Data Quality is Everyone’s Problem, but Who is Responsible?
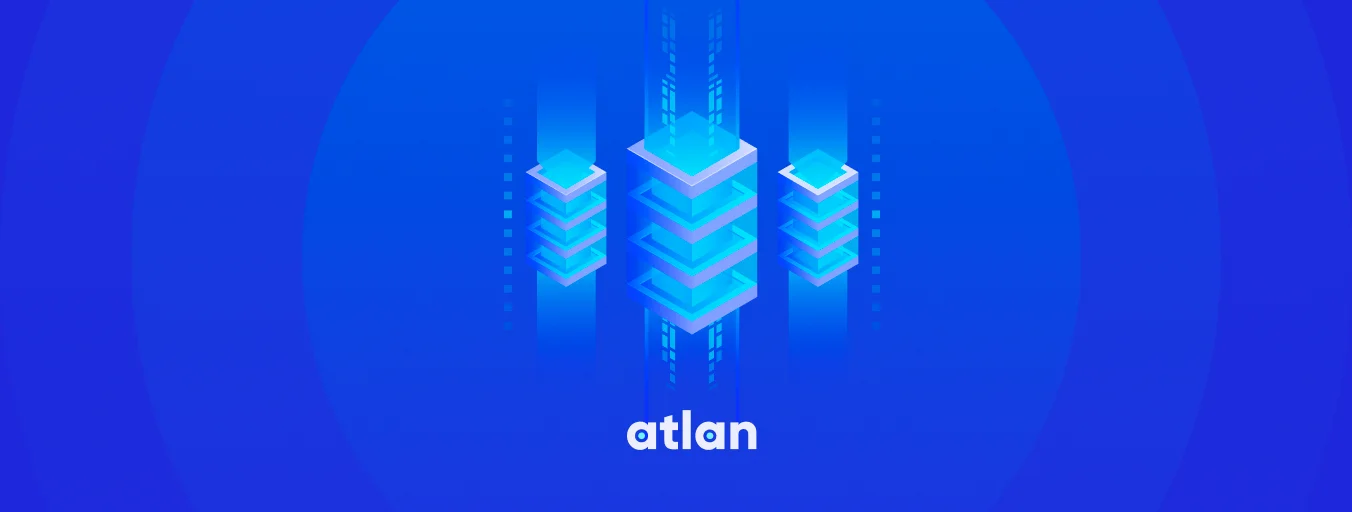
Share this article
Gartner defines data quality as the set of processes and technologies for identifying, understanding, preventing, escalating, and correcting issues in data that supports effective decision-making and governance across all business processes.
While it’s widely accepted that maintaining high data quality is crucial for the success of any organization, the question of responsibility remains a contentious issue. Is it the sole prerogative of the IT department, or does everyone in the organization have a role to play?
Modern data problems require modern solutions - Try Atlan, the data catalog of choice for forward-looking data teams! 👉 Book your demo today
In this article, we will understand:
- Why is data quality important?
- Who is responsible for data quality?
Ready? Let us delve in!
Table of contents #
- Why is data quality so important for you in 2023?
- Who is responsible for data quality? 10 Key roles!
- Role of a chief data officer (CDO) or data quality manager
- Role of data governance team/data stewards
- Role of data architects
- Role of data engineers
- Role of data analysts
- Role of data scientists
- Role of business analysts
- Role of database administrators (DBAs)
- Role of quality assurance (QA) testers
- Role of end-users/business units
- Summary
- Who is responsible for data quality : Related reads
Why is data quality so important for you in 2023? #
Data quality refers to the condition of a set of data values, measured in terms of factors such as accuracy, completeness, consistency, reliability, and timeliness. High-quality data is essential for generating reliable analytics, making informed decisions, and achieving strategic objectives.
Here are the reasons why data quality is such an integral part of any organization:
- Decision-making
- Customer satisfaction
- Compliance and risk management
- Operational efficiency
- Trust and reputation
- Financial implications
- Competitive advantage
- Data-driven culture
- Innovation
- Integration and scalability
Let us understand each of them in detail
1. Decision-making #
Poor quality data can lead to faulty decisions that could jeopardize the business. High-quality data is critical for accurate analytics and reporting, which in turn influence decision-making processes at all organizational levels.
2. Customer satisfaction #
Accurate data is crucial for customer relationship management. Inaccurate customer information can lead to various issues, like failure to deliver services or goods, billing errors, and poor customer service.
3. Compliance and risk management #
Many industries have stringent data regulations. Failure to maintain high-quality data could lead to non-compliance, legal challenges, and financial penalties.
4. Operational efficiency #
Poor data quality can result in inefficient workflows. For example, if an inventory system has incorrect data, it could lead to stock-outs or overstock situations, affecting sales and warehouse costs.
Here is a guide to solving data quality issues → Data Quality Issues? Here Are 6 Ways to Fix Them!
5. Trust and reputation #
Low-quality data can erode trust both internally and externally. Employees lose faith in data-driven decision-making process, and the reputation of the business can be damaged in the eyes of customers, partners, and other stakeholders.
6. Financial implications #
Poor data quality could have direct financial costs. According to Gartner, the average financial impact of poor data quality on organizations is $9.7 million per year.
7. Competitive advantage #
High-quality data can be a significant differentiator in today’s competitive markets. It enables organizations to quickly seize opportunities and detect challenges well in advance.
8. Data-driven culture #
High-quality data is fundamental for fostering a data-driven culture. When people trust the data, they are more likely to rely on it to make informed decisions.
9. Innovation #
Reliable data is the foundation for any kind of R&D or innovation. Inaccurate data can send research projects or new product development down futile paths, wasting resources and time.
10. Integration and scalability #
As businesses grow or merge, the complexity of their data architectures often grows exponentially. High-quality data makes it easier to integrate new systems and scale existing ones.
Maintaining data quality is not just a technical requirement but a business imperative. It requires a holistic approach that combines technology, processes, and a data-aware culture.
Who is responsible for data quality? 10 Key roles! #
The responsibility for data quality is shared across an organization, involving everyone from top executives to front-line employees. While IT departments often manage the technical aspects, data governance should be a cross-departmental effort. Everyone who handles data at any stage has a role to play in ensuring its quality, making it a collective responsibility.
However, there are common roles within a data team that are particularly focused on ensuring data quality.
- Chief data officer (CDO) or Data quality manager
- Data governance team/Data stewards
- Data architects
- Data engineers
- Data analysts
- Data scientists
- Business analysts
- Database administrators (DBAs)
- Quality assurance (QA) testers
- End-users/Business units
Let us understand each of the roles and their responsibilities in data quality in much more detail:
Role of a chief data officer (CDO) or data quality manager #
The role of a chief data officer (CDO) or data quality manager in the context of data quality is multi-faceted and pivotal to an organization’s success in the modern, data-driven world. Here’s a detailed breakdown:
Vision and strategy #
- Set clear goals: One of the first tasks in the realm of data quality is to define what ‘good quality data’ means for the organization, which will vary depending on the business type and needs.
- Long-term strategy: They need to think beyond immediate needs and develop a sustainable strategy for maintaining data quality in the long term, including plans for data growth, technological changes, and business scaling.
Quality metrics and KPIs #
- Definition and monitoring: The CDO is often responsible for defining the key metrics and KPIs that will be used to measure data quality.
- Regular audits: They usually oversee regular data quality audits to identify issues and areas for improvement.
Resource management #
- Human capital: They decide the roles required to maintain data quality and are involved in the hiring and training processes.
- Technological resources: Decisions about the allocation of servers, databases, and other technical resources that directly affect data quality are also made or approved by them.
Culture #
- Promoting a data-driven culture: For data quality measures to be effective, they need to be ingrained in the organization’s culture. The CDO plays a key role in advocating for a data-focused approach across departments.
- Continuous improvement: They ensure that data quality is not a one-off project but an ongoing initiative, promoting a culture of continuous improvement and refinement.
Through these roles and responsibilities, the CDO or Data Quality Manager becomes the linchpin for ensuring that data is reliable, accurate, and usable, directly contributing to the business’ success.
Role of data governance team/data stewards #
Data governance teams and data stewards are crucial linchpins in the machinery of data management, especially when it comes to maintaining data quality. They straddle the line between business processes, technology, and organizational strategy to ensure that data is a reliable asset rather than a liability. Here’s a detailed breakdown of their responsibilities and role in maintaining data quality.
Quality assurance #
Data stewards are the gatekeepers of data quality. They establish key performance indicators (KPIs) for data quality and continuously monitor them.
Quality audits #
They perform regular audits of the data, often using automated tools, to identify anomalies, inconsistencies, and errors. The findings from these audits form the basis of corrective actions.
Data correction #
When low-quality data is identified, they are responsible for tracing back to the source of the error and facilitating corrective actions to amend the data.
Feedback loop with business units #
Act as the first point of contact for business units in the event of data quality issues. They help to translate business needs into data quality criteria.
Resolution coordination #
When quality issues arise, they often coordinate with various departments like IT, business units, and external vendors to ensure a speedy and effective resolution.
Change management #
As organizations evolve, so do their data needs. Data stewards help manage these changes, ensuring that the governance policies and data quality are not compromised.
Compliance #
Ensure that data management and quality assurance practices are in compliance with regulatory standards like GDPR, HIPAA, etc.
Advocacy and culture-building #
One of the often overlooked roles is advocating for data quality within the organization. By showing the benefits of high-quality data and the risks of poor-quality data, they help in building a culture that respects data as a valuable asset.
By implementing and overseeing an array of tasks and protocols, data stewards play a vital role in assuring data quality. Their work ensures that data is reliable, secure, and usable, making them indispensable in modern organizations that increasingly rely on data for decision-making, operations, and competitive advantage.
Role of data architects #
Let us delve deeper into the responsibilities and the role of data architects in ensuring data quality.
Robustness #
A well-designed data architecture should be fault-tolerant and minimize data corruption, loss, or duplication. Implementing redundant systems, backups, and checks can contribute to more robust data.
Scalability #
As the organization grows, so does the data. The architecture must be designed to scale easily. Poor scalability can lead to bottlenecks that compromise data quality as the system becomes overloaded.
Maintainability #
The architecture must be designed so that it is easy to update and maintain. Poor maintainability can lead to deterioration in data quality over time, as it becomes increasingly difficult to update or correct data.
Data governance #
The architecture should enable easy implementation of data governance practices, such as data quality audits, data lineage tracking, and role-based access controls, all of which are crucial for maintaining high data quality.
Data consistency #
The design should enforce data integrity and consistency. This includes the use of primary and foreign keys, data validation rules, and transaction controls to ensure that the data is reliable and accurate.
Metadata management #
Proper architecture should also provide the ability to manage metadata effectively. This allows for better understanding and control over data quality by capturing information about data lineage, transformations, and quality metrics.
Real-time data quality checks #
Some advanced architectures enable real-time or near-real-time data quality checks, allowing for immediate identification and rectification of any issues.
Auditability #
Having a well-structured data architecture allows easier audits of the data for quality checks, which is especially important for organizations that must adhere to regulatory requirements.
Flexibility #
Data quality needs can change over time. A flexible architecture allows for adjustments to be made to data quality rules, validations, and structures without requiring an overhaul of the system.
In summary, data architects play a critical role in designing the foundation upon which high data quality can be achieved and maintained. Their choices influence how easily data can be stored, accessed, managed, and quality-checked, thereby having long-term implications for the organization’s data quality.
Role of data engineers #
Data engineers play a critical role in ensuring data quality, especially in the stages of data ingestion, processing, and transformation. Below, we break down these responsibilities and roles in more detail:
Validation checks #
Implement checks and balances during the data ingestion and processing phases. This could be as simple as field validation (e.g., no alphabetic characters in a phone number field) or as complex as dependency checks between databases.
Anomaly detection #
Use statistical methods to identify outliers or anomalies in the data. This is crucial for preemptively identifying errors or fraud.
Data profiling #
Conduct data profiling to understand the metadata and data distribution. This helps in identifying if certain columns have a large number of null values, or if there are sudden changes in data distribution.
Data standardization #
Apply a consistent set of formats for data, so that it’s easier to validate and use. For example, date fields should be in a consistent format across all data sets.
Data cleansing #
Implement methods for cleaning data, such as imputation for missing values, normalization for numerical fields, or text-cleaning procedures for text data.
Auditing and monitoring #
Implement audit trails for the data and set up automated monitoring for key metrics. If a data quality issue arises, it should trigger alerts.
Documentation #
Document the data pipelines, transformations, and quality checks for future reference and for sharing with other team members.
Collaboration #
Work closely with data analysts, data scientists, and business stakeholders to understand their data quality needs and adapt data pipelines accordingly.
By taking responsibility for these aspects of data quality, data engineers serve as the gatekeepers and facilitators of high-quality data in an organization.
Role of data analysts #
Data analysts play a critical role in maintaining and improving data quality due to their close interaction with data sets for various organizational purposes. Let’s delve into the details of their responsibilities and their role in data quality.
Early detection of issues #
As they sift through data sets, analysts are usually the first to identify inconsistencies, errors, or missing values that could affect data quality and, ultimately, the validity of their analyses.
Feedback loop #
Data analysts often work closely with data engineers and data architects, providing them with feedback on what is required for a data set to be usable. This iterative process is crucial for ongoing data quality improvement.
Metadata management #
Analysts often document their observations about data quality issues so that this meta-information can be used for improving data collection methods or data transformation algorithms.
Validation #
Before presenting their findings, data analysts need to validate the data they’re using to ensure its accuracy. This often includes cross-referencing with other data sources and re-checking statistical assumptions.
Quality checks #
Analysts often have to build quality checks into their data retrieval and processing scripts. These checks might include identifying and flagging potential outliers, missing values, or inconsistencies for further investigation.
Educating business users #
Data analysts may also be involved in educating other employees in best practices for data collection, entry, and usage to minimize quality issues at the source.
Advocacy for resources #
Because they understand the business impact of poor data quality, data analysts are often key advocates for resources and tools to improve data quality.
Identifying gaps #
Analysts can point out where additional data could provide more value or where current data is not sufficiently meeting the organizational needs, indirectly highlighting areas where data quality can be improved.
By taking on these responsibilities and activities, data analysts serve as both a filter and a conduit, ensuring that only high-quality data is utilized in decision-making processes and that data quality issues are addressed promptly. Their role is not just reactive—identifying problems—but also proactive, helping to define what “quality” means in the context of the specific analyses and decisions that the organization needs to make.
Role of data scientists #
Let us delve deeper into the role of Data Scientists when it comes to data quality:
Data verification #
Data scientists are often among the first to notice inconsistencies or irregularities in the data due to their deep involvement with it. They must be vigilant in verifying the data they use for modeling, which often involves summarizing data distributions, checking for outliers, missing values, and so on.
Data preprocessing #
Before running any advanced algorithms, data scientists have to preprocess the data. This step involves cleaning and transforming the data, handling missing values, and dealing with outliers, all of which are crucial for data quality.
Feature validation #
Poor data quality can severely impact the effectiveness of the features used in the models. Data scientists have to ensure that the features they engineer or select adhere to high-quality standards.
Model sensitivity #
High-quality data is essential for models that are robust and generalize well to new, unseen data. Poor-quality data can lead to models that are overly sensitive to noise rather than capturing underlying patterns.
Anomaly detection #
Data scientists sometimes build models specifically to identify anomalies in data, which can be instrumental in improving data quality by flagging errors or inconsistencies for further investigation.
Feedback to data governance teams #
Data scientists often provide valuable feedback to data governance teams or data stewards about potential issues with data quality. This information can be crucial for ongoing data quality initiatives.
Collaboration #
They often collaborate with data engineers, data architects, and business analysts to ensure that data pipelines are designed to maintain high levels of data quality.
Role of business analysts #
Business Analysts play a vital role in ensuring data quality, particularly because they serve as the bridge between business needs and technical solutions. Below is a detailed look at how Business Analysts contribute to maintaining high-quality data.
Defining quality attributes #
Business Analysts have the responsibility of defining what “quality” means in the context of the data being used. They set the metrics or key performance indicators (KPIs) that the data should meet. This could include attributes like accuracy, completeness, reliability, and timeliness.
Mapping business rules to data quality #
A core business rule might require that customer data be 100% accurate for billing purposes. Business Analysts are responsible for translating such rules into technical specifications for the data team. They may specify validation rules, permissible value ranges, or other criteria that data must meet to be considered “high-quality.”
Identifying sources of error #
Business Analysts review the data pipeline to identify where data quality could be compromised. This could be at the point of data capture, during transformation, or at any other stage in the data lifecycle. They then propose solutions to rectify these issues.
Prioritizing data quality initiatives #
Not all data is of equal importance to a business. Business Analysts help in prioritizing which data quality issues need immediate attention, based on how crucial they are to achieving business objectives.
Role of database administrators (DBAs) #
Database Administrators (DBAs) play an integral role in maintaining data quality, although their responsibilities are often more technical in nature compared to other roles focused on analytics or governance. Here is a detailed breakdown:
Data integrity constraints #
DBAs can set up rules within the database that automatically enforce certain types of data quality, such as unique keys or required fields.
Auditing #
They can enable and configure database features that track who made what changes when, which can be invaluable for tracing the source of data quality issues.
Data cleansing #
Though not traditionally their core responsibility, DBAs can help facilitate the process of data cleansing by creating and maintaining utilities that automatically clean and enrich data.
Collaboration #
DBAs work closely with data analysts, data scientists, and data stewards to understand what “quality” means in the context of their specific roles and to ensure that the database supports these quality requirements.
Error detection and reporting #
By monitoring database logs and setting up alerts, DBAs can quickly identify and respond to incidents that could jeopardize data quality, such as failed data imports or unauthorized data modifications.
Policy implementation #
They are responsible for implementing company policies around data at a technical level, making sure that guidelines around data quality are followed.
By carrying out these responsibilities effectively, DBAs play an invaluable role in maintaining and improving the quality of data within an organization. Their technical expertise ensures that the data is not just secure and efficient but also reliable and accurate, serving as the backbone for organizational data quality.
Role of quality assurance (QA) testers #
In the context of data quality, Quality Assurance (QA) Testers play an indispensable role in ensuring that the data pipelines and operations meet the predefined standards. This role is vital for maintaining the integrity, accuracy, and reliability of data as it moves through the system. Below are the detailed responsibilities and roles of QA Testers in data quality:
Validation and verification #
QA Testers validate that the data is correct, complete, and formatted properly, verifying that the data pipeline’s output meets all defined quality standards.
Identifying issues #
By conducting systematic tests, they identify issues related to data inconsistencies, duplication, incorrect entries, and security vulnerabilities, thereby helping in the timely rectification of such issues.
Ensuring consistency #
Data should be consistent across all databases and pipelines; QA Testers ensure this by cross-referencing and checking multiple data sources.
Performance metrics #
By measuring key performance indicators, they can quantitatively gauge data quality, thereby providing actionable insights for improvements.
Compliance #
In regulated industries, QA Testers ensure that the data processing and storage comply with legal and industry standards, thus mitigating risks related to non-compliance.
Improving data reliability #
Continuous quality checks make sure that the data is reliable and can be trusted for making business decisions.
Quality gatekeeping #
Before any data component is moved to production, QA Testers act as the final gatekeepers to ensure that the component will not compromise the overall data quality.
Role of end-users/business units #
The role of end-users and business units in maintaining data quality is often underestimated, but it’s actually quite crucial. Here’s a detailed explanation:
Data accuracy #
Since end-users often enter data into the system, the accuracy of this data is critical. A misspelled customer name, a wrong address, or an incorrectly entered transaction amount can have far-reaching implications.
Data consistency #
End-users can help maintain data consistency by adhering to standardized processes and protocols for data entry and manipulation. This is essential for ensuring that data is reliable across different parts of the organization.
Identifying inconsistencies #
When working with data regularly, end-users are usually the first to spot inconsistencies, errors, or omissions. Whether it’s a missing value in a report, a discrepancy in financial numbers, or incorrect customer information, they provide the essential ‘human filter’ to catch errors.
Feedback loop #
An important aspect is the feedback mechanism. End-users should have an easy way to report any data issues they encounter. This creates a loop where data quality issues can be quickly identified and rectified, often before they have a chance to escalate into bigger problems.
User training and education #
Being the first line of interaction with the data, end-users should be adequately trained to recognize the importance of data quality. This includes understanding the implications of data errors and inconsistencies, not just for their role but for the organization as a whole.
Validation and verification #
Some organizations implement checks that require end-users to validate or verify data. For instance, confirming the accuracy of a customer’s information before finalizing a transaction can be a crucial step in maintaining data quality.
In summary, end-users play a multi-faceted role in maintaining data quality. They are not just consumers of data but also serve as gatekeepers and quality checkers who can greatly contribute to an organization’s overall data integrity.
Summary #
It’s critical for these roles to coordinate with each other and work as a cohesive unit. Regular meetings, audits, and feedback loops can help everyone stay aligned with the organization’s data quality objectives.
Understanding this hierarchical yet interconnected structure can help businesses ensure that data quality is not just a checkbox but an ingrained organizational ethos.
Who is responsible for data quality : Related reads #
- Data Quality Explained: Causes, Detection, and Fixes
- Data Quality Measures: A Step-by-Step Implementation Guide
- How to Improve Data Quality: Strategies and Techniques to Make Your Organization’s Data Pipeline Effective
- How to Ensure Data Quality in Healthcare Data: Best Practices and Key Considerations
- Data Quality in Data Governance
- 6 Popular Open Source Data Quality Tools in 2023: Overview, Features & Resources
- Automated quality control of data pipelines
Share this article