Data Observability vs Data Cleansing: 5 Points to Differentiate
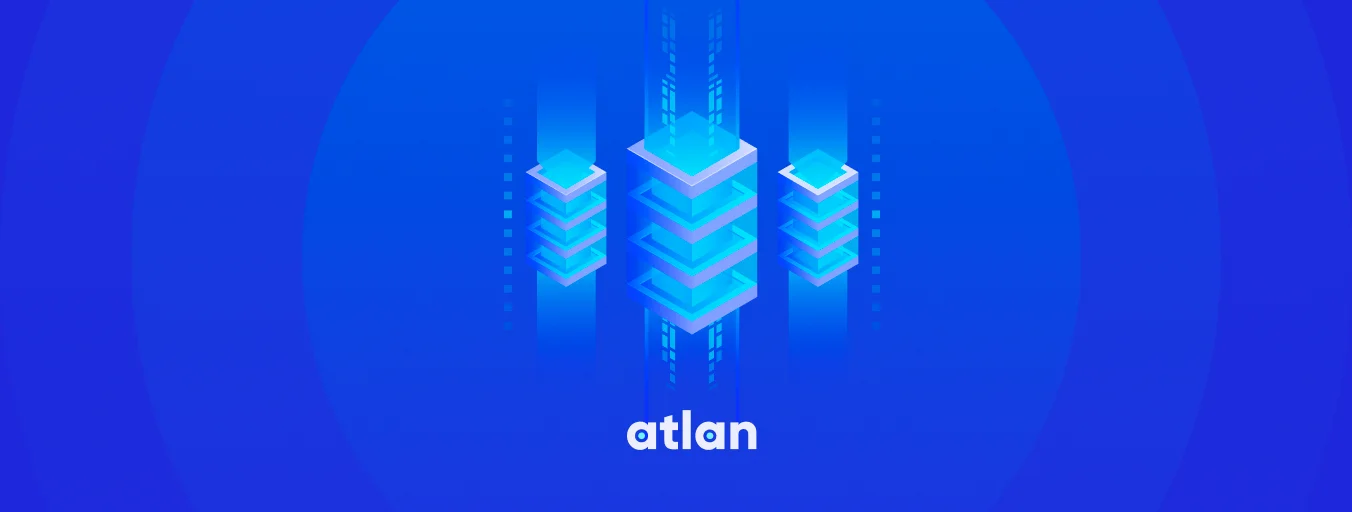
Share this article
Data observability and data cleansing are the two pillars that safeguard the integrity and reliability of the data ecosystem. As organizations navigate the complex landscape of data operations, the distinction between these two concepts is paramount.
In this article, we will learn the fundamental differences between data observability and data cleansing, and unearth their unique roles and significance.
Let’s dive in!
Table of contents #
- Data observability vs data cleansing: Understanding the basics
- What is data cleansing?
- Data observability vs data cleansing: How are they different?
- 5 Ways how data observability and data cleansing related to each other
- Data observability vs data cleansing: 12 Strategic tips to make the most of them
- Summarizing it all together
- Data observability vs data cleansing: Related reads
Data observability vs data cleansing: Understanding the basics #
Data observability and data cleansing are foundational practices that play a pivotal role in shaping the quality and reliability of information.
Let’s first understand what data observability and data cleansing are. Then we will dive into their importance and how they collectively contribute to a robust data-driven ecosystem.
What is data observability and what are its features? #
Data observability is the practice of actively monitoring and gaining real-time insights into the behavior, performance, and quality of data as it moves through various processes within an organization’s data ecosystem.
It involves using specialized tools and techniques to continuously track data flows, transformations, and processing stages. It enables the detection of anomalies, errors, and deviations from expected data patterns as they occur.
Data observability provides organizations with a deeper understanding of how data behaves and performs, facilitating proactive issue identification ensuring the reliability and accuracy of data operations. Its features are:
- Real-time monitoring
- Anomaly detection
- Performance optimization
- Data lineage tracking
- Alerts and notifications
- Visibility into pipelines
Let’s look into each of data observability’s features in brief:
1. Real-time monitoring #
Data observability tools continuously monitor data pipelines and processes, providing immediate insights into data behavior and performance.
2. Anomaly detection #
These tools can detect anomalies, errors, and deviations from expected data patterns as they occur, enabling quick identification and resolution of issues.
3. Performance optimization #
Data observability helps identify bottlenecks and performance issues in data pipelines, contributing to their optimization for efficient data flow.
4. Data lineage tracking #
Observability solutions trace the journey of data through its transformations and processes, facilitating understanding of data movement and transformations.
