What is Data Lake Metadata Management? Benefits, Examples, Tools, & Key Factors to Consider
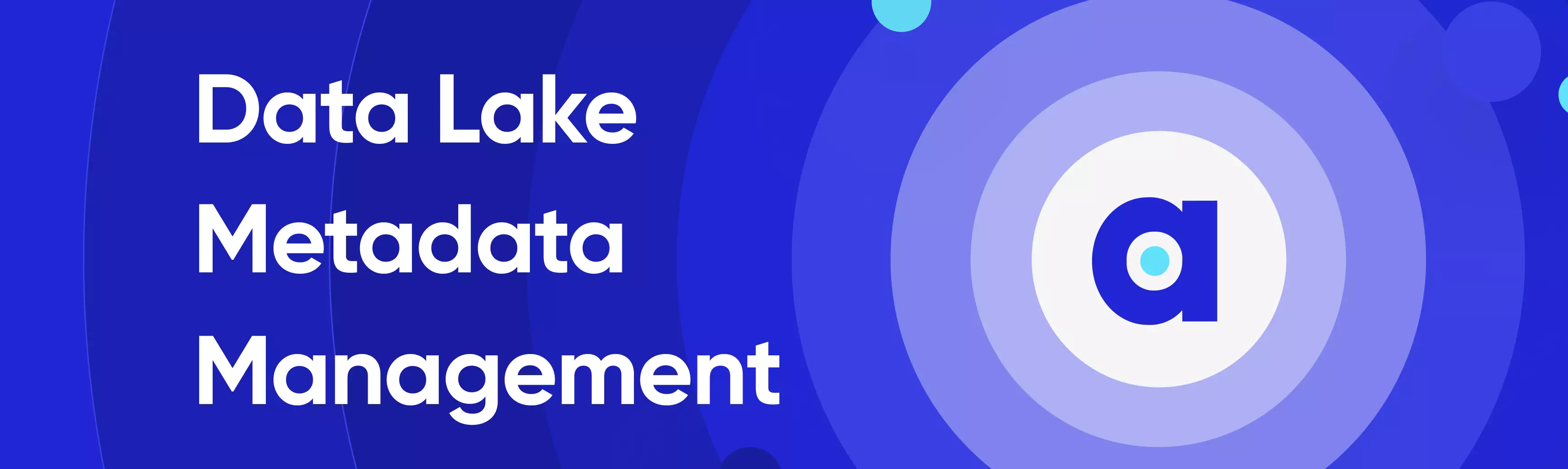
Share this article
Today, data-driven businesses rely on data lakes to store and manage vast amounts of diverse data. However, the success of a data lake relies not only on the infrastructure and technologies employed but also on effective metadata management.
Why?
Because metadata holds the key to unlocking the full potential of a data lake. It provides crucial information about the stored data, its origins, structure, relationships, and usage, enabling organizations to derive meaningful insights and make informed decisions.
Table of contents #
- The crucial role of metadata management in data lakes
- How do metadata management tools actually work with data lakes?
- How metadata management of data lakes helps drive business growth: Practical examples
- Evaluating metadata management tools: Factors to consider
- Bringing it all together
- Metadata management of data lakes: Related reads
The crucial role of metadata management in data lakes #
In this section of the blog, we will look into the critical reasons why metadata management plays a pivotal role in the establishment and ongoing operation of an enterprise-wide data lake. Here are several reasons why:
- Data discovery
- Data quality
- Data governance and compliance
- Data integration
- Data usability
- Data security
- AI and machine learning
Let us look into each of these reasons in detail:
1. Data discovery #
Metadata describes the data stored in the data lake, providing details such as its source, its structure, its meaning, its relationships with other data, and its usage. This makes it easier for users to discover relevant data in the vast amounts of data stored in the data lake.
2. Data quality #
Metadata helps ensure the quality of the data in the data lake. By documenting the lineage of data (where it came from, who modified it, when, and why), metadata can help track and fix data quality issues.
