Data Lake vs Data Swamp: Differences & Cautionary Steps
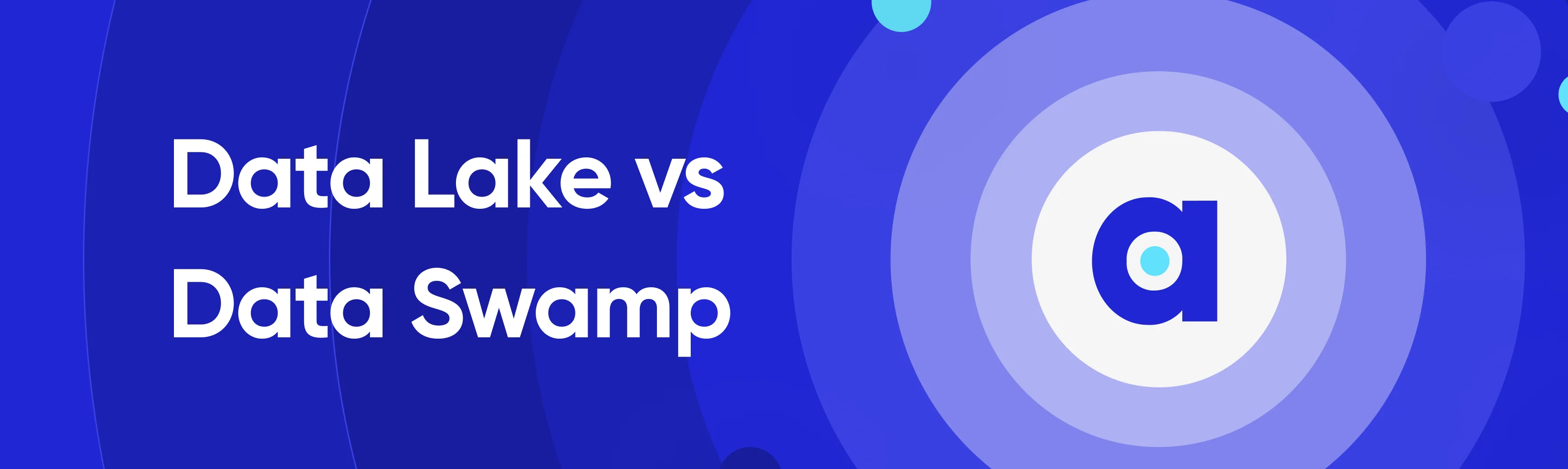
Share this article
Data lake vs. data swamp: The difference #
A data lake is a well-organized and structured storage repository for vast amounts of diverse data, while a data swamp is an unorganized and chaotic data storage system lacking proper structure, making data difficult to retrieve and utilize effectively.
In the world of big data, two concepts often make waves – the data lake and the data swamp. Picture a serene lake, with waters deep and clear, filled with diverse forms of life. This is the data lake, a massive, flexible reservoir brimming with raw, detailed data from various sources. It is an analyst’s treasure trove, offering a wealth of insights waiting to be uncovered.
However, this sparkling image can become clouded if left unchecked. Without proper maintenance, governance, and quality controls, this invaluable data lake can deteriorate into a data swamp.
Understanding the transformation from data lake to data swamp, the causes, the signs, and preventive measures, is a critical expedition for businesses seeking to leverage the power of big data effectively.
Table of contents #
- Data lake vs. data swamp: The difference
- What is a data lake?
- Reasons why they are important
- What is data swamp?
- Common characteristics
- Data lake vs data swamp: A deep dive
- Signs of a data lake turning into a data swamp
- How to avoid data swamps?
- Related reads
What is a data lake? #
A data lake is a storage repository that can store a vast amount of raw data in its native format until it is needed. The data in a data lake could come from various sources like web servers, social media, IoT devices, real-time data feeds, and business applications.
It’s a place where you can dump data of any type and structure, such as structured, semi-structured, and unstructured data, without the need to build a model first or transform the data. In a data lake, you only structure the data when you’re ready to use it, not before. Hence, it provides a lot of flexibility and agility in dealing with a variety of data.
