Data Observability & Data Mesh: How Are They Related?
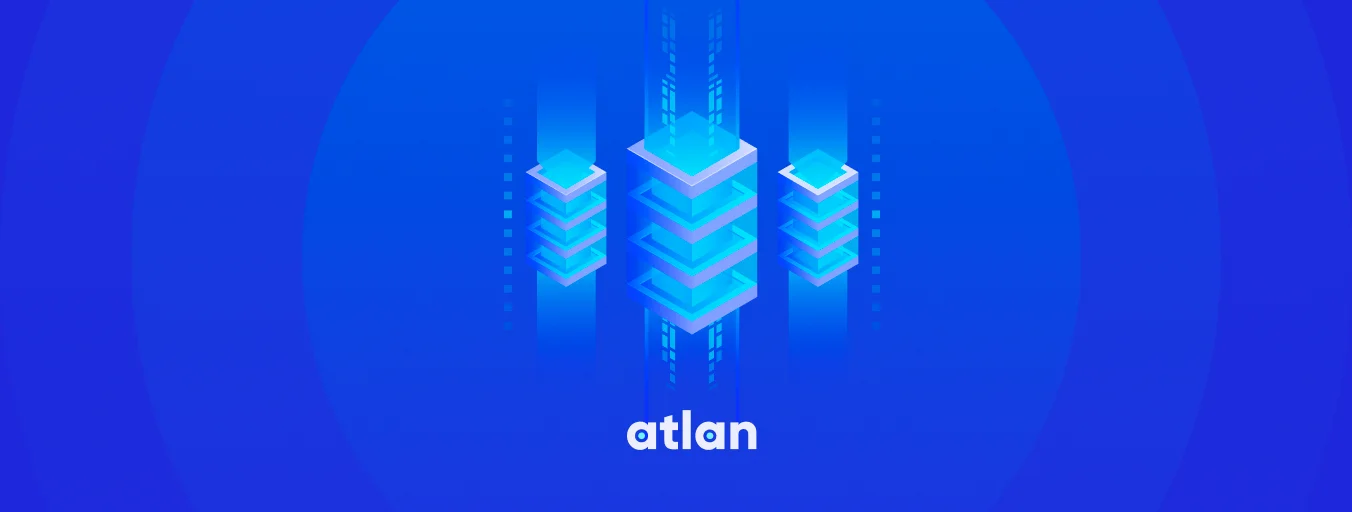
Share this article
In the domain of data management methodologies, two influential concepts have emerged that hold the potential to reshape the manner in which organizations extract value from their data assets: Data observability and data mesh.
They intersect in ways that synergistically enhance the efficacy of modern data-driven decision-making processes. At its core, data observability serves as a vigilant guardian of data integrity, meticulously overseeing the health, quality, and performance of data pipelines, processes, and systems.
The Ultimate Guide to Data Mesh - Learn all about scoping, planning, and building a data mesh 👉 Download now
In parallel, the data mesh framework introduces a paradigm shift in data management, championing a decentralized model wherein data is conceptualized as discrete products. Under this architectural ethos, individual teams or domains assume responsibility for distinct data products, fostering heightened ownership, accessibility, agility, and innovation.
This is where the convergence with data observability manifests most prominently. In this blog, we will dive deeper into data observability, data mesh and the crucial relationship between them in detail.
Let’s dive in!
Table of contents #
- What is data observability & why is it important?
- What is a data mesh & why is it important?
- How are data observability and data mesh related?
- 6 Strategic tips to leverage data observability and data mesh the most!
- Bottom line
- Data observability & data mesh: Related reads
What is data observability & why is it important? #
Data observability refers to the ability to gain insights into the health and quality of a dataset throughout its lifecycle. Just as monitoring and observability have become crucial in software engineering to ensure systems’ reliability, data observability applies similar principles to data.
This means having visibility into your data pipelines, data quality, data lineage, and the operational health of the data infrastructure.
4 Fundamental purposes of data observability #
1. Enhanced data quality #
By actively monitoring datasets, organizations can detect anomalies or irregularities in the data, ensuring its accuracy, consistency, and reliability.
2. Operational efficiency #
Knowing the state and health of your data infrastructure allows for proactive management. This reduces downtime, ensures smooth data pipeline operations, and minimizes the chances of data-related incidents.
3. Build trust #
For data-driven decision-making, stakeholders need to trust the data they are using. With greater visibility into the data’s health and lineage, that trust is reinforced.
4. Faster debugging #
When issues arise, having a comprehensive understanding of the data ecosystem allows for quicker root cause analysis and resolution.
What are the building blocks of data observability? #
Well, there are seven key components of data observability that are integral to fulfilling its purpose. They are:
- Data freshness
- Data lineage
- Data quality metrics
- Anomaly detection
- Volume and throughput
- Operational metrics
- Metadata management
Let’s understand them briefly.
1. Data freshness #
Data freshness involves monitoring the timeliness of data. Ensuring that data is being updated and ingested into systems as expected is crucial. Any lags or delays can impact downstream applications or analytics.
