Modern Data Management: 8 Things You Can Gain From It
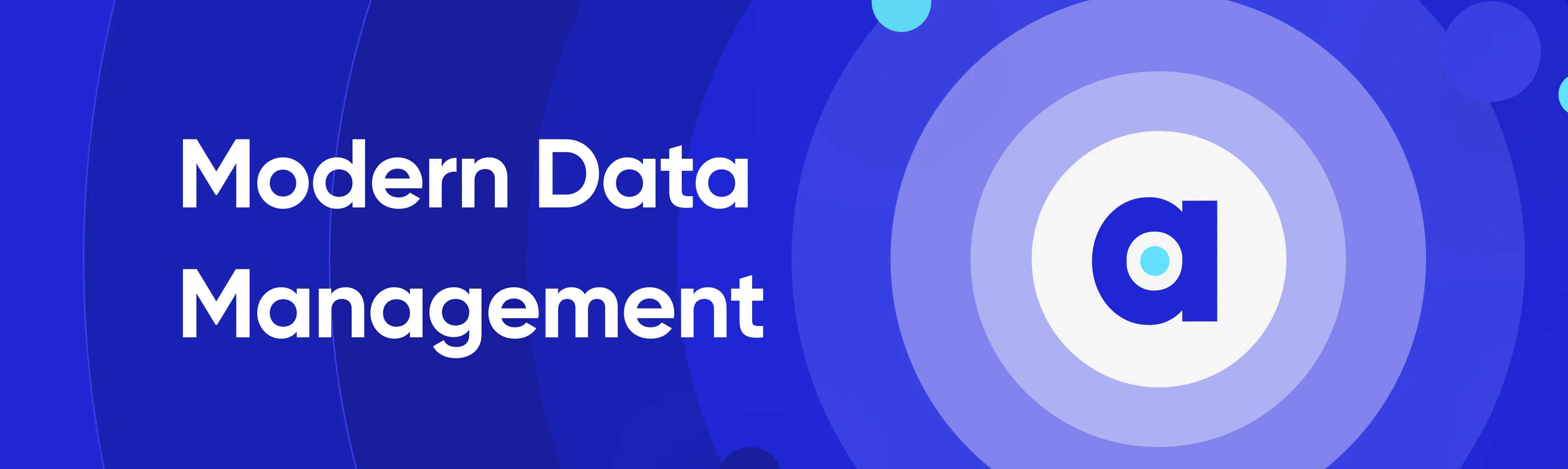
Share this article
Modern data management involves a set of practices, techniques, and technologies used to handle data as a valuable resource. Its aim is to ensure the availability, integrity, security, and usability of data within an organization.
In this blog, we will understand what is modern data management in detail, how it differs from traditional data management, and how business leaders stand to gain from it.
Let’s go!
Table of contents #
- Unlocking the power of data: A deep dive into modern data management
- Exploring the features and benefits of a modern data platform
- Exploring the key distinctions: modern data management vs. traditional data management
- Traditional vs modern data management: A tabular view
- 8 Benefits of modern data management for business leaders
- Immediate and long-term steps to embrace modern data management in your organization
- Summary
- Modern data management: Related reads
Unlocking the power of data: A deep dive into modern data management #
In this section, we’ll take a detailed look at modern data management, including some specifics about modern data platforms:
- Data governance
- Data quality management
- Data integration and interoperability
- Master data management (MDM)
- Metadata management
- Data security
- Data privacy
- Data operations (DataOps)
Let’s explore these specifics in detail below:
1. Data governance #
- This is a set of processes that ensure the data meets the necessary standards of quality, consistency, and security.
- This involves developing and implementing data policies, standards, practices, and procedures.
- Modern data governance also encompasses issues such as data privacy, compliance, and ethical data usage.
2. Data quality management #
- This practice involves maintaining high-quality data through the continuous measurement and improvement of data’s accuracy, completeness, relevance, and timeliness.
- Modern data quality management often involves automated processes for cleaning and standardizing data, as well as tools for monitoring and reporting on data quality.
3. Data integration and interoperability #
- This involves combining data from different sources and providing a unified view.
- Modern data integration techniques often leverage APIs and microservices architectures to enable data to flow seamlessly between different systems.
4. Master data management (MDM) #
- This is the process of creating a single, accurate, and authoritative source of truth for a company’s essential data.
- MDM helps avoid duplication and inconsistencies, thereby ensuring that different parts of the organization are using the same data.
5. Metadata management #
- Metadata is data about data. Managing metadata helps keep track of what data is available, where it’s from, who’s responsible for it, and how it’s formatted, among other things.
- Modern metadata management tools often use AI to automate metadata creation and maintenance.
6. Data security #
- This involves protecting data from unauthorized access and breaches.
- Modern data security practices involve not only protecting the data itself but also the metadata and the data pipelines.
7. Data privacy #
- This involves ensuring that data is collected, stored, and used in compliance with laws and regulations, such as GDPR and CCPA.
- Modern data privacy management involves practices like anonymization, pseudonymization, and encryption.
