LinkedIn DataHub Guide: Setup, Features, and Alternatives (2025)
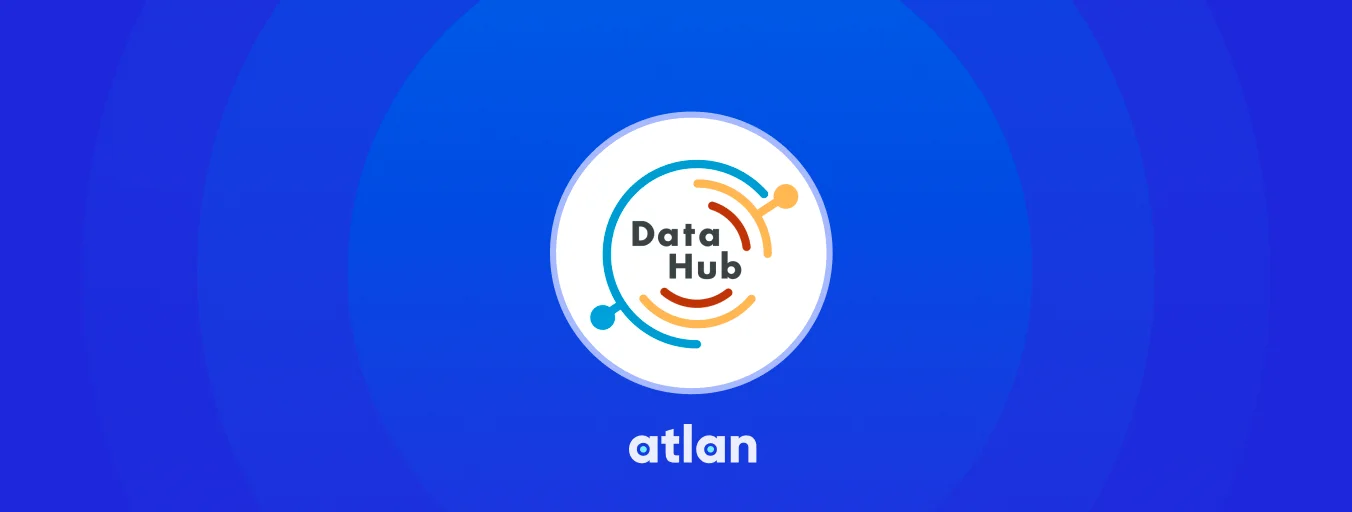
Share this article
LinkedIn DataHub is an open-source metadata platform that empowers organizations to manage, discover, and collaborate on their data assets efficiently.
Designed for scalability, DataHub integrates seamlessly with popular data tools and supports comprehensive data governance, including metadata tracking and data lineage.
See How Atlan Streamlines Metadata Management – Start Tour
Through its collaborative framework, LinkedIn DataHub enables teams to document and visualize data flows, enhancing transparency and compliance across data pipelines.
This robust platform is ideal for enterprises seeking a flexible, community-driven solution to centralize metadata and optimize data management processes.
Quick answer:
Here’s a 2-minute summary on what to expect from this article:
- LinkedIn DataHub is an open-source data cataloging tool that supports data discovery, observability, governance, and metadata management.
- This article aims to give you a sense of the capabilities, architecture, and setup process for LinkedIn DataHub. We also explore viable alternatives — open-source and enterprise data catalogs.
What is DataHub? #
DataHub, built by LinkedIn, is a metadata-powered platform that helps you enable data cataloging, discovery, observability, and governance for your business. It provides you with a 360º view of all your technical and logical metadata, allowing you to find and use all the data at your disposal.
Table of contents #
- What is DataHub?
- Why did LinkedIn build DataHub?
- Capabilities of DataHub
- DataHub Architecture
- How to install DataHub?
- DataHub alternatives
- Related reads
Why did LinkedIn build DataHub? #
LinkedIn has a great track record of building, scaling, and open-sourcing tools and technologies like Kafka, Pinot, Rest.li, Gobblin, and most recently, Venice, among others.
A few years before DataHub, LinkedIn open-sourced WhereHows, which was their “data discovery and lineage platform for the big data ecosystem". With learnings from building and using WhereHows at scale, LinkedIn decided to create DataHub.