6 Reasons why data lakes are important #
In this section, we will understand six reasons why data lakes are highly important, especially in the era of big data and data-driven decision-making:
- Storage for diverse data
- Scalability
- Agility
- Real-time analytics
- Lower cost
- Advanced analytics capabilities
Let’s quickly look into each one of them.
1. Storage for diverse data #
With the exponential growth of data, including unstructured and semi-structured data from various sources, traditional data storage systems often fall short. Data lakes can store this diverse data effectively.
2. Scalability #
Data lakes are designed to store and manage vast amounts of data. They can scale up and down easily as per the requirements of the organization.
3. Agility #
With a data lake, data is available for exploration by data scientists and analysts without the need to structure it first. This allows for quicker insights and a more explorative approach to data, which can often lead to unexpected discoveries.
4. Real-time analytics #
Data lakes allow data to be ingested in real-time, which enables real-time analytics. This is crucial for many businesses where timely insights matter, such as in stock market trading or e-commerce.
5. Lower cost #
Many data lake solutions are based on open-source technologies, which can lower the cost of storage. The data does not need to be cleaned, transformed, or loaded onto expensive databases, reducing the overall cost.
6. Advanced analytics capabilities #
Data lakes allow businesses to leverage advanced analytics and machine learning capabilities to get deep insights from their data. Data scientists can explore vast datasets to find patterns, correlations, trends, etc., which can guide decision-making.
However, data lakes also come with their own challenges. The most notable is the risk of becoming a “data swamp” – a repository of data that is disorganized, unmanaged, and essentially unusable. Organizations need proper data governance strategies to ensure that data in the lake remains accessible, usable, and secure.
Additionally, because data lakes allow for storage of all types of data, the question of data privacy and compliance with data protection regulations can become a significant concern.
What is data swamp? #
A data swamp is a deteriorated and unmanageable data lake. When a data lake becomes unorganized, cluttered, and difficult to navigate due to the lack of proper data management practices, it is called a data swamp.
This transformation occurs when data management practices are neglected, leading to an accumulation of unstructured and unclassified data. With that being said, let’s look at some characteristics of data swamps.
5 Common characteristics of data swamps #
Here we will delve deeper into the five common characteristics of data swamps and understand the challenges they pose in harnessing the potential of data.
- Lack of metadata
- Poor data quality
- No governance
- Security and compliance issues
- Inefficient use of resources
Let’s look at each one of them.
1. Lack of metadata #
Metadata plays a crucial role in understanding the characteristics and context of data. Without sufficient metadata, users struggle to identify the data’s source, meaning, or relevance. This lack of information hampers data discovery and makes it challenging to utilize the data effectively for analysis and decision-making.
2. Poor data quality #
In a data swamp, data quality deteriorates due to the absence of data governance and management. The data may suffer from inconsistencies, inaccuracies, and incompleteness, leading to unreliable insights. Poor data quality can seriously undermine the trust and credibility of analytical outcomes and hinder data-driven decision-making.
3. No governance #
With no governance policies or processes in place, a data lake can quickly become a data swamp. Data governance refers to the overall management of data availability, usability, integrity, and security. It includes the policies, procedures, and practices necessary to ensure the control and management of data.
4. Security and compliance issues #
In a data swamp, data security becomes a significant concern. Without proper governance and access controls, sensitive information may be exposed, leading to potential data breaches and privacy violations. Non-compliance with data protection regulations can result in legal consequences and reputational damage.
5. Inefficient use of resources #
A data swamp can consume a lot of storage and processing power without providing much value in return. This inefficiency wastes resources and reduces the overall return on investment in the data lake infrastructure.
In short, these common characteristics of data swamps highlight the critical importance of implementing robust data governance and management practices. Without sufficient metadata, data discovery and utilization become challenging, hindering effective analysis and decision-making.
Data lake vs data swamp: A deep dive #
Data lakes and data swamps are concepts that stem from the world of Big Data. Both involve large volumes of data, but they are fundamentally different in their organization, utility, and value to an organization.
Now, let’s understand them clearly by breaking down the differences into key categories.
1. Data quality #
Data lakes have measures in place to maintain high data quality. This includes procedures for checking for duplicate entries, validating the format of the data, and cross-referencing with other data sources for accuracy.
