9 Components to Build the Best Data Quality Framework
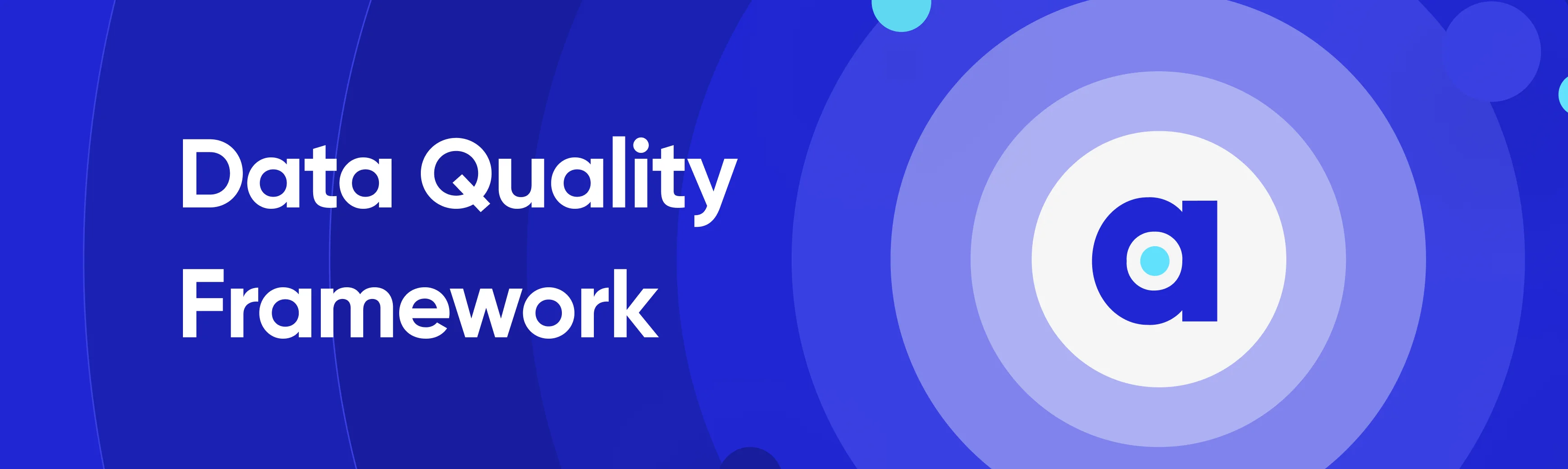
Share this article
A data quality framework is a comprehensive set of guidelines, methods, and tools that businesses use to manage, improve, and ensure the quality of their data.
It involves strategies to validate, clean, transform, and monitor data so that it’s accurate, consistent, complete, reliable, and timely for its intended uses. It’s a crucial part of any data governance program because it helps organizations make data-driven decisions with confidence.
In this blog, we delve into the key components, creation process, and available resources for mastering data quality frameworks. Whether you’re a data professional, analyst, or business leader, this blog will equip you with the knowledge to unleash the power of high-quality data in your projects and decision-making processes.
Let’s dive in!
Table of contents
- Key components of a data quality framework
- What is a data quality framework composed of?
- Best data quality frameworks
- How can you create a data quality framework
- Template for crafting an effective data quality framework
- Books and resources for mastering data quality framework
- Summarizing all the points
- Data quality framework: Related reads
What are the key components of a data quality framework?
Today, organizations must establish robust frameworks to ensure the quality of their data assets. So, let us take a look at the key components of a data quality framework:
- Data governance
- Data profiling
- Data quality rules
- Data quality assessment
- Data cleaning
- Data monitoring
- Data issue management
- Data reporting
- Continuous improvement
Now, let us look into each of the above key components in brief:
1. Data governance
Data governance includes policies, standards, and guidelines that provide a direction on how data should be collected, stored, managed, and used within an organization. This is the foundation of any data quality framework.
2. Data profiling
Data profiling involves examining the data available in an organization and collecting statistics or informative summaries about that data. It helps in identifying anomalies, inconsistencies, or inaccuracies in the data.
3. Data quality rules
Data quality rules are sets of predefined rules or constraints that help in checking the accuracy, validity, consistency, and completeness of data. They can be business rule checks, cross-dataset checks, or checks against external data sets or services.
4. Data quality assessment
This involves a regular audit or review of data quality performance using the data quality rules. It’s usually done using data quality scorecards that are tailored to meet the organization’s data quality needs.
