Roadblock! 5 Barriers to Big Data Governance
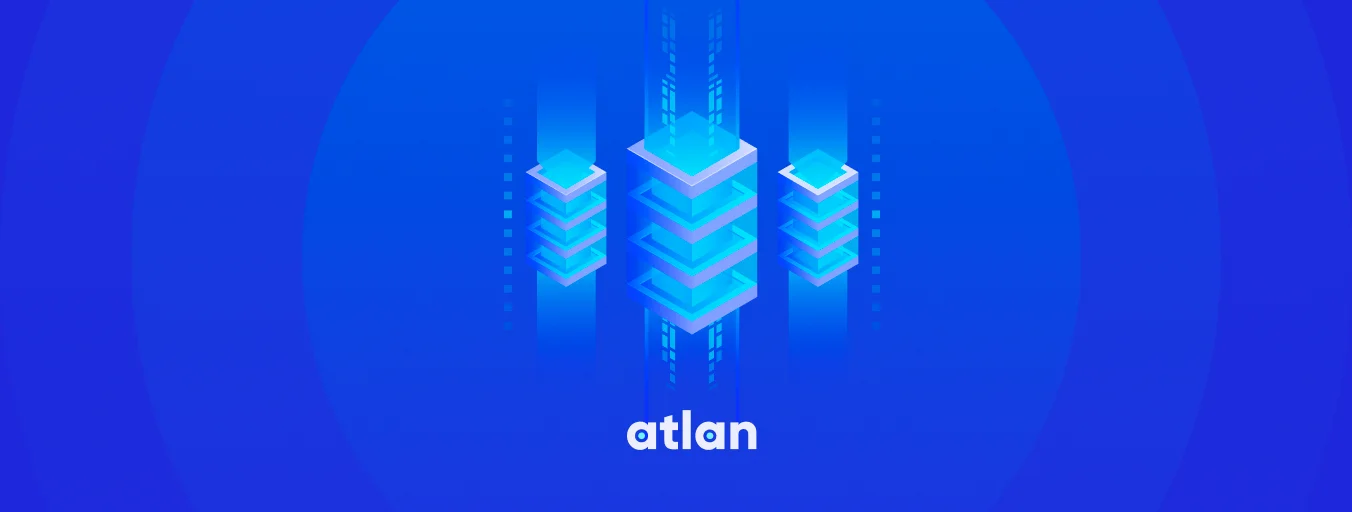
Share this article
Big data governance stands as a critical pillar, ensuring the reliability, security, and compliance of vast and intricate datasets. Yet, navigating the path to effective big data governance is not without its challenges.
In this article on “Barriers of Big Data Governance,” we delve into the challenges that organizations encounter in their quest to establish robust and comprehensive governance frameworks. Besides, we will also learn to build effective big data governance.
Let us begin!
Table of contents #
- What is big data governance?
- 5 Barriers to big data governance implementation
- Barriers to big data governance in healthcare
- What are the best practices for big data governance?
- How to choose the best tools for big data governance?
- Summarizing it all together
- Related reads
What is big data governance, & what does it entail? #
Big data governance refers to the set of processes, policies, and practices that organizations establish to ensure the effective management, quality, security, and compliance of their large and complex datasets.
It encompasses the entire lifecycle of data, from collection and storage to processing, analysis, and distribution. Here’re the salient features of big data governance:
- Data quality management
- Data security and privacy
- Data lineage and traceability
- Metadata management
- Data access and authorization
- Compliance and regulatory adherence
- Data lifecycle management
- Collaboration and communication
Let us now understand each of them in detail:
1. Data quality management #
- Data quality is foundational to effective big data governance.
- Organizations must establish standards for data accuracy, completeness, consistency, and reliability.
- Data quality management involves identifying and rectifying data errors, inconsistencies, and redundancies.
- By ensuring high-quality data, organizations can rely on accurate insights for decision-making and analysis.
2. Data security and privacy #
- Big data governance emphasizes robust data security and privacy measures.
- It involves implementing access controls, encryption, and authentication mechanisms to safeguard sensitive data.
- Organizations need to adhere to data privacy regulations (like GDPR) and establish policies to protect data from breaches, unauthorized access, and data leaks.
