What is Self-Service BI and What Are Its Benefits?
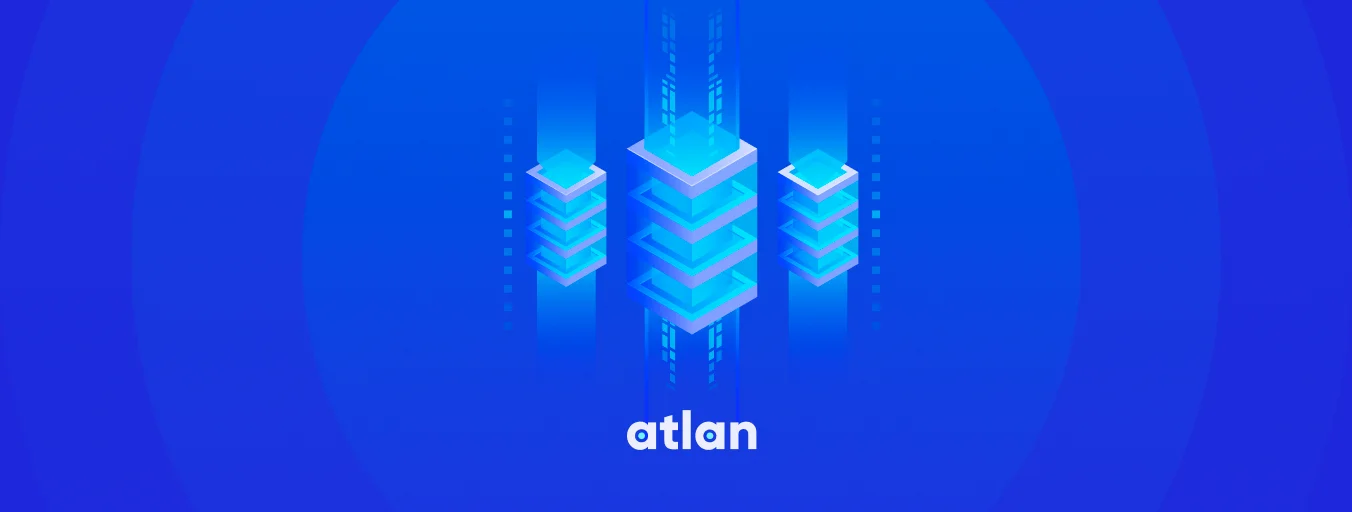
Share this article
Facing delays and inefficiencies due to a bottleneck in data analysis is a pressing challenge for many organizations. Isn’t it? That’s where self-service BI comes in!
Traditionally, businesses have relied heavily on IT departments or data scientists to generate reports and dashboards, resulting in delays and a queue of unmet data requests.
Self-service BI eliminates the bottleneck of relying on data specialists by empowering end-users to generate their own insights.
In this article, we will dive deeper into the various benefits of self-service BI, how it’s different from traditional BI, steps to implement it, and more!
Modern data problems require modern solutions - Try Atlan, the data catalog of choice for forward-looking data teams! 👉 Book your demo today
Table of contents
- What is self-service BI & what are the key roles involved in it?
- 10 Key benefits of self-service BI
- 12 Critical challenges in self-service BI
- Examples of self-service BI tools
- How to implement a self-service BI strategy: 13 Steps!
- Traditional BI vs self-service BI: How are they different?
- Summary
- Related reads
What is self-service BI & what are the key roles involved in it?
Self-service BI (Business Intelligence) is the practice where end users design and deploy their own reports and analyses within an approved and supported architecture and tools portfolio.
Instead of relying on IT or data teams to create reports, users can use self-service BI tools to explore, visualize, and share insights from data.
Key roles in self-service BI
The key roles involved in self-service BI are typically:
- Business users: These are the end-users who utilize self-service BI tools to analyze data and make informed decisions. They do not necessarily have technical expertise in data analytics but use intuitive interfaces to gather insights. They might be managers, team leaders, or individual contributors in various departments.
- Data analysts: While self-service BI is designed to be user-friendly, data analysts play a crucial role in setting up and maintaining the more complex aspects of the BI tools. They help in data modeling, creating complex reports, and ensuring that the data is accurate and up-to-date.
