Best Data Mesh Articles: The Ultimate Guide in 2024
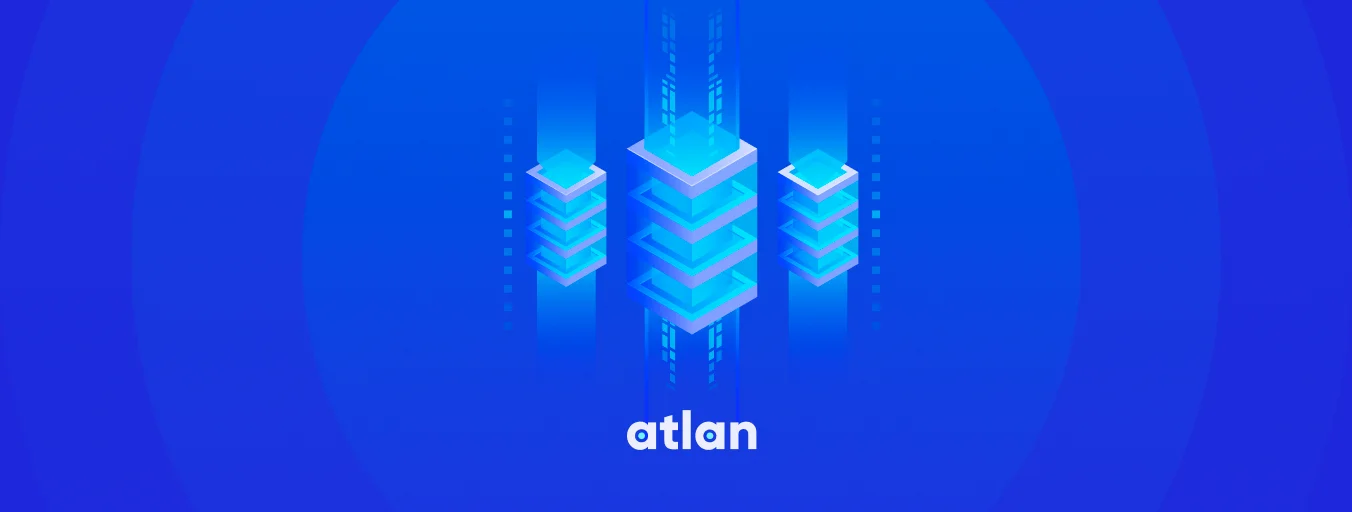
Share this article
In the landscape of modern data architecture, a paradigm shift is underway, and its name is “Data Mesh.” This has been making waves in recent times, redefining how organizations manage and harness their data resources.
As the challenges of traditional data architectures become more apparent, data mesh has emerged as a compelling solution that promises to revolutionize the way we think about data processing and distribution.
The Ultimate Guide to Data Mesh - Learn all about scoping, planning, and building a data mesh 👉 Download now
Let’s delve into the buzz surrounding data mesh and understand why it’s capturing the attention of businesses across industries through the compilation of best-in-class data mesh blogs from Atlan.
Table of contents #
- What is a data mesh?
- Advantages of data mesh
- Principles of data mesh
- Data mesh architecture
- How to set up a data mesh
- Snowflake data mesh
- Data mesh with data governance
- How is data mesh different?
- Related reads
What is a data mesh? #
Data mesh is a decentralized data architecture where data is treated as a product and managed by dedicated data product owners.
The data mesh decentralizes data ownership by transferring the responsibility from the central data team to the business units that create and consume data.
It operates on the principles of domain-driven design, product thinking, and federated governance.
What are the advantages of data mesh? #
The data mesh is a way to resolve data quality, ownership, accountability, and trust issues, which are common with monolithic data architectures.
The top advantages of the data mesh architecture include:
- Greater autonomy and control over your data, leading to faster decision-making
- Product thinking gets embedded everywhere
- Easier data discovery and accessibility
- Greater scalability of data systems with autonomous data domains and teams
- Better data quality when the team creating data is in charge of managing it and extracting value from it
- Interoperability across data domains
- Better regulatory compliance and data security
And if you want to learn more about the fundamentals of metadata, the reason why metadata is the foundation for your data mesh needs, and insightful case studies;
Read more → What is Data Mesh? - Examples, Case Studies, and More!
What are the principles of data mesh? #
Well, there are four fundamental data mesh principles are:
- Domain-oriented decentralized data ownership and architecture
- Data as a product
- Self-serve data infrastructure as a platform
- Federated computational governance
The data mesh and its underlying principles offer the promise of increasing value from data at scale, even in a complex and volatile business context.
But, how?
Get all your questions answered regarding the principles of data mesh once and for all by reading more → Data Mesh Principles — 4 Core Pillars & Logical Architecture
Data mesh architecture: What is it & why does it matter in 2024? #
Well, let’s first understand what data mesh architecture actually is.
The data mesh architecture is a decentralized and scalable approach to data processing and management within an organization. It allows data consumers to access and query data where it lives without transporting it to a data lake or a warehouse.
It challenges the traditional assumption that organizations should put all of their data in a single place — a monolithic architecture — to extract its true value.
All right. But, why does it matter?
Data mesh architecture: Why does it matter? #
Let’s look at several reasons why data mesh architecture matter in 2024.
- It can turbocharge analytics by providing rapid access to fast-growing distributed domain sets.
- The data mesh architecture puts the onus of extracting value from data on the data product owner, which frees up the technologists to pursue strategic tasks that enhance the value of data for the entire organization.
- It facilitates data democratization, empowering every data consumer — data scientists, analysts, business managers — to access, analyze and gain business insights from any data source, without needing help from data engineers.