5. Data cleaning
This involves detecting and correcting (or removing) corrupt, inaccurate, or erroneous records from a dataset or database.
6. Data monitoring
This involves the continuous tracking and monitoring of data quality metrics to ensure ongoing compliance with the data quality standards.
7. Data issue management
Involves resolving data quality issues that are found during data profiling, data quality assessment, and data monitoring.
8. Data quality reporting
This provides a report on the state of data quality within an organization. It’s an important tool for communicating data quality status and progress to stakeholders.
9. Continuous improvement
As the needs of an organization change, the data quality framework should adapt to meet these new requirements. It involves continuous analysis, measurement, improvement, and control of data quality efforts.
A successful data quality framework combines these elements with technology (like data cataloging tools, data quality software, etc.), processes (like defining data quality metrics, setting up data stewardship roles, etc.), and people (like data stewards, data owners, etc.). It helps ensure that high-quality data is available, thereby supporting decision-making and operational efficiency.
What is a data quality framework composed of?
A data quality framework can be thought of as a structure that encompasses strategies, procedures, standards, technologies, and measures to ensure and improve data quality. The composition of such a framework includes:
- Data quality standards
- Data profiling
- Data quality assessment
- Data quality reporting
- Data cleaning
- Monitoring and control
- Data governance
Let us look into each of them in brief:
1. Data quality standards
These are the criteria that your data needs to meet to be considered of ‘high quality’. The specific standards used can vary greatly depending on the nature of the data and the use case.
For example, in a financial institution, data quality standards might include an accurate representation of transactional amounts and correct customer details.
2. Data profiling
This is the process of examining the data available in an existing database and collecting statistics and information about that data.
For instance, data profiling could identify the average age of customers in a retail company’s database, or the number of missing or null values in a certain column.
3. Data quality assessment
This is the process of evaluating the quality of your data against the predefined standards. Data quality issues like duplicates, inconsistencies, and inaccuracies are identified during this step.
For instance, in a dataset of employee records, an assessment might find that some records have been duplicated. In other words, the ‘Salary’ column contains a non-numeric value.
4. Data quality reporting
After data is assessed, a report is generated that includes the findings of the data quality check. This might involve:
- Generating a data quality score
- Highlighting specific data quality issues
- Producing other summaries
- Visualizations of data quality
5. Data cleaning
This step involves resolving the issues found during the assessment stage. Data cleaning could involve tasks such as:
- Removing duplicates
- filling in missing values
- Correcting inaccurate data
6. Data quality improvement
This refers to ongoing efforts to improve data quality, such as refining data collection procedures. This also involves implementing new technology to prevent data quality issues from occurring in the first place.
For instance, a business might implement a new data entry system with built-in data validation rules to prevent incorrect data from being entered.
7. Monitoring and control
This is a continuous process of checking the data quality at regular intervals to ensure the standards are being maintained.
8. Data governance
This is the overarching strategy that aligns all the data quality efforts to ensure they’re in line with business objectives. It involves setting up policies, procedures, and responsibilities related to data quality.
Now, let us take an example of a retail company implementing a data quality framework. The company may define its data quality requirements in the below manner:
- Data quality standards: The company decides that all customer records should have a valid email address, a non-null purchase history, and accurate demographic information.
- Data profiling: The company examines its current customer database to identify patterns, anomalies, and areas that need attention.
- Data quality assessment: The company finds that 10% of their customer records have an invalid or missing email address.
- Data quality reporting: The company generates a report detailing the issues with their data, including the specific problem with email addresses.
- Data cleaning: The company undertakes an effort to correct or fill in missing/invalid email addresses, possibly by reaching out to customers directly or using other available data.
- Data quality improvement: The company decides to implement a new customer onboarding form that validates email addresses at the point of entry, preventing this issue from occurring in the future.
- Monitoring and control: The company sets up regular checks of its customer data to quickly identify and address any future data quality issues.
- Data governance: The company establishes a data governance team responsible for overseeing and guiding these efforts, setting policies for data collection and management, and ensuring alignment with business goals.
These components work together in a data quality framework, with each one building upon and informing the others.
Choosing the best data quality frameworks for your next project
There are several established data quality frameworks available that provide guidelines and best practices for maintaining and improving data quality. Here are a few examples:
1. The DAMA guide to the data management body of knowledge (DAMA-DMBOK):
The DAMA-DMBOK is a comprehensive guide to data management that includes a chapter on data quality. It’s a valuable resource for anyone looking to develop a data quality framework.