3. Data lineage and traceability #
- Data lineage tracks the journey of data from its source to its destination, providing transparency into data transformations, processes, and changes.
- Establishing data lineage ensures accountability.
- It aids in understanding data transformations which is crucial for both compliance and analytical accuracy.
4. Metadata management #
- Metadata is crucial for understanding the context, structure, and meaning of big data.
- Metadata management involves cataloging and organizing metadata to ensure that users can easily locate, understand, and utilize data assets.
- Effective metadata management facilitates data discovery, reduces redundancy, and enhances collaboration.
5. Data access and authorization #
- Big data governance enforces controlled access to data based on user roles and responsibilities.
- Organizations establish policies for data access, defining who can access what data and under what circumstances.
- This ensures that data is only accessible to authorized individuals, reducing the risk of data breaches and misuse.
6. Compliance and regulatory adherence #
- Compliance with industry regulations and data protection laws is a critical aspect of big data governance.
- Organizations must ensure that their data practices align with legal requirements such as GDPR, HIPAA, and others.
- This involves tracking data usage, obtaining appropriate consent, and maintaining records of data processing activities.
7. Data lifecycle management #
- Big data governance encompasses the entire data lifecycle, from creation to deletion.
- It involves defining data retention and archival policies, as well as procedures for data disposal.
- Effective data lifecycle management ensures that data is retained only for as long as necessary and is securely disposed of when no longer needed.
8. Collaboration and communication #
- Effective big data governance involves collaboration between different teams and departments.
- Communication is essential for ensuring that data governance practices are understood, adopted, and consistently followed across the organization.
- Clear communication promotes alignment, reduces redundancy, and enhances data accuracy.
By implementing these features, organizations can harness the full potential of big data while minimizing risks and maximizing the value of their data-driven initiatives.
5 Barriers/Challenges to big data governance implementation #
In this section, we unveil the challenges that stand in the way of implementing effective big data governance. From the intricacies of data awareness to the complexities of security, each barrier presents a unique puzzle to be solved on the path to unlocking the true value of data.
Here are the five most important barriers to big data governance:
- Lack of data awareness and understanding
- Data silos and fragmentation
- Complex regulatory and compliance landscape
- Technical challenges and data complexity
- Resistance to change and cultural shift
Let us understand each of them in detail:
1. Lack of data awareness and understanding #
- Many organizations struggle with a lack of awareness and understanding of the importance of big data governance.
- Stakeholders, including employees and management, might not fully comprehend the potential risks of poor data governance.
- This leads to apathy toward implementing effective governance practices.
- This lack of awareness hampers the adoption of data governance initiatives and can result in insufficient allocation of resources and support.
2. Data silos and fragmentation #
- Data silos occur when different departments or teams within an organization manage their data independently.
- This is leading to fragmented data management practices.
- These silos hinder the establishment of centralized governance policies and processes.
- Disparate systems, inconsistent data definitions, and varied data quality standards across silos impede data integration efforts.
- This may hinder the creation of a unified and comprehensive data governance framework.
3. Complex regulatory and compliance landscape #
- The regulatory and compliance landscape for big data is intricate and continuously evolving.
- Organizations must navigate a maze of regulations, including industry-specific rules like GDPR, HIPAA, and more.
- The challenge lies in understanding and adhering to these regulations while maintaining data quality and privacy.
- The complexity of compliance requirements can lead to confusion, potential breaches, and legal liabilities if not managed effectively.
4. Technical challenges and data complexity #
- Managing and governing vast volumes of diverse and complex data is a formidable challenge.
- Different data formats, structures, and sources make it difficult to establish consistent governance processes.
- Inadequate tools for data integration, data profiling, and data lineage tracking can hinder effective data governance efforts.
- Moreover, technological limitations can prevent real-time data governance, especially for rapidly changing or streaming data.
5. Resistance to change and cultural shift #
- Implementing effective big data governance often requires a cultural shift within the organization.
- Resistance to change, especially from stakeholders accustomed to working within silos, can hinder the adoption of new governance practices.
- Convincing employees and leadership to embrace new data-driven behaviors and prioritize data governance can be challenging.