And the components of data mesh architecture play a crucial role in ensuring:
- Universal interoperability: It’s the key to aggregating and correlating data across domains.
- Governance: A global governance program should map the standards, definitions, policies, and processes around data across domains. Observability: If you cannot observe data, you cannot trust it.
- Domain-agnostic standards: Such standards are essential for ensuring that data definitions are universal, without any room for ambiguity.
Want to learn how? And you also want to learn everything about data mesh architecture in detail?
Then this one is a must-read for you → Data Mesh Architecture: Why It Matters to You in 2024?
Now, let’s understand “how to set up a data mesh!” #
Setting up the data mesh architecture requires you to follow four primary steps.
They are:
- Treat your data as a product
- Map the distribution of domain ownership clearly
- Build a self-serve data infrastructure
- Ensure federated governance
Data mesh is a modern analytics architecture targeted at mid-sized and large organizations. Organizations are keen on implementing the data mesh architecture to move away from a service-oriented view of data.
To learn more about each step involved, click here → Data Mesh Setup and Implementation - An Ultimate Guide
Snowflake and data mesh: What’s the buzz about? #
Ready to dive in?
Let’s begin!
In recent years, Data Mesh has become increasingly popular as a framework for effectively solving the challenges of traditional data architectures. As businesses grow, their data footprint scales rapidly in both volume and complexity. Traditional monolithic data architectures are ill-suited to keep pace with the growing needs of data-driven organizations.
Data Mesh is an alternative architecture that ensures that organizations continue to get value from data at scale, by adopting a decentralized, domain-centric approach to data management.
Data Mesh is a lot more than just a technical architecture. It is a philosophy of data management rooted in distributed ownership, product thinking, and strong governance principles. That being said, one of the basic tenets of a successful Data Mesh is the adoption of a robust, self-service data platform.
It is, therefore, extremely important to choose the right set of technologies for this platform. Doing so will enable data teams to achieve high levels of autonomy, productivity, and operational efficiency with the Data Mesh.
Snowflake Data Cloud is one such platform. Snowflake’s multi-cluster shared data architecture consolidates data warehouses, data marts, and data lakes. This makes it ideal for setting up a self-serve data mesh platform.
And that’s not all!
Check out the link for more → Snowflake Data Mesh: Step-by-Step Setup Guide
How is data mesh connected with data governance? #
Data mesh is a decentralized approach to data architecture and organizational structure, promoting domain-oriented ownership of data products. Data governance is essential in a data mesh to ensure consistency, quality, and security across domains, enabling seamless collaboration and data-driven decision-making throughout the organization.
Now, let’s dive into the steps to implement data mesh!
Step 1: Assess the current data landscape
Step 2: Identify domain teams
Step 3: Establish data product ownership
Step 4: Implement federated data governance
Step 5: Create a data catalog
Step 6: Define data access policies and mechanisms
Step 7: Automate data quality checks and monitoring
Step 8: Develop cross-functional collaboration
Step 9: Measure and iterate
By implementing these steps, you will be able to build a robust data governance framework that supports a data mesh architecture, empowering teams with relevant data and insights, and driving data-driven decision-making across the organization.
Want to learn more about those steps elaborately? Check this out → How to Implement Data Mesh From a Data Governance Perspective?
How is data mesh different from data fabric, data lake, data mart & data warehouse? #
Let’s find that out!
#1 Data mesh vs data fabric #
Data mesh emphasizes data decentralization, autonomy, and productization. In contrast, the data fabric architecture advocates centralization and unified data access. Both are valuable approaches to democratizing data and insights, but they differ in their underlying philosophy and architecture.
Learn more → Data Mesh vs Data Fabric: What Suits You & How to Choose Them?
#2 Data mesh vs data lake #
The main difference between a data mesh and a data lake is that a data mesh is a design strategy for enterprise data platform architecture. Meanwhile, a data lake is a central repository that stores data — structured and unstructured — in a raw format.
Learn more → Data Mesh Vs. Data Lake — Differences & Use Cases For 2024
#3 Data mesh vs data mart #
A data mesh is essentially an approach where instead of a centralized data team owning the end-to-end data pipeline, every domain team owns its own data, end-to-end. On the other hand, a data mart is essentially a repository of data gathered from a variety of sources, intended to assist a particular community of knowledge workers.
Learn more → Data Mesh vs Data Mart - How & Why Are They Different?
#4 Data mesh vs data warehouse #
Data mesh vs Data warehouse both concepts aim to optimize data utilization - they embody contrasting philosophies and architectures. Data warehouse, a long-standing traditional approach, centralizes data into a single repository, emphasizing a top-down, monolithic structure.
Learn more → Data Mesh vs Data Warehouse - How Are They Different?
Bottom line? #
In a nutshell, the buzz around data mesh signifies a monumental shift in the way organizations approach data management and utilization. As this paradigm continues to gain traction, it opens the door to a future where businesses harness data’s true potential through innovation and adaptability. So, what are you waiting for? Check out all the intriguing blogs up there, and stay ahead in this ever-evolving data landscape!
Best data mesh articles: Related reads #
- What is Data Mesh? - Examples, Case Studies, and Use Cases
- Gartner on Data Mesh: Is It The Future of Data Architecture?
- Data Mesh Architecture: Why It Matters to You in 2024?
- Data Mesh Principles — 4 Core Pillars & Logical Architecture
- How to Implement Data Mesh From a Data Governance Perspective
- Data Mesh Setup and Implementation - An Ultimate Guide
- Data Mesh vs Data Fabric: What Suits You the Most
- Data Mesh vs Data Warehouse: How Are They Different?
- Data Mesh vs. Data Lake — Differences & Use Cases
Share this article