2. The data quality framework from the Australian Institute of Health and Welfare (AIHW):
The data quality framework by the Australian Institute of Health and Welfare is a framework specifically designed for the health sector. It covers six dimensions of data quality:
- Institutional environment
- Relevance
- Timeliness
- Accuracy
- Coherence
- Interpretability
3. TDWI’s data quality management framework
This framework is described in the TDWI report “Data Quality and the Bottom Line”. It’s a comprehensive guide that covers everything from data governance to data profiling, and it provides actionable advice for businesses looking to improve their data quality.
4. The MIT Information Quality (MITIQ) program
This program has developed a data quality framework that emphasizes the business impacts of data quality. The framework covers data quality dimensions, business processes, and the role of data governance.
5. ISO 8000 data quality model
The ISO 8000 data quality model is an international standard for data and information quality management. It provides a model for describing, measuring, and managing data quality.
6. The data quality assessment framework (DQAF) by IMF
The International Monetary Fund has developed a framework specifically for the assessment of data quality, especially in the realm of macroeconomic and financial statistics. The framework focuses on five dimensions: assurances of integrity, methodological soundness, accuracy and reliability, serviceability, and accessibility.
These frameworks all approach data quality from slightly different perspectives, but they each provide valuable guidelines and insights for ensuring and improving the quality of data in an organization.
It’s worth noting that each organization will likely need to customize any chosen framework to best suit their unique needs and circumstances.
How can you create a data quality framework: A step-by-step guide
Here is a general step-by-step process for creating a data quality framework for an organization:
- Understand the business needs
- Define data quality goals
- Data quality assessment
- Establish data governance
- Implement data quality rules
- Automate data quality processes
- Data cleansing
- Monitor, control, and report
- Implement continuous improvement practices
- Training and culture
- Review and update the framework regularly
Let us look into each step in detail:
1. Understand the business needs
Identify the critical data elements that are used to drive business decisions. These are typically data elements that appear in reports, dashboards, and other decision-making tools. Also, understand the current data-related pain points, such as reporting inaccuracies, slow processing times, etc.
2. Define data quality goals
Define what data quality means for your organization. This typically involves identifying the key dimensions of data quality (e.g., accuracy, completeness, timeliness, consistency, and relevance). Each dimension should have a specific goal associated with it that aligns with business needs.
3. Data quality assessment
Profile your data to understand its current quality status. Data profiling involves statistical analysis and review of data to understand patterns, anomalies, and errors. This step is critical to understand the extent and nature of your data quality issues.
4. Establish data governance
Create a data governance committee or designate data stewards who will own the data quality process. The governance structure should be accountable for meeting the data quality goals.
5. Implement data quality rules
Based on your goals, create data quality rules that can be applied to validate and clean data. These rules should cover all critical data elements identified earlier.
6. Automate data quality processes
Automation is key to maintaining high data quality over time. Implement data quality tools that can automate the process of checking and cleaning data.
7. Data cleansing
Correct the current issues identified in your data assessment. This may involve standardizing data, removing duplicates, correcting errors, and filling gaps in data.
8. Monitor, control, and report
Establish ongoing monitoring and reporting of data quality metrics. These metrics should be regularly reviewed by the data governance committee to ensure you’re meeting your data quality goals.
9. Implement continuous improvement practices
Data quality isn’t a one-time initiative. It’s an ongoing practice that should continuously be reviewed and improved. Use feedback from the data quality reports to refine your processes, and consider using techniques like Six Sigma. In other words lean to facilitate continuous improvement.
10. Training and culture
Train staff on the importance of data quality and the processes you’ve implemented. Creating a culture that values data quality is key to ensuring these initiatives are successful.
11. Review and update the framework regularly
The data quality framework should be a living document. Review it regularly and make updates as needed to ensure it continues to align with your business needs and goals.
Remember, the data quality framework should be customized to fit the specific needs and capabilities of the organization. It may take time to establish, but once in place, it can greatly improve the reliability and usability of your data.
A template for crafting an effective data quality framework
Here’s a basic template for a Data Quality Framework document along with a few elements to keep in mind:
1. Executive summary
- Write a brief description of the purpose and goal of the Data Quality Framework.
2. Introduction
- A deeper explanation of the Data Quality Framework’s role and the business needs that it will serve.
3. Business needs and objectives
- A detailed account of the business needs and objectives that necessitate the framework.
- Identification of critical data elements.