Data swamps lack the mechanisms to ensure data quality, leading to data being inaccurate, inconsistent, or incomplete.
2. Metadata #
Metadata in a data lake is well-managed, making it easy to tag, organize, find, and understand data.
Either the metadata is missing in a data swamp, or it’s poorly organized, making it difficult to understand what data is available and how to use it.
3. Data governance #
Data lakes have robust data governance policies and procedures in place. These procedures ensure the control, management, and security of data in the lake.
Data governance is missing or weak in a data swamp. This results in data disarray, with data being added or changed without control or structure.
4. Access and usability #
Data in a data lake is accessible and easy to use for deriving insights. Data scientists and analysts can find the data they need and trust that it is reliable and accurate.
Data in a data swamp is hard to find and understand, making it nearly unusable for analytics or decision-making.
5. Value to the organization #
A well-managed data lake is a valuable asset to an organization. It can provide the basis for data analytics, machine learning, and data-driven decision-making.
A data swamp, on the other hand, is a liability. It consumes storage and resources without providing valuable insights, and it may even lead to misguided decisions due to poor data quality.
6. Security and compliance #
Data lakes have security measures in place, such as access controls and encryption, and they have procedures for ensuring compliance with data protection regulations.
Data swamps often lack these security measures and compliance procedures, making them vulnerable to data breaches and legal risks.
Here’s a concise tabular comparison of key factors between a data lake and a data swamp for you:
Factors | Data Lake | Data Swamp |
---|---|---|
Data Quality | High data quality maintained with checks and validation. | Data quality is poor, leading to inaccuracies and inconsistency. |
Metadata | Well-managed metadata, facilitating organization and usage. | Lack of metadata or poorly organized, hindering data understanding. |
Data Governance | Robust governance ensuring control, management, and security. | No governance, resulting in data disarray and lack of structure. |
Access and Usability | Data is easily accessible and reliable for analysis. | Data is hard to find and understand, limiting usability. |
Value to the Org. | Valuable asset for data analytics and decision making. | Liability, consuming resources without providing insights. |
Security & Compliance | Security measures and compliance procedures in place. | Vulnerable to breaches and non-compliance risks. |
Remember that the difference between a data lake and a data swamp is largely a matter of management and governance. With the right planning and procedures, a data lake can be a powerful tool for leveraging big data.
What are the signs of a data lake turning into a data swamp? #
It’s important to be able to recognize the signs that a data lake is turning into a data swamp, as early detection can facilitate timely remediation. Here are some key indicators:
- Poor data quality
- Lack of metadata
- Difficulties in accessing and using data
- Lack of governance
- Security concerns
- Decrease in performance
- Non-compliance
Let’s look into each one of the signs briefly:
1. Poor data quality #
One of the first signs of a data swamp is poor data quality. This might include inconsistencies, inaccuracies, or gaps in the data. If data quality issues are not addressed when they arise, they can quickly turn a valuable data lake into a swamp.
2. Lack of metadata #
Metadata is like a guide to your data lake. If the metadata - information about the data such as its source, type, when it was ingested, etc., - is not properly maintained, it can be a sign that the data lake is becoming a swamp. Without metadata, finding and understanding data in the lake can become a significant challenge.
3. Difficulties in accessing and using data #
If users are having difficulties accessing the data they need, or if they find that the data is not usable once they do access it, this is a clear sign of a problem. This could be due to poor data organization, poor data quality, or a lack of metadata.
4. Lack of governance #
Governance includes rules, processes, and controls for how data is added, used, updated, and deleted in the data lake. If governance is lacking or ineffective, the data lake could quickly become a swamp.
5. Security concerns #
A secure data lake has measures in place to protect data and control access. If there are security breaches, or if the necessary security measures are not in place, the data lake could be at risk.
6. Decrease in performance #
If you notice a decrease in performance, like longer loading or processing times, it could be an indication that the data lake is becoming overloaded and turning into a data swamp.
7. Non-compliance #
If your data lake is not in compliance with relevant regulations - for example, regulations related to data privacy - it’s a sign of a data swamp. Non-compliance can lead to legal consequences and damages to the organization’s reputation.
