Modern Metadata Management: What It Promises, Key Features & Factors to Consider for Improved Data Governance
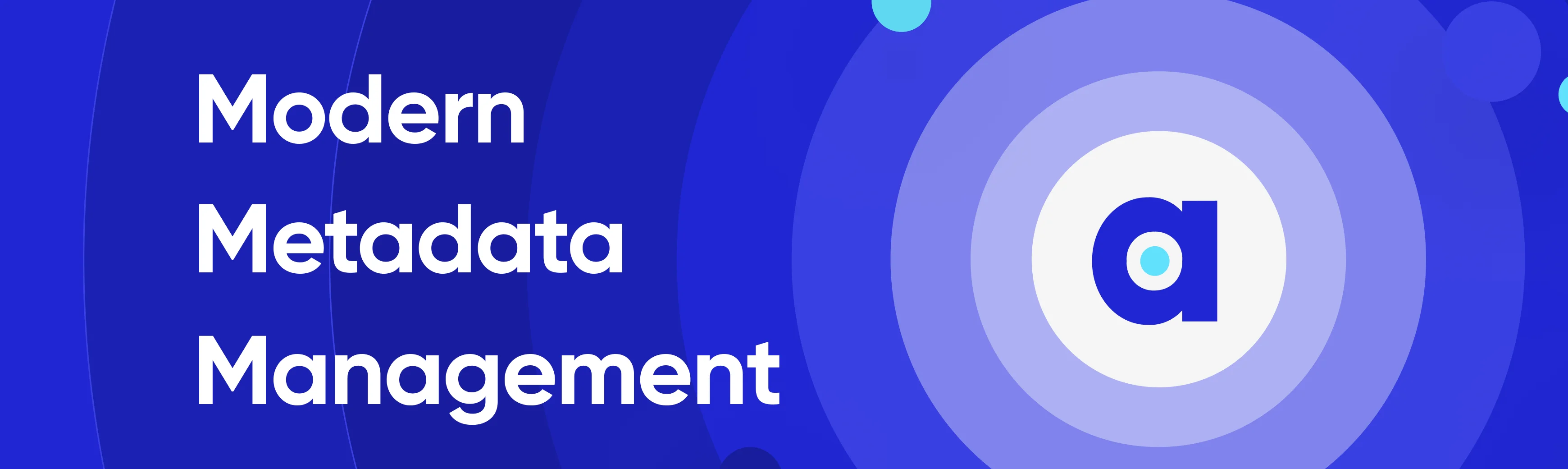
Share this article
Modern metadata management is at a crucial point of evolution, necessitating a shift in approach to keep up with the rest of the data infrastructure. This new approach acknowledges that today’s diverse data teams, made up of engineers, analysts, data scientists, and more, require a more comprehensive, flexible, and collaborative system for metadata.
In this context, “modern” refers to a solution that mirrors the speed, flexibility, and scalability of the current data stack. As data tools and methods diversify, the management of metadata must adapt to cater to the unique needs of these varied data consumers.
Current solutions, while having made significant strides, often fall short of providing a single, unifying view of an organization’s data assets.
Table of contents #
- How modern metadata management solutions can transform your data management?
- Evolving metadata management: Key characteristics for the modern era (With examples)
- Modern metadata management: Best practices
- What to look for in a modern metadata management solution?
- Summary
- Modern metadata management: Related reads
How modern metadata management solutions can transform your data management? #
A new generation of metadata management is therefore required, one that expands beyond tables to include :
- BI dashboards
- Code snippets
- SQL queries
- Models
- Features
- Jupyter notebooks as data assets
This modern approach treats metadata as a form of data that can be analyzed and managed like any other kind of data.
Furthermore, it should be able to cope with the increasing volume of metadata, almost making metadata itself a form of big data.
One of the key traits of this novel approach to metadata management is end-to-end visibility. Rather than piecemeal solutions, it offers a single source of truth about every data asset in the organization. This includes lineage, quality, and preparation details for each data asset.
Additionally, in a world where data has become more democratized and collaboration is paramount, modern metadata management systems should be designed to embed seamlessly into the workflows of diverse data users.
They should provide:
- Intuitive and interactive interfaces
- Promote communication and collaboration
- Integrate effectively with other tools to make various micro-tasks delightful
While we may not know the exact form this modern approach to metadata management will take, it’s clear that the forthcoming transformation will reflect the needs of today’s data-driven world. The focus will be on addressing the limitations of the past and creating a solution that can adapt to the constantly evolving landscape of data technology.
