What is an Operational Data Store: A Complete Guide for 2024
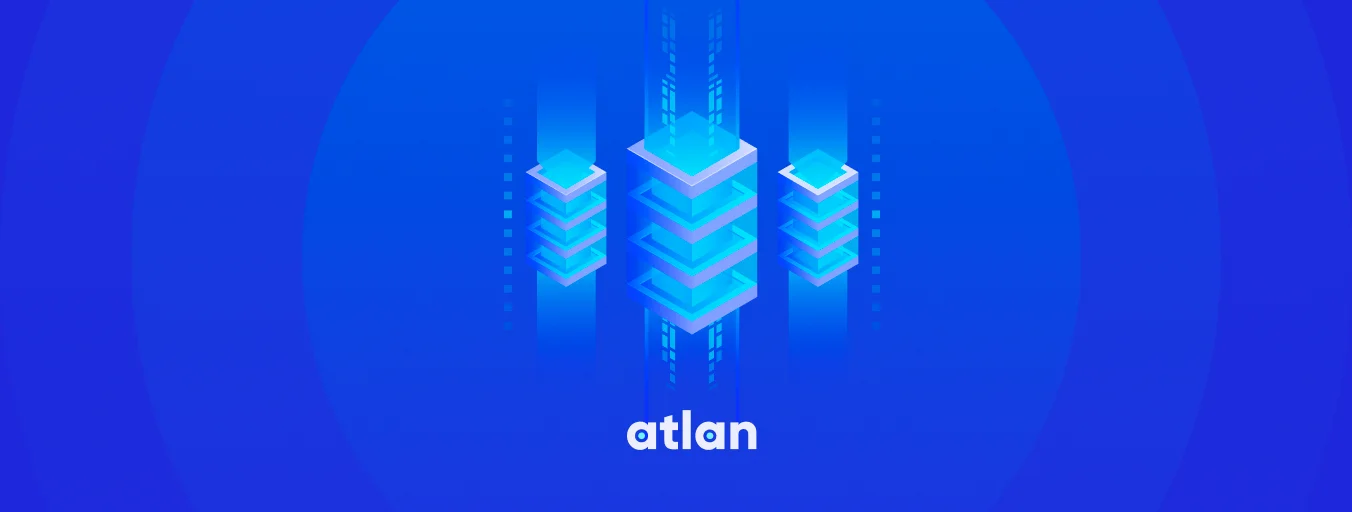
Share this article
Operational data store provides a consolidated and up-to-date repository of operational data from various sources. This helps organizations to have a single source of truth and facilitates real-time data access and integration.
In many businesses, data is often distributed across different systems, making it challenging to obtain a comprehensive and real-time view of their operations. Operational data store helps in bringing this data together, which is crucial for making informed, timely decisions.
Modern data problems require modern solutions - Try Atlan, the data catalog of choice for forward-looking data teams! 👉 Book your demo today
This article has provided an in-depth exploration of operational data store, shedding light on its key concepts and its critical role in modern organizations.
Lets dive in!
Table of contents #
- What is an operational data store?
- 7 Key examples of an operational data store
- How do operational data stores work?
- 8 Essential benefits of an operational data store
- Types of operational data store
- How can your business make use of an operational data store?
- Operational data store vs. data warehouse vs. data lake
- Summarizing it all together
- Operational data store: Related reads
What is an operational data store? #
An operational data store is a centralized repository of integrated, subject-oriented, and detailed data that is specifically designed to support operational and tactical decision-making within an organization.
It plays a crucial role in providing real-time visibility and access to the data necessary for day-to-day business operations and decision-making processes.
Key characteristics and purposes of an ODS #
- Integration
- Subject-oriented
- Detailed data
- Real-time or near real-time
- Tactical decision support
Let’s discuss the characteristics in details:
- Integration: An operational data store integrates data from various source systems, such as transactional databases, external data feeds, and other operational data sources. This integration helps ensure that data from different parts of the organization is available in one place.