8. Data operations (DataOps) #
- This practice aims to improve the speed, quality, and reliability of data analytics.
- It involves automating many data management tasks and establishing a culture of collaboration between data teams and other stakeholders.
Exploring the features and benefits of a modern data platform #
Now, let’s talk about the Modern Data Platform and how it fits into the broader scope of modern data management.
A modern data platform is a technological solution that supports all the above aspects of data management.
It’s characterized by being:
- Flexible
- scalable
- Capable of handling diverse types of data
It enables self-service capabilities for a diverse range of users and agile data management. Modern data platforms are also designed for the cloud, allowing for quick setup and a pay-as-you-go model.
These platforms typically have several layers, including:
- Data ingestion
- Storage, transformation
- Analysis
They often feature:
- Data catalogs for metadata management
- Data governance tools
- Security features
Many also support real-time data processing and advanced data science use cases.
In summary, modern data management is a comprehensive approach to managing, processing and leveraging data in today’s complex and dynamic business environment.
It involves a combination of practices, from data governance and quality management to integration, security, and privacy.
These practices are often supported by a modern data platform, a technology solution designed to handle the demands of modern data management.
Exploring the key distinctions: Traditional data management vs. modern data management #
Traditional and modern data management differ in several fundamental ways, largely due to the changing nature of data, advancements in technology, and shifts in business needs. Here are some key differences:
- Scale and variety of data
- Technology
- Data governance and quality
- Self-service
- Real-time and predictive capabilities
Let’s explore these in detail:
1. Scale and variety of data #
- Traditional data management was primarily focused on structured data that was housed in relational databases.
- Modern data management, on the other hand, is designed to handle the vast volumes of structured, semi-structured, and unstructured data that businesses generate today.
- This includes everything from traditional databases to big data from social media, IoT devices, and more.
2. Technology #
- Traditional data management typically relied on monolithic, on-premises systems for storage and processing.
- These systems could be rigid and difficult to scale. In contrast, modern data management makes use of flexible, cloud-based technologies that allow for scalability, agility, and distributed processing.
- This shift allows organizations to manage large volumes of data more effectively and to adjust their computing resources based on demand.
3. Data governance and quality #
- While traditional data management did include governance and quality checks, these were often manual, cumbersome processes.
- Modern data management, however, leverages automation and advanced algorithms to ensure data quality, consistency, and compliance.
- This leads to more efficient, reliable, and scalable data governance.
4. Self-service #
- Traditional data management often required specialized IT personnel to extract and prepare data for analysis.
- Modern data management, on the other hand, is characterized by self-service capabilities, where business users can access and analyze data themselves without extensive technical skills.
- This democratization of data helps accelerate decision-making and fosters a data-driven culture within organizations.
5. Real-time and predictive capabilities #
- Traditional data management was largely based on historical, batch-processed data.
- Modern data management often incorporates real-time data processing and predictive analytics capabilities. It allows businesses to respond to changes as they occur and make proactive and data-driven decisions.
- Together, it can drive innovation, improve operational efficiency, and enhance customer experiences.
- This evolution is shifting companies from reactive, historical data analysis towards a proactive and forward-thinking approach to business strategy.
Traditional vs modern data management: A tabular view #
While we have seen the differences between traditional and modern data management in a descriptive manner, let’s see them in a comparison table:
Traditional Data Management | Modern Data Management | |
---|---|---|
Data Types | Primarily structured data | Structured, semi-structured, and unstructured data |
Technology | Monolithic, on-premises systems | Cloud-based, scalable technologies |
Scale | Limited scalability | Highly scalable to handle big data |
Data Governance and Quality | Manual processes | Automation and advanced algorithms |
Access to Data | Primarily IT and data professionals | Democratized; self-service for various roles |
Processing | Batch processing; historical data | Real-time processing; predictive analytics |
Data Storage | On-premises databases | Cloud storage, data lakes, data warehouses |
Integration | Limited, often difficult | Advanced data integration, APIs, microservices |
Security | Perimeter-based security | Data-centric security; advanced threat detection |
Flexibility | Rigid, slow to change | Agile, adaptable to business needs |
Remember that the degree to which these characteristics apply can vary widely among organizations. It depends on their specific circumstances, maturity, and the particular technologies they have adopted.
8 Benefits of modern data management for business leaders #
As a business leader, paying attention to modern data management can bring numerous benefits to your organization:
- Informed decision making
- Improved efficiency
- Scalability
- Competitive advantage
- Risk management
- Customer insights
- Operational optimization
- Cost savings
Let’s dive into each of these benefits in brief:
1. Informed decision making #
- Modern data management tools can provide better insights, in real-time, that enable data-driven decision-making.
- This could mean improved operational efficiency, higher customer satisfaction, and potentially greater profit margins.
2. Improved efficiency #
- With self-service capabilities and automation, employees across your organization can access and utilize data more efficiently.
- This not only frees up your IT team’s resources but also fosters a data-driven culture where all departments can leverage data in their work.
