata. These next-generation data catalogs can therefore propel enterprise metadata management projects by allowing business users to participate in understanding, enriching, and using metadata to inform and further their data and analytics initiatives.” -Gartner, Augmented Data Catalogs 2019
How does machine learning data catalogs work? #
Once configured, the machine learning data catalog would:
- Crawl your data sources (on-premise or cloud data warehouses, lakes, databases)
- Understand and interpret technical metadata
- Create business descriptions and other such information to catalog data with context automatically
- Run periodic audits to verify the accuracy, quality, and integrity of data
Fundamentally data cataloging process includes most of the following steps:
- Discover what data exists and the relations between data
- Enrich & annotate metadata for more context
- Classify & govern data
- Make it easier for users & other services in an organization to discover, trust and use data.
Machine learning data catalogs just intend to automate all of the above processes to ensure more efficiency & productivity of data teams.
Why do you need machine learning data catalogs? #
Machine learning data catalogs (MLDCs) that simplify finding and inventorying siloed data assets are a crucial first step in data and analytics projects.
Gartner predicts that over 60% of traditional data catalog projects that don’t use machine learning to find and inventory data will fail.
Challenges with traditional data catalogs #
Maintaining traditional data catalogs is excruciating because organizations:
- Generate petabytes of data every day
- Store data in messy, unclassified, and unusable formats
- Handle most aspects of cataloging manually
Data consumers cannot use obsolete, unverified data to inform business decisions. With increasingly tighter regulations on data security, integrity, and privacy, that can burn a hole in the pocket. Even a minor GDPR infringement would cost either €10 million or 2% of your annual revenue, whichever amount is higher.
The consequences of using traditional data catalogs #
Legacy data catalogs require extensive manual intervention, leading to:
- Endless delays in projects
- Hefty fines for not complying with data-related regulations (GDPR, CCPA, and cohort)
- Difficulties in cross-team collaborations (in an increasingly distributed environment)
What are the key capabilities of a machine-learning data catalog? #
According to G2, a machine learning powered data catalog should:
- Organize and consolidate data in a single repository (i.e., a single source of truth)
- Allow data consumers (especially business users) to search for and access the data they need
- Let users categorize, comment and share data sets easily to improve collaboration
- Offer intelligent recommendations (using machine learning algorithms) to relevant data
- Enable user access management (UAM) for better data governance
Six essential features #
While evaluating them, look for these essential machine learning data catalog features:
- Auto-cataloging
- Google-like semantic search
- Automated data lineage mapping
- Easy collaboration
- Automated quality audits and governance
- Intelligent recommendations
1. Auto-cataloging #
A machine learning data catalog should automate tedious aspects of cataloging such as crawling metadata, classifying PII data, and profiling for quality (missing values, outliers, and other anomalies). Regardless of where the data comes from (cloud warehouses, data lakes, or RDBMS), the catalog must be able to find and organize it.
How does this help?
Take this instance where there’s a bunch of retail sales data that are most commonly used by the revenue and marketing team to work on offers but naturally they need not have access to PII data that will automatically come with the same data assets Auto-cataloging can help identify the PII data and help mask it only to be revealed to people with authorized access.
Regardless of where the data comes from (cloud warehouses, data lakes, or RDBMS), the catalog must be able to find and organize it.
Auto-cataloging on Atlan data catalog- Source: Atlan.
Auto-classified data sets with adequate context help data consumers interpret the data and use it to make strategic decisions.
2. Google-like semantic search #
Smart data catalogs like MLDCs should empower business and technical users alike to run “Google-like” searches on the metadata to address business outcomes.
Google-like semantic search on data catalog - Source: Atlan.
Typing “Sales” on the search window should display a list of relevant data sets, which can be fine-tuned using filters on data type, source, format, and more.
The catalog should also provide one search window for all data and dashboards to improve user experience and make working with data a breeze.
We have reached a day and age where every person in an organization is a data user, but it’s not possible for everyone to learn to run SQL queries. Machine learning data catalogs help make the search as visual and intuitive as a search engine making data discovery easy for everyone.
3. Automated data lineage mapping #
Data lineage shows the origins of data sets, how they’ve evolved through their lifecycles, and foresees the assets that will be affected by future changes. Proving lineage for building trust in data and ensuring compliance warrants tracking the transformation that data sets undergo.