A greater degree of chaos can ensue with more sources and data assets, especially when you have complex data pipelines and jobs in place. To prevent that chaos and to ensure that all their data was searchable, discoverable, and understandable, LinkedIn needed to have a comprehensive data catalog with a new architecture that:
- Had a modular, service-oriented design
- Had support for both push-and-pull options for metadata ingestion
- Was able to provide deep integrations with tools in the modern data stack
- Was able to support search and discovery with full-text search
- Had integrated end-to-end granular data lineage
- Had data governance capabilities for data privacy and security
An overview of LinkedIn DataHub
What are some capabilities of DataHub? #
DataHub is primarily LinkedIn’s data catalog that serves for search and discovery use cases. On top of the core data cataloging functions, DataHub also provides features such as fine-grained data lineage, federated data governance, and more. Let’s talk about some of the capabilities of DataHub, LinkedIn’s data cataloging tool:
- Search and discovery
- End-to-end data lineage
- Data governance
1. Search and discovery #
DataHub’s search and discovery capabilities are backed by a full-text search engine that indexes everything from the base technical metadata to the logical metadata, such as tags and classifications.
The search engine is supported by Elasticsearch, which stores all the data in documents that are, in turn, indexed as inverted indexes to enable a super fast search experience. For programmatic access, DataHub also provides access to full-text search, search across entities, and search across lineage via GraphQL.
The search functionality in DataHub is accessible via an intuitive UI that you can use to search all the data assets in DataHub, including asset names, descriptions, ownership information, fine-grain attributes, and so on.
Users who want a more structured approach to search and discovery can use filters and advanced (custom) filters for refining the results from the full-text search. For an even more advanced search query experience, you can use a mix of pattern matching, logical expressions, and filtering.
2. End-to-end data lineage #
DataHub uses file-based lineage to store and ingest data lineage information from various platforms, datasets, pipelines, charts, and dashboards. You need to store the lineage information in the prescribed YAML-based lineage file format.
Here’s an example of a lineage YAML file that contains lineage information for several data assets. DataHub allows integrations like dbt and Superset to ingest lineage data into the system automatically. You can also use Airflow’s Lineage API to ingest the lineage data in DataHub.
Understanding data lineage is highly critical when making changes to code that might break scrips, jobs, DAGs, etc., upstream or downstream. A feature upvoted several times by the DataHub community was that of column-level data lineage, which is extremely important for business users to understand how data gets changed and transformed on its way to the consumption layer.
With its latest release, DataHub has started supporting column-level data lineage. For more information, refer to the documentation for fine-grained lineage.
3. Data governance #
LinkedIn has thought about a number of critical questions around schema preservation, evolution, and annotation. LinkedIn uses a combination of PDL (Pegasus Definition Language) and Avro, which can be used for lossless transformation amongst themselves and can be for storage and streaming purposes.
A lot of other data definition languages, such as SQL-based DDL, Thrift, and Protobuf, can also be amended to support advanced annotations and lossless conversions. Still, LinkedIn has probably chosen these two because of prior experiences on other projects, such as Rest.li, Avro2TF, and Kafka, among others.
This choice not only helps in better data cataloging but also in better data lineage and governance capabilities with the following features:
- Role-based access controls
- Tags, glossary terms, and domains
- Actions Framework
Role-based access controls
DataHub allows a relatively fine-grained method to control access to data assets using a combination of RBAC and the metadata that’s stored in your DataHub system.
For authentication and authorization, DataHub supports a role-based access control mechanism (parts of the initial RFC have been implemented). You can enforce different types of policies to provide varying sets of privileges on different resource types. You can attach these policies to users or groups.
Tags, glossary terms, and domains
On the business side of data governance, DataHub enables better data governance by allowing you to assign assets to owners and use business-specific metadata, such as tags, glossary terms, and domains.
Actions Framework
DataHub’s Actions Framework allows you to enhance your data governance experience by enabling you to trigger external workflows for observability purposes.
Understanding DataHub Architecture #
A blog post on LinkedIn’s engineering blog explains the three generations of metadata applications. It explains how WhereHows came from the first generation of metadata application architecture, and how DataHub became one of the few implementations of the third-generation architecture defined by granular microservices where events and logs power metadata ingestion and consumption.