- Subject-oriented: An operational data store is typically organized around specific subjects or business areas, making it easier for business users to access the data they need based on their specific needs. For example, a retail operational data store might focus on sales, inventory, and customer data.
- Detailed data: An operational data store stores granular, detailed data, as opposed to data warehouses or data marts, which often contain aggregated or summarized data. This granularity allows for a more in-depth analysis and better support for operational decision-making.
- Real-time or Near real-time: In industries like retail, financial services, and manufacturing, where quick decisions are crucial, operational data store is often updated in real-time or near real-time. This ensures that the data is always current, enabling timely decisions.
- Tactical decision support: operational data store primarily serves the needs of operational and tactical decision-makers. It provides data that helps answer questions related to day-to-day operations, such as inventory management, order processing, and customer interactions.
7 Key examples of an operational data store #
Understanding examples of an operational data store is crucial for grasping its practical applications and optimizing its benefits, enabling efficient data management and decision-making in real-world scenarios. It provides tangible insights into how operational data store functions and enhances its strategic implementation for improved operational efficiency.
Here are some examples of how an operational data store might be used in different industries:
- Retail
- Healthcare
- Banking and Finance
- Manufacturing
- Telecommunications
- E-commerce
- Energy and utilities
Let’s delve into the details of the examples.
1. Retail #
- Inventory management: An operational data store can store real-time inventory data from multiple stores and distribution centers, helping retailers monitor stock levels, track sales, and optimize replenishment.
- Point of Sale (POS) data: operational data store can collect transaction data from various stores, enabling retailers to analyze sales patterns, customer behavior, and pricing strategies.
2. Healthcare #
- Patient records: An operational data store can store patient information from electronic health records (EHR) systems, making it easier for healthcare providers to access up-to-date patient data, such as medical history, prescriptions, and test results.
- Billing and claims: Healthcare organizations can use an operational data store to process insurance claims, track payments, and manage billing data.
3. Banking and finance #
- Transaction processing: Operational data store can capture and process banking transactions, including deposits, withdrawals, and transfers, ensuring that account balances are accurate in real-time.
- Fraud detection: An operational data store can be used to detect unusual or suspicious transactions by analyzing data from multiple sources, helping prevent fraud.
4. Manufacturing #
- Production monitoring: Manufacturers can use an operational data store to collect data from sensors and machinery on the factory floor, monitoring equipment performance, quality control, and production efficiency.
- Supply chain management: Operational data store can store data related to the supply chain, including orders, shipments, and inventory levels, helping organizations optimize their supply chain operations.
5. Telecommunications #
- Network performance monitoring: Telecommunications companies can use an operational data store to collect and analyze data from network devices to ensure optimal network performance and identify and resolve issues in real-time.
- Customer billing: Operational data store can store data related to customer billing, including call records, data usage, and subscription plans.
6. E-commerce #
- User behavior tracking: Operational data store can capture user interactions on an e-commerce website, including page views, shopping cart activity, and checkout processes, to optimize the user experience and marketing efforts.
- Product inventory: An operational data store can store real-time product availability and pricing information, ensuring that customers see accurate information when making purchasing decisions.
7. Energy and utilities #
- Smart grid data: Operational data store can store data from smart meters and grid sensors to monitor and manage electricity consumption, grid stability, and renewable energy integration.
- Maintenance and asset management: Utilities can use an operational data store to track the condition and maintenance schedules of their infrastructure assets, such as transformers and substations.
These are just a few examples of how Operational Data Stores can be applied across different industries to support operational processes and decision-making. The specific use cases and data stored in an operational data store can vary widely depending on the organization’s needs and goals.
How do operational data stores work? #
An operational data store is like a central data hub for a company. It collects information from different sources within the company and keeps it organized. Imagine it as a giant filing cabinet for data.
