Data Profiling Example: 10 Real World Examples
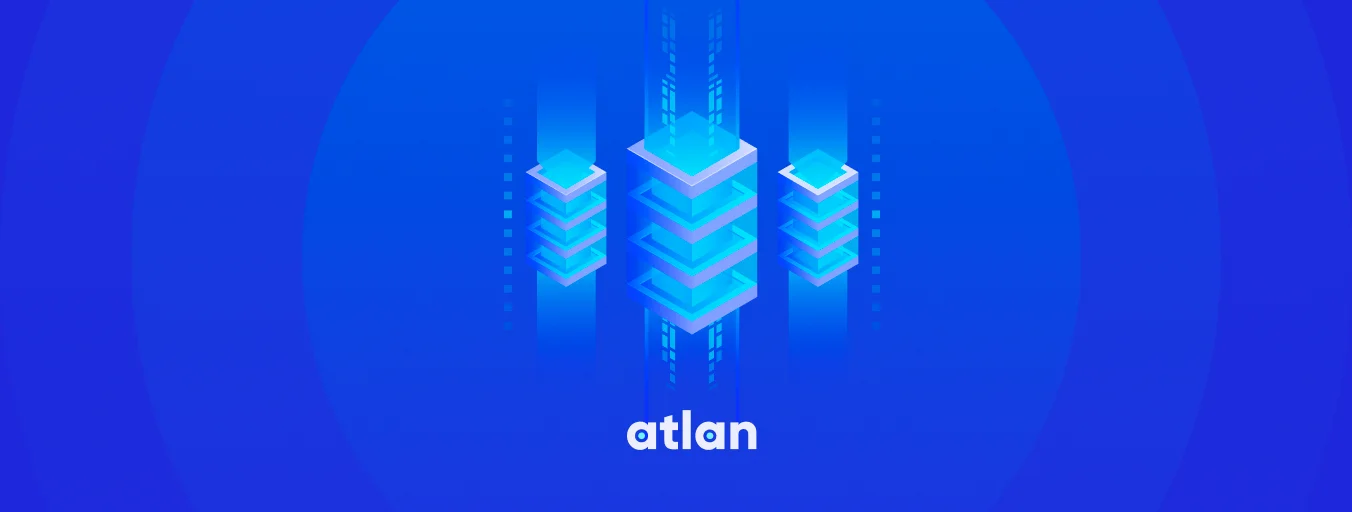
Share this article
Data profiling is the process of analyzing a dataset’s content and structure, holds significant importance in data management. By examining data characteristics, patterns, and quality, it enables organizations to identify anomalies and enhance data quality.
Modern data problems require modern solutions - Try Atlan, the data catalog of choice for forward-looking data teams! 👉 Book your demo today
In the following examples, we’ll illustrate how data profiling is applied in real-world scenarios, highlighting its role in improving data integrity, integration, and informed decision-making for businesses and analysts alike.
Ready? Let’s dive in!
Table of contents #
- 10 Data profiling examples to know
- Retail store: inventory management
- Healthcare: Patient record accuracy
- Banking: Fraud detection
- Real estate: Property valuation
- E-commerce: Customer purchase behavior
- Transportation: Fleet efficiency
- Manufacturing: Quality control
- Education: Student performance analysis
- Energy: Energy consumption optimization
- Agriculture: Crop yield optimization
- To summarize
- Related reads
10 Data profiling examples to know #
In an age where data drives decisions, understanding the quality and nature of this data is paramount. Data profiling allows businesses to take a closer look at their data, ensuring it is both accurate and useful.
For business owners and decision makers, this means better insights, more informed choices, and greater business success. Let’s explore ten examples that showcase the power and significance of data profiling:
- Retail store: Inventory management
- Healthcare: Patient record accuracy
- Banking: Fraud detection
- Real estate: Property valuation
- E-commerce: Customer purchase behavior
- Transportation: Fleet efficiency
- Manufacturing: Quality control
- Education: Student performance analysis
- Energy: Energy consumption optimization
- Agriculture: Crop yield optimization
Let’s understand these examples in details:
1. Retail store: inventory management #
The scenario #
A chain of retail stores wanted to optimize their inventory. They needed to know which products were selling fast, which ones remained on the shelves for too long, and if any discrepancies existed between their digital records and actual stock.
Data profiling in action #
By profiling their sales data alongside their inventory records, the retail store identified discrepancies between what the digital system showed and the actual physical count. Additionally, through analyzing purchase histories, they recognized patterns in product sales.
Outcome #
This allowed the chain to adjust their inventory orders, focusing on products with higher demand and reducing stock that had low turnover. This led to reduced storage costs and increased sales revenue.
2. Healthcare: Patient record accuracy #
The scenario #
A hospital aimed to improve patient care by ensuring that their patient records were accurate and up-to-date. Inaccurate records could lead to misdiagnoses or incorrect treatments.
Data profiling in action #
The hospital began by checking for discrepancies or missing information in their patient database. They looked for mismatched patient IDs, missing medical histories, or inconsistent treatment records.
Outcome #
The profiling revealed several inconsistencies, enabling the hospital to update and correct patient records. This not only ensured safer patient care but also increased trust in the institution’s record-keeping system.
3. Banking: Fraud detection #
The scenario #
A bank wanted to enhance its security measures by identifying unusual transaction patterns that might indicate fraudulent activities.
Data profiling in action #
The bank used data profiling to assess transaction records, seeking out anomalies like unusually high transactions, frequent international transactions, or odd transaction timings.
Outcome #
The bank identified potential red flags and took preventive measures, either by alerting the account holder or freezing suspicious accounts temporarily. This proactive approach protected both the bank and its customers from potential financial loss.