Evolving metadata management: Key characteristics for the modern era (With examples) #
Now, let’s elaborate on the key characteristics of modern metadata management:
- Data assets are more than tables
- End-to-end data visibility
- Metadata as “big data”
- Embedded collaboration
Let’s dive deeper into each of these characteristics:
1. Data assets are more than tables #
- Traditional metadata management primarily dealt with data tables.
- In the modern data environment, there are several other types of data assets that are just as crucial, such as BI dashboards, code snippets, SQL queries, models, features, and Jupyter notebooks.
- For instance, a business analyst might create a BI dashboard that uses data from several tables, which is then shared with a product manager for decision-making.
- In this case, the BI dashboard itself becomes an important data asset that needs to be cataloged and managed in the metadata system.
2. End-to-end data visibility #
- Modern metadata management systems aim to provide a single source of truth for all data assets.
- This means integrating data lineage tools, data quality tools, data prep tools, and more into one cohesive system.
- For example, when looking at a particular data asset, a data scientist should be able to see
- Its origin (lineage)
- Any transformations it has undergone (prep)
- Its current status (quality), and
- Where it is being used (like in which dashboards or reports)
3. Metadata as “big data” #
- With the increasing volume of metadata, handling it effectively becomes a task akin to dealing with big data.
- For instance, one can consider the logs generated by user queries in a system like Snowflake.
- These logs can be parsed to create a column-level lineage, assign popularity scores to data assets, and even deduce the potential owners and experts for each asset.
- This is an example of treating metadata as a form of data itself, which can be analyzed and utilized for better data management and decision-making.
4. Embedded collaboration #
- The concept of embedded collaboration is about making work happen where you are, with the least amount of friction.
- In the context of metadata, this involves integrating the metadata system seamlessly with the workflows and tools that data teams are already using.
- For instance, when a data analyst encounters an issue with a data asset, they should be able to report it directly from the metadata system. This issue could then be automatically logged in a tracking system like JIRA, without requiring the analyst to manually enter it.
- Similarly, requests for data access, approvals, and other tasks should be able to be carried out directly within the metadata system.
- Besides, notifications should be sent through common communication channels, like Slack or email.
These characteristics combined can make metadata management more effective, efficient, and adaptable to the dynamic needs of today’s diverse data teams.
Modern metadata management best practices #
Modern metadata management involves the organization and administration of all data that describes other data. This has become a critical aspect of modern data governance and management. It is used in data warehousing, data integration, data analysis, and more.
Proper metadata management provides transparency and understanding about data origins, transformations, and usability, which in turn enables businesses to make informed decisions and comply with data regulations.
Modern metadata management best practices involve:
- Defining a metadata management strategy
- Establishing metadata standards
- Utilizing a metadata repository
- Implementing automation in metadata collection
- Ensuring metadata quality
- Prioritizing security and compliance
- Providing metadata accessibility
- Encouraging collaboration and user engagement
- Fostering a metadata-driven culture
- Regularly reviewing and updating metadata policies
Let us look at each of the above best practices in detail:
1. Defining a metadata management strategy #
A well-defined strategy should be the starting point of effective metadata management. It involves determining the organization’s goals related to metadata management, which may include improving data quality, promoting data understanding, and ensuring compliance with regulations.
2. Establishing metadata standards #
Metadata should follow specific standards to ensure consistency, compatibility, and usability. The standards can be industry-wide or organization-specific. They include the metadata types that need to be collected, the method of collection, and the formats to be used.
3. Utilizing a metadata repository #
A centralized metadata repository is crucial for effective metadata management. It provides a common platform where all metadata can be stored, managed, and accessed. A metadata repository also helps in maintaining the integrity of metadata and provides a single source of truth.
4. Implementing automation in metadata collection #
With the increase in data volume, it becomes challenging to manually collect and manage metadata. Automated tools and solutions should be employed for efficient and accurate metadata collection and updating.
5. Ensuring metadata quality #
The quality of metadata is as crucial as the quality of the data it describes. There should be checks and measures to ensure the accuracy, completeness, and timeliness of metadata.
6. Prioritizing security and compliance #
Security measures should be put in place to protect sensitive metadata. Additionally, compliance with data regulations, such as GDPR and CCPA, should be ensured. The metadata should be managed in a way that supports the fulfillment of these requirements.