5. Alerts and notifications #
Real-time alerts and notifications are generated when anomalies or data quality issues are detected, allowing timely corrective actions.
6. Visibility into pipelines #
Data observability offers insights into data flow, transformations, and processing stages, empowering organizations to monitor the health of their data pipelines.
What is data cleansing? #
Data cleansing, also known as data cleaning or data scrubbing, refers to the process of identifying, correcting, and eliminating errors, inaccuracies, inconsistencies, and duplicates within datasets.
The goal of data cleansing is to improve the overall quality and integrity of the data, ensuring that it is accurate, complete, and consistent. This process involves various activities, such as correcting misspelled words, removing duplicate records.
Data cleansing is crucial for ensuring that the data used for analysis, reporting, and decision-making is accurate, reliable, and free from errors that could lead to flawed insights or erroneous conclusions. Here’re the key features of data cleansing:
- Error correction
- Duplicate removal
- Standardization
- Handling missing data
- Validation
- Data integrity
Let’s look at the features of data cleansing in brief:
1. Error correction #
Data cleansing involves identifying and correcting errors in data entries, ensuring accurate and reliable information.
2. Duplicate removal #
Duplicate records are identified and removed from datasets, preventing data redundancy and maintaining a single source of truth.
3. Standardization #
Data cleansing ensures that data is represented in a consistent format, enhancing uniformity across datasets.
4. Handling missing data #
Missing data points are addressed through imputation or other techniques to ensure completeness and accuracy in analysis.
5. Validation #
Data validation checks ensure that data adheres to predefined rules, formats, and constraints, enhancing data accuracy.
6. Data integrity #
The process improves data integrity by rectifying inconsistencies and inaccuracies, leading to reliable insights and decisions.
In summary, data observability focuses on real-time insights and monitoring of data behavior, while data cleansing revolves around improving data quality by identifying and rectifying errors and inconsistencies.
Both practices contribute to maintaining reliable data operations and enhancing the accuracy and integrity of data used for analysis and decision-making.
Data observability vs data cleansing: How are they different? #
Let us delve into the key points that differentiate data observability from data cleansing:
- Focus and purpose
- Timing
- Activities
- Goals
- Use cases
Let us understand each of them in detail.
1. Focus and purpose #
Data observability #
Focus:
- Data observability primarily focuses on monitoring and gaining insights into the behavior, performance, and quality of data as it flows through processes, pipelines, and systems in real-time.
Purpose:
- The primary purpose of data observability is to ensure the reliability, efficiency, and accuracy of data operations.
- It helps organizations identify anomalies, bottlenecks, and data lineages, contributing to data operational excellence.
Data cleansing #
Focus:
- Data cleansing centers around improving the quality of data by identifying and rectifying errors, inaccuracies, inconsistencies, and duplicates within a dataset.
Purpose:
- The main goal of data cleansing is to enhance the overall quality and integrity of the data, ensuring that it is accurate, complete, and consistent. Clean data is essential for accurate analysis and decision-making.
2. Timing #
Data observability #
- Data observability provides insights into data behavior and performance as data flows through pipelines in real-time.
- It offers immediate feedback on data operations, enabling organizations to address issues promptly and proactively.
Data cleansing #
- Data cleansing typically occurs as a separate step before analysis, data integration, or other data-related processes.
- It involves reviewing and cleaning the dataset to prepare it for accurate analysis, decision-making, and other downstream activities.
3. Activities #
Data observability #
- Activities in data observability include real-time monitoring, anomaly detection, performance optimization, and data lineage tracking.
- Observability tools continuously track data flows, detect deviations from expected behavior, and offer insights into data throughput and transformations.
Data cleansing #
- Activities in data cleansing encompass tasks like deduplication (removing duplicate records), correction of inaccuracies (fixing errors), handling missing data, standardization (ensuring uniform formats), and validation (checking adherence to rules and constraints).
4. Goals #
Data observability #
- The main goal of data observability is to ensure that data operations are reliable, efficient, and accurate.
- It helps organizations maintain data quality, identify issues early, and optimize data processes to ensure smooth data flow.
Data cleansing #
- The primary goal of data cleansing is to improve data quality for better decision-making and analysis.
- By removing errors and inconsistencies, data cleansing enhances the accuracy and reliability of insights derived from the data.
5. Use cases #
Data observability #
- Use cases for data observability include real-time monitoring of data pipelines, detecting anomalies in data behavior (such as sudden spikes or drops). It ensures the performance and reliability of data processing.
Data cleansing #
- Data cleansing is used to prepare datasets for analysis, reporting, or integration.
- It’s applied when datasets have inaccuracies, missing data, duplicates, or other quality issues.