2. Data lineage #
Data lineage provides a visual representation of how data moves and transforms across systems. It offers insights into the origin of data, its transformations, and where it’s consumed, helping trace issues back to their source.
3. Data quality metrics #
This encompasses various metrics like data accuracy, completeness, consistency, and reliability. Monitoring these metrics gives an idea of the overall quality of the dataset.
4. Anomaly detection #
Anomaly detection involves using statistical or machine learning models to detect outliers or anomalies in the data that could indicate potential issues.
5. Volume and throughput #
Monitoring the volume of data and the throughput of data pipelines helps in understanding the load on systems and can give early warnings about potential bottlenecks or failures.
6. Operational metrics #
This includes metrics related to the infrastructure, like system health, uptime, latency, and error rates. It’s crucial to ensure the data infrastructure’s reliability.
7. Metadata management #
Keeping track of metadata, or data about the data, helps in understanding the context, usage, and quality of datasets.
When implementing data observability, it’s essential to use a combination of manual checks, automated monitoring tools, and possibly machine learning models to ensure comprehensive coverage. Integrating these components provides a holistic view of the data ecosystem’s health and ensures that the data’s quality and reliability support the business’s needs.
What is a data mesh & why is it important? #
Data mesh is a decentralized data architecture and organizational approach. It moves away from the traditional centralized data monolith model and instead treats data as a product. In this approach, domain-oriented teams take responsibility for their data, treating it as a product that they offer to other teams within the organization.
4 Fundamental purposes of a data mesh #
1. Decentralization #
Traditional data platforms often struggle to scale, both in terms of technology and organization. Data mesh seeks to address these challenges by decentralizing data ownership and infrastructure.
2. Speed and agility #
By shifting ownership to domain-oriented teams, there’s faster decision-making, and the teams can be more agile in adapting to their specific needs.
3. Improved data quality #
When teams treat data as a product, they inherently put more focus on its quality, reliability, and usability.
4. Enhanced innovation #
With data easily accessible and in a product-oriented manner, teams across the organization can use it innovatively without relying on a central team for all their data needs.
What are the building blocks of a data mesh? #
The following six key components are the vital building blocks of a data mesh.
Here they are:
- Data as a product
- Domain-oriented data ownership
- Self-serve data infrastructure
- Interoperable data products
- Data product discovery and documentation
- Decentralized governance
Let’s understand each one briefly.
1. Data as a product #
This is the core principle of data mesh. Each data product has its lifecycle, from creation to maintenance, and is owned by a specific team.
2. Domain-oriented data ownership #
Rather than a centralized data team owning all data, domain-specific teams (like marketing, sales, or operations) own and manage their data products. They ensure its quality, reliability, and availability to other teams.
3. Self-serve data infrastructure #
Data infrastructure becomes a platform, providing tools and services for domain teams to manage and share their data products. This decentralizes data tooling and allows for customization to specific domain needs.
4. Interoperable data products #
Though data products are decentralized, they need to be interoperable. This means adhering to organizational standards for data formats, quality, and security, ensuring that teams can seamlessly use data products from other domains.
5. Data product discovery and documentation #
With numerous data products across the organization, discoverability becomes crucial. Comprehensive documentation, catalogs, and search tools ensure that teams can easily find and understand the data products available to them.
6. Decentralized governance #
While central data governance models can become bottlenecks, decentralized governance empowers domain teams to make decisions about their data while still adhering to organizational standards and regulations.
Data mesh represents a paradigm shift in how organizations think about and handle their data. It’s a response to the challenges faced by large-scale, centralized data platforms, aiming to make data more accessible, high-quality, and user-focused by decentralizing its management and infrastructure.
How are data observability and data mesh related? #
Even though data observability and data mesh are different concepts in the world of data management, are closely related at their heart. At the heart of both ideas is a commitment to the quality, reliability, and usability of data.
While data mesh decentralizes the ownership and infrastructure of data, treating it as a product, data observability ensures the health, quality, and trustworthiness of this data throughout its lifecycle.
The symbiotic relationship between these two concepts ensures that as organizations distribute their data responsibilities across domains, they maintain rigorous oversight and understanding of their data’s health.
What is observability in data mesh? #
Observability in data mesh refers to the ability to gain detailed insights into the health, quality, and lineage of datasets throughout their lifecycle in a decentralized data architecture.
As organizations transition to treating data as a product within domain-specific teams, data observability ensures consistent monitoring and maintenance of data quality and reliability across these domains.
Diving deeper into the relationship between data observability and data mesh #
The following six factors help us understand the relationship between data observability and data mesh.
1. Data quality and ownership #
Data mesh’s principle of treating data as a product naturally emphasizes the quality of data. As domain teams take ownership of their data products, there’s an inherent responsibility to ensure its quality, completeness, and reliability.
Data observability provides the tools and metrics to gauge and maintain this quality.
2. Decentralization and observability challenges #
As organizations shift from centralized data models to the data mesh model, the complexity of observing data can increase due to multiple sources, formats, and infrastructures.