Three generations of metadata platform architectures #
Here’s a summary of the three generations of metadata platforms, as scoped and defined by LinkedIn:
- First-generation architecture: Crawl-based metadata systems where everything in the platform is served from a monolith containing the frontend, the backend, search, relationships, etc. Examples are Amundsen and Airbnb Data Portal.
- Second-generation architecture: SOA (service-oriented architecture) with a push-based metadata service layer separated from the rest of the stuff but accessible via an API. Examples include Marquez.
- Third-generation architecture: Even more granular service design, with metadata sourced from events and logs from the data sources backed by support for streaming and push-based APIs. Examples include DataHub, Apache Atlas, and Egeria.
Let’s now look at DataHub’s architecture in a bit more detail.
DataHub Architecture Overview #
As prescribed in the specification of the third-generation metadata platform architectures, DataHub uses different services to ingest and serve the metadata.
All the services are integrated with technologies such as GraphQL, REST APIs, and Kafka. For serving different use cases like search, discovery, and governance, DataHub needs to support different data access patterns internally, which, in turn, needs purpose-built databases like MySQL, Elasticsearch, and neo4j.
These three data sources make the serving layer of DataHub and fulfill all the requests from the frontend, API integrations, and other downstream applications.
High level understanding of DataHub architecture. Image source: Atlan.
In the above diagram, you can see DataHub has three broadly-scoped layers, i.e., the sourcing, the serving, and the consumption. The sourcing layer sources data from one or many of the data sources.
Data from these sources is pulled and pushed to the metadata ingestion before being picked up by Kafka to share it with purpose-built data stores in the serving layer. This layer directs the requests to the correct type of data store to ensure the fastest response times.
The consumption layer comprises the business, consuming metadata via the frontend application, and other system integrations consuming data using GraphQL, the REST API, and Kafka.
How to install DataHub? #
Installing DataHub for trying it is very simple. You can install DataHub from the official Docker image and get it up and running in minutes. If you’re looking to deploy DataHub in production, you can deploy it virtually on any cloud platform, such as AWS or Google Cloud with Docker and Kubernetes. Here’s a detailed tutorial on how to get started with DataHub:
DataHub alternatives #
There are several other open-source alternatives to DataHub. Some take very different approaches to address the same problems of search, discovery, and governance. Lyft’s Amundsen and Uber’s Databook-inspired OpenMetadata are two such open-source data cataloging tools. We’ve spent some time comparing these tools comprehensively. Check out the following articles:
Like LinkedIn DataHub? You will love Atlan! #
Comparable to peers like Amundsen (Lyft), Apache Atlas, Metacat (Netflix), etc —LinkedIn DataHub is also an open-source solution that will likely need a significant investment of time and educated efforts to even set up a demo for your team.
While you are evaluating open-source metadata platforms, you can always quickly check out and experience off-the-shelf tools like Atlan.
Watch Atlan Demo: Data Catalog for the Modern Data Stack
How organizations making the most out of their data using Atlan #
The recently published Forrester Wave report compared all the major enterprise data catalogs and positioned Atlan as the market leader ahead of all others. The comparison was based on 24 different aspects of cataloging, broadly across the following three criteria:
- Automatic cataloging of the entire technology, data, and AI ecosystem
- Enabling the data ecosystem AI and automation first
- Prioritizing data democratization and self-service
These criteria made Atlan the ideal choice for a major audio content platform, where the data ecosystem was centered around Snowflake. The platform sought a “one-stop shop for governance and discovery,” and Atlan played a crucial role in ensuring their data was “understandable, reliable, high-quality, and discoverable.”
For another organization, Aliaxis, which also uses Snowflake as their core data platform, Atlan served as “a bridge” between various tools and technologies across the data ecosystem. With its organization-wide business glossary, Atlan became the go-to platform for finding, accessing, and using data. It also significantly reduced the time spent by data engineers and analysts on pipeline debugging and troubleshooting.