Lineage also helps build better, more relevant models. So, MLDCs should be able to parse through your query logs in your data warehouses, data lakes, and other data sources automatically to create a visual map of data lineage. Often lack of knowledge is a deterrent to action that can create real value.
Lineage is not just about bug fixes, it’s also about giving a data user the confidence to go ahead and make changes they want in data with clear visibility of impact. Propagation through lineage also allows carrying compliance and security tags to data flowing from particular assets.
Automated data lineage mapping on data catalog - Source: Atlan.
An MLDC would track every transformation that a data set undergoes and represent it graphically to help users verify its lineage.
4. Easy collaboration #
In the post-pandemic era, distributed teams are here to stay. MLDCs should facilitate collaboration across teams and geographies within organizations with in-built features for in-line chats, comments, annotations, data set ratings, and sharing data sets with a single URL.
Modern data catalogs let data consumers discuss and collaborate within the platform through features like chats, comments, and more.
5. Automated quality audits and governance #
Automated quality audits are an excellent way to ensure data quality, integrity, and trustworthiness. Running scheduled audits to spot data regressions, data loss, or distributional shifts over time can help certify data accuracy.
Running scheduled audits to verify the quality and integrity of data is a great way to certify its accuracy and usability.
Tracking data usage right from the source is essential for better governance. Additionally, modern data catalogs simplify governance for IT and data stewards by providing a single dashboard to establish policies and manage access logs and requests.
Handling access from a single window reduces delays in authorizing requests, which removes bottlenecks and simplifies the lives of your IT teams.
6. Intelligent recommendations #
Intelligent recommendations of other data sets that might be relevant or of interest to data consumers enhance the overall user experience.
Just like the “People also ask” and “Searches related to…” sections on Google search, this feature can show similar data sets or curate data that match the user’s search criteria to increase the value derived from data.
A quick recap on the machine learning data catalog #
- Machine learning data catalogs (MLDCs) enable real-time data discovery and automatic cataloging of data sets with adequate context.
- With MLDCs, organizations can build a single source of truth for all data, track lineage, and search and access the right data via a single dashboard.
- Modern, augmented data catalogs facilitate improved collaboration within the organization, empower all data users to make data-driven decisions, simplify governance, and facilitate data democratization.
- While evaluating data catalogs, look for automated ingesting, inventorying, tagging, profiling, lineage mapping, and enrichment of data sets.
Can machine-learning data catalogs increase business value? #
Machine learning data catalogs can definitely increase business value. They effectively contribute towards reducing speed to insights, greater business engagement, retention of great data talent - and of course, better utilization of data and the modern data stack.
Are you looking to deploy a machine-learning data catalog that will be the perfect fit for your business requirements? Your business requirements can range from data democratization initiatives to data governance strategy setups, to something as simple as increasing the efficiency of your data team - use this step-by-step checklist to create your customized evaluation criteria framework. This way you get a clear picture - of the machine learning data catalog under consideration matching your immediate and long-term business needs.
Enterprises using Atlan’s machine-learning-augmented data catalog have reported:
- Up to 60x speed to insight
- 70% greater business engagement
- ROI realization within two weeks
Take a quick 3-minute Demo | Speak to our team about your requirements
Machine learning data catalog: Related reads #
- Enterprise data catalog: Definition, Importance & benefits
- What Is a Data Catalog? & Do You Need One?
- Data catalog benefits: 5 key reasons why you need one
- Open Source Data Catalog Software: 5 Popular Tools to Consider in 2024
- AI Data Catalog: Exploring the Possibilities That Artificial Intelligence Brings to Your Metadata Applications & Data Interactions
- Business Data Catalog: Users, Differentiating Features, Evolution & More
- Top Data Catalog Use Cases Intrinsic to Data-Led Enterprises
- AWS Glue Data Catalog: Architecture, Components, and Crawlers
- Airbnb Data Catalog — Democratizing Data With Dataportal
- Lexikon: Spotify’s Efficient Solution For Data Discovery And What You Can Learn From It
- Google Cloud Data Catalog Guide - Everything You Need to Know
Share this article