Here’s how it works:
- Data collection
- Making data talk
- Real-time updates
- Storing data
- Getting what you need
- Keeping things tidy
- Sharing and rules
- Sharing with others
- Watching over it
Let’s understand how operational data store work in detail:
-
Data collection: Information from different places, like sales, customer service, and inventory, is gathered and put into the operational data store. It’s like putting all your puzzle pieces in one place.
-
Making data talk: The operational data store takes this information and makes it speak the same language. Think of it as translating data so that everyone can understand it, no matter where it comes from.
-
Real-time updates: The operational data store keeps information fresh, like a constantly updated newsfeed. So, if something changes in the company, the operational data store knows right away.
-
Storing data: All this data is kept in a special storage area, like a safe deposit box for information. It’s organized neatly so you can find what you need.
-
Getting what you need: Whenever someone in the company needs data for their work, they can easily ask the operational data store for it. It’s like asking a librarian for a specific book in the library.
-
Keeping things tidy: Old data that’s not needed anymore might be stored away, so the Operational Data Store doesn’t get cluttered. But important historical data is preserved.
-
Security and rules: The operational data store makes sure only the right people can access certain data. It’s like having locks on some of the filing cabinet drawers.
-
Sharing with others: Sometimes, the operational data store shares data with other systems, like a fax machine sending a copy of a document to another office.
-
Watching over it: People check on the operational data store regularly to make sure it’s working well, like how you might maintain your bike to keep it running smoothly.
An operational data store is like a super-organized data superhero that helps a company keep all its information in one place, updated, and easily accessible for everyone who needs it. It’s like the brain that keeps the company running smoothly.
8 Essential benefits of an operational data store #
Using an operational data store provides several advantages that are crucial for organizations, especially those operating in fast-paced industries.
Here are some of the key benefits of an operational data store:
- Real-time visibility
- Tactical decision support
- Data integration
- Subject-oriented data
- Efficient data processing
- Data quality and accuracy
- Cost-effective
- Support for compliance
Let us understand each of them in detail.
1. Real-time visibility #
Operational data store offers real-time or near real-time access to current and detailed data. This immediate access to data is essential for industries where swift decisions are crucial.
It enables organizations to stay up-to-date with operational processes, track changing conditions, and respond quickly to emerging situations. For example, in stock trading, a real-time operational data store ensures that traders have the most current market data to make informed decisions.
2. Tactical decision support #
Operational data store is specifically designed to support operational and tactical decision-making. It provides detailed data at a granular level, making it suitable for answering questions related to day-to-day operations.
Retailers, for instance, rely on operational data store to manage inventory, adjust pricing, and personalize promotions based on real-time sales data.
3. Data integration #
Operational data store integrates data from various sources within an organization, including transactional databases, external data feeds, and other operational systems. This integration helps eliminate data silos and ensures that data from different parts of the organization is available in one place.
This promotes data consistency and eliminates the need for decision-makers to access and combine data from disparate sources manually.
4. Subject-oriented data #
Operational data store is typically organized around specific subjects or business areas. This makes it easier for business users to access the data they need based on their specific requirements.
For example, a manufacturing operational data store might have sections dedicated to production, quality control, and equipment performance, ensuring that relevant data is readily available to the respective teams.
5. Efficient data processing #
Operational data store often requires minimal data transformation compared to data warehouses. This means that data can be made available for immediate use without complex processing. It reduces the time and resources needed to prepare data for analysis.
6. Data quality and accuracy #
Operational data store places a strong emphasis on data accuracy and consistency. This ensures that the data used for tactical decisions is reliable and trustworthy.
For instance, in the financial services industry, operational data store data is crucial for immediate transaction processing and must be highly accurate to prevent errors and fraud.
7. Cost-effective #
Operational data store typically doesn’t require the same level of storage and processing as data warehouses or data lakes. This made it a cost-effective solution for organizations that need real-time or near-real-time access to operational data.
The focus on operational and tactical data needs also helps reduce unnecessary data storage costs.
8. Support for compliance #
In regulated industries like finance and healthcare, operational data store can help organizations meet compliance requirements by providing a centralized repository of accurate and traceable data.