- Overcoming resistance requires robust change management strategies, training programs, and consistent communication about the benefits of governance.
By recognizing and strategically addressing these barriers, organizations can establish effective governance practices that maximize the value of their big data investments while minimizing risks and uncertainties.
Barriers to big data governance in healthcare #
In this section, we will look at how overcoming obstacles to efficient big data governance is supremely important in an industry like healthcare. This is simply because of the troves of data that the healthcare industry generates every day.
Big data governance in the healthcare industry faces several significant barriers due to the complex nature of healthcare data, regulatory concerns, technological challenges, and ethical considerations.
These are the following barriers to big data governance faced in the healthcare industry:
- Data complexity and diversity
- Data volume and velocity
- Data quality and accuracy
- Privacy and security
- Regulatory compliance
- Interoperability
- Lack of data governance frameworks
- Cultural resistance and change management
- Skills gap
- Ethical considerations
- Cost and infrastructure
- Data stewardship and ownership
Let us understand each of them in detail:
1. Data complexity and diversity #
- Healthcare data comes from various sources, including structured data (e.g., EHRs) and unstructured data (e.g., medical images, clinical notes).
- This diversity makes it challenging to establish standardized governance practices that apply universally to all data types.
- Each data type has its own structure and requirements, making it challenging to create standardized governance processes that can accommodate all types of data effectively.
2. Data volume and velocity #
- The healthcare industry generates a vast amount of data every day.
- This volume, coupled with the rapid rate at which data is generated (velocity), poses challenges in terms of data storage, processing, and analysis.
- Traditional systems may struggle to handle the sheer amount of data.
- The sheer volume of healthcare data generated daily can overwhelm traditional data management systems.
- The high velocity at which new data is generated requires robust infrastructure capable of processing and storing data in real time.
3. Data quality and accuracy #
- Healthcare decisions are critical and based on accurate information.
- Ensuring data quality involves dealing with duplicate records, missing information, and incorrect entries. It requires continuous monitoring, validation, and data cleansing efforts.
- Healthcare data must be accurate and reliable for clinical decision-making. Inaccurate or incomplete data can lead to incorrect diagnoses and treatment decisions.
- Ensuring data quality and accuracy is essential, but it’s complex due to the varied sources of data and potential entry errors.
4. Privacy and security #
- Healthcare data contains sensitive patient information.
- Healthcare data is sensitive and subject to strict regulations such as the Health Insurance Portability and Accountability Act (HIPAA) in the United States.
- Ensuring data privacy and security while allowing for data sharing and analysis is a delicate balance. Unauthorized access to patient data can lead to breaches and violations.
- Ensuring privacy and security while allowing legitimate data sharing and analysis involves encryption, access controls, authentication mechanisms, and auditing.
- Striking the right balance between data accessibility and security is complex.
5. Regulatory compliance #
- The healthcare industry is heavily regulated, with data governance requirements varying across regions.
- Complying with these regulations, such as GDPR in Europe or HIPAA in the US, while still benefiting from big data analytics can be challenging.
- Non-compliance can result in severe legal and financial consequences.
- Organizations need to implement measures to comply with laws like HIPAA, which include data access controls, audit trails, breach reporting, and patient consent mechanisms.
6. Interoperability #
- Healthcare data often originates from disparate systems and formats.
- Interoperability challenges arise when trying to integrate data from different sources into a cohesive dataset.
- Standardizing data formats, structures, and terminologies is crucial for meaningful analysis.
- Healthcare data is often siloed across different systems, making it challenging to integrate and analyze holistically.
- Standardizing data formats (like FHIR for EHRs), developing interoperability standards, and adopting common data models are necessary to enable seamless data exchange.
7. Lack of data governance frameworks #
- Many healthcare organizations lack comprehensive data governance frameworks that outline policies, roles, responsibilities, and processes.
- Establishing a framework that covers data ownership, data lifecycle management, data quality, and compliance is crucial.
8. Cultural resistance and change management #
- Implementing data governance often requires changes in organizational culture and practices.
- Healthcare professionals might be resistant to adopting new technologies and processes, which can impede the successful implementation of data governance initiatives.
- Integrating data governance practices requires buy-in from stakeholders and comprehensive change management strategies to educate and train staff about the benefits of new processes.