4. Data quality goals
- Explanation of the data quality goals and the key dimensions of data quality relevant to your organization (e.g., accuracy, completeness, timeliness, consistency, and relevance).
5. Data governance
- Outline of the data governance structure including roles and responsibilities.
6. Data quality rules
- Details of the data quality rules for validation and cleaning of data.
7. Data quality assessment
- Procedure and frequency of data quality assessments.
- Statistical methods or tools used for data profiling.
8. Data quality monitoring, control, and reporting
- Description of the procedures for monitoring, control, and reporting.
- Explanation of the metrics used for data quality evaluation.
- The frequency at which these metrics will be reported and reviewed.
9. Data quality improvement
- Explanation of the continuous improvement process.
- Methods for implementing improvements based on data quality reports.
10. Data cleansing
- The procedure for correcting the issues identified in your data assessment.
11. Training and culture
- Description of the staff training plan.
- Measures to be taken to build a data-centric culture.
12. Framework review and update procedure
- Explanation of how and when the Data Quality Framework will be reviewed and updated.
13. Conclusion
- Final thoughts and a brief recap of the Data Quality Framework.
Appendix
- Include any supporting documentation or materials like a glossary of terms, reference materials, etc.
This template provides an overarching structure for creating a Data Quality Framework, but remember to tailor it to the specifics of your organization. It’s essential to regularly review and update the document to reflect any changes in the organization’s objectives or the data environment.
Books and resources for mastering data quality framework
Here are some books and resources where you can learn more about data quality frameworks:
Books:
- “Data Quality: The Accuracy Dimension” by Jack E. Olson - This book focuses on the dimension of accuracy within data quality, and gives a detailed account of how to ensure data is accurate.
- ”Data Quality Assessment” by Arkady Maydanchik - This book provides a comprehensive resource for understanding and implementing data quality assessment in your organization.
- ”Executing Data Quality Projects: Ten Steps to Quality Data and Trusted Information” by Danette McGilvray - This book presents a systematic, proven approach to improving and creating data and information quality within the enterprise.
- ”Data Governance: How to Design, Deploy and Sustain an Effective Data Governance Program” by John Ladley - This book provides a comprehensive overview of data governance, including the necessary components of a successful program.
- ”The Data Warehouse ETL Toolkit: Practical Techniques for Extracting, Cleaning, Conforming, and Delivering Data” by Ralph Kimball and Joe Caserta - This book provides a comprehensive guide to the entire ETL (Extract, Transform, Load) process, and includes valuable advice on ensuring data quality throughout.
Online Resources:
- DAMA International’s Data Management Body of Knowledge (DMBOK): DAMA International’s Guide to the Data Management Body of Knowledge is a comprehensive, rigorous reference that outlines the scope and understanding necessary to create and manage a data management program.
- Data Governance Institute (DGI): The DGI provides in-depth resources on data governance and data quality, including a useful model for a data governance framework.
- Data Quality Pro: This is a free online resource that offers a wealth of articles, webinars, and tutorials on various data quality topics.
- Online Courses: Websites like Coursera, edX, and Udemy offer courses on data quality management and data governance that can help deepen your understanding of these topics.
- IBM’s Information Governance Catalog: While not a learning resource in the traditional sense, this catalog provides a real-world example of a comprehensive, well-documented information governance framework.
Remember that the process of establishing a data quality framework in your organization is iterative and will involve learning from both successes and failures. Therefore, take advantage of the collective knowledge shared through these resources, but don’t be afraid to adapt and create a framework that best fits your organization’s unique needs.
Summarizing all the points
A data quality framework is a structured plan to ensure and manage the quality, reliability, and integrity of data in an organization. It involves different dimensions of data quality including accuracy, completeness, consistency, timeliness, validity, and uniqueness.
The essential components of a data quality framework, provide insights into its composition and practical application. The data quality framework should be customized to fit the specific needs and capabilities of the organization. It may take time to establish, but once in place, it can greatly improve the reliability and usability of your data.
Data quality framework: Related reads
- Data Quality Explained: Causes, Detection, and Fixes
- Data Quality Measures: Best Practices to Implement
- How to Improve Data Quality in 10 Actionable Steps?
- Data Quality in Healthcare: Best Practices & Key Considerations
- What Is Data Lineage & Why Is It Important?
- What Is a Data Catalog? & Why Do You Need One in 2023?
- What is Data Governance? Its Importance, Principles & How to Get Started?
- What is Metadata? - Examples, Benefits, and Use Cases
- Is Atlan compatible with data quality tools?
Share this article