Atlan vs Amundsen: A Comprehensive Comparison of Features, Integration, Ease of Use, Governance, and Cost for Deployment
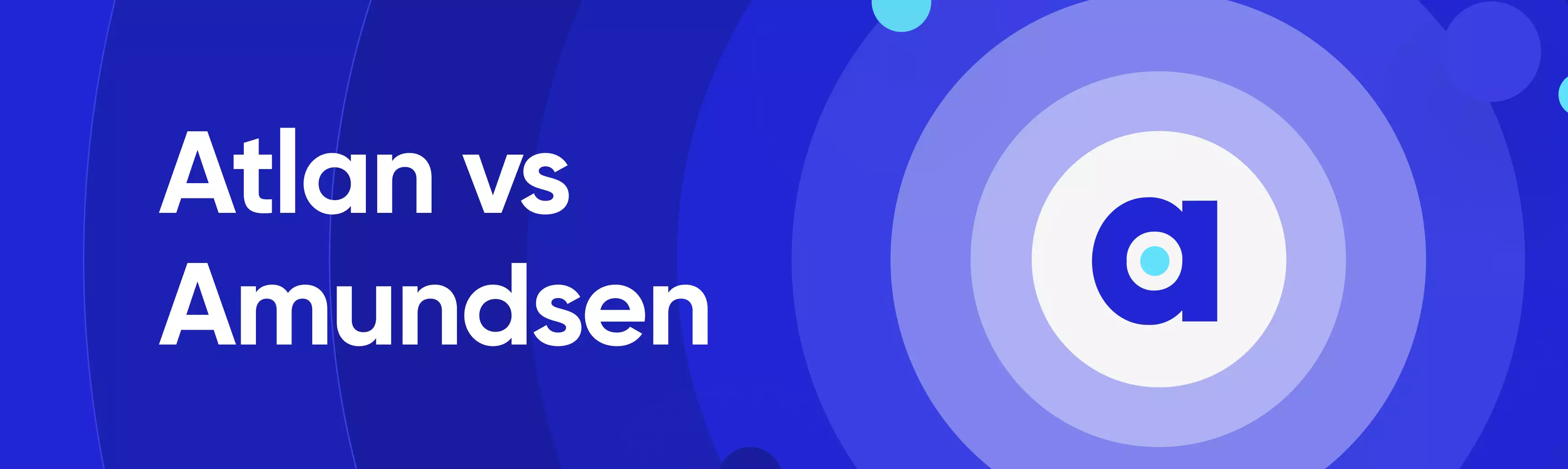
Share this article
Atlan and Amundsen launched at around the same time.
Atlan was initially developed as an internal data tool at SocialCops to assist with large-scale data projects that involved cataloging data assets. SocialCops utilized Atlan in diverse industries, such as energy, healthcare, and education.
Is Open Source really free? Estimate the cost of deploying an open-source data catalog 👉 Download Free Calculator
On the other side of the world, Lyft was growing tremendously, dealing with a lot of data, when they felt the need to create a data discovery and governance tool. Thus, Amundsen came into existence. When Amundsen worked well for them, they open-sourced it for everyone else to use.
This article compares two data catalogs based on their features, technical architecture, cost of deployment, and other factors.
What is Atlan? #
Atlan is an active metadata platform built by a data team for data teams. Atlan brings all your data sources together to create a single repository, a single source of truth for data assets in your business. It then gives drives collaboration between different teams and empowers them to search for and use the data they need.
Atlan’s rich features around search, discovery, and governance have led many companies like Autodesk, Nasdaq, Unilever, Juniper Networks, Postman, Monster, WeWork, and Ralph Lauren to adopt and deploy Atlan at scale.
What is Amundsen? #
Amundsen is an open-source data discovery and metadata engine created by the engineering team at Lyft. Since its release as open-source software, Amundsen has been adopted by the AI & Data arm of the Linux Foundation.
Over 200 engineers have contributed to the project. With its convincing search and discovery functionality, as well as its integration capabilities, companies such as Lyft, Instacart, and Loft have chosen to adopt it.
Amundsen home page. Source: Amundsen.
Table of contents #
- What is Atlan?
- What is Amundsen?
- Atlan vs. Amundsen: Core features
- Things you must consider while evaluating these data catalogs
- Summary
- Atlan vs Amundsen: Related reads
Atlan vs. Amundsen: Core features #
- Data discovery
- Data lineage
- Data governance
- Collaboration
- Active metadata
Data discovery #
As data cataloging and discovery is the only centralized way of searching and sorting through your data assets, it needs to be interactive and intuitive for users across your business. A Google-like simple search experience is the way to go, so both Atlan and Amundsen use an Elasticsearch-based search engine to serve queries.
Atlan’s approach to search and discovery was to replicate the feeling one gets while shopping. You look, browse, read the information about the product, and finally buy it. By filtering, searching, and sorting through data assets, users can find what they’re looking for quite easily. Moreover, Atlan has built an additional layer of metadata enrichment by embedding trust signals like Slack conversations, Jira ticket comments, and so on within your data assets.