Data observability tools and practices become even more vital in this decentralized landscape to monitor data health consistently across the organization.
3. Self-serve infrastructure and monitoring #
One of the pillars of data mesh is the self-serve data infrastructure. Similarly, for data observability to be effective in a decentralized system, monitoring tools need to be self-serve too.
Domain teams should have the capability to set up, modify, and monitor their data observability metrics without always relying on a central team.
4. Interoperability and standardization #
While data mesh promotes the decentralization of data products, it emphasizes the interoperability of these products. Data observability plays a role here by setting and monitoring standards.
Ensuring consistent data formats, quality thresholds, and other standards across different domain teams helps maintain the integrity of the overall data ecosystem.
5. Feedback loops and continuous improvement #
With data mesh, domain teams are closer to the data consumers, which facilitates faster feedback loops.
Data observability can enrich these feedback loops by providing detailed insights into potential data issues, allowing teams to iterate and improve their data products quickly.
6. Building trust in decentralized systems #
Trust is pivotal when consuming data from multiple domain teams in a mesh architecture.
Data observability ensures that regardless of which domain the data originates from, its quality, lineage, and freshness can be tracked and verified, fostering trust among data consumers.
In a nutshell, while data mesh sets the stage for how modern organizations should structure and manage their data, data observability provides the necessary oversight to ensure the health and trustworthiness of this distributed data ecosystem. They are intertwined concepts, each amplifying the effectiveness and benefits of the other.
6 Strategic tips to leverage data observability and data mesh the most! #
Leveraging data observability and data mesh effectively requires a strategic approach. Here are the top 6 tips for organizations to make the most of these concepts:
- Foster a data-centric culture
- Implement comprehensive data monitoring tools
- Standardize data practices
- Empower domain teams with training
- Ensure data discoverability
- Continuously review and iterate
Let’s look into each of the above tips in brief:
1. Foster a data-centric culture #
- Why: A shift to a decentralized model like data mesh or incorporating observability requires buy-in from all stakeholders.
- How: Educate teams about the benefits of treating data as a product and the importance of observability. Encourage teams to take ownership of their data and actively participate in observability practices. Celebrate data successes and learn collectively from data challenges.
2. Implement comprehensive data monitoring tools #
- Why: The complexity of data infrastructure in decentralized systems necessitates robust tools to ensure data health.
- How: Choose observability tools that provide detailed insights into data quality, freshness, lineage, and anomalies. Ensure these tools are user-friendly to be adopted by domain teams in the data mesh structure.
3. Standardize data practices #
- Why: With multiple domain teams owning their data products, standardization ensures consistency and interoperability.
- How: Create organizational guidelines on data formats, quality metrics, documentation, and metadata. Regularly review and update these guidelines to adapt to changing needs.
4. Empower domain teams with training #
- Why: For data mesh to be successful, domain teams need the skills to manage their data products effectively.
- How: Offer regular training sessions on data management, observability practices, and the use of relevant tools. Create a community of practice where teams can share experiences and learn from one another.
5. Ensure data discoverability #
- Why: In a decentralized system, easy discoverability of data products is essential to prevent redundancy and improve collaboration.
- How: Implement a data catalog or directory that lists all available data products with comprehensive documentation. Use automated tools to update this catalog in real-time.
6. Continuously review and iterate #
- Why: The landscape of data is dynamic. Regular reviews ensure that the organization’s practices remain relevant and effective.
- How: Set up periodic reviews of data products, observability metrics, and overall data strategy. Incorporate feedback from different stakeholders, including data consumers, to ensure the ecosystem is meeting their needs.
By incorporating these tips, organizations can seamlessly integrate data observability and data mesh into their data strategy, ensuring high-quality, reliable, and easily accessible data across all domains.
Bottom line? #
- As we explore the relationship between data observability and data mesh, a seamless integration becomes evident. Data observability complements data mesh by enhancing data quality, ownership, and discoverability, crucial aspects in a decentralized ecosystem.
- By embracing a data-centric culture, implementing robust monitoring tools, standardizing practices, empowering domain teams through training, ensuring data discoverability, and perpetually reviewing and iterating strategies, organizations can unlock the full potential of Data Observability and Data Mesh.
- These concepts are not merely trends, but strategic imperatives in today’s data landscape, ushering in a future where data is both powerful and trustworthy.
Data observability & data mesh: Related reads #
- Data Observability: Definition, Key Elements, & Benefits
- What is Data Mesh? - Examples, Case Studies, and Usecases
- Best Data Mesh Articles: The Ultimate Guide in 2023
- Data Mesh Setup and Implementation - An Ultimate Guide
- 12 Popular Data Observability Tools in 2023: An Overview
- Data Quality Measures: Best Practices to Implement
- Data Catalog Guide: Examples, What to Look For, and More
- Data Lineage: Everything You Need To Know To Get Started
- Data Management 101: Four Things Every Human of Data Should Know
- What is Data Integrity and Why is It Important?
Share this article