4. Real estate: Property valuation #
The scenario #
A real estate company was looking to provide more accurate property valuations to its clients, both for buying and selling purposes.
Data profiling in action #
The company profiled data from recent property sales, looking at factors like location, size, and amenities. They then compared this data with their current property listings.
Outcome #
The profiling allowed the company to give more accurate property valuations, which instilled trust in their clients and made property transactions smoother and more transparent.
5. E-commerce: Customer purchase behavior #
The scenario #
An e-commerce platform aimed to enhance its marketing strategy by understanding its customers’ buying behavior, including product preferences, buying frequency, and seasonal purchase patterns.
Data profiling in action #
The e-commerce company profiled its user data, focusing on purchase histories, search patterns, and product reviews. This allowed them to segment their customer base and identify distinct buying patterns.
Outcome #
With the insights from data profiling, the e-commerce platform was able to tailor its marketing campaigns more effectively, resulting in higher customer engagement, increased sales, and improved customer loyalty.
6. Transportation: Fleet efficiency #
The scenario #
A transportation company with a large fleet of vehicles wanted to improve its operational efficiency, reduce fuel consumption, and ensure timely deliveries.
Data profiling in action #
The company started profiling data related to vehicle routes, fuel consumption, delivery times, and vehicle maintenance records. This helped in pinpointing inefficient routes, vehicles consuming more fuel, and scheduling inconsistencies.
Outcome #
Based on the data profiling insights, the company optimized its routes, scheduled regular vehicle maintenance, and improved delivery timelines. This led to significant cost savings, increased customer satisfaction, and a more environmentally-friendly operation.
7. Manufacturing: Quality control #
The scenario #
A manufacturing plant producing electronic components wanted to ensure the quality and reliability of its products. They needed to identify defects early in the production process to reduce product recalls and customer complaints.
Data profiling in action #
The company conducted data profiling on various production parameters, such as voltage, current, and temperature during the manufacturing process. They also analyzed data related to product testing, including resistance and performance metrics.
Outcome #
Data profiling enabled the manufacturer to detect patterns associated with faulty components and pinpoint when and where defects were most likely to occur in the production line.
This allowed them to implement targeted improvements in their manufacturing processes, resulting in a significant reduction in product defects and increased customer satisfaction.
8. Education: Student performance analysis #
The scenario #
A school district sought to improve student performance and educational outcomes. They aimed to identify factors that influenced student success and tailor their educational programs accordingly.
Data profiling in action #
The school district profiled student data, including demographics, attendance records, standardized test scores, and extracurricular activities. They also analyzed teacher qualification data and classroom sizes.
Outcome #
Data profiling revealed key insights, such as the positive impact of smaller class sizes on student performance and the correlation between student involvement in extracurricular activities and improved academic results.
Armed with this information, the district could allocate resources more effectively, hire qualified teachers strategically, and design programs that catered to the unique needs of their students.
9. Energy: Energy consumption optimization #
The scenario #
An energy utility company wanted to reduce energy waste and improve efficiency in delivering electricity to its customers. They needed to identify areas where energy consumption was excessive or where power outages were more frequent.
Data profiling in action #
The utility company profiled data related to energy consumption, weather conditions, infrastructure maintenance records, and customer complaints. They also analyzed data from smart meters installed at customer locations.
Outcome #
Through data profiling, the utility company identified regions with higher energy consumption during specific weather conditions, enabling them to proactively reinforce infrastructure and manage energy distribution more efficiently.
Additionally, they could respond to power outage reports more promptly, leading to improved customer satisfaction and a more resilient energy grid.
10. Agriculture: Crop yield optimization #
The scenario #
Agricultural businesses aimed to maximize crop yields while minimizing resource usage, such as water and fertilizers. They sought insights into the factors influencing crop growth and yield.
Data profiling in action #
Farmers profiled data from various sources, including soil quality measurements, weather data, crop planting dates, and irrigation schedules. They also analyzed historical crop yield data.
Outcome #
Data profiling revealed correlations between specific soil conditions, weather patterns, and crop yields. Armed with this knowledge, farmers could optimize planting schedules, adjust irrigation practices, and apply fertilizers more efficiently.
This resulted in higher crop yields, reduced resource consumption, and increased profitability for agricultural businesses.
In each of these real-world examples, data profiling provided clarity, highlighted inconsistencies, and offered a path towards optimization and growth. For business owners and decision-makers, understanding and leveraging data profiling isn’t just beneficial—it’s essential in today’s data-driven landscape.
To summarize #
Data profiling gives insight into data’s structure, content, and quality, simplifying data cleaning and enhancing understanding. Knowledge of data profiling types aids business leaders in making informed decisions.
The data profiling examples highlight the tangible benefits of data profiling. With modern tools and the rise of AI and big data, regular data profiling is essential. For businesses in today’s data-driven era, maintaining a focus on data profiling, supported by real-world examples, is crucial.
We explored the fundamental aspects of data profiling, emphasizing its role in ensuring data integrity, consistency, and accuracy. It caters to both seasoned professionals and newcomers, offering practical insights into real-world scenarios where data profiling plays a pivotal role.
By delving into the detailed examples, from retail inventory management to agricultural crop yield optimization, this guide showcases how data profiling empowers organizations to make better decisions and thrive in today’s competitive landscape.
Data profiling example: Related reads #
- Data Profiling: Definition, Techniques, Process, and Examples
- 11 Essential Benefits of Data Profiling You Can’t Overlook in 2023!
- How To Improve Data Quality In 12 Actionable Steps?
- A Blueprint for Bulletproof Data Quality Management
- Data Quality is Everyone’s Problem, but Who is Responsible?
Share this article