A key goal of Atlan is to help organizations maximize the use of their data for AI use cases. As generative AI capabilities have advanced in recent years, organizations can now do more with both structured and unstructured data—provided it is discoverable and trustworthy, or in other words, AI-ready.
Tide’s Story of GDPR Compliance: Embedding Privacy into Automated Processes #
- Tide, a UK-based digital bank with nearly 500,000 small business customers, sought to improve their compliance with GDPR’s Right to Erasure, commonly known as the “Right to be forgotten”.
- After adopting Atlan as their metadata platform, Tide’s data and legal teams collaborated to define personally identifiable information in order to propagate those definitions and tags across their data estate.
- Tide used Atlan Playbooks (rule-based bulk automations) to automatically identify, tag, and secure personal data, turning a 50-day manual process into mere hours of work.
Book your personalized demo today to find out how Atlan can help your organization in establishing and scaling data governance programs.
Linkedin DataHub: Related reads #
- Explore LinkedIn DataHub: A hosted demo environment with pre-populated sample data
- DataHub tutorial: We will guide you through the steps required to configure and install LinkedIn DataHub.
- Amundsen vs DataHub: What is the difference? Which data discovery tool should you choose?
- Open-source data catalog software: 5 popular tools to consider in 2025
- Enterprise metadata management and its importance in the modern data stack
- AI Governance: How to Mitigate Risks & Maximize Business Benefits
- Gartner on AI Governance: Importance, Issues, Way Forward
- Data Governance for AI
- AI Data Governance: Why Is It A Compelling Possibility?
- BCBS 239 2025: Principles for Effective Risk Data Management and Reporting
- BCBS 239 Compliance: What Banks Need to Know in 2025
- BCBS 239 Data Governance: What Banks Need to Know in 2025
- BCBS 239 Data Lineage: What Banks Need to Know in 2025
- HIPAA Compliance: Key Components, Rules & Standards
- CCPA Compliance: 7 Requirements to Become CCPA Compliant
- CCPA Compliance Checklist: 9 Points to Be Considered
- How to Comply With GDPR? 7 Requirements to Know!
- Benefits of GDPR Compliance: Protect Your Data and Business in 2025
- IDMP Compliance: It’s Key Elements, Requirements & Benefits
- Data Governance for Banking: Core Challenges, Business Benefits, and Essential Capabilities in 2025
- Data Governance in Action: Community-Centered and Personalized
- Data Governance Framework — Examples, Templates, Standards, Best practices & How to Create One?
- Data Governance Tools: Importance, Key Capabilities, Trends, and Deployment Options
- Data Governance Tools Comparison: How to Select the Best
- Data Governance Tools Cost: What’s The Actual Price?
- Data Governance Process: Why Your Business Can’t Succeed Without It
- Data Governance and Compliance: Act of Checks & Balances
- Data Governance vs Data Compliance: Nah, They Aren’t The Same!
- Data Compliance Management: Concept, Components, Getting Started
- Data Governance for AI: Challenges & Best Practices
- A Guide to Gartner Data Governance Research: Market Guides, Hype Cycles, and Peer Reviews
- Gartner Data Governance Maturity Model: What It Is, How It Works
- Data Governance Roles and Responsibilities: A Round-Up
- Data Governance in Banking: Benefits, Implementation, Challenges, and Best Practices
- Data Governance Maturity Model: A Roadmap to Optimizing Your Data Initiatives and Driving Business Value
- Open Source Data Governance - 7 Best Tools to Consider in 2025
- Federated Data Governance: Principles, Benefits, Setup
- Data Governance Committee 101: When Do You Need One?
- Data Governance for Healthcare: Challenges, Benefits, Core Capabilities, and Implementation
- Data Governance in Hospitality: Challenges, Benefits, Core Capabilities, and Implementation
- 10 Steps to Achieve HIPAA Compliance With Data Governance
- Snowflake Data Governance — Features, Frameworks & Best practices
- Data Governance Policy: Examples, Templates & How to Write One
- 7 Best Practices for Data Governance to Follow in 2025
- Benefits of Data Governance: 4 Ways It Helps Build Great Data Teams
- Key Objectives of Data Governance: How Should You Think About Them?
- The 3 Principles of Data Governance: Pillars of a Modern Data Culture
Share this article