3. Data governance and compliance #
Metadata helps enforce data governance policies and comply with data privacy and protection regulations. It allows tracking of who has access to what data, what they can do with it, and what they have done with it.
4. Data integration #
Metadata can facilitate the integration of data from various business units into the unified data lake. It can be used to map data elements across different systems, enabling a consistent view of data across the enterprise.
5. Data usability #
Metadata provides context for data, making it more understandable and usable. This is especially important in a data lake, where data from various sources and in various formats is stored.
6. Data security #
Metadata can also be used to implement security controls, such as access permissions and data masking.
7. AI and machine learning #
Metadata is crucial for AI and machine learning applications that consume data from the data lake. It helps algorithms understand what the data represents, which can improve their performance.
Implementing an effective metadata management strategy requires tools and processes to collect, store, update, and use metadata. It also requires a culture that values data as an enterprise asset and understands the importance of metadata in realizing the value of that asset.
How do metadata management tools work with data lakes? #
Metadata management tools work with data lakes in several key ways, and they are:
- Data cataloging
- Data lineage tracking
- Semantic tagging
- Data quality monitoring
- Data governance enforcement
- Integration with other tools
Let us look into each of these tools in brief:
1. Data cataloging #
Metadata management tools automatically catalog all data ingested into the data lake. They record information about the source, format, structure, and content of the data, as well as any transformations applied to it. This information is stored in a metadata repository and can be searched and browsed by users.
2. Data lineage tracking #
Metadata management tools track the lineage of the data. They record where it came from, who has accessed it, what changes have been made to it, and where it has been used. This information can be used to troubleshoot data quality issues, audit data usage, and ensure compliance with data governance policies.
3. Semantic tagging #
Metadata management tools can automatically tag data with semantic metadata, which describes the meaning and context of the data. This can be used to improve data discovery and integration.
For example, a tool might tag all data elements that contain customer names, so they can be easily found and integrated across different data sources.
4. Data quality monitoring #
Some metadata management tools can monitor data quality based on metadata. They can check whether the data conforms to specified formats, ranges, or other constraints, and alert users to any anomalies.
5. Data governance enforcement #
Metadata management tools can enforce data governance policies based on metadata. They can control who has access to what data, what they can do with it, and what they have done with it. They can also help ensure that data is handled in compliance with data privacy and protection regulations.
6. Integration with other tools #
Metadata management tools often integrate with other tools in the data lake ecosystem. They can feed metadata to data integration tools to facilitate data mapping and transformation. They can also provide metadata to BI and analytics tools to enhance data exploration and visualization.
In order to be effective, metadata management tools need to be part of an overall data management strategy that includes processes for metadata creation, storage, update, use, and archiving. They also need to be supported by a data culture that recognizes the value of metadata and encourages its use.
How metadata management of data lakes helps drive business growth: Practical examples #
Here are a few practical illustrations that show how metadata management of data lakes can drive business growth and tangible impact:
- Customer segmentation and personalized marketing
- Product development
- Supply chain optimization
- Regulatory compliance
- Fraud detection
Let us look into each of these examples:
1. Customer segmentation and personalized marketing #
An e-commerce company has vast amounts of data about its customers and their behaviors. By managing metadata effectively, the company can discover all the data elements related to customer behavior across different data sources in their data lake.
By integrating this data and applying advanced analytics, the company can identify distinct customer segments and tailor marketing messages to each segment, thereby increasing customer engagement and sales.
2. Product development #
A software company uses a data lake to store data from various sources, including customer feedback, software usage data, and market research data.
With robust metadata management, they can quickly locate and integrate relevant data to identify patterns and trends, such as frequently requested features or common usability issues.
This insight can drive the development of new features and improvements that increase customer satisfaction and retention.
3. Supply chain optimization #
A manufacturing company maintains data about its supply chain in a data lake. Using metadata management tools, it can quickly identify data related to supplier performance, inventory levels, and production schedules.