This is essential for auditing, reporting, and demonstrating adherence to industry regulations.
In short, using an operational data store is essential for organizations that need to make rapid, data-driven operational decisions. It offers real-time access to high-quality data, promotes data integration, and is structured to meet the specific needs of tactical decision-makers across various business areas.
5 Types of operational data store you should know! #
Operational data stores come in various types, each designed to serve different purposes and business needs. The choice of ODS type depends on the specific requirements of an organization.
Here are some of the main types of operational data stores (ODS):
- Near-real-time ODS
- Batch ODS
- Analytical ODS
- Data mart
- Data consolidation ODS
Let us understand each of them in detail.
1. Near-real-time ODS #
- Near-real-time ODS is similar to real-time ODS but typically involves slightly delayed updates, which can range from a few seconds to a few minutes. This type of ODS is suitable when instant updates are not critical but data still needs to be very current for tactical decision-making.
- Use cases
- Inventory management systems that can tolerate a short delay in inventory updates.
- Manufacturing systems that need to monitor production and equipment performance with a slight delay.
- Customer relationship management (CRM) systems that track customer interactions in near real-time.
2. Batch ODS #
- Batch ODS, as the name suggests, updates data in batches or at scheduled intervals, rather than in real-time. Data updates may occur daily, hourly, or at other predefined intervals. Batch ODS is a cost-effective solution for organizations with less stringent real-time data requirements.
- Use cases
- Daily financial reports for accounting and auditing purposes.
- Daily inventory reconciliations for retail businesses.
- Regularly updated marketing campaign performance reports.
3. Analytical ODS #
- Analytical ODS focuses on storing and providing data for analytical and reporting purposes. It is designed to support data analysis, data mining, and reporting requirements. Analytical ODS often contains historical data and may include aggregated data for complex reporting and analysis.
- Use cases
- Business intelligence (BI) systems that generate reports, dashboards, and data visualizations.
- Data warehouses that store historical data for trend analysis.
- Customer segmentation and market analysis for marketing departments.
4. Data mart #
- A data mart is a subset of an ODS that is specifically tailored to a particular business function or department. Data marts may contain a subset of the data from the ODS, organized to support the unique needs of a specific group, such as marketing, sales, or finance.
- Use cases
- Sales data mart for the sales team to track sales performance.
- Marketing data mart for marketing analysts to study campaign effectiveness.
- Finance data mart for financial analysts to perform financial analysis and budgeting.
5. Data consolidation ODS #
- Data consolidation ODS focuses on integrating data from multiple sources into a single, unified repository. It aims to eliminate data silos and provide a centralized view of data from various systems.
- Use cases
- Merging customer data from various touchpoints (e.g., website, mobile app, call center) to create a single customer profile.
- Integrating data from different operational systems in a merger or acquisition scenario.
The choice of ODS type depends on factors such as the organization’s data requirements, the importance of real-time data, budget constraints, and the specific business processes it aims to support. Some organizations may even combine multiple ODS types to meet different needs across the enterprise.
How can your business make use of an operational data store? #
Your business can make effective use of an operational data store in several ways to enhance its operations, decision-making processes, and overall efficiency:
- Real-time monitering
- Customer insights
- Enhanced reporting
- Support for business intelligence
- Inventory and supply chain management
- Enhanced customer service
Let’s understand them shortly.
-
Real-time monitoring: An operational data store can provide your business with real-time data on various aspects of your operations. For example, in retail, it can track inventory levels, sales, and customer transactions as they happen. This real-time insight can help you make quick and informed decisions.
-
Customer insights: An operational data store can store customer data and interactions across various touchpoints. This enables you to gain a holistic view of your customers, personalize marketing efforts, and improve customer service based on their preferences and behavior.
-
Enhanced reporting: Operational data store can serve as a reliable source for generating reports and dashboards. Your business can use it to create customized reports that provide insights into sales performance, financial metrics, or operational KPIs.