- IT professionals: IT staff are responsible for the technical setup, maintenance, and security of the BI tools. They ensure that the data sources are properly integrated and that the system is running smoothly and securely.
- Data stewards or governance teams: These individuals or teams are responsible for managing the data quality, compliance, and usage policies. They ensure that the data used in self-service BI adheres to company policies and regulatory standards.
- BI developers or administrators: They are responsible for the technical development and administration of the BI platform. This includes creating data models, integrating data sources, setting up user permissions, and customizing the platform to meet the specific needs of the organization.
- Executive sponsors: Senior executives or managers who champion the adoption of self-service BI in the organization. They help in securing budget, aligning BI initiatives with business goals, and promoting a culture of data-driven decision-making.
Each role contributes to a holistic approach in enabling an organization to leverage its data effectively for business insights and decision-making. Self-service BI democratizes data analysis, allowing non-technical users to generate reports and insights without relying heavily on IT or data science departments.
10 Key benefits of self-service BI
Self-service BI has gained considerable traction over the past years due to its capability to democratize data access and empower individuals to derive insights on their own.
Here are some notable benefits of self-service BI:
- Empowerment of end users
- Reduction in reporting backlog
- Cost savings
- Enhanced decision making
- Real-time insights
- Data literacy
- Fostered innovation
- Robust data visualization
- Collaborative environment
- Scalability
Let us understand each of them in detail:
1. Empowerment of end users
By allowing end users to directly access and analyze data, self-service BI reduces dependency on IT or data specialists, enabling quicker and more agile decision-making.
This democratization of data fosters a culture where insights are shared freely, leading to a collaborative and informed business environment.
2. Reduction in reporting backlog
IT departments often face a backlog of reporting requests. Self-service BI alleviates this issue as users can generate their own reports.
This shift not only streamlines operations but also frees up IT resources for more complex, value-added tasks.
3. Cost savings
By reducing the need for extensive IT involvement in routine reporting, organizations can realize significant cost savings. Additionally, this decentralization can lead to faster decision-making, eliminating potential revenue losses from delayed actions.
4. Enhanced decision making
With more hands-on access to data, decision-makers can dive deeper into insights, leading to more informed and strategic choices. This direct engagement with data fosters proactive problem-solving and unlocks potential growth avenues.
5. Real-time insights
Self-service BI tools often allow real-time data exploration, helping businesses react quickly to evolving situations.
This immediacy in data access equips companies to anticipate market trends, adapt to customer behaviors, and make proactive decisions, ensuring they always stay one step ahead of the competition.
6. Data literacy
Encouraging users to interact with data directly fosters a culture of data literacy, elevating the overall analytical capability of an organization.
As more individuals become comfortable with data, collaboration and innovation are amplified.
7. Fostered innovation
When users from diverse backgrounds and departments have the ability to explore data, it can lead to unique insights and innovative solutions.
This cross-pollination of ideas and perspectives often sparks groundbreaking strategies and approaches.
8. Robust data visualization
Many self-service BI tools prioritize data visualization, making it easier for users to comprehend complex data patterns through graphs, charts, and dashboards.
This focus on visualization aids in the faster assimilation of insights, allowing teams to spot trends and anomalies swiftly.
9. Collaborative environment
These platforms often support collaboration, allowing users to share insights, reports, and dashboards, promoting a collective data-driven approach.
This shared ecosystem fosters open communication and knowledge exchange, breaking down departmental silos and elevating the entire team’s analytical capabilities.
10. Scalability
Modern self-service BI solutions are built to scale, accommodating the growth of data and users without a decline in performance. This adaptability ensures that as your business evolves, your BI tools can seamlessly handle increased demands.
Furthermore, a scalable solution means you’re future-proofed against data surges, ensuring consistent and efficient data analysis at every stage of your company’s growth.
However, while the benefits are many, it’s essential for organizations to ensure proper data governance, security protocols, and training when rolling out self-service BI tools to avoid potential pitfalls such as data misinterpretation or security breaches.
12 Critical challenges in self service BI
Self-service BI offers numerous advantages, from user empowerment to agility in decision-making.