9. Skills gap #
- The healthcare industry requires professionals skilled in data management, analytics, security, and compliance.
- However, there’s often a shortage of professionals with these skills in the healthcare industry.
- Bridging the skills gap through training programs and hiring experts is essential for effective data governance implementation.
10. Ethical considerations #
- Using patient data for research raises ethical dilemmas related to informed consent, data anonymization, and potential bias in analyses.
- Striking a balance between advancing medical knowledge and safeguarding patients’ rights is critical.
11. Cost and infrastructure #
- Building and maintaining the necessary infrastructure to store, process, and analyze large amounts of data can be costly.
- Smaller healthcare organizations might face financial constraints that hinder their ability to invest in advanced technologies.
12. Data stewardship and ownership #
- Determining who owns and is responsible for healthcare data can be complex, especially in cases where patients’ personal data is involved.
- Clear definitions of data ownership, stewardship, and responsibility are essential for maintaining accountability.
A multidisciplinary approach is necessary to overcome these challenges and establish effective big data governance practices in the healthcare industry.
What are the best practices for big data governance? #
Just as skilled navigators chart their course through uncharted waters, organizations must steer their data-driven journey with precision and strategy.
This section serves as the guiding star in this journey, illuminating the path toward data integrity, security, and value extraction.
These practices are not mere recommendations, but proven strategies that organizations can leverage to transform their data into a strategic asset.
Here are the best practices for big data governance:
- Data governance framework
- Data ownership and accountability
- Data classification and sensitivity
- Data security and privacy
- Data access controls
- Data retention and deletion policies
- Data lineage and auditing
- Data integration and interoperability
- Change management and collaboration
- Data stewardship
- Continuous improvement
- Data ethics and transparency
Let us understand each of them in detail:
1. Data governance framework #
- Establish a comprehensive data governance framework that outlines policies, roles, responsibilities, and processes.
- This framework should cover data ownership, data lifecycle management, data quality standards, and compliance measures.
- A well-defined framework provides a roadmap for implementing consistent governance practices.
2. Data ownership and accountability #
- Clearly define data ownership, ensuring that specific individuals or teams are responsible for different aspects of data management.
- This includes data creation, validation, storage, access control, and sharing.
- Having accountable stakeholders helps maintain data integrity and security.
- Establishing accountability helps prevent data-related issues and ensures that data management responsibilities are well-defined.
3. Data quality management #
- Implement processes to monitor and ensure data quality.
- It includes data profiling, validation, cleaning, and enrichment processes to maintain high-quality data for decision-making.
- Data quality directly impacts the accuracy and reliability of analysis and decision-making.
4. Data classification and sensitivity #
- Classify data based on its sensitivity and regulatory requirements.
- This helps define access controls, encryption levels, and other security measures.
- Sensitivity labeling ensures that appropriate security measures are applied to different types of data.
- This enables the organization to apply appropriate security controls, access permissions, and encryption mechanisms to protect sensitive data from unauthorized access or breaches.
5. Data security and privacy #
- Implement robust security measures to protect data from unauthorized access and breaches.
- Use encryption, access controls, authentication mechanisms, and regular security audits.
- For healthcare data, ensure compliance with relevant regulations like HIPAA or GDPR.
- Privacy measures ensure compliance with regulations by protecting personally identifiable information (PII) and sensitive patient data.
6. Data retention and deletion policies #
- Develop policies for data retention and deletion.
- Data that is no longer needed should be properly archived or deleted to reduce the risk of unauthorized access and ensure compliance with data protection regulations.
- These policies establish guidelines for how long data should be retained based on legal, regulatory, and business requirements.
- Proper data retention and deletion practices ensure compliance and prevent data accumulation.
7. Data lineage and auditing #
- Data lineage tracks the complete journey of data, from its origin to its various transformations and destinations.
- Auditing logs data access and usage, providing transparency and accountability, which is crucial for compliance and identifying unauthorized activities.
- Establish data lineage to track the origin and transformations of data throughout its lifecycle.
- Regularly audit data access and usage to ensure compliance with governance policies and detect any anomalies.
8. Data integration and interoperability #
- Standardize data formats, terminologies, and data models to ensure interoperability across different systems and sources.