3. Scalability #
- Modern data management systems are designed to grow your business. They can handle vast volumes of data and adjust to fluctuating demand.
- This ensures that your data infrastructure won’t hinder your growth.
4. Competitive advantage #
- Leveraging modern data management can provide you with a competitive edge.
- Real-time insights, predictive analytics, and the ability to handle diverse data can all lead to innovative products, services, and business models.
5. Risk management #
- Modern data management typically includes advanced security measures and compliance tools, which are vital in the era of cyber threats and stringent data privacy regulations.
- Effective data management can help protect your organization’s reputation and avoid costly fines.
6. Customer insights #
- Understanding your customers is key to improving your product and service offerings.
- Modern data management allows for the consolidation and analysis of various data sources, providing a more holistic view of customer behavior and preferences.
7. Operational optimization #
- With modern data management, you can streamline your operations by identifying bottlenecks, inefficiencies, or opportunities for automation in your processes.
8. Cost savings #
-
While transitioning to modern data management requires an initial investment, the efficiency, scalability, and insights it offers can lead to significant cost savings in the long term.
-
Given the above, modern data management should be seen not just as an IT concern, but as a strategic imperative that can drive significant value for your organization.
How to embrace modern data management in your organization: Immediate & long-term steps #
Transitioning to modern data management involves both immediate and long-term steps. Here’s a roadmap to get you started:
Immediate steps #
- Assess your current data management practices
- Define your data strategy
- Identify key stakeholders
- Create a data governance framework
Let’s look into each of the above short-term steps in brief:
1. Assess your current data management practices #
- Before you can decide where to go, you need to understand where you are.
- Evaluate your current data management processes, tools, and infrastructure, and identify gaps or challenges.
- This could involve surveying your staff, reviewing your IT assets, or even bringing in an external consultant.
2. Define your data strategy #
- Align your data management efforts with your business goals.
- What kind of data do you need to collect and analyze to achieve these goals? Who will need access to this data, and how will they use it?
- Your data strategy should guide all your subsequent decisions about data management.
3. Identify key stakeholders #
- Modern data management should not be the sole responsibility of your IT department.
- Involve stakeholders from across your organization, including leadership, operations, finance, and marketing.
- Each of these groups will have unique perspectives and needs when it comes to data.
4. Create a data governance framework #
- Develop policies and procedures for how data will be collected, stored, accessed, and used in your organization.
- This should also cover data security and privacy considerations.
Long-term steps #
- Select and implement data management tools
- Ensure data quality
- Provide training and support
- Promote a data-driven culture
- Monitor, evaluate, and adjust
Let’s look into each of the above long-term steps in brief:
1. Select and implement data management tools #
- Based on your data strategy and governance framework, choose the technologies that will form your modern data platform.
- This could include data ingestion tools, data lakes or warehouses, data transformation tools, business intelligence tools, and more.
2. Ensure data quality #
- Implement processes to ensure the accuracy, completeness, and consistency of your data.
- This could include data validation rules, data cleaning processes, and data quality monitoring tools.
3. Provide training and support #
- Ensure your staff is equipped to use your new data management tools effectively.
- This might involve training sessions, documentation, and ongoing IT support.
4. Promote a data-driven culture #
- Encourage your staff to make data-driven decisions.
- This could involve leadership modeling data-driven decision-making, providing staff with access to data and tools, and celebrating successes that come from data-driven insights.
5. Monitor, evaluate, and adjust #
- Once you’ve implemented your modern data management system, it’s important to continually monitor its performance, evaluate its effectiveness, and make adjustments as necessary.
- Data management is not a “set it and forget it” endeavor, but rather a continuous process of improvement.
Remember, transforming your data management practices is a significant undertaking that will take time and resources, but the benefits can be substantial. Besides, it is not a linear process; you may find that you need to revisit earlier steps based on what you learn later in the process.
Summary #
In conclusion, modern data management leverages cloud technologies, self-service capabilities, advanced analytics, and machine learning to provide scalable, real-time insights. This can significantly benefit organizations by improving decision-making, efficiency, scalability, competitive advantage, risk management, customer insights, operational optimization, and cost savings.
To deploy modern data management practices, organizations should assess current practices, define a data strategy, identify key stakeholders, create a data governance framework, choose and implement data management tools, ensure data quality, provide training and support, promote a data-driven culture, and monitor and adjust as necessary.
Modern data management: Related reads #
- The Future of the Modern Data Stack in 2023 - 4 new emerging trends and 6 big trends from last year
- Metadata Management: Benefits, Automation, Best Practices, and Tools
- What is metadata management and why is it so important?
- Top 6 Metadata Management Best Practices for 2023
- Active Metadata: Definition, Characteristics, Use Cases & More
- Enterprise Metadata Management and Its Importance in the Modern Data Stack
- Snowflake Metadata Management: Importance, Challenges, and Identifying The Right Platform
- Databricks Metadata Management FAQs, Tools, Getting Started
- Data Catalog Vs. Metadata Management: Differences, and How They Work Together?
- Difference between Master Data Management(MDM) and Metadata Management
Share this article