Data observability and data cleansing serve different but complementary purposes in the data management lifecycle. Organizations benefit from integrating both practices into their data management strategies to maintain reliable, efficient, and accurate data operations while ensuring the integrity of data used in decision-making processes.
5 Ways how data observability and data cleansing related to each other #
Data observability and data cleansing are related in their contribution to overall data management and quality assurance. While they serve distinct purposes, their integration can greatly enhance an organization’s ability to ensure reliable and high-quality data.
Let’s explore how these two concepts are related to each other:
- Enhancing data quality
- Proactive issue detection and resolution
- Data pipeline optimization
- Enhancing decision-making
- Holistic data management
Let us understand each of them in detail:
1. Enhancing data quality #
Data observability
- Data observability monitors data pipelines and processes in real-time, allowing organizations to detect anomalies, errors, and inconsistencies as they occur.
- By identifying deviations from expected data behavior, observability can highlight potential data quality issues.
Data cleansing
- Data cleansing focuses on identifying and rectifying errors, inaccuracies, and inconsistencies within datasets.
- It directly addresses data quality issues by removing duplicates, correcting inaccuracies, and standardizing data formats.
Integration
- Data observability’s real-time insights can trigger data cleansing processes when anomalies or quality deviations are detected.
- For instance, if a sudden spike in duplicate records is observed, the observability system can initiate data cleansing to remove these duplicates.
2. Proactive issue detection and resolution #
Data observability
- Observability tools detect anomalies, performance bottlenecks, and other data-related issues in real-time.
- It enables proactive identification of issues before they escalate and impact data quality.
Data cleansing
- Data cleansing involves identifying and rectifying errors and inconsistencies in datasets before they are used for analysis or other purposes.
- It ensures that data issues are resolved before they affect downstream processes.
Integration
- Observability’s early detection of anomalies can prompt immediate data cleansing to prevent these anomalies from propagating and affecting data quality across the pipeline.
3. Data pipeline optimization #
Data observability
- Observability helps identify bottlenecks, performance issues, and inefficiencies in data pipelines.
- It contributes to optimizing the flow of data and ensuring smooth operations.
Data cleansing
- Clean data ensures accurate analysis and decision-making, preventing errors caused by data inaccuracies.
Integration
- Data observability identifies bottlenecks, and data cleansing addresses data quality issues, leading to an optimized pipeline that not only performs efficiently but also provides reliable insights.
4. Enhancing decision-making #
Data observability
- Reliable data behavior insights from observability contribute to accurate and confident decision-making.
- It ensures that the data used in decision-making processes is accurate and up-to-date.
Data cleansing
- Clean data improves the accuracy of insights and decisions derived from the data. Decision-makers can trust the data’s quality, leading to more informed choices.
Integration
- Integrating observability insights with the data catalog ensures that decision-makers have access to real-time data behavior information alongside accurate data.
- This is leading to more reliable and confident decisions.
5. Holistic data management #
Data observability
- Observability provides insights into data behavior, pipeline health, and performance metrics. It contributes to maintaining data operational excellence and reliability.
Data cleansing
- Data cleansing contributes to overall data quality and integrity, ensuring that data used in various processes is accurate and consistent.
Integration
- Integrating observability and data cleansing creates a comprehensive data management approach. Data quality is assured both in real-time and in terms of long-term consistency.
Integrating these practices enhances data management by providing real-time insights into data behavior while proactively addressing errors and inconsistencies. This integration strengthens an organization’s ability to harness the full potential of its data for confident decision-making and efficient operations.
Data observability vs data cleansing: 12 Strategic tips to make the most of them #
Leveraging the power of data observability and data cleansing requires a strategic approach to ensure that your organization benefits maximally from these practices.
Here are some strategic tips to make the most of data observability and data cleansing:
- Define clear objectives
- Prioritize critical data streams
- Automation and integration
- Data catalog integration
- Real-time alerts and notifications
- Proactive issue resolution
- Regular data cleansing cycles
- Data ownership and accountability
- Continuous improvement
- Collaboration and training
- Measure and report
- Adapt to changes
Let us understand each of them in detail:
1. Define clear objectives #
Clearly outline the objectives you aim to achieve with data observability and data cleansing, are you looking to:
- Enhance real-time monitoring
- Improve data quality
- Optimize performance
- Ensure data compliance
- Defining your goals will guide your strategies
2. Prioritize critical data streams #
- Identify the most critical data streams, pipelines, or processes that need continuous monitoring and cleansing.
- Focus on areas that impact decision-making, operational efficiency, or regulatory compliance the most.