Data lineage #
Amundsen supports both table and column-level data lineage. Setting it up is easy but still needs some work, as you have to manually configure and build the Amundsen frontend library after enabling data lineage.
Atlan’s automation-focused data lineage features involve automated SQL parsing, and propagation of tags, classifications, and descriptions. In conjunction with in-line action, Atlan’s column-level lineage creates a powerful combination to track data lineage for looking at data asset failures impact analysis, and so on.
Data governance #
Amundsen’s larger data governance goal includes four core areas: data ownership, data quality, metadata curation and documentation, and data lineage. With these four, Amundsen intends to build trust in your data assets. Amundsen allows you to assign owners and maintainers to all your assets. It also lets you see the most frequent users of a data asset.
Continuing the automation arc, Atlan’s data governance is driven by Playbooks that automatically identify PII, HIPAA, and GDPR data. Atlan also allows you to define masking and hashing policies for critical data. In addition to the usual RBAC governance controls, Atlan takes a three-pronged approach to governance based on personas, purposes, and compliance needs.
The future of data governance with Atlan is exciting. In a recent webinar, Varun Banka, co-founder of Atlan, shared a first look at some new AI-powered capabilities. These served as a teaser for how Atlan plans to use AI and automation to revolutionize data governance workflows and experiences.
Collaboration #
Like other open-source tools, Amundsen is extensible. This means that you can build the features you need. Not all businesses have the luxury or the capability of doing that, but the team at Convoy did it by integrating Slack into Amundsen. With that integration, Slack conversations and relevant questions could be seen within the Amundsen platform with all the data assets. Unfortunately, Amundsen does not come with out-of-the-box integrations with other collaboration tools to reduce friction when looking for and using data assets.
Atlan takes the approach of embedded collaboration with the help of deep integrations with collaboration tools like Slack and Jira. For instance, you can tag Slack users or channels in conversations about a data asset on Atlan. You can also create and manage Jira tickets and see activity on a particular data asset with the help of the Jira integration. Moreover, Altan also integrates with tools like Tableau, Looker, and PowerBI via a Chrome plugin that helps you get all the metadata, including ownership information from the data assets in your BI tool.
Active metadata #
Atlan acive metadata platform. Image by Atlan.
Metadata activation is one of the superpowers of Altan. The drive towards active metadata pushes for always-on, intelligent, and action-oriented metadata. This means Atlan leverages metadata that is always available and in use, metadata that is connected in a way that allows deduction of meaning or intelligence, and finally, metadata that can be used to create and trigger new workflows to save time and money.
How does active metadata manifest in your data flows? Here are some examples:
One of the most annoying things you see in ill-managed data catalogs is the high frequency of stale and unused data assets. You can use Atlan to purge stale metadata based on custom rules.
Similarly, introducing a data catalog doesn’t add a lot of value if you cannot identify which of these assets comply with data quality rules to be classified as usable. Hence, freshness and accuracy are both critical metrics for data assets.
Your metadata should be able to indicate both of these. Atlan’s automation-based approach allows you to perform all these tasks with ease.
Amundsen does not provide a way to activate metadata out of the box. Building functionality to activate the metadata is entirely possible but requires much work.
Atlan vs. Amundsen: Things you must consider while evaluating these data catalogs #
- Managed vs. self-hosted tools
- Ease of setting up
- Integration with other tools
- Risk of failure adopting the tool
- The true cost of deploying an open-source tool
- Architecture
Managed vs. self-hosted data catalogs #
Amundsen is an open-source data catalog, while Atlan is an enterprise one. Open-source data catalogs are suitable for testing new approaches to solving data cataloging and discovery problems. Still, they can be challenging to manage at scale, especially when they’re early in their lifecycle with minimal community support and documentation.
As previously mentioned in the article, most companies don’t have expendable engineering capacity, so it is wise to use a managed data catalog without the ability to deploy serious engineering effort into maintenance and feature development for open-source project.
Tools like Atlan are, by definition, built to scale. They’re created using continuous customer feedback. With the infrastructure, DevOps, and feature development heavy lifting taken care of, managed data catalogs make a lot more sense if you’re looking for a scalable solution.
Ease of setting up #
Setting up Amundsen is easy, but scaling it is not. You can deploy Amundsen on the cloud platform of your choice. The barebones Amundsen setup will let you connect to your data sources but won’t help keep your data safe and secure because you’ll have to configure SSO with your OIDC provider. Integrating a SQL IDE such as Apache Superset is also a pain. You’ll have to make several changes and then build and deploy the project again.
You don’t need to do all that with Atlan. It comes with everything that’s expected of an enterprise tool, i.e., an architecture for high availability, reliability, a plan for disaster recovery, short RPO, and so on. Setting these up with an open-source tool is challenging and will cost you much more.