However, it also brings a set of challenges that organizations must acknowledge and address to ensure its successful implementation.
Here are some of the primary challenges associated with self-service BI:
- Data quality and accuracy
- Data security and privacy concerns
- Potential for data silos
- Misinterpretation of data
- Lack of standardization
- Over-reliance on tools
- Complexity for some users
- Governance challenges
- Scalability concerns
- Training and support
- Integration with other systems
- Licensing costs
Lets explore these challenges in detail.
1. Data quality and accuracy
As more users have the ability to access and manipulate data, there’s a risk of data inconsistencies arising, especially if different departments or teams define or clean data differently.
Establishing clear data protocols and guidelines is paramount to ensure uniformity and trustworthiness of the data being analyzed.
2. Data security and privacy concerns
Without strict governance, there’s a potential risk of sensitive or confidential data being exposed or accessed by unauthorized personnel.
Implementing layered security measures and regular audits is imperative to ensure that data remains shielded from both internal and external threats.
3. Potential for data silos
When departments or teams use BI tools independently, they might create isolated data sets or reports, leading to fragmented insights.
A unified strategy and proper communication channels become imperative to ensure that insights are seamlessly integrated across the organization.
4. Misinterpretation of data
Without proper training or context, end-users might draw incorrect conclusions from the data, leading to misguided decisions.
Ensuring clear documentation and guidance within the BI tool can further prevent misinterpretations and promote more accurate data analysis.
5. Lack of standardization
Multiple users creating reports can result in a myriad of formats, metrics, and designs, leading to a lack of uniformity and potential confusion.
Additionally, without standardized reporting protocols, valuable time might be spent reconciling or clarifying disparate data presentations rather than acting on insights.
6. Over-reliance on tools
A tool is only as good as its user. There’s a danger that users might overly trust automated insights without applying critical thinking.
Relying solely on tool-generated insights could miss nuanced data interpretations that only experienced professionals can identify.
7. Complexity for some users
While many self-service BI tools are user-friendly, they might still be daunting for some users, leading to underutilization or incorrect usage.
Tailored training sessions and intuitive user guides can help bridge this knowledge gap, ensuring that all team members, regardless of their technical proficiency, can harness the full power of the BI tool.
8. Governance challenges
Striking a balance between user autonomy and centralized control can be difficult. Too much freedom might lead to the aforementioned issues, while too much control might negate the benefits of self-service BI.
Additionally, involving both IT and business stakeholders in the governance process can ensure a holistic approach that respects both data security and user empowerment.
9. Scalability concerns
As the organization grows and more users access the BI tools, there could be performance or integration challenges.
Moreover, as the volume of data expands, ensuring efficient storage and timely data processing becomes paramount to maintain the tool’s effectiveness.
10. Training and support
Providing ongoing training and support for all end-users can be resource-intensive, especially if the tool is complex or frequently updated.
Additionally, a well-informed user base can lead to more accurate insights and foster a culture of true data-driven decision-making across the organization.
11. Integration with other systems
Not all self-service BI tools might seamlessly integrate with existing IT systems or third-party software, leading to potential data integration challenges.
Furthermore, troubleshooting integration issues can be time-consuming, diverting IT resources from other critical tasks.
12. Licensing costs
As more users adopt the platform, licensing or subscription costs can grow, making it a significant expense for some organizations.
Balancing the cost with the tangible benefits becomes crucial to ensure that the investment in self-service BI delivers a worthwhile return.
To mitigate these challenges, organizations need to adopt a proactive approach, including implementing robust data governance, providing consistent training, ensuring data security measures are in place, and regularly reviewing and updating their BI strategies.
Examples of self-service BI tools
Self-service Business Intelligence (BI) tools have become increasingly popular as they enable business users to access, analyze, and visualize data without extensive technical knowledge. Some notable examples of self-service BI tools include:
- Tableau
- Microsoft Power BI
- Google data studio
- Domo
- Sisense
- SAP business objects lumira
- Zoho analytics
- TIBCO spotfire
Let us understand each of them in detail.
1. Tableau
Known for its powerful data visualization capabilities, Tableau allows users to create interactive and shareable dashboards. It’s user-friendly and supports a wide range of data sources.