- Not all data streams or pipelines are equally critical. Identify the ones that have the most significant impact on your organization’s operations, decision-making, or regulatory compliance. Prioritize these for in-depth monitoring and cleansing efforts.
3. Automation and integration #
- Leverage automation and integration capabilities to streamline data observability and data cleansing processes.
- Integrate observability and cleansing tools with your data pipelines to automate anomaly detection and quality improvement.
4. Data catalog integration #
- Integrate data observability insights into your data catalog. This enhances the catalog’s value by adding real-time information about data behavior, quality, and lineage, making it a comprehensive source for informed data usage.
- This integration enriches the catalog’s information with real-time insights about data behavior, quality, and lineage. This holistic view provides users with a comprehensive understanding of the data’s journey and condition.
5. Real-time alerts and notifications #
- Set up real-time alerts and notifications based on observability insights. Immediate notifications about anomalies or issues allow your team to take quick action and mitigate potential problems.
- These alerts ensure that your team is promptly notified when anomalies, performance issues, or data quality concerns arise, enabling quick remediation.
6. Proactive issue resolution #
- Implement proactive practices for resolving issues identified through observability and cleansing. This might involve having dedicated teams or processes in place to address anomalies and data quality concerns promptly.
- Don’t just detect problems; proactively resolve them. Establish processes or teams responsible for addressing issues identified through observability and cleansing. Swift action prevents issues from escalating and impacting operations or decision-making.
7. Regular data cleansing cycles #
- Establish regular data cleansing cycles rather than doing it ad hoc. Create a schedule to periodically review, clean, and validate datasets to maintain consistent data quality.
- Data cleansing should be a recurring practice, not a one-time event. This routine maintenance keeps your data quality consistent over time.
8. Data ownership and accountability #
- Assign data ownership and accountability within your organization. Clearly define roles and responsibilities for data observability and data cleansing to ensure that issues are promptly addressed.
- Designate individuals or teams responsible for monitoring data observability, managing data cleansing processes, and addressing issues as they arise.
9. Continuous improvement #
- Treat data observability and data cleansing as ongoing processes. Continuously review your strategies, tools, and practices to identify areas for improvement and optimize your approach.
- Identify areas for improvement and refine your practices to keep up with evolving data challenges.
10. Collaboration and training #
- Foster collaboration between IT, data teams, business users, and compliance experts. Ensure that all stakeholders understand the significance of data observability and data cleansing and are trained to effectively utilize them.
- Encourage collaboration among different teams. Foster communication between IT, data teams, business users, and compliance experts.
- Provide training to ensure that all stakeholders understand the importance of observability and cleansing and know how to leverage them effectively.
11. Measure and report #
- Establish key performance indicators (KPIs) to measure the impact of data observability and data cleansing.
- Regularly report on these KPIs to demonstrate the value these practices bring to the organization.
- Define key performance indicators (KPIs) to measure the impact of your data observability and cleansing efforts. This data-driven approach helps validate the effectiveness of your strategy.
12. Adapt to changes #
- As your data landscape evolves, adapt your data observability and data cleansing strategies accordingly. New data sources, processes, or technologies might require adjustments to your approach.
- Your data landscape is not static. As your organization evolves, so do your data sources, processes, and technologies.
- Adapt your data observability and cleansing strategies to accommodate these changes, ensuring their continued relevance and effectiveness.
Leveraging data observability and data cleansing strategically involves a combination of setting clear objectives, automating processes, integrating tools, fostering collaboration, and ensuring continuous improvement.
By aligning these practices with your organization’s goals and priorities, you can create a robust data management strategy that enhances data reliability, quality, and operational efficiency.
Summarizing it all together #
Data observability keeps a vigilant eye on data behavior and pipeline health in real-time, ensuring operational excellence and timely anomaly detection. On the other hand, data cleansing dives deep into datasets, rectifying errors, removing inconsistencies, and perfecting data accuracy.
Data observability and data cleansing stand as distinct pillars, each with its unique role in the data landscape. By recognizing their differences and harnessing their synergy, organizations pave the way for data-driven success in an era where the quality of information reigns supreme.
Data observability vs data cleansing: Related reads #
- Data Privacy vs Data Security: How & Why They Aren’t Same?
- Data Management 101: Four Things Every Human of Data Should Know
- What is Data Stewardship?
- Data Quality Measures: Best Practices to Implement
- What is Data Governance? It’s Importance & Principles
- Data Observability: Definition, Key Elements, and Business Benefits
- Data Lineage & Data Observability: Why Are They Important?
- Data Observability & Data Mesh: How Are They Related?
- Data Observability for Data Engineers: What, Why & How
Share this article