Integrating with other data tools #
Amundsen integrates with all the popular tools used in the data stack today. Moreover, it supports tools like Pandas Profiling for data quality and profiling checks, Apache Airflow for extracting data lineage and other data asset-related information, etc. Amundsen’s Databuilder library makes it easy to create and integrate any new data sources you might need.
Atlan goes a step beyond what Amundsen has done. Atlan has created deep integrations with tools like dbt and Snowflake to provide purpose-built features, such as column-level lineage and the treatment of dbt metrics as first-class citizens on the Atlan metadata platform. Atlan’s dbt + GitHub integration is another example. Atlan’s deep integration with Snowflake also made it the first data catalog to be certified as a Snowflake Ready Technology Partner.
Risk of failure adopting the tool #
Although there is always a risk of low or no adoption of any new tool, there are some indicators that can suggest a high probability of this happening. For example, a business that switched from Amundsen to Atlan noted that because Amundsen lacked embedded collaboration features, it led to knowledge and documentation silos on Slack, wiki spaces, and other platforms.
Failed Amundsen deployment. Image by Atlan.
A data cataloging tool is supposed to be something other than just for engineering teams. Instead, it is meant for everyone in the business to explore and use the data they need. When users find the tool too technical to use or the features too complicated to tune and refine, it does result in a failure. This risk reduces substantially when using a tool like Atlan that takes care of the ease of use for everyone in the business.
True cost of deploying an open-source tool #
From the first appearance, setting up and deploying open-source data catalogs looks very easy. Most of them come with well-written quick starts on Docker, some even on Kubernetes. The real pain begins when you start thinking about how the tool will fit into your data stack, how it will fare with your data privacy and security standards, and how easy it will be for people in your business to use.
The barebones setup is quick and easy. The tricky stuff is taking care of infrastructure, building authentication, ensuring high availability, architecting for high scalability, etc. If you don’t have expert engineers who have done this before, it is a non-trivial problem to solve. If you don’t have engineering capacity available, or even if you have it but want to make more appropriate use of your time, it’s better to go for a tool like Atlan, which is built on a mix of open-source and enterprise tools.
Architecture #
Amundsen architecture #
Amundsen is based on a microservices-based architecture with three core services: the frontend, search, metadata, and two supporting services, databuilder and common. You can deploy these services separately on Docker directly or use Kubernetes to orchestrate your containers for elastic scaling.
Amundsen uses a React and Flask-based frontend, an Elasticsearch-based search experience, and the ability to support multiple metadata stores, including neo4j, MySQL, Apache Atlas, etc., a metadata extraction and ingestion service.
When deploying Amundsen, you’ll need to make some code changes to ensure an integration with your OIDC provider to prevent unauthorized access to the data catalog. You can also deploy Amundsen on AWS with AWS Neptune as the graph database backend and AWS Fargate as the serverless compute engine.
Amundsen architecture. Source: Amundsen.
Atlan architecture #
Atlan runs its microservices on Kubernetes. Atlan uses a mix of managed open-source and enterprise tools like Loft, Rancher, Velero, Apache Calcite, Apache Atlas, and more. These tools play a crucial role in providing high availability, high scalability, and reliability to Atlan.
Atlan uses Apache Ranger for managing access and policies, Elasticsearch to power the natural-language search, Apache Kafka to update the latest metadata and communicate asynchronously across services, Redis as the caching layer, and HashiCorp Vault to store user credentials.
Atlan is deployed on AWS. To ensure data security and privacy at all times, all Atlan resources are isolated with AWS VPCs. Atlan mimics a single-tenant setup using CloudCover’s ArgoCD and Loft-based implementation that provides three layers of isolation between resources.
Summary #
This article walked you through the core features of the open-source data catalog Amundsen and the enterprise active metadata platform Atlan. It also took you through various factors you must know when comparing these data catalogs.
While we spoke about how both tools stack up, you should also consider their plans. Amundsen’s public roadmap is outdated and was last updated in 2021, although their Medium blog and YouTube channel has had some activity after that too. A lack of clarity here casts shadows over the reliability of the product and its future development.
Atlan’s focus on AI-led data governance and automation clarifies its stance for future development.
Finally, cost is one of the most critical aspects of implementing a new tool. Consider what these data catalogs will cost you if you deploy and run them in the long term. You can deploy Amundsen as a PoC on your machine and also ask for a proof-of-value from Atlan.
Atlan vs Amundsen: Related reads #
- Amundsen Data Catalog: Understanding Architecture, Features, Ways to Setup & More
- Amundsen Set Up Tutorial: A Step-by-Step Installation Guide Using Docker
- Amundsen Alternatives: DataHub, Metacat, and Apache Atlas
- Amundsen Demo : Explore and get a feel for Amundsen with a pre-configured sandbox environment.
- Atlan Product Demo
- Atlan vs. DataHub : Which Tool Offers Better Collaboration and Governance Features?
- Atlan vs Apache Atlas : What to Consider When Evaluating?
- Amundsen vs. DataHub: Which Data Discovery Tool Should You Choose?
Share this article