-
Support for business intelligence: An operational data store can be used as a data source for Business Intelligence (BI) tools and analytics platforms. This empowers your business analysts to explore data, discover trends, and gain actionable insights.
-
Inventory and supply chain management: In industries like retail and manufacturing, an operational data store can help optimize inventory levels, track supply chain activities, and improve demand forecasting by providing real-time data on product availability and sales.
-
Enhanced customer experience: By having a 360-degree view of your customers, you can personalize interactions, anticipate their needs, and provide better customer service, which can lead to increased loyalty and repeat business.
An Operational Data Store can serve as a valuable tool for your business to centralize, integrate, and utilize operational data effectively. By leveraging real-time and accurate data, your business can gain a competitive edge, respond to market changes swiftly, and improve overall performance.
Operational data store vs. data warehouse vs. data lake: Tabular difference #
Here’s a comparison of Operational Data Stores (ODS), data warehouses, and data lakes in tabular form, highlighting their key differences:
Aspect | Operational Data Store (ODS) | Data warehouse | Data lake |
---|---|---|---|
Data type | Detailed and real-time | Historical and summarized | Raw and structured/unstructured |
Purpose | Tactical and operational | Analytical and reporting | Storing and processing big data |
Data freshness | Real-time or near real-time | Periodic batch updates | Variable - can be near real-time |
Data structure | Highly structured | Structured | Flexible (structured/unstructured) |
Data storage | Typically short-term | Long-term archival | Long-term archival |
Query and analysis | Supports real-time queries | Supports complex analytics | Requires extensive processing |
Schema design | Subject-oriented | Star or snowflake schema | Schema-on-read or schema-on-write |
Data quality | Emphasizes data accuracy | Focuses on data consistency | Data quality can vary widely |
Data source | Operational systems | Various operational systems | Various data sources |
Data transformation | Minimal transformation | Significant transformation | Limited to no transformation |
User base | Operational and tactical | Business analysts and report | Data scientists and analysts |
Tools and technologies | Often uses ETL tools | OLAP tools, BI platforms | Big data frameworks (e.g., Hadoop) |
Scalability | Scales as needed for real-time | Scalable for analytics | Scales to handle big data |
Cost | Moderate to high | Moderate to high | Can be cost-effective |
Use cases | Inventory management, real-time monitoring | Historical analysis, reporting | Big data analytics, machine learning |
While these distinctions help clarify the differences between ODS, data warehouses, and data lakes, it’s important to note that in some organizations, these systems may complement each other. The choice of which data architecture to use often depends on an organization’s specific data needs and objectives.
Summarizing it all together #
In the ever-evolving landscape of data management and analytics, the significance of an operational data store (ODS) in 2023 cannot be overstated. Its purpose is to store and consolidate real-time operational data, providing a reliable source for decision-making.
ODS facilitates real-time data access and integration, allowing organizations to make informed decisions based on the latest information from various sources. ODS plays a pivotal role in ensuring data accuracy and consistency by acting as a data cleansing and transformation layer before the data is moved to data warehouses or data marts.
In conclusion, the operational data store remains an indispensable component of the data architecture in 2023. Its role in ensuring real-time data availability, data quality, and operational efficiency positions it as a key enabler for organizations aiming to make data-driven decisions in the present and the future.
As businesses continue to navigate the complexities of the data landscape, understanding and implementing ODS will be a fundamental step towards success in the digital age.
Operational data store: Related reads #
- What is Operational Metadata & How It drive business Success?
- Managing Data Warehouse Challenges: Strategies for Success
- Data Warehousing Guide: Everything You Need to Know in 2023
- What Is Modern Data Stack: History, Components, Platforms, and the Future
- Cloud Data Warehouses: Cornerstone of the Modern Data Stack
- Data Warehouse: Definition, Concept, Components, and Architecture
- Data Mart vs. Data Warehouse: Should You Use Either or Both?
Share this article