By keeping an eye out for these signs, organizations can identify issues early and take steps to prevent their data lake from becoming a data swamp. It’s essential to implement robust data governance strategies, ensure data quality, manage metadata effectively, maintain appropriate security measures, and regularly monitor performance and compliance.
How to avoid data swamps? #
With strategic planning and implementation of best practices, businesses can steer clear of data swamps and ensure their data lakes remain valuable assets. So, let’s explore six effective ways to avoid data swamps and maintain a well-managed and reliable data ecosystem.
Here they are:
- Develop a data strategy
- Implement data governance
- Maintain metadata
- Ensure data quality
- Implement security measures
- Regular maintenance and monitoring
Now, let’s explore these ways in detail.
1. Develop a data strategy #
Every organization should develop a comprehensive strategy outlining the purpose and methodology for data collection, storage, and utilization. It should include details about the types of data that need to be stored, who will be using it, the frequency of data update, and the retention policy.
The data strategy should align with the organization’s business objectives and clearly demonstrate how data-driven insights can help achieve these objectives.
2. Implement data governance #
Data governance involves setting up a framework for data management and usage across an organization. This includes deciding on data access permissions, maintaining data quality, managing data assets, and ensuring legal compliance.
Having a data governance policy in place helps to ensure that the data is managed in a structured manner, making it easy to access, understand, and secure. It also involves assigning roles and responsibilities to individuals or teams for maintaining and governing the data.
3. Maintain metadata #
Metadata provides essential information about the data like its origin, structure, content, and format. Metadata management ensures that all data in the data lake is cataloged and indexed correctly, which makes it easier to locate and use.
Without appropriate metadata, it can be almost impossible to understand and use the data effectively, leading to a data swamp.
4. Ensure data quality #
Data quality management involves assessing, improving, and maintaining the quality of data. This includes making sure the data is accurate, consistent, complete, reliable, and timely. Poor data quality can lead to unreliable insights, and misguided business decisions, and could even result in compliance issues.
Data quality should be regularly checked and validated to ensure it meets the quality criteria.
5. Implement security measures #
Data security is paramount in preventing unauthorized access, leaks, and breaches. This could involve measures like encryption for data at rest and in transit, managing access controls and permissions, implementing firewalls, and maintaining data anonymization processes where necessary.
Additionally, regular security audits and penetration testing can help identify vulnerabilities and patch them before they can be exploited.
6. Regular maintenance and monitoring #
Like any system, a data lake requires regular maintenance to ensure it continues to function effectively. This includes tasks like monitoring system performance, checking for errors, patching software, validating data quality, and testing system backups.
Regular maintenance helps to prevent issues that can turn a well-structured data lake into a data swamp.
In a nutshell, effectively managing a data lake involves a blend of strategic planning, governance policies, security measures, and regular monitoring. Without these measures, a data lake can rapidly become a data swamp, thereby negating the potential benefits of a data lake. It’s crucial to remember that the key to successfully implementing a data lake lies in the continual management and governance of the data it stores.
Recap: What have we learnt so far? #
- A data lake is a valuable repository that can store vast amounts of raw data in its native format until it is needed. It offers flexibility and agility in dealing with various data types, making it an essential asset in the era of Big Data and data-driven decision-making.
- However, the lack of proper management and governance can lead to a data swamp, where data quality, metadata, governance, accessibility, and value to the organization diminish significantly.
- To avoid data swamps, organizations must develop a comprehensive data strategy, implement data governance, maintain metadata, ensure data quality, implement robust security measures, and conduct regular maintenance and monitoring.
- By proactively addressing these key areas, businesses can maximize the potential of their data lakes, leveraging the insights they provide to drive strategic decision-making and maintain a well-organized and reliable data ecosystem.
Data lake vs. data swamp: Related reads #
- Data Swamp: Is It Sinking You?
- Data Mart vs Data Lake: What’s Right for Your Team?
- Data Fabric vs Data Lake: What’s Right for You and When?
- Data Mesh vs. Data Lake — Differences & Use Cases
- Data Warehouse vs Data Lake vs Data Lakehouse
Share this article