7. Providing metadata accessibility #
Metadata should be easily accessible to the relevant stakeholders. However, the level of accessibility can vary depending on the role of the user. A balance between accessibility and security should be maintained.
8. Encouraging collaboration and user engagement #
To make the best use of metadata, it is essential to encourage user engagement. Training and collaboration tools can be used to involve more people in the metadata management process and to make it more user-friendly.
9. Fostering a metadata-driven culture #
Promote a culture where decisions are made based on metadata. This involves training employees to understand and utilize metadata and ensuring management’s commitment towards metadata-driven decision making.
10. Regularly reviewing and updating metadata policies #
As business needs and technologies change, metadata management practices should also evolve. Regular reviews and updates should be carried out to ensure that the metadata management practices are aligned with the current needs and environment.
Following these best practices for metadata management can help organizations better understand their data, improve data quality, increase operational efficiency, and ensure regulatory compliance.
What to look for in a modern metadata management solution? #
If an organization wants to adopt modern metadata management, they should look for a tool with the following core capabilities:
- Support for managing various data assets
- Comprehensive data visibility
- Scalability
- Embedded collaboration
- Cloud-based and elastic
- User-friendly and collaborative interface
- Automated metadata collection
- Advanced search and discovery capabilities
- Security and compliance features
Let’s understand these capabilities further:
1. Support for managing various data assets #
- The tool should not only manage data tables but also handle other types of data assets, including BI dashboards, code snippets, SQL queries, data models, features, Jupyter notebooks, etc.
- This ensures that all data-related assets are cataloged and made discoverable.
2. Comprehensive data visibility #
- It should provide a holistic view of all data assets.
- It needs to integrate information from various data tools into one single source of truth.
- Users should be able to see the lineage, transformations, quality, and usage of each data asset, facilitating trust and reliability in the data.
3. Scalability #
- Given that metadata itself is becoming “big data”, the tool needs to be scalable.
- It should be able to handle large volumes of metadata, process it quickly, and provide insights based on the analysis of the metadata.
4. Embedded collaboration #
- The tool should support seamless integration with workflows and tools that data teams already use.
- This could mean reporting issues directly from within the tool, triggering access requests, and integrating notifications with communication tools like Slack or email.
5. Cloud-based and elastic #
The tool should ideally be cloud-based and leverage the advantages of the cloud such as elasticity, ease of setup, and scalability. This ensures the tool can be updated and improved without significant IT overhead.
6. User-friendly and collaborative interface #
- The tool should be designed with the user experience in mind, ensuring that various roles within the data team can use it effectively.
- It should promote collaboration and communication among team members, reducing friction and promoting productivity.
7. Automated metadata collection #
- The tool should automate the collection and updating of metadata as much as possible.
- This reduces the workload on data stewards and increases the speed and accuracy of metadata management.
8. Advanced search and discovery #
- Users should be able to easily search and discover data assets within the tool.
- This would include features like search auto-completion, faceted search, and the ability to rank results based on relevance or popularity.
9. Security and compliance #
- Last but not least, the tool must ensure the security of the data and metadata it manages.
- It should provide controls for data access and support compliance with data governance policies and regulations.
Such a tool would truly represent a modern approach to metadata management, fitting seamlessly into the modern data stack and addressing the needs of diverse data teams.
Summary #
Modern metadata management is undergoing a significant evolution to meet the demands of today’s data-driven world. By adopting a modern metadata management solution that encompasses these capabilities, organizations can establish a robust foundation for effective data management, enhance collaboration across data teams, and enable data-driven decision-making.
Embracing the evolving nature of metadata management will empower organizations to navigate the ever-changing data landscape with confidence and achieve their data-driven goals.
Modern metadata management: Related reads #
- Types of metadata
- The difference between Data and Metadata
- Metadata Management 101
- Active Metadata: 101 Guide From Pioneers Of The Concept
- What is Metadata? - Examples, Benefits, and Use Cases
- What Is a Metadata Catalog? — Basics & Use Cases
- What is Metadata Management and Why is it So Important?
- How Operational Metadata Drives Business Success: A Comprehensive Guide
- Mastering Business Metadata: How to Achieve Data Excellence and Streamlined Collaboration
- 6 metadata management best practices to follow in 2023
Share this article