Combining this data with predictive analytics, the company can anticipate supply chain issues, optimize inventory levels, and reduce production downtime, leading to cost savings and increased productivity.
4. Regulatory compliance #
A financial services firm has to comply with a wide range of regulations that require it to report on various aspects of its operations. Effective metadata management can help the firm identify and integrate the necessary data from its data lake to automate and streamline the creation of these reports, reducing the risk of non-compliance and the associated penalties.
5. Fraud detection #
A credit card company uses a data lake to store transaction data. By managing metadata effectively, the company can link together disparate pieces of data to detect unusual patterns that might indicate fraudulent activity. Rapid detection allows the company to respond swiftly, reducing financial losses and improving customer trust.
In each of these examples, effective metadata management enables the company to leverage its data lake to gain insights that drive business growth and create tangible impact.
Evaluating metadata management tools: Factors to consider #
When evaluating metadata management tools for your data lake initiative, consider the following factors:
- Integration capabilities
- Automated metadata collection
- Scalability
- Search and discovery features
- Data governance and compliance support
- Data quality management
- Collaboration features
- Cost
Let us look into each of the above factors in detail:
1. Integration capabilities #
The tool should be able to work seamlessly with your existing data infrastructure, including your data lake, databases, ETL tools, BI tools, and other data management and analytics platforms. It should support the data formats and protocols used by these systems.
2. Automated metadata collection #
Manual metadata entry is time-consuming and error-prone. Look for tools that can automatically extract metadata from data sources, track data lineage, and update the metadata repository in real time.
3. Scalability #
As your data grows, your metadata management solution should be able to scale to handle the increasing volume, velocity, and variety of data and metadata.
4. Search and discovery features #
The tool should provide powerful search and discovery features that allow users to easily find relevant data based on various metadata attributes. It should also support semantic search, which allows users to search for data based on its meaning rather than just its name or label.
5. Data governance and compliance support #
Your chosen tool should support data governance tasks, such as managing data access and usage, enforcing data policies, and ensuring compliance with data privacy and protection regulations.
6. Data quality management #
Some metadata management tools offer features to monitor and improve data quality based on metadata. This can be a valuable addition to your data management capabilities.
7. Collaboration features #
The tool should facilitate collaboration among users, such as allowing them to share, comment on, and annotate metadata.
8. Vendor support and community #
Consider the level of support provided by the vendor, as well as the size and activity level of the user community. These can be important resources for troubleshooting and learning best practices.
9. Cost #
Finally, consider the total cost of ownership of the tool, including not just the purchase price, but also the costs of implementation, training, maintenance, and upgrades.
Remember, the best metadata management tool for your data lake initiative is the one that fits your specific needs and constraints. It may be beneficial to conduct a pilot project with a shortlist of tools to see which one works best in your environment.
Bringing it all together #
Metadata management is crucial for the successful operation and utilization of data lakes. Effective metadata management can drive your business growth and bring tangible impact. In this guide, we learnt how metadata management holds the key to unlocking the true potential of a data lake.
Atlan: The next generation of metadata management
Atlan is a third-generation, active metadata platform that unifies metadata from every tool and data domain, creates personalized discovery experiences for every data user, and drives automation across every part of the modern data stack through orchestration.
Metadata management of data lakes: Related reads #
- Metadata Management: Benefits, Automation, Best Practices, and Tools
- What is metadata management and why is it so important?
- Top 6 Metadata Management Best Practices for 2024
- Active Metadata: Definition, Characteristics, Use Cases & More
- Enterprise Metadata Management and Its Importance in the Modern Data Stack
- Snowflake Metadata Management: Importance, Challenges, and Identifying The Right Platform
- Databricks Metadata Management — FAQs, Tools, Getting Started
- DataHub: LinkedIn’s Open-Source Tool for Data Discovery, Catalog, and Metadata Management
- Data Catalog Vs. Metadata Management: Differences, and How They Work Together?
- Difference between Master Data Management(MDM) and Metadata Management
Share this article