- Interoperability enables better data sharing and collaboration across the organization.
- This facilitates seamless data integration and analysis.
9. Change management and collaboration #
- Change management strategies help navigate resistance to adopting new governance practices by involving stakeholders in the decision-making process.
- Collaboration between IT, business units, legal, and compliance teams ensures alignment and consistent implementation.
- Implement change management strategies to facilitate the adoption of data governance practices.
- Foster collaboration among different departments, teams, and stakeholders to ensure alignment with organizational goals.
10. Data stewardship #
- Data stewards are individuals or teams responsible for specific datasets’ quality, security, and compliance.
- They act as champions for data governance within their domains and facilitate communication between different stakeholders
- Appoint data stewards responsible for specific datasets or domains.
- Data stewards are accountable for data quality, compliance, and resolving data-related issues.
11. Continuous improvement #
- Data governance is an ongoing process. Regularly review and update governance policies and practices to adapt to changes in data sources, regulations, and technology.
- This iterative approach ensures that governance practices remain effective over time.
12. Data ethics and transparency #
- Consider ethical considerations when using data for analysis.
- Be transparent about data usage, obtain necessary consent, and avoid biases that might emerge from improper data handling.
- Adhering to ethical considerations ensures that data usage respects individuals’ rights and privacy.
- Transparency involves communicating how data is used, obtaining informed consent, and avoiding bias in analysis.
By implementing these practices, organizations can ensure that their big data initiatives are well-governed, compliant, and aligned with the organization’s goals and values. Effective big data governance not only mitigates risks but also maximizes the value derived from data-driven initiatives.
How to choose the best tools for big data governance? #
Selecting the right tools for big data governance is essential to effectively manage, secure, and ensure the quality of your data. Choosing the right tool for your organization involves careful consideration of various factors:
1. Business requirements #
Understand your organization’s specific needs. Do you require robust metadata management, data lineage tracking, or data quality assessment? Prioritize the features that align with your business goals.
2. Integration capabilities #
Consider how well the tool integrates with your existing data ecosystem. Seamless integration with your data storage, processing, and analysis tools is crucial for effective governance.
3. Scalability #
Ensure that the tool can scale to handle your organization’s current and future data volumes. As data grows, your governance tool should be able to accommodate the increased workload.
4. User experience #
Choose a tool with an intuitive interface that your teams can easily navigate. User adoption is essential for successful governance implementation.
5. Security and compliance #
Data privacy and security are paramount. Choose a tool that supports encryption, access controls, and other security measures to safeguard sensitive data.
6. Vendor support #
Consider the level of support provided by the vendor. Timely assistance and regular updates are crucial for addressing issues. It is essential to stay up to date with evolving data governance requirements.
7. Cost #
Evaluate the total cost of ownership, including licensing, implementation, training, and maintenance. Consider both short-term and long-term expenses.
By thoroughly evaluating these tools against your organization’s specific needs and considering factors such as integration, scalability, user experience, and security, you can make an informed decision that supports your big data governance objectives effectively.
Summarizing it all together #
In the ever-expanding landscape of big data, governance emerges as the steadfast guardian of data integrity, security, and value.
As we traverse the realms of big data governance, we’ve explored its essence, significance, and the challenges it presents. The journey led us through the fundamental principles of data privacy automation.
From the vantage point of data engineers, we explored the art of backfilling data—a meticulous dance that bridges gaps in historical records, infusing accuracy into insights and safeguarding against mistakes.
Embrace the journey of data governance with open arms, for within its depths lie the seeds of trust, innovation, and enduring success. As the digital age forges ahead, let these insights be your compass, guiding you through the challenges and opportunities of managing the vast expanse of data that powers our world.
Barriers to big data governance: Related reads #
- Data Lineage Explained: A 10-min Guide to Understanding the Importance of Tracking Your Data’s Journey
- What is Data Governance? Its Importance & Principles
- Data Governance 101: Principles, Examples, Strategy & Programs
- What is Metadata? - Examples, Benefits, and Use Cases
- Metadata Management: Benefits, Automation & Use Cases
- Speed Bump! 10 Data Governance Challenges to Overcome
Share this article