2. Microsoft Power BI
Integrated with Microsoft’s suite of products, Power BI is a robust tool offering advanced analytics, visualizations, and a strong integration with Excel and other Microsoft products.
3. Google data studio
This free tool from Google integrates seamlessly with other Google products like Google Analytics, Google Ads, and Google Sheets, making it an ideal choice for users already in the Google ecosystem.
4. Domo
Domo is known for its ability to integrate with a vast array of data sources, including databases, spreadsheets, social media, and cloud-based data repositories. It offers real-time data visualization and collaboration tools.
5. Sisense
Sisense is designed to simplify complex data analysis with its powerful drag-and-drop features and ability to handle large volumes of data.
6. SAP business objects lumira
This tool allows users to combine data from multiple sources and visualize it for insightful decision-making. It’s known for its integration with SAP’s suite of business applications.
7. Zoho analytics
A part of the Zoho suite, this tool offers data visualization, dashboards, and an AI-powered assistant for data analysis. It’s suitable for small to medium-sized businesses.
8. TIBCO spotfire
A comprehensive tool offering advanced analytics, Spotfire is known for its high-speed analytics and powerful data visualization capabilities.
Each of these tools has its unique strengths and is suited to different types of business needs and skill levels. The choice of a self-service BI tool often depends on factors like the size of the organization, the types of data sources to be integrated, the complexity of the data analysis required, and the existing IT infrastructure.
How to implement a self service BI strategy: 13 Steps!
Implementing a self-service BI strategy requires careful planning, collaboration, and foresight to ensure that the tools adopted truly serve the business’s objectives while empowering users.
Here are the essential steps for rolling out an effective self-service BI strategy:
- Define clear objectives
- Assess your data landscape
- Choose the right tools
- Establish data governance
- Data preparation
- Training & onboarding
- Pilot implementation
- Feedback loop
- Promote collaboration
- Monitor & adjust
- Review licensing & costs periodically
- Stay updated
- Establish a center of excellence
Let us understand each steps in detail.
1. Define clear objectives
Understand what you aim to achieve with self-service BI. Are you looking to speed up decision-making, reduce IT reporting backlog, foster a data-driven culture, or all the above?
Setting precise goals from the outset will streamline subsequent steps and provide a clear benchmark for measuring success.
Moreover, clear objectives resonate with team members, ensuring alignment and enthusiastic participation throughout the implementation journey.
2. Assess your data landscape
Take stock of your current data sources, data quality, and infrastructure. Identify gaps, redundancies, and areas for consolidation.
Recognize the flow of data across different departments and understand how various teams interact with it. This comprehensive assessment ensures a seamless integration of the self-service BI tools and maximizes data utility across the organization.
3. Choose the right tools
Based on your objectives and the complexity of your data, select a BI tool that aligns with your needs. Ensure it is user-friendly and scalable.
It’s also crucial to consider the tool’s integration capabilities with your existing systems and its adaptability to future technological advancements.
A strong community or vendor support can be invaluable for troubleshooting and best practice sharing, further enhancing the tool’s long-term utility for your organization.
4. Establish data governance
Define guidelines and protocols for data access, security, quality, and usage. Decide who can access what data and the procedures for introducing new data sources.
A robust data governance framework also ensures compliance with regulatory standards, minimizing potential risks. By embedding best practices and clarity in processes, you can foster trust and reliability in the data, making it a valuable asset for all users.
5. Data preparation
Before users can derive insights, data must be clean, reliable, and structured appropriately. This might involve ETL processes (Extract, Transform, Load) or data wrangling.
An efficient data preparation phase ensures that users don’t waste time sifting through irrelevant or erroneous data. Moreover, a unified and well-prepared data source minimizes the risk of drawing incorrect conclusions, thereby reinforcing trust in the data-driven insights obtained.
6. Training & onboarding
Equip your team with the skills they need. This involves not just tool-specific training but also broader data literacy education. A well-informed team can extract greater value from BI tools, driving innovation and informed decision-making.
Continuous learning opportunities, such as workshops and refresher courses, ensure that the team stays updated and maximizes the potential of the BI platform.
7. Pilot implementation
Before a full-scale rollout, implement the tools and processes with a smaller group. Gather feedback, identify challenges, and refine your approach.
This controlled environment can spotlight unforeseen issues, allowing for adjustments in real-time. Additionally, success stories from this pilot phase can act as powerful testimonials to drive broader adoption across the organization.
8. Feedback loop
Even post-implementation, maintain a consistent feedback mechanism. Encourage users to report issues, suggest improvements, and share their success stories.
This continuous dialogue fosters a sense of ownership among users and ensures that the BI platform evolves in tandem with the users’ needs. Moreover, actively responding to feedback reinforces trust and emphasizes the organization’s commitment to a data-driven culture.
9. Promote collaboration
Foster a culture where users share insights, dashboards, and reports. Collaboration can lead to richer insights and avoid duplicative efforts.
A collaborative environment also ensures that knowledge is dispersed throughout the organization, reducing the reliance on a few key individuals.
By promoting open dialogue and shared learning, businesses can harness the collective intelligence of their teams and drive more holistic, data-informed strategies.
10. Monitor & adjust
Use analytics to track how often the BI tool is used, which features are most popular, and where users face difficulties. Adjust your strategy based on these insights.
Periodic evaluations will help ensure that the platform remains relevant and beneficial to users, allowing for iterative improvements that enhance user experience and optimize data-driven decision-making processes.
11. Review licensing & costs periodically
As your organization grows and the BI tool’s usage evolves, periodically review your licensing to ensure it aligns with your needs and budget.
Staying proactive in this review can prevent unexpected expenditures and ensure that you’re leveraging the most cost-effective options for your evolving data needs.
12. Stay updated
The world of BI is always evolving. Stay updated with the latest trends, features, and best practices. Consider attending workshops, webinars, or industry conferences.
Engaging with industry peers and experts can offer fresh perspectives and solutions to evolving challenges.
13. Establish a center of excellence
As the BI adoption grows, consider creating a BI Center of Excellence (CoE) – a dedicated team or group that promotes best practices, offers training, and serves as a knowledge hub.
This CoE can act as the nexus point for BI-related queries, ensuring that users have a go-to resource for guidance.
By following these steps, businesses can ensure that their self-service BI strategy not only empowers users but also aligns with organizational goals, remains cost-effective, and continues to deliver value over time.
Traditional BI vs self-service BI: How are they different?
Traditional BI is the conventional way of gathering, analyzing, and presenting business data using a centralized data warehouse and IT-driven reporting processes. On the other hand, self-service BI is a modern approach that enables business users to access and analyze data without extensive IT involvement.
- Traditional BI pros and cons: Traditional BI is typically managed by IT departments, making it more controlled and secure. It’s great for complex data scenarios but can slow down decision-making due to its reliance on IT for reports.
- Self-Service BI pros and cons: Self-service BI allows non-technical users to analyze and interpret data, leading to quicker insights and fostering a data-driven culture. However, it needs a strong data governance framework to maintain data accuracy and consistency.
- Choosing the right BI: The choice between traditional and self-service BI depends on an organization’s needs. Factors to consider include data complexity, the need for control and security, desired speed of insights, and the goal of fostering a data-driven culture.
To learn more about them, check this out → Traditional BI vs. Self-Serve BI: Differences & Benefits
Summary
Self-service BI has emerged as a game-changer in the realm of data analytics, empowering individuals at all levels of an organization to harness the power of data in real-time.
By decentralizing data access and analysis, it fosters a truly data-driven culture, enabling quicker, more informed decisions. However, as with any powerful tool, its success hinges on thoughtful implementation, consistent training, and vigilant governance.
When executed well, self-service BI can be the catalyst that propels a business forward, breaking down data silos and democratizing insights. In the evolving landscape of business intelligence, self-service BI is not just a trend; it’s the future of data-driven decision-making.
Self-service BI: Related reads
- Data Catalog for Power BI: How to Setup Guide
- Traditional BI vs. Self-Serve BI: Differences & Benefits
- Power BI Data Governance: Importance, Elements, Features & Implementation Approach
- How to Integrate a Data Catalog With BI Tools? Steps, Factors to Consider & Practical Examples
- BI & Metadata Management: The Rhino